- 1Department of Clinical Psychology and Psychotherapy, Institute of Psychology and Education, University Ulm, Ulm, Germany
- 2Research Institute Diabetes Academy Mergentheim (FIDAM), Bad Mergentheim, Germany
- 3Department of Psychological Methods and Assessment, Ludwigs-Maximilian University Munich, Munich, Germany
Background: Mental health problems are prevalent among people with diabetes, yet often under-diagnosed. Smart sensing, utilizing passively collected digital markers through digital devices, is an innovative diagnostic approach that can support mental health screening and intervention. However, the acceptance of this technology remains unclear. Grounded on the Unified Theory of Acceptance and Use of Technology (UTAUT), this study aimed to investigate (1) the acceptance of smart sensing in a diabetes sample, (2) the determinants of acceptance, and (3) the effectiveness of an acceptance facilitating intervention (AFI).
Methods: A total of N = 132 participants with diabetes were randomized to an intervention group (IG) or a control group (CG). The IG received a video-based AFI on smart sensing and the CG received an educational video on mindfulness. Acceptance and its potential determinants were assessed through an online questionnaire as a single post-measurement. The self-reported behavioral intention, interest in using a smart sensing application and installation of a smart sensing application were assessed as outcomes. The data were analyzed using latent structural equation modeling and t-tests.
Results: The acceptance of smart sensing at baseline was average (M = 12.64, SD = 4.24) with 27.8% showing low, 40.3% moderate, and 31.9% high acceptance. Performance expectancy (γ = 0.64, p < 0.001), social influence (γ = 0.23, p = .032) and trust (γ = 0.27, p = .040) were identified as potential determinants of acceptance, explaining 84% of the variance. SEM model fit was acceptable (RMSEA = 0.073, SRMR = 0.059). The intervention did not significantly impact acceptance (γ = 0.25, 95%-CI: −0.16–0.65, p = .233), interest (OR = 0.76, 95% CI: 0.38–1.52, p = .445) or app installation rates (OR = 1.13, 95% CI: 0.47–2.73, p = .777).
Discussion: The high variance in acceptance supports a need for acceptance facilitating procedures. The analyzed model supported performance expectancy, social influence, and trust as potential determinants of smart sensing acceptance; perceived benefit was the most influential factor towards acceptance. The AFI was not significant. Future research should further explore factors contributing to smart sensing acceptance and address implementation barriers.
1 Introduction
Diabetes emerged as one of the most serious and common chronic diseases with approximately half a billion people affected worldwide (1, 2). The metabolic disease has far-reaching implications (3–7), creating an immense burden for affected individuals as well as health care systems (2, 8). Moreover, the prevalence rates of mental health problems are significantly higher among people with diabetes (9). For instance, studies show prevalence rates of comorbid depression in people with diabetes ranging from 12%–27% (10, 11), which is considerably higher compared to the general population. Comorbid mental health problems may not only aggravate the burden associated with diabetes but also give rise to an even greater strain of affected individuals and healthcare systems (12–14). Hence, effective treatments supporting mental health are needed for this population.
Current mental health treatment options for people with diabetes range from face-to-face treatment (15–17) to digital interventions (18, 19). In order to provide optimal and effective treatment, accurate and early detection of mental problems is key. Early detection of symptoms enables affected individuals to take preventive measures and clinicians to intervene appropriately (20–22). However, due to economic constraints and limited resources in health care systems (23, 24), mental health problems often go unrecognized and untreated in general practice (25, 26), thus creating a need for innovative diagnostic methods. One such method could be smart sensing, with the goal of achieving scalable, precise, and time-effective detection of symptoms (27–29). Smart sensing refers to the passive collection of digital markers and features via smartphones and other wearables (30). Tracking usage data from mobile devices as well as built-in sensor data (e.g., GPS, accelerometer, light sensors) offers the possibility to draw conclusions about health status and behaviors of individuals (31, 32). Recent studies support the great potential in the context of mental health (33–38). For example, studies were able to distinguish between individuals with vs. without depressive symptoms based on their GPS data with an accuracy of 86% (39) and could classify depressive symptoms based on sensing variables with an accuracy of 81% (40).
In the daily lives of individuals with diabetes, sensor and tracking technologies already constitute integral components frequently (41). The continuous monitoring and self-regulation necessitated by the metabolic disorder commonly involve the prominent utilization of sensor technology and insulin pumps (42). Within this framework, smartphones take over an increasingly crucial and efficient role as an interface (43–46). Considering the existing prevalence of monitoring and sensing modalities for individuals with diabetes, this demographic may constitute a noteworthy target group for the implementation of smart sensing technologies with a focus on mental health.
However, parallel to integrating innovative diagnostic methods like smart sensing into clinical practice, it is necessary to evaluate its acceptance among people with diabetes as a prerequisite for utilization. The framework for technology acceptance and usage, known as the Unified Theory of Acceptance and Use of Technology (UTAUT) (47), has found extensive application (48, 49). The UTAUT pinpoints four factors influencing acceptance and behavioral intention. Accordingly, the fundamental determinants of acceptance are: (1) performance expectancy (perceived personal advantage gained from using the technology), (2) effort expectancy (expected simplicity of use), (3) social influence (belief that the technology is valuable to others), and (4) facilitating conditions (anticipated assistance and accessibility of tangible resources). This model could serve as a framework for exploring acceptance of smart sensing and its underlying factors. Furthermore, trust has been recognized as a pivotal factor influencing the acceptance of technology and artificial intelligence augmented systems in various application domains (50–52). For example, an individual may receive feedback from a smart sensing system indicating the detection of heightened stress levels and an increased risk of worsening mental symptoms, thus suggesting preventive measures. Depending on the trust in the system, users could either follow the recommended actions or reject them. The first studies that applied the UTAUT framework in the context of smart sensing therefore additionally assessed trust in the technology as a potential facilitator and predictor towards acceptance (53).
Following up on a previous study conducted in a healthy population (53), the present study explores the acceptance of smart sensing a population of people with diabetes to address the following question:
What is the acceptance of smart sensing in the context of mental health for people with diabetes?
Furthermore, the present study seeks to apply the extended UTAUT framework to validate this model in a diabetes sample.
We hypothesize that the UTAUT factors performance expectancy, social influence as well as the factor trust are potential determinates of acceptance of smart sensing.
To successfully implement technologies and ensure uptake and optimal use, it is essential to investigate options to promote the acceptance of said technologies. One way to influence acceptance is using acceptance facilitating interventions (AFI). With a theory-based approach (e.g., UTAUT), AFI target specific factors to influence acceptance—for example, by emphasizing personal gain (performance expectancy) or depicting positive user reports (social influence). Considering possible modalities for AFI, especially video-based AFI offer numerous benefits and have been successfully implemented in the context of blended therapy and internet-based mental health interventions (54, 55). Furthermore, with regard to the situational circumstances suitable for the deployment of AFI, optimizing the time spent in clinical waiting rooms for the implementation of video-based interventions could prove advantageous. Although, AFI have been effectively used in numerous studies (55–58), there has been limited research on the impact in the context of smart sensing as well as in a diabetes population.
Thus, the present study investigates how the acceptance of smart sensing in a diabetes sample is influenced by a UTAUT-based AFI compared to an attention control group.
We hypothesize that (a) the self-reported acceptance, (b) the interest in using a smart sensing app, and (c) the rate of actual installation of a smart sensing app on personal smartphones will be higher in the intervention group compared to the control group.
Lastly, in order to understand the acceptance of smart sensing in a more comprehensive way there are a few factors possibly influencing behavioral intention that should be paid attention to. A meta-analysis of the UTAUT (49) found education to be an important factor influencing behavioral intention (r = 0.18, p < 0.05). Furthermore, a study on personality traits as predictors of perceived and actual usage of technology (59) found significant correlations between behavioral intention to use a technology and conscientiousness (r = 0.15, p < .05) and agreeableness (γ = 0.29, p < .05). Hence, in sensitivity analysis we analyzed the correlations between behavioral intention to use smart sensing and (a) education and (b) personality.
2 Methods and materials
2.1 Study design and sample
A short-term randomized controlled trial with one measurement time point at post-treatment was conducted online to investigate the effect of the AFI. Participants were randomly assigned to either an intervention group (IG) or a control group (CG). The randomized allocation of participants was automatically managed by the online survey platform LimeSurvey. The study was approved by the Ethics Committee of Ulm University (398/21 – CL/bal.). Participants were enrolled from June 1, 2022 till December 31, 2022. Reporting on this study we follow the CONSORT guidelines (60) (see Supplementary S1).
2.2 Inclusion criteria and data collection procedures
The survey, including all procedures and data collection, was conducted online. Participants were recruited via an e-mail list targeting a study panel of people with diabetes diagnosis as well as study flyers. People were eligible to participate if they met the following self-reported inclusion criteria: (1) aged 18 years or older, (2) being diagnosed with diabetes, (3) having internet access, (4) providing informed consent, and (5) agreement to data processing procedures according to the European General Data Protection Regulation. If any criteria were not fulfilled, participation was rejected.
After answering socio-demographic questions, participants were randomized to either the IG or CG and watched the according video (AFI or control video). Although group allocation was not explicitly mentioned, participants were aware of two study conditions due to the informed consent. After watching the video, the acceptance of smart sensing as well as all assumed determinants were assessed. Furthermore, interest in signing up for a smart sensing study and actual installation rate of a smart sensing app was assessed.
2.3 Intervention and control condition
Participants in the intervention group watched a whiteboard based AFI video with a total duration of 4:34 min. The UTAUT model served as a basis for the structure and content of the video. Accordingly, the video focused on the following assumed determinants of acceptance: performance expectancy (e.g., application areas, such as self-monitoring and early recognition of mental health symptoms, personal benefits), effort expectancy (e.g., passive data collection, personal involvement), facilitating conditions (e.g., low necessary personal resources), and social influence (e.g., population-specific positive examples and user reports). Based on previous studies, the video additionally aimed to generate trust in the technology (e.g., data safety, anonymized processing). The AFI video started with a general explanation of smart sensing. Next it delved into which data can be collected via smart sensing as well as the process of data collection. The video further explored different application areas of smart sensing. Lastly, positive user experiences, tailored to a population with diabetes, were presented. A more detailed outline of the AFI can be found in Supplementary S2.
Participants in the control group watched an educational video on the concept of mindfulness, the influence of mindfulness on health, and suggestions on how to integrate mindfulness into one's daily life. The duration of the video was 3:00 min.
2.4 Measures and outcomes
2.4.1 Participant characteristics
Demographic variables (i.e., age, gender, nationality, relationship status, and education level), personality dimensions, general mental health symptoms, and diabetes-specific mental health aspects were assessed using a set of questionnaires.
Basic personality dimensions were assessed with the 10-item version of the Big Five Inventory (BFI-10) (61). The BFI-10 evaluates openness, conscientiousness, extraversion, agreeableness, and neuroticism with a 5-point Likert scale from “fully disagree” to “fully agree”. For evaluation purposes, the mean score was computed for each subscale.
2.4.1.1 Health status
Depression symptoms over the past two weeks were assessed using the 8-item Patient Health Questionnaire (PHQ-8). Using a 4-point Likert scale ranging from “Not at all” to “Nearly every day”, a sum score is computed (range 0–24). Higher scores indicate higher depressive symptoms, and scores of 10 or higher are considered to indicate clinically elevated depressive symptoms (62).
Anxiety symptoms over the last two weeks were assessed with the 7-item Generalized Anxiety Disorder Questionnaire (GAD-7). Items are answered on a 4-point Likert scale from “not at all” to “nearly every day”. Sum scores range from 0 to 21 with higher scores indicating higher anxiety symptoms (63).
Sleeping problems were assessed with the Insomnia Severity Index (ISI-7). The scale consists of seven questions regarding worries, occurrence, and severity of abnormal sleep patterns, and their harmful effects. The questions are answered on a 5-point Likert-scale ranging from 0 to 4. High sum scores (range 0–28) indicate more sleep problems (64).
The Self-Efficacy Scale (SES) assesses self-efficacy using questions on one's perceived personal competence and control. The inventory consists of 10 items answered on a 4-point Likert scale with higher scores (range 10–40) indicating higher self-efficacy (65).
The Fear of Progression Questionnaire Short Form (FoP-Q-SF) assesses worries and fear of disease progression and its consequences using 12 items and a 5-point Likert scale (from “never” to “very often”). Higher total scores (range 12–60) indicate higher fear of progression (66).
To assess the degree of possible participation and hindrance, the Index for the Assessment of Health Impairments (IMET) was used. The IMET consists of nine questions answered on a 11-point Likert scale. Item scores are summed to a total score (range 0–90) indicating the extent of impairment (67).
Emotional distress related to diabetes was assessed using the Diabetes Distress Scale (DDS). The questionnaire consists of 17 items requesting emotional problems related to diabetes in the last 4 weeks. The items can be summed to a total score as well as four subscale scores to evaluate specific levels of distress. The scale scores range from 0 to 6 with higher scores indicating higher distress (68).
The Diabetes Self-Management Questionnaire–Revised (DSMQ-R) is a 20-item battery which assesses diabetes self-care activities aiming to manage glucose levels and prevent long-term complications. For analysis of diabetes self-care, the sum score (range 0–10) was calculated (69).
2.4.2 Acceptance measures
Self-reported acceptance was assessed with the behavioral intention scale of the UTAUT questionnaire (47, 49, 70). The scale consists of four items rating one's intention to use a smart sensing app on a five-point Likert scale (ranging from “fully disagree” to “fully agree”). The sum score was categorized as suggested in previous studies (54–58): low acceptance = sum scores from 4 to 9, moderate acceptance = sum scores from 10 to 15, and high acceptance = sum scores from 16 to 20. Secondly, the level of interest was gauged based on the count and proportion of participants who explicitly expressed a willingness to utilize a smart sensing app. Subsequently, the behavioral outcome was evaluated directly by examining the count and percentage of actual installations of the smart sensing app.
2.4.3 Determinants of acceptance
As potential determinants of acceptance, performance expectancy (3 items), effort expectancy (3 items), social influence (2 items), and facilitating conditions (2 items) were assessed with the UTAUT questionnaire (47, 49, 70). All items were rated on a five-point Likert scale from “fully disagree” to “fully agree”. All UTAUT items can be found in Supplementary S3.
Trust in the technology was assessed with the short form of the German Automation Trust Scale, adapted to the digital health context (50, 71). Seven items are rated on a seven-point Likert scale from “fully disagree” to “fully agree”. The sum score ranges from 7 to 49 with high scores indicating high trust in the technology.
2.5 Statistical analysis
All data analysis followed the per-protocol principle. Participants that dropped out before the randomization or did not receive the intervention as well as were removed. Demographic, mental health, and acceptance-related variables were analyzed using standard descriptive statistics. P-values <0.05 were considered to indicate statistical significance in all analyses.
2.5.1 Acceptance of smart sensing for health
Following previous studies on the acceptance of digital interventions (54–58), the acceptance of smart sensing was assessed as self-reported acceptance, rates of self-reported interest in using smart sensing, and actual installation rates of a smart sensing application (technologically validated via a smart sensing app). The general acceptance of smart sensing is assumed using the acceptance in the CG which did not receive any AFI.
2.5.2 Predictors of acceptance: latent structural equation modeling
The influence of potential determinants of acceptance was investigated using latent structural equation modeling (SEM). A measurement model consisting of latent factors for all items of acceptance, performance expectancy, effort expectancy, facilitating conditions, social influence, and trust was defined as a first step. In the next step, the effects of the latent factors on acceptance were introduced. The proposed predictors performance expectancy, social influence and trust were tested one-sided based on the previous model on acceptance of smart sensing (53).
The root mean square error of approximation (RMSEA) as a non-centrality parameter and the standardized root mean square residual (SRMR) as a residual index were used to assess the goodness of fit (72–74). Acceptable model fit was determined using accepted cut-off guidelines for RMSEA (<0.08) and SRMR (≤0.08) (75–77). Missing date was handled using full information maximum likelihood (78). Robust (Huber-White) standard errors were obtained.
2.5.3 Intervention effects
The effects on acceptance were analyzed on a dimensional level. A t-test was used for the observed data and effects on the latent level were investigated using the SEM, introducing group allocation into the model as a dummy-coded predictor.
2.5.4 Sensitivity analysis
To explore the relationship between education, personality and acceptance we performed correlative sensitivity analysis. Education level (higher values indicating higher education) was summarized according to the International Standard Classification of Education: ISCED-11. Personality was investigated for each subscale (openness, conscientiousness, extraversion, agreeableness, and neuroticism).
2.6 Software
The statistical software R was used for all analyses (79). The R package “lavaan” was used as the core package for all structural equation models (80). See Supplementary S4 for an overview of all packages and versions used in the present analysis.
3 Results
A total of N = 132 individuals provided informed consent, were included in the study, and randomized to their groups (CG: n = 72; IG: n = 60). The study flow is depicted in Figure 1. Participants were between 27 and 81 years of age (M = 57.63, SD = 12.39). Gender was distributed unequally (40.2% female, n = 53). Most participants (60.6%, n = 80) had an advanced qualification level (e.g., bachelor degree and higher). A majority had a diabetes type-1 diagnosis (74.2%, n = 98) compared to a diabetes type-2 diagnosis (25.8%, n = 34). On average, participants reported mental health symptoms below clinical relevance (PHQ-8: M = 5.74, SD = 4.57; GAD-7: M = 4.32, SD = 3.72; ISI-7: M = 7.60, SD = 5.76). Diabetes Distress was M = 1.71 (SD = 0.86), whilst the average diabetes self-management score suggested suboptimal behavior (M = 4.13, SD = 0.51). For further details and group-specific information see Table 1.
3.1 General acceptance of smart sensing
In the CG a total of n = 20 participants (27.8%) reported low, n = 29 (40.3%) moderate, and n = 23 (31.9%) high acceptance (see Figure 2). The unmanipulated self-reported acceptance of smart sensing in the CG was average M = 12.64 (SD = 4.24, Min = 4, Max = 19).
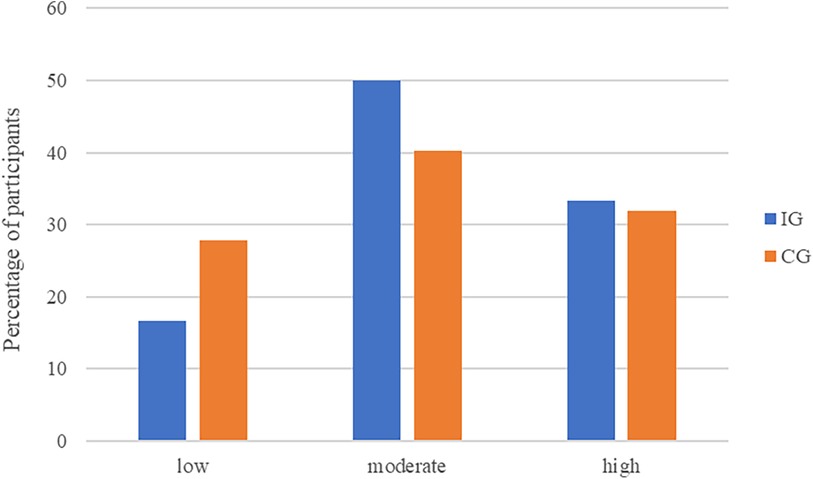
Figure 2. Acceptance of smart sensing levels across treatment groups. The UTAUT behavioral intention sum score categorized acceptance as low (sum score: 4–9), moderate (sum score: 10–15), and high (sum score: 16–20).
A total of n = 36 (50.0%) participants indicated interest in trying out smart sensing in another study (no interest: n = 16, 22.2%; not responded: n = 20, 27.8%). Of all 36 participants with interest, only n = 12 (33.3%; 16.7% of all participants in the CG) installed the smart sensing app.
3.2 Predictor variables associated with acceptance
The final measurement model for acceptance, performance expectancy, effort expectancy, facilitating conditions, social influence, and trust, showed an acceptable fit (RMSEA = 0.074, SRMR = 0.058). See Supplementary S5 for all model parameters.
In the next step, the latent effects on acceptance across groups were analyzed. Performance expectancy (γ = 0.64, p < .001), social influence (γ = 0.23, p = .032) and trust (γ = 0.27, p = .039) were identified as predictor variables of acceptance (overall model fit: RMSEA = 0.073, SRMR = 0.059). Effort expectancy and facilitating conditions were not significant. The three variables explained 83.8% of the variance of the latent acceptance factor. The final path model is displayed in Figure 3. All model parameters are included in Supplementary S6.
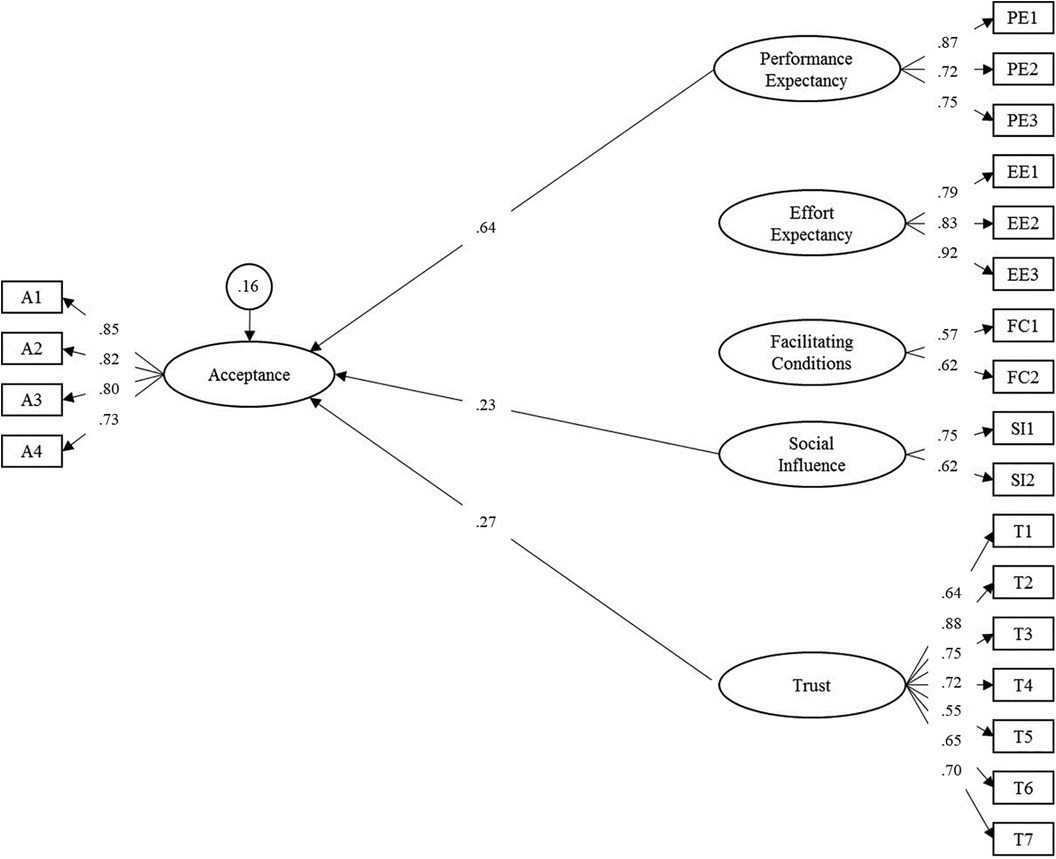
Figure 3. Adapted structural equation model for the acceptance towards smart sensing and associated predictor variables. Latent variables are represented in ellipses: A, acceptance; PE, performance expectancy; EE, effort expectancy; FC, facilitating conditions; SI, social influence; T, trust. Observed items are indicated as rectangles. Regression paths are represented by single-headed arrows. Non-significant paths were deleted in the final model. Residual variances of endogenous latent variables are presented in circles. All exogenous latent variables were allowed to correlate. For improved readability, all latent correlations and residual variances of manifest items were omitted. Please see Supplementary S6 for a full list of all parameters.
3.3 Intervention effects
With an average self-reported acceptance of M = 13.47 (SD = 3.80, Min = 4, Max = 20) in the IG, the level of acceptance of smart sensing was not significantly higher than in the CG (d = 0.20, 95%-CI: −0.14–0.55, t = 1.17, df = 130, p = .244). This result was corroborated by the SEM analysis on latent level (γ = 0.25, 95%-CI: −0.16–0.65, p = .233). The distributions of acceptance levels in the IG and the CG are displayed in Figure 2.
In the IG, n = 26 (43.3%) participants stated interest to try smart sensing in a subsequent study (no interest: n = 20, 33.3%; not responded: n = 14, 23.3%). Among the 26 participants with interest, n = 12 (46.2%; 20.0% of all participants in the IG) actually installed the smart sensing app on their smartphones. The intervention effects and group-specific results are given in Table 2.
3.4 Sensitivity analysis
We found no significant correlation between education and behavioral intention (r = 0.06, p = .513). For personality only conscientiousness significantly correlated with behavioral intention (r = 0.22, p = .031). For the correlation matrix see Supplementary S7.
4 Discussion
We investigated the acceptance of smart sensing and the effect of an AFI towards smart sensing in a diabetes sample. The general acceptance toward smart sensing varied a lot between participants. The hypothetic model of acceptance towards smart sensing with three significant predictors (performance expectancy, social influence and trust) fit the data well, explained 84% variance of the self-reported acceptance, and thereby supported the validity of the model. The UTAUT-based intervention was not able to affect the acceptance of smart sensing.
Given that the treatment guidelines for diabetes recommend yearly and occasion-related diagnosis of common consequential and comorbid diseases including depression and other psychological disorders (81, 82), the integration of smart sensing systems, offering fine-granular, unobtrusive, objective and ecological valid assessments, could function as a form of passive screening support. This has the potential to improve healthcare systems, where resources are often restricted and time-efficient solutions are needed (23, 24). In order to translate encouraging findings from smart sensing studies (37, 38, 83) into tangible healthcare solutions, it is essential to address underlying processes governing both initial and long-term use. This involves a comprehensive understanding of user acceptance and the influencing factors. However, the challenge at hand appears to be two-fold. First, it necessitates a comprehensive examination of general acceptance, and second, it involves addressing the disparity between acceptance and the tangible use of the technology.
This study revealed an average baseline acceptance within our somatic sample (M = 12.64, SD = 4.24) which was higher compared to a healthy population (M = 10.9, SD = 3.73) (53). This inclination could be attributed to the pervasive presence of sensing and monitoring technologies in the daily routines of individuals living with diabetes (42). Although, this trend extended to the installation rates of smart sensing applications, a mere half of the study participants expressed interest in a smart sensing application. Moreover, only 17% and 20% of the CG and IG, respectively, proceeded to install the smart sensing app. Thus, the transfer from intention to use towards actual utilization needs to be addressed in future studies. This seems particularly relevant for the diabetes population, where existing daily self-management must be considered (84). Furthermore, it is important to not only measure the initiation of smart sensing usage but also its continuous use, including frequency and duration. Given that effective smart sensing systems often function as longitudinal assessments, it becomes crucial to explore strategies that foster optimal user performance. Within this context, user engagement and usability play a crucial role, warranting further investigation into personal habits that may facilitate the utilization of smart sensing (85, 86). Additionally, future research could additionally focus on design aspects supporting uptake of smart sensing applications (87, 88) as well specific factors such as potential structural or attitudinal barriers (89).
Consistently with a parallel study involving a healthy population (53), this study identified performance expectancy, social influence, and trust as associated factors of acceptance. Results regarding the hypothetic factor model of smart sensing acceptance and its potential determinants were consistent across these studies, supporting validity of the model. Notably, in our study, performance expectancy was of even greater importance in relation to other potential determinants. This could indicate the importance of addressing crucial needs and pointing out provided benefits of smart sensing.
In this study, the concept of trust was defined as the confidence that a system has the capability to assist in accomplishing an individual's objectives within situations characterized by uncertainty or vulnerability (52). With this understanding, people would show trust by believing in system predictions and following recommendations. However, given that privacy and data security are pivotal considerations in the implementation of smart sensing, future studies should delve into more nuanced distinctions within the realm of trust factors and explore diverse aspects. Smart sensing has the potential to facilitate highly sensitive health predictions, such as mental health screenings. Consequently, it would be beneficial to differentiate between trust in the system, trust in the potential predictions made by the system, and trust in the proper handling of this data. This distinction becomes particularly pertinent in light of political developments, underscoring the need for measures to ensure data privacy, security, and prevention of misuse (90). Moreover, the type of data collected and the entities with whom this data is shared play a crucial role in acceptance. For instance, in a recent study people indicated higher willingness to share sleep data with their physicians than location data, while the acceptance of the inclusion of this data in patient records was rather low in general (91). On a more general note, the public attitude and acceptance of digital health care systems remains a major barrier (53, 92–94). Broader public approaches could play a role in educating and thus leading to familiarization of digital healthcare on a population level. Moving forward, transparency concerning the usage, processing, and storage of data, as well as delineating who has access to the data and who does not, should be emphasized. This could be helpful to fostering user trust and, consequently, enhancing the acceptance of smart sensing technologies.
The implemented AFI did not impact acceptance of smart sensing. Therefore, a pivotal consideration is the improvement of the intervention itself. The current AFI format strategically targeted acceptance determinants based on the UTAUT, supplemented with everyday examples in a whiteboard design. Based on the cognitive theory of multimedia learning, dividing information into verbal and visual components results in reduced cognitive load (95). This could be further improved through a strengthened narrative approach, such as illustrating app functions and presenting extended case examples or short hands-on experience (95–97). Furthermore, expert opinions, as demonstrated in previous (53, 54, 56), might be useful to influence acceptance. It may be worthwhile to explore a mixed modality approach that includes expert opinions tailored to the specific needs and characteristics of the target population, alongside with dynamically visualized content. A similar study on acceptance of smart sensing in psychotherapy patients that focused on information presented by an expert showed promising results for an AFI to influence behavioral intention to use smart sensing (94). Future studies could additionally focus on the effectiveness of interventions in relation to baseline levels of acceptance. Given the above average acceptance in our sample a targeted approach towards individuals with lower levels of baseline acceptance could prove to be more beneficial. This could be implemented using a Solomon four-group study design and looking at interaction effects (98). Consequently, the effectiveness of AFI formats within the realm of smart sensing remains unclear and requires further exploration.
While interpreting the results and discussing future implications, it is crucial to acknowledge several limitations of the present research. First, the trial was critically underpowered to detect a significant intervention effect on acceptance, emphasizing the need for future confirmatory studies with an appropriately sized sample. Second, cross-sectional data are an insufficient basis for uncovering causal relationships. To comprehensively understand the dynamics of smart sensing acceptance and its potential determinants, longitudinal assessments featuring multiple measurement time points are needed. Third, the consideration of common-method bias is necessary, given that acceptance and its predicting variables were evaluated with the same questionnaire. This introduces a source of variance attributable to the measurement method rather than to the constructs, potentially inflating higher variable correlations. To mitigate this, further independent acceptance outcome measures should be considered. The generalization of our findings is further limited as our sample exhibited an overrepresentation of individuals with reported German nationality and high education levels. Moreover, despite covering a broad age range (27–81 years), the relatively high average age (58 years) poses a limitation on the transfer of the findings to a younger population. This is particularly pertinent given the elevated technological affinity and smartphone usage rates among younger individuals (99), potentially leading to distinct differences in the acceptance of smart sensing. Furthermore, the reported mental health symptoms, including depression, anxiety, and diabetes distress, were within a sub-clinical range. Given that performance expectancy emerged as the most strongly associated correlate of acceptance, it is plausible that the perceived personal benefit might be higher for more burdened individuals. These nuances should be taken into account in future research to provide a more comprehensive understanding of smart sensing acceptance across diverse contexts.
5 Conclusions
This study found a heterogenous distribution of acceptance of smart sensing with a relatively large percentage of participant reporting low acceptance, posing a hindrance to the implementation of smart sensing in research and practice. Performance expectancy, social influence, and trust in smart sensing were strongly associated with higher acceptance, suggesting that these aspects may constitute relevant influencing factors towards acceptance. Especially perceived benefit influenced the acceptance amongst the diabetic sample and should be paid special attention in the future. The developed AFI did not affect smart sensing acceptance, thus more effective intervention strategies must be developed. Further exploration of acceptance facilitating interventions on smart sensing are needed. Moving forward, research should look into barriers towards acceptance of smart sensing, which are essential for future implementation in routine health care. The results from this study of people with diabetes furthermore suggest that looking into different somatic areas and groups might detect important individual differences regarding smart sensing acceptance. To fully harness the potential of smart sensing technologies, acceptance, implementation and relevant stakeholders need to be taken into account.
Data availability statement
Data requests should be directed to the corresponding author (JK). Data can be shared with researchers who provide a methodologically sound proposal, which is not already covered by other researchers. Data can only be shared for projects if the General Data Protection Regulation is met. Requestors may need to sign additional data access agreements. Support depends on available resources.
Ethics statement
The studies involving humans were approved by Ethics Commitee of Ulm University. The studies were conducted in accordance with the local legislation and institutional requirements. The participants provided their written informed consent to participate in this study.
Author contributions
JK and YT initiated this study. JK, YT and HB contributed to the study design and concept. JK, YT, and AS contributed to the study recruitment and data collection. JK performed the analysis and drafted the manuscript. All authors had access to all the data in the study, revised the manuscript, approved the final version, and agree to be accountable for the content of the work.
Funding
The author(s) declare that no financial support was received for the research, authorship, and/or publication of this article.
Self-funded by Ulm University.
Conflict of interest
The authors declare that the research was conducted in the absence of any commercial or financial relationships that could be construed as a potential conflict of interest.
Publisher's note
All claims expressed in this article are solely those of the authors and do not necessarily represent those of their affiliated organizations, or those of the publisher, the editors and the reviewers. Any product that may be evaluated in this article, or claim that may be made by its manufacturer, is not guaranteed or endorsed by the publisher.
Supplementary material
The Supplementary Material for this article can be found online at: https://www.frontiersin.org/articles/10.3389/fdgth.2024.1352762/full#supplementary-material
References
1. Sun H, Saeedi P, Karuranga S, Pinkepank M, Ogurtsova K, Duncan BB, et al. IDF diabetes atlas: global, regional and country-level diabetes prevalence estimates for 2021 and projections for 2045. Diabetes Res Clin Pract. (2022) 183:109119. doi: 10.1016/j.diabres.2021.109119
2. Abajobir AA, Abate KH, Abbafati C, Abbas KM, Abd-Allah F, Abdulkader RS, et al. Global, regional, and national disability-adjusted life-years (DALYs) for 333 diseases and injuries and healthy life expectancy (HALE) for 195 countries and territories, 1990–2016: a systematic analysis for the global burden of disease study 2016. Lancet. (2017) 390:1260–344. doi: 10.1016/S0140-6736(17)32130-X
3. Hermanns N, Bremmer M, Snoek F. Diabetes distress. In: Ismail K, Barthel A, Bornstein SR, Licinio J, editors. Depression and Type 2 Diabetes. Oxford: Oxford University Press (2018). p. 85–102.
4. Kulzer B, Lüthgens B, Landgraf R, Hermanns N. Diabetesbezogene belastungen, wohlbefinden und einstellung von menschen mit diabetes. Der Diabetologe. (2015) 11:211–8. doi: 10.1007/s11428-015-1335-8
5. Fisher L, Polonsky WH, Hessler DM, Masharani U, Blumer I, Peters AL, et al. Understanding the sources of diabetes distress in adults with type 1 diabetes. J Diabetes Complications. (2015) 29:572–7. doi: 10.1016/j.jdiacomp.2015.01.012
6. Ismail K, Winkley K, Rabe-Hesketh S. Systematic review and meta-analysis of randomised controlled trials of sychological interventions to improve glycaemic control in patients with type 2 diabetes. Lancet. (2004) 363:1589–97. doi: 10.1016/S0140-6736(04)16202-8
7. Winkley K, Landau S, Eisler I, Ismail K. Psychological interventions to improve glycaemic control in patients with type 1 diabetes: systematic review and meta-analysis of randomised controlled trials. Br Med J. (2006) 333:65. doi: 10.1136/bmj.38874.652569.55
8. Heald AH, Stedman M, Davies M, Livingston M, Alshames R, Lunt M, et al. Estimating life years lost to diabetes: outcomes from analysis of national diabetes audit and office of national statistics data. Cardiovasc Endocrinol Metab. (2020) 9:183–5. doi: 10.1097/XCE.0000000000000210
9. Benton M, Cleal B, Prina M, Baykoca J, Willaing I, Price H, et al. Prevalence of mental disorders in people living with type 1 diabetes: a systematic literature review and meta-analysis. Gen Hosp Psychiatry. (2023) 80:1–16. doi: 10.1016/j.genhosppsych.2022.11.004
10. Holt RIG, de Groot M, Lucki I, Hunter CM, Sartorius N, Golden SH. NIDDK international conference report on diabetes and depression: current understanding and future directions. Diabetes Care. (2014) 37:2067–77. doi: 10.2337/dc13-2134
11. Farooqi A, Gillies C, Sathanapally H, Abner S, Seidu S, Davies MJ, et al. A systematic review and meta-analysis to compare the prevalence of depression between people with and without type 1 and type 2 diabetes. Prim Care Diabetes. (2022) 16:1–10. doi: 10.1016/j.pcd.2021.11.001
12. Ducat L, Philipson LH, Anderson BJ. The mental health comorbidities of diabetes. JAMA. (2014) 312:691. doi: 10.1001/jama.2014.8040
13. Molosankwe I, Patel A, José Gagliardino J, Knapp M, McDaid D. Economic aspects of the association between diabetes and depression: a systematic review. J Affect Disord. (2012) 142:S42–55. doi: 10.1016/S0165-0327(12)70008-3
14. Wallace K, Zhao X, Misra R, Sambamoorthi U. The humanistic and economic burden associated with anxiety and depression among adults with comorbid diabetes and hypertension. J Diabetes Res. (2018) 2018:1–9. doi: 10.1155/2018/4842520
15. Rubin RR, Ciechanowski P, Egede LE, Lin EHB, Lustman PJ. Recognizing and treating depression in patients with diabetes. Curr Diab Rep. (2004) 4:119–25. doi: 10.1007/s11892-004-0067-8
16. van der Feltz-Cornelis CM, Nuyen J, Stoop C, Chan J, Jacobson AM, Katon W, et al. Effect of interventions for major depressive disorder and significant depressive symptoms in patients with diabetes mellitus: a systematic review and meta-analysis. Gen Hosp Psychiatry. (2010) 32:380–95. doi: 10.1016/j.genhosppsych.2010.03.011
17. Safren SA, Gonzalez JS, Wexler DJ, Psaros C, Delahanty LM, Blashill AJ, et al. A randomized controlled trial of cognitive behavioral therapy for adherence and depression (CBT-AD) in patients with uncontrolled type 2 diabetes. Diabetes Care. (2014) 37:625–33. doi: 10.2337/dc13-0816
18. Franco P, Gallardo AM, Urtubey X. Web-based interventions for depression in individuals with diabetes: review and discussion. JMIR Diabetes. (2018) 3:e13. doi: 10.2196/diabetes.9694
19. Bendig E, Bauereiss N, Schmitt A, Albus P, Baumeister H. ACTondiabetes—a guided psychological internet intervention based on acceptance and commitment therapy (ACT) for adults living with type 1 or 2 diabetes: results of a randomised controlled feasibility trial. BMJ Open. (2021) 11:e049238. doi: 10.1136/bmjopen-2021-049238
20. Kramer T, Als L, Garralda ME. Challenges to primary care in diagnosing and managing depression in children and young people. Br Med J. (2015) 350:h2512. doi: 10.1136/BMJ.H2512
21. Wurcel V, Cicchetti A, Garrison L, Kip MMA, Koffijberg H, Kolbe A, et al. The value of diagnostic information in personalised healthcare: a comprehensive concept to facilitate bringing this technology into healthcare systems. Public Health Genomics. (2019) 22:8–15. doi: 10.1159/000501832
22. Kroenke K. Depression screening and management in primary care. Fam Pract. (2018) 35:1–3. doi: 10.1093/FAMPRA/CMX129
23. Trautman S, Beesdo-Baum K. The treatment of depression in primary care—a cross-sectional epidemiological study. Dtsch Arztebl Int. (2017) 114:721–8. doi: 10.3238/ARZTEBL.2017.0721
24. Kroenke K, Unutzer J. Closing the false divide: sustainable approaches to integrating mental health services into primary care. J Gen Intern Med. (2017) 32:404–10. doi: 10.1007/S11606-016-3967-9
25. Nutting PA, Gallagher K, Riley K, White S, Dickinson WP, Korsen N, et al. Care management for depression in primary care practice: findings from the RESPECT-depression trial. Ann Fam Med. (2008) 6:30–7. doi: 10.1370/afm.742
26. Wittchen H-U, Mühlig S, Beesdo K. Mental disorders in primary care. Dialogues Clin Neurosci. (2003) 5:115–28. doi: 10.31887/DCNS.2003.5.2/huwittchen
27. Moshe I, Terhorst Y, Opoku Asare K, Sander LB, Ferreira D, Baumeister H, et al. Predicting symptoms of depression and anxiety using smartphone and wearable data. Front Psychiatry. (2021) 12:625247. doi: 10.3389/fpsyt.2021.625247
28. Opoku Asare K, Terhorst Y, Vega J, Peltonen E, Lagerspetz E, Ferreira D. Predicting depression from smartphone behavioral markers using machine learning methods, hyperparameter optimization, and feature importance analysis: exploratory study. JMIR Mhealth Uhealth. (2021) 9:e26540. doi: 10.2196/26540
29. Hennemann S, Kuhn S, Witthöft M, Jungmann SM. Diagnostic performance of an app-based symptom checker in mental disorders: comparative study in psychotherapy outpatients. JMIR Ment Health. (2022) 9(1):E32832. doi: 10.2196/32832
30. Terhorst Y, Knauer J, Baumeister H. Smart sensing enhanced diagnostic expert systems. In: Montag C, Baumeister H, editors. Digital Phenotyping and Mobile Sensing. 2nd ed. Berlin: Springer (2023). p. 413–25. doi: 10.1007/978-3-030-98546-2_24
31. Garatva P, Terhorst Y, Messner E-M, Karlen W, Pryss R, Baumeister H. Smart sensors for health research and improvement. In: Montag C, Baumeister H, editors. Digital Phenotyping and Mobile Sensing. 2nd ed. Berlin: Springer (2023). p. 395–411. doi: 10.1007/978-3-030-98546-2_23
32. Onnela J-P, Rauch SL. Harnessing smartphone-based digital phenotyping to enhance behavioral and mental health. Neuropsychopharmacology. (2016) 41:1691–6. doi: 10.1038/npp.2016.7
33. Saeb S, Lattie EG, Schueller SM, Kording KP, Mohr DC. The relationship between mobile phone location sensor data and depressive symptom severity. PeerJ. (2016) 4:e2537. doi: 10.7717/peerj.2537
34. Baumeister H, Montag C. Digital Phenotyping and Mobile Sensing. Cham: Springer International Publishing (2019). doi: 10.1007/978-3-030-31620-4
35. Pratap A, Atkins DC, Renn BN, Tanana MJ, Mooney SD, Anguera JA, et al. The accuracy of passive phone sensors in predicting daily mood. Depress Anxiety. (2019) 36:72–81. doi: 10.1002/da.22822
36. Adler Id DA, Wang F, Mohr DC, Choudhury T. Machine learning for passive mental health symptom prediction: generalization across different longitudinal mobile sensing studies. PLoS One. (2022) 17:e0266516. doi: 10.1371/JOURNAL.PONE.0266516
37. Rohani DA, Faurholt-Jepsen M, Kessing LV, Bardram JE. Correlations between objective behavioral features collected from Mobile and wearable devices and depressive mood symptoms in patients with affective disorders: systematic review. JMIR Mhealth Uhealth. (2018) 6:e165. doi: 10.2196/mhealth.9691
38. Benoit J, Onyeaka H, Keshavan M, Torous J. Systematic review of digital phenotyping and machine learning in psychosis Spectrum illnesses. Harv Rev Psychiatry. (2020) 28:296–304. doi: 10.1097/HRP.0000000000000268
39. Saeb S, Zhang M, Karr CJ, Schueller SM, Corden ME, Kording KP, et al. Mobile phone sensor correlates of depressive symptom severity in daily-life behavior: an exploratory study. J Med Internet Res. (2015) 17:1–11. doi: 10.2196/jmir.4273
40. Sarda A, Munuswamy S, Sarda S, Subramanian V. Using passive smartphone sensing for improved risk stratification of patients with depression and diabetes: cross-sectional observational study. JMIR Mhealth Uhealth. (2019) 7:e11041. doi: 10.2196/11041
41. Hunt CW. Technology and diabetes self-management: an integrative review. World J Diabetes. (2015) 6:225. doi: 10.4239/wjd.v6.i2.225
42. Umpierrez GE, Klonoff DC. Diabetes technology update: use of insulin pumps and continuous glucose monitoring in the hospital. Diabetes Care. (2018) 41:1579–89. doi: 10.2337/dci18-0002
43. Grady M, Venugopal U, Robert K, Hurrell G, Schnell O. Health care professionals’ clinical perspectives and acceptance of a blood glucose meter and mobile app featuring a dynamic color range indicator and blood sugar mentor: online evaluation in seven countries. JMIR Hum Factors. (2019) 6:e13847. doi: 10.2196/13847
44. Kirwan M, Vandelanotte C, Fenning A, Duncan MJ. Diabetes self-management smartphone application for adults with type 1 diabetes: randomized controlled trial. J Med Internet Res. (2013) 15:e235. doi: 10.2196/jmir.2588
45. Charpentier G, Benhamou P-Y, Dardari D, Clergeot A, Franc S, Schaepelynck-Belicar P, et al. The diabeo software enabling individualized insulin dose adjustments combined with telemedicine support improves HbA1c in poorly controlled type 1 diabetic patients. Diabetes Care. (2011) 34:533–9. doi: 10.2337/dc10-1259
46. Quinn CC, Clough SS, Minor JM, Lender D, Okafor MC, Gruber-Baldini A. Welldoc TM mobile diabetes management randomized controlled trial: change in clinical and behavioral outcomes and patient and physician satisfaction. Diabetes Technol Ther. (2008) 10:160–8. doi: 10.1089/dia.2008.0283
47. Venkatesh V, Morris MG, Davis GB, Davis FD. User acceptance of information technology: toward a unified view. MIS Q. (2003) 27:425–78. doi: 10.2307/30036540
48. Philippi P, Baumeister H, Ebert DD, Lin J, Messner E-M, Terhorst Y. Acceptance towards digital health care—further development of the UTAUT model. In Preparation. (2021).
49. Blut M, Chong A, Tsiga Z, Venkatesh V. Meta-analysis of the unified theory of acceptance and use of technology (UTAUT): challenging its validity and charting a research agenda in the red ocean. J Assoc Inform Syst. (Forthcoming 2021):1–128.
50. Kraus J, Scholz D, Stiegemeier D, Baumann M. The more you know: trust dynamics and calibration in highly automated driving and the effects of take-overs, system malfunction, and system transparency. Hum Factors. (2020) 62:718–36. doi: 10.1177/0018720819853686
51. Molnar LJ, Ryan LH, Pradhan AK, Eby DW, St. Louis RM, Zakrajsek JS. Understanding trust and acceptance of automated vehicles: an exploratory simulator study of transfer of control between automated and manual driving. Transp Res Part F Traffic Psychol Behav. (2018) 58:319–28. doi: 10.1016/J.TRF.2018.06.004
52. Lee JD, See KA. Trust in automation: designing for appropriate reliance. Hum Factors. (2004) 46:50–80. doi: 10.1518/HFES.46.1.50_30392
53. Terhorst Y, Weilbacher N, Suda C, Simon L, Messner E-M, Sander LB, et al. Acceptance of smart sensing: a barrier to implementation—results from a randomized controlled trial. Front Digit Health. (2023) 5. doi: 10.3389/fdgth.2023.1075266
54. Baumeister H, Terhorst Y, Grässle C, Freudenstein M, Nübling R, Ebert DD. Impact of an acceptance facilitating intervention on psychotherapists’ acceptance of blended therapy. PLoS One. (2020) 15:e0236995. doi: 10.1371/journal.pone.0236995
55. Ebert DD, Berking M, Cuijpers P, Lehr D, Pörtner M, Baumeister H. Increasing the acceptance of internet-based mental health interventions in primary care patients with depressive symptoms. A randomized controlled trial. J Affect Disord. (2015) 176:9–17. doi: 10.1016/j.jad.2015.01.056
56. Baumeister H, Nowoczin L, Lin J, Seifferth H, Seufert J, Laubner K, et al. Impact of an acceptance facilitating intervention on diabetes patients’ acceptance of internet-based interventions for depression: a randomized controlled trial. Diabetes Res Clin Pract. (2014) 105:30–9. doi: 10.1016/j.diabres.2014.04.031
57. Baumeister H, Seifferth H, Lin J, Nowoczin L, Lüking M, Ebert DD. Impact of an acceptance facilitating intervention on Patients’ acceptance of internet-based pain interventions—a randomised controlled trial. Clin J Pain. (2015) 31:528–35. doi: 10.1097/AJP.0000000000000118
58. Lin J, Faust B, Ebert DD, Krämer L, Baumeister H. A web-based acceptance-facilitating intervention for identifying patients’ acceptance, uptake, and adherence of internet- and mobile-based pain interventions: randomized controlled trial. J Med Internet Res. (2018) 20:e244. doi: 10.2196/jmir.9925
59. Barnett T, Pearson AW, Pearson R, Kellermanns FW. Five-factor model personality traits as predictors of perceived and actual usage of technology. Eur J Inf Syst. (2015) 24:374–90. doi: 10.1057/ejis.2014.10
60. Schulz KF, Altman DG, Moher D. CONSORT 2010 statement: updated guidelines for reporting parallel group randomised trials. Br Med J. (2010) 340:c332. doi: 10.1136/bmj.c332
61. Rammstedt B, John OP. Measuring personality in one minute or less: a 10-item short version of the big five inventory in English and German. J Res Pers. (2007) 41:203–12. doi: 10.1016/j.jrp.2006.02.001
62. Kroenke K, Strine TW, Spitzer RL, Williams JBW, Berry JT, Mokdad AH. The PHQ-8 as a measure of current depression in the general population. J Affect Disord. (2009) 114:163–73. doi: 10.1016/j.jad.2008.06.026
63. Spitzer RL, Kroenke K, Williams JBW, Löwe B. A brief measure for assessing generalized anxiety disorder: the GAD-7. Arch Intern Med. (2006) 166:1092–7. doi: 10.1001/archinte.166.10.1092
64. Bastien CH, Vallières A, Morin CM. Validation of the insomnia severity index as an outcome measure for insomnia research. Sleep Med. (2001) 2:297–307. doi: 10.1016/S1389-9457(00)00065-4
65. Schwarzer R, Jerusalem M. SWE. Skala zur Allgemeinen Selbstwirksamkeitserwartung. Trier: Leibniz-Institut für Psychologie (ZPID) (2003).
66. Mehnert A, Herschbach P, Berg P, Henrich G, Koch U. Fear of progression in breast cancer patients–validation of the short form of the Fear of Progression Questionnaire (FoP-Q-SF). Z Psychosom Med Psychother. (2006) 52:274–88. doi: 10.13109/zptm.2006.52.3.274
67. Deck R, Mittag O, Hüppe A, Muche-Borowski C, Raspe H. IMET. Index zur Messung von Einschränkungen der Teilhabe [Verfahrensdokumentation aus PSYNDEX Tests-Nr. 9005870 und Fragebogen]. Trier: Leibniz-Zentrum für Psychologische Information und Dokumentation (ZPID) (2011). doi: 10.23668/psycharchives.381
68. Polonsky WH, Fisher L, Earles J, Dudl RJ, Lees J, Mullan J, et al. Assessing psychosocial distress in diabetes. Diabetes Care. (2005) 28:626–31. doi: 10.2337/diacare.28.3.626
69. Schmitt A, Gahr A, Hermanns N, Kulzer B, Huber J, Haak T. The diabetes self-management questionnaire (DSMQ): development and evaluation of an instrument to assess diabetes self-care activities associated with glycaemic control. Health Qual Life Outcomes. (2013) 11:138. doi: 10.1186/1477-7525-11-138
70. Philippi P, Baumeister H, Apolinário-Hagen J, Ebert DD, Hennemann S, Kott L, et al. Acceptance towards digital health interventions—model validation and further development of the unified theory of acceptance and use of technology. Internet Interv. (2021) 26(100459). doi: 10.1016/j.invent.2021.100459
71. Jian J-Y, Bisantz AM, Drury CG. Foundations for an empirically determined scale of trust in automated systems. Int J Cogn Ergon. (2000) 4:53–71. doi: 10.1207/S15327566IJCE0401_04
72. Browne MW, Cudeck R. Alternative ways of assessing model fit. Sociol Methods Res. (1992) 21:230–58. doi: 10.1177/0049124192021002005
73. Moshagen M. The model size effect in SEM: inflated goodness-of-fit statistics are due to the size of the covariance matrix. Struct Equ Modeling. (2012) 19:86–98. doi: 10.1080/10705511.2012.634724
74. Moshagen M, Erdfelder E. A new strategy for testing structural equation models. Struct Equ Modeling. (2016) 23:54–60. doi: 10.1080/10705511.2014.950896
75. Hu LT, Bentler PM. Cutoff criteria for fit indexes in covariance structure analysis: conventional criteria versus new alternatives. Struct Equ Modeling. (1999) 6:1–55. doi: 10.1080/10705519909540118
76. Bentler PM. Comparative fit indexes in structural models. Psychol Bull. (1990) 107:238–46. doi: 10.1037/0033-2909.107.2.238
77. Steiger JH. Structural model evaluation and modification: an interval estimation approach. Multivariate Behav Res. (1990) 25:173–80. doi: 10.1207/s15327906mbr2502_4
78. Enders CK. Applied Missing Data Analysis. New York: The Guilford Press (2010). ISBN: 9781462549863.
79. R Development Core Team. R: A Language and Environment for Statistical Computing. R Foundation for Statistical Computing (2016). Available online at: https://www.R-project.org/ (Accessed December 1, 2023).
80. Rosseel Y. Lavaan: an R package for structural equation modeling. J Stat Softw. (2012) 48:1–3. doi: 10.18637/jss.v048.i02
81. Bundesärztekammer (BÄK)Kassenärztliche Bundesvereinigung (KBV), Arbeitsgemeinschaft der Wissenschaftlichen Medizinischen Fachgesellschaften (AWMF). Nationale VersorgungsLeitlinie Typ-2-Diabetes—leitlinienreport. Version 3.0. (2023). doi: 10.6101/AZQ/000500
83. Cornet VP, Holden RJ. Systematic review of smartphone-based passive sensing for health and wellbeing. J Biomed Inform. (2018) 77:120–32. doi: 10.1016/j.jbi.2017.12.008
84. Falvo D, Holland BE. Medical and psychosocial aspects of chronic illness and disability. Jones Bartlett Learn. (2017).
85. Salgado T, Tavares J, Oliveira T. Drivers of mobile health acceptance and use from the patient perspective: survey study and quantitative model development. JMIR Mhealth Uhealth. (2020) 8:e17588. doi: 10.2196/17588
86. Baumel A, Yom-Tov E. Predicting user adherence to behavioral eHealth interventions in the real world: examining which aspects of intervention design matter most. Transl Behav Med. (2018) 8:793–8. doi: 10.1093/tbm/ibx037
87. Baumel A, Faber K, Mathur N, Kane JM, Muench F. Enlight: a comprehensive quality and therapeutic potential evaluation tool for mobile and web-based eHealth interventions. J Med Internet Res. (2017) 19:e82. doi: 10.2196/jmir.7270
88. Terhorst Y, Philippi P, Sander LB, Schultchen D, Paganini S, Bardus M, et al. Validation of the mobile application rating scale (MARS). PLoS One. (2020) 15:e0241480. doi: 10.1371/journal.pone.0241480
89. Andrade LH, Alonso J, Mneimneh Z, Wells JE, Al-Hamzawi A, Borges G, et al. Barriers to mental health treatment: results from the WHO world mental health surveys. Psychol Med. (2014) 44:1303–17. doi: 10.1017/S0033291713001943
90. The European Pariliament, The Council Of The European Union. Regulation (EU) 2016/679 on the protection of natural persons with regard to the processing of personal data and on the free movement of such data (general data protection regulation—gDPR). Off J Eur Union. (2018) 2014:958–81. doi: 10.5771/9783845266190-974
91. Nicholas J, Shilton K, Schueller SM, Gray EL, Kwasny MJ, Mohr DC. The role of data type and recipient in Individuals’ perspectives on sharing passively collected smartphone data for mental health: cross-sectional questionnaire study. JMIR Mhealth Uhealth. (2019) 7:e12578. doi: 10.2196/12578
92. Gao S, He L, Chen Y, Li D, Lai K. Public perception of artificial intelligence in medical care: content analysis of social Media. J Med Internet Res. (2020) 22:e16649. doi: 10.2196/16649
93. Montag C, Sindermann C, Baumeister H. Digital phenotyping in psychological and medical sciences: a reflection about necessary prerequisites to reduce harm and increase benefits. Curr Opin Psychol. (2020) 36:19–24. doi: 10.1016/j.copsyc.2020.03.013
94. Rottstädt F, Becker E, Wilz G, Croy I, Baumeister H, Terhorst Y. Enhancing the acceptance of smart sensing in psychotherapy patients: findings from a randomized controlled trial. Front Digit Health. (2024) 6. doi: 10.3389/fdgth.2024.1335776
95. Mayer RE. Multimedia Learning. vol. 41. Academic Press (2002). p. 85–139. doi: 10.1016/S0079-7421(02)80005-6
96. Castro-Alonso JC, Wong M, Adesope OO, Ayres P, Paas F. Gender imbalance in instructional dynamic versus static visualizations: a meta-analysis. Educ Psychol Rev. (2019) 31:361–87. doi: 10.1007/s10648-019-09469-1
97. Schneider S, Krieglstein F, Beege M, Rey GD. Successful learning with whiteboard animations—a question of their procedural character or narrative embedding? Heliyon. (2023) 9:e13229. doi: 10.1016/j.heliyon.2023.e13229
98. Solomon RL. An extension of control group design. Psychol Bull. (1949) 46:137–50. doi: 10.1037/h0062958
Keywords: smart sensing, diabetes mellitus, digital health, acceptance, implementation, UTAUT
Citation: Knauer J, Baumeister H, Schmitt A and Terhorst Y (2024) Acceptance of smart sensing, its determinants, and the efficacy of an acceptance-facilitating intervention in people with diabetes: results from a randomized controlled trial. Front. Digit. Health 6:1352762. doi: 10.3389/fdgth.2024.1352762
Received: 8 December 2023; Accepted: 6 May 2024;
Published: 28 May 2024.
Edited by:
Jennifer Apolinário-Hagen, Heinrich Heine University of Düsseldorf, GermanyReviewed by:
Beenish Chaudhry, University of Louisiana at Lafayette, United StatesSimon Huldreich Kohl, Helmholtz Association of German Research Centres (HZ), Germany
© 2024 Knauer, Baumeister, Schmitt and Terhorst. This is an open-access article distributed under the terms of the Creative Commons Attribution License (CC BY). The use, distribution or reproduction in other forums is permitted, provided the original author(s) and the copyright owner(s) are credited and that the original publication in this journal is cited, in accordance with accepted academic practice. No use, distribution or reproduction is permitted which does not comply with these terms.
*Correspondence: Johannes Knauer, am9oYW5uZXMua25hdWVyQHVuaS11bG0uZGU=