- 1Department of Clinical Psychology, Friedrich Schiller University of Jena, Jena, Germany
- 2DZPG (German Center for Mental Health), Partner Site Halle-Jena-Magdeburg, Jena, Germany
- 3Department of Clinical-Psychological Intervention, Friedrich Schiller University of Jena, Jena, Germany
- 4Department of Clinical Psychology and Psychotherapy, University Ulm, Ulm, Germany
- 5DZPG (German Center for Mental Health), Partner Site Mannheim-Ulm-Heidelberg, Ulm, Germany
- 6Department of Psychological Methods and Assessment, Ludwigs-Maximilian University Munich, Munich, Germany
- 7DZPG (German Center for Mental Health), Partner Site München, Munich, Germany
Objective: Smart sensing has the potential to make psychotherapeutic treatments more effective. It involves the passive analysis and collection of data generated by digital devices. However, acceptance of smart sensing among psychotherapy patients remains unclear. Based on the unified theory of acceptance and use of technology (UTAUT), this study investigated (1) the acceptance toward smart sensing in a sample of psychotherapy patients (2) the effectiveness of an acceptance facilitating intervention (AFI) and (3) the determinants of acceptance.
Methods: Patients (N = 116) were randomly assigned to a control group (CG) or intervention group (IG). The IG received a video AFI on smart sensing, and the CG a control video. An online questionnaire was used to assess acceptance of smart sensing, performance expectancy, effort expectancy, facilitating conditions and social influence. The intervention effects of the AFI on acceptance were investigated. The determinants of acceptance were analyzed with structural equation modeling (SEM).
Results: The IG showed a moderate level of acceptance (M = 3.16, SD = 0.97), while the CG showed a low level (M = 2.76, SD = 1.0). The increase in acceptance showed a moderate effect in the intervention group (p < .05, d = 0.4). For the IG, performance expectancy (M = 3.92, SD = 0.7), effort expectancy (M = 3.90, SD = 0.98) as well as facilitating conditions (M = 3.91, SD = 0.93) achieved high levels. Performance expectancy (γ = 0.63, p < .001) and effort expectancy (γ = 0.36, p < .001) were identified as the core determinants of acceptance explaining 71.1% of its variance. The fit indices supported the model's validity (CFI = .95, TLI = .93, RMSEA = .08).
Discussion: The low acceptance in the CG suggests that enhancing the acceptance should be considered, potentially increasing the use and adherence to the technology. The current AFI was effective in doing so and is thus a promising approach. The IG also showed significantly higher performance expectancy and social influence and, in general, a strong expression of the UTAUT factors. The results support the applicability of the UTAUT in the context of smart sensing in a clinical sample, as the included predictors were able to explain a great amount of the variance of acceptance.
1 Introduction
Digital technologies have the potential to significantly transform psychotherapeutic treatment and care (1–3). The hope is that they can help to bridge healthcare gaps and make treatments more effective and efficient (4–6). Smart sensing is one of those technologies that may contribute to improvements in psychotherapy. It primarily involves the passive analysis and collection of data generated by digital devices, such as smartphones or smart wearables (7, 8). Such data may encompass measurements like step counts, sleep duration, or smartphone usage. In the future, smart sensing could even capture more complex biophysiological data (9). This technology vastly extends the information available to psychotherapists during the treatment process. Smart sensing offers the distinct advantage that fine-grained data (e.g., continuous assessment of activity) can be collected unobtrusively without burden on patients. Furthermore, the technology enables more objective data collection in the natural life context of patients leading to high ecological validity and the elimination of common biases such as recall biases or social desirability, which have long posed challenges in psychotherapy (10, 11). The gathered information can be integrated at every stage of the psychotherapeutic process: diagnosis and problem analysis, treatment planning, implementation of interventions, monitoring, and the evaluation of the treatment process (3, 12).
Data collected through smart sensing has already been utilized in various domains of health research (13–15), such as measuring physical activity or sending activity-promoting app notifications in cases of extended sitting (16), or monitoring chronic conditions like Parkinson's disease (17). In the context of mental health conditions, smart sensing has also been employed (7, 8). From cross-sectional observation studies, there is evidence that mental symptoms are associated with smartphone or wearable data (18–20), which might enable predictions of mental disorders by this data in the future. There is also evidence for phenotyping and diagnosing diseases such as psychosis (21) and bipolar disorder (22) or for mood prediction (23, 24).
However, various steps need to be taken before integrating smart sensing technology into standard clinical care. One is to gauge its acceptability and discern the factors linked with its adoption. The Unified Theory of Acceptance and Use of Technology (UTAUT) (25) offers a model for investigating the acceptance of technology and its influencing factors. It is a well-established framework for understanding the adoption and acceptance of digital health applications (26, 27), and has already been applied to diverse contexts (27–29). The theory identifies performance expectancy, which relates to the perceived personal benefits of using the technology, effort expectancy, denoting the anticipated ease of use, social influence, representing the belief that others find the technology valuable, and facilitating conditions, encompassing the expected support and availability of practical resources, as the fundamental determinants of acceptance (25, 26). A first study also applied the UTAUT model in the context of smart sensing (30). While the study supports the general applicability of the UTAUT model for smart sensing, it was conducted in the general population and currently no evidence of the model is available in patients in psychotherapy. Hence, it is of importance to investigate the generalizability of the UTAUT model in a clinical sample.
In addition to understanding the determinates of acceptance, it is essential to explore opportunities for enhancing the acceptance to ensure the successful implementation of smart sensing. It has been proven before that Acceptance Facilitating Interventions (AFIs) can be effective in enhancing the acceptance of internet-based or blended psychotherapy (31–35). AFIs typically align with an acceptance model such as UTAUT (26) or other models [e.g., the Health Action Process Approach (36)]. To directly target the presumed determinants of acceptance, a UTAUT-based AFI should emphasize performance expectancy by pointing out the personal benefits, effort expectancy by demonstrating the technology in action, social influence by providing expert or user experiences, and facilitating conditions by addressing concerns regarding practical resources or the availability of technical assistance.
The present study aimed to (1) assess the acceptance toward smart sensing in a sample of psychotherapy patients (2) investigate the effectiveness of a UTAUT-based AFI in enhancing the acceptance of smart sensing and (3) investigate the determinants of acceptance. The AFI was presented to the intervention group (IG) in the form of an information video on smart sensing, while the control group (CG) was shown a control video that contained information about depression and anxiety (active control condition). Two hypotheses were investigated: (a) Patients who watched the AFI-video show a higher acceptance of smart sensing. (b) The UTAUT model applies to psychotherapy patients, which means that the covariance matrix implicated by the UTAUT does not differ significantly from the observed covariance matrix. In addition, we conducted exploratory analyses to assess the effect of the AFI on relevant subgroups and to investigate the association between psychological distress and the acceptance of smart sensing.
2 Materials and methods
2.1 Sample and study design
We report on a randomized controlled trial focusing on the cross-sectional comparison of two groups. The study was conducted in April and May, 2023. In this online intervention study patients were randomly assigned to either the control group (CG) or the intervention group (IG). A simple, unrestricted randomization was used, which was carried out by an automated and validated tool by the survey software (LimeSurvey Community Edition Version 6.2.9). The algorithm initially led to different group sizes (IG: 80, CG: 69). Assignment to CG or IG was obscured for participants. Patients in the IG received an informational video (AFI) on the topic of Smart sensing. Patients in the CG, on the other hand, received a video on the topic of depression and anxiety instead. Further details about the videos are provided in Section 2.5.1.
Patients were invited to participate via email. In order to be included in the study, patients either had to be undergoing psychotherapeutic treatment at the psychotherapeutic outpatient clinic of Friedrich-Schiller-University Jena, be on the waiting list for such treatment or have completed their psychotherapy within the last 2 years. Participants also had to have sufficient knowledge of the German language, and be over 18 years of age. Participants did not receive any reimbursements for participation.
To determine the required sample size, an a priori power analysis was conducted. Previous AFI interventions on acceptance ranged from no significant effects to significant effects with large effect sizes (31–34). Based on these study results, the effect size was assumed to be d = 0.40. With a power of 80%, a one-sided t-test and a significance level of 5%, a sample size of 78 participants per group was required.
A total of 433 patients were contacted. Out of the contacted patients, 205 clicked on the study link. The final analysis included data from N = 116 patients, Figure 1 depicts the study flow.
Patient ages ranged from 18 to 87 years (M = 38.86, SD = 15.83). Of the patients, 63.8% were female (n = 74), 33.6% were male (n = 39), and 2.6% identified as gender-diverse (n = 3). Additionally, 39.7% had a university degree (n = 46). There were n = 64 patients in the intervention group and n = 52 in the control group. Table 1 presents sociodemographic data separately for both groups and Table 2 shows that both groups reported the same amount of psychological distress.
2.3 Ethics statement
The study followed the Declaration of Helsinki for medical research involving human subjects and was approved by the Ethics Committee of the Friedrich-Schiller University Jena (Reg.-Nr.: FSV 22/103). All participants provided written informed consent.
2.4 Questionnaires
2.4.1 Acceptance
The assessment of acceptance and its determinants was grounded in the Unified Theory of Acceptance and Use of Technology (UTAUT) model (25). To gauge the efficacy of the intervention and the impact of these determinants, we utilized a slightly modified questionnaire, similar to those employed and validated in prior research endeavors aimed at measuring the acceptance of internet-based interventions (30, 31, 33, 35). The questionnaire comprises 5 scales (acceptance, performance expectancy, effort expectancy, social influence, facilitating conditions) and a total of 14 items. All items were rated on a 5-point Likert scale, ranging from 1 = “strongly disagree” to 5 = “strongly agree.” Detailed information on the questionnaire's scales and items, along with reliability statistics for this study, are presented in the supplement (Supplementary Tables S1 and S3).
2.4.2 Psychological distress
Psychological distress was assessed using the German version of the Depression-Anxiety-Stress Scale (DASS) (37). The questionnaire comprises 21 items and gauges psychological distress across the domains of depression, stress, and anxiety, each consisting of seven items. Responses were captured on a 4-point Likert scale ranging from 0 = “Did not apply to me at all” to 3 = “Applied to me very much, or most of the time.” The DASS was selected due to its economy, ability to provide nuanced insights into various symptom domains of psychological distress, and established reliability and validity. Convergent validity was demonstrated through the correlation between the Beck Anxiety Inventory (38) and the DASS anxiety scale (r = .76), as well as the correlation between the Beck Depression Inventory (39) and the DASS depression scale (r = .68).
2.5 Material
2.5.1 Intervention video
The AFI video had a duration of approximately 9 min. The video aimed to address the acceptance predictors outlined in the UTAUT model, with a particular focus on performance expectancy. The video was tailored to the target audience of psychotherapy patients, implemented a narrative style, and was based on a whiteboard design to achieve load reduction (40–42). Content within the video included an explanation of what smart sensing entails, how it can be utilized, information regarding data collection, data privacy considerations, and the benefits of smart sensing. The potential applications of smart sensing were further elucidated through a fictional patient scenario, highlighting the advantages of smart sensing. In this scenario, a therapist and a patient jointly recognize a positive correlation between physical activity and the patient's satisfaction. Subsequently, an objective is formulated within the therapy to increase physical activity. Furthermore, the video emphasized the use of smart sensing to monitor treatment progress and goal achievement during therapy, devising additional treatment objectives, and underlining the potential utility of smart sensing even beyond the course of therapy. Towards the end of the video, an expert statement from a psychotherapist was presented, expounding upon further advantages of Smart sensing and recounting personal positive experiences with it. A script outlining the video's content is provided in the appendix.
2.5.2 Control video
The control video for the control group was thought as an active control condition. It had a duration of approximately 8.5 min. This video consisted of two psychoeducational segments, one addressing depression and the other focusing on generalized anxiety disorders. Both videos were created by the German Federal Ministry of Health and are publicly available (43, 44). These segments were amalgamated into a single video to ensure a comparable duration to that of the AFI Video. The aim of this video was to capture the participants’ attention due to the perceived importance of the subject matter without influencing their acceptance of smart sensing. Notably, smart sensing was not discussed in this video.
2.6 Implementation and Procedure
Patients initially completed the questionnaire pertaining to their sociodemographic characteristics. Subsequently, patients were presented with either the AFI video or control video. To ensure that the video was viewed, patients were required to wait for a minimum of 4 min before they could click on the “continue” button. Additionally, they were asked to confirm whether they had watched the video in its entirety. Following this, patients received information on smart sensing to ensure that even the CG had a basic understanding of the topic. The following information was provided: “Smart sensing involves the continuous passive collection of digital markers while using smart devices such as smartphones or smartwatches. Digital markers include parameters such as the range of motion, step count, and sleep patterns. Smart sensing, for instance, records your step count via a smartphone or your sleep patterns through a smartwatch.” Following the information session, patients completed the UTAUT questionnaire and the DASS. At the end of the study, participants were also given the option to receive automated feedback on the results of the DASS questionnaire. Furthermore, patients had the opportunity to provide their email address if they were interested in participating in further studies involving smart sensing.
2.7 Statistical analyses
The data was analyzed using IBM SPSS Statistics 29.00 and IBM SPSS Amos 29. To compare level of acceptance between IG and CG, the Mann-Whitney U test was employed due to deviations from the assumption of normal distribution. To examine the influence of the surveyed acceptance predictors (performance expectancy, effort expectancy, social influence, facilitating conditions), a confirmatory structural equation model was applied to the whole sample, whereby the CG and IG were combined. The structural equation model was developed based on UTAUT and validated by Philippi et al. (27). The influence of the acceptance predictors on acceptance was tested using standardized regression weights and their significance. Model fit was evaluated using the Comparative Fit Index (CFI), the Tucker Lewis Index (TLI) and the Root Mean Square Error of Approximation (RMSEA). RMSEA as a non-centrality parameter was used to assess the goodness of fit due to the tendency of the χ2-test to reject the misspecified models too harshly (45–47). Following well-established guidelines we defined a good model fit as CFI and TLI >.90, RMSEA <.08 (48).
Additionally, exploratory t-tests were conducted. The effects of the intervention on various subgroups were also examined to analyze in which groups the intervention is particularly meaningful and to explore potential mechanisms of the intervention. Exploratory analyses were performed for the following subgroups: male vs. female, young vs. old (with the median age as the cutoff point) as well as lower vs. higher educational levels (higher degrees beyond secondary school were considered as higher education). To investigate the association between acceptance and psychological distress, Pearson correlation coefficient was computed.
3 Results
3.1 Group Comparison of acceptance and its predictors
On a 5-point Likert scale, the IG showed a moderate level of acceptance (M = 3.16), while the CG showed a low level (M = 2.76). Table 3 presents the comparison of groups in terms of acceptance and acceptance predictors. The IG showed significantly higher acceptance than CG (U = 1,299.0, Z = −2.033, p = .042). The effect size was d = 0.40 (95% CI: 0.03–0.77), corresponding to a moderate effect. Hence, hypothesis (a) was confirmed. Furthermore, patients in the IG had significantly higher performance expectancy toward smart sensing (U = 2,404.5, Z = −3.575, p < .001), with an effect size of d = 0.74 (95% CI: 0.36–1.11), as well as significantly higher scores on social influence (U = 1,056.0, Z = 3.460, p < .001), with an effect size of d = 0.63 (95% CI: 0.25–1.00).
3.2 Model for prediction of acceptance of smart sensing
Performance expectancy (γ = 0.63, p < .001) and effort expectancy (γ = 0.36, p < .001) were identified as predictors of acceptance in the structural equation model. Together, the two determinants explained 71.1% of the variance of the latent acceptance factor. Social influence did not achieve statistical significance as a predictor of acceptance (γ = −0.08, p = .551). The fit indices supported the model's validity (CFI = .95, TLI = .93, RMSEA = .08). Hence, hypothesis (b) was confirmed. Figure 2 depicts the structural equation model with estimated parameters. The full parameter list of the measurement model and correlations between the acceptance predictors and acceptance of smart sensing are presented in the supplement (Supplementary Tables S2 and S3).
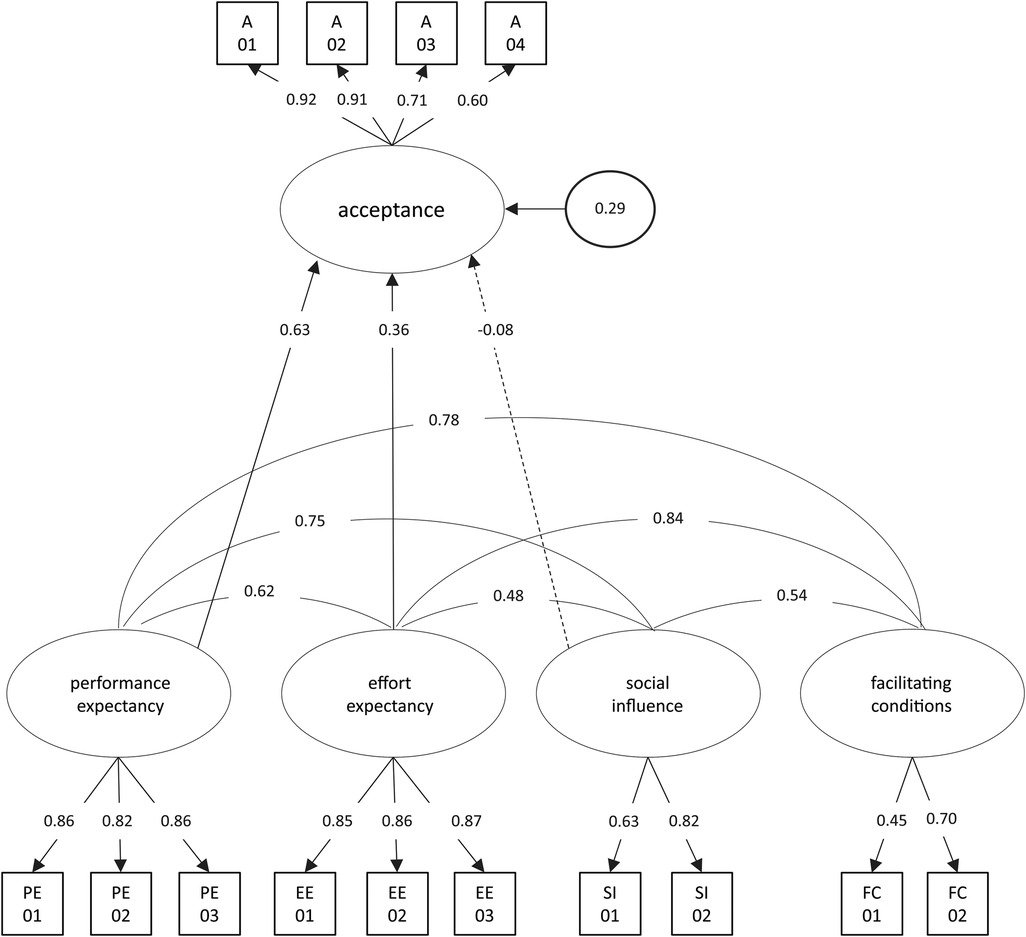
Figure 2. Structural equation model for the acceptance toward smart sensing. Latent variables are represented in ellipses: A, acceptance; PE, performance expectancy; EE, effort expectancy; FC, facilitating conditions; SI, social influence. The dashed line indicates a nonsignificant path. Observed items are indicated as rectangles. Path loadings are represented as single-headed arrows. All exogenous latent variables were allowed to correlate. For improved readability, all latent correlations and residual variances of manifest items were omitted.
3.3 Exploratory analyses
3.3.1 Subgroup analyses
Subgroup analyses revealed meaningful interaction effects regarding gender, age, and education level. A significant intervention effect on acceptance was found in the subgroup of women (t = 3.54, p < .001) with an effect size of d = 0.84 (95% CI: 0.35–1.32). Conversely, there was no significant effect in men (t = −0.59, p = .561). Furthermore, a significant intervention effect was observed in the older patient group (t = 2.37, p = .021) with an effect size of d = 0.62 (95% CI: 0.09–1.14), whereas there was no significant effect in the younger patient group (t = 0.55, p = .586). Similarly, a significant intervention effect was observed for patients with lower educational levels [t = 2.92, p = .007, d = 1.15 (95% CI: 0.30–1.97)], but not for patients with higher education (t = 1.56, p = .114). Table 4 includes the means and standard deviations of acceptance for each subgroup, along with the p-values of the intervention effects.
3.3.2 Psychological distress and acceptance
There were no significant associations in the overall sample between acceptance and depression [r = .02 (95% CI: −.17 to −.20), p = .867], acceptance and anxiety [r = .01 (95% CI: −.17 to −.20), p = .893], acceptance and stress [r = .06 (95% CI: −.13 to −.24), p = .560], or between acceptance and overall psychological distress [r = .03 (95% CI: −.15 to −.21), p = .728].
4 Discussion
4.1 Effect of AFI
Acceptance is a fundamental precondition for the dissemination, uptake, and clinical impact of smart sensing. The mean acceptance of the CG suggests that the baseline acceptance for smart sensing in psychotherapy patients is at a low to moderate level. We hence conclude that enhancing the acceptance should be considered, potentially increasing the use and adherence to the technology. The present UTAUT-based AFI was able to significantly increase the acceptance of smart sensing in psychotherapy patients and increased performance expectancy and social influence as well. The effect size of the intervention on acceptance (d = 0.40) fell within moderate range. Considering determinants of acceptance according to the UTAUT model, performance and effort expectancy achieved high levels with means close to 4 on a 5-point Likert scale in the IG (compare Table 4). Social influence was increased by the AFI reaching a moderate to high level. Additionally, the overall sample showed high levels of facilitating conditions.
To the best of our knowledge, this is the first study to explore the use of an AFI for smart sensing in psychotherapy patients. Consequently, a direct comparison with study outcomes in similar clinical populations is not feasible. Compared to the general population (30), psychotherapy patients exhibited similar levels of acceptance for smart sensing in the control group, while this study revealed a greater intervention effect by the AFI. We identified the following reasons for the greater intervention effect: (1) The AFI in this study was tailored to the group of psychotherapy patients and specifically targeted their needs, whereas the AFI in the comparative study did not address specific needs but introduced the technology and possible applications in a more general manner. (2) The AFI in this study was three times longer than in the comparative study, which allowed us to provide more in-depth information about smart sensing. (3) Performance expectancy was the most important predictor of acceptance for smart sensing in both this patient population and the general population (30). However, it is questionable whether a population with good mental health expects meaningful benefits from smart sensing, while a population of psychotherapy patients might have a clearer connection to the benefits of the technology. Lastly, we optimized the intervention by applying state of the art instructional design principles to split the cognitive load across auditory and visual channels in the white-board video and implemented a narrative explanation style in the AFI (40–42).
For clinical practice our results support the implementation of scalable AFI in an online video format to increase the acceptance of smart sensing in patients. Given the time- and location independent nature of such AFI, they may become a feasible and effective way to implement smart sensing at various stages before (e.g., installation and symptom tracking before treatment for a data informed decision and recommendation of psychotherapeutic modules), during (e.g., to monitor treatment progress via smart sensing), and after psychotherapy [e.g., using smart sensing to recognize re-establishing dysfunctional behavior patterns and initiate just-in-time interventions (3)]. That said, the therapeutic relationship between the patient and therapist (49) represents one of the most crucial therapeutic factors in psychotherapy. Hence, it is reasonable to assume that a recommendation for the use of smart sensing by the treating psychotherapist would significantly enhance the acceptance and could outperform the effects of digital AFI. The evaluation of expert-delivered face-to-face AFIs or stepped-information processes combining digital and face-to-face AFIs would be a very valuable addition to this study.
4.2 Influence of acceptance predictors
We confirmed our hypothesis that the UTAUT holds in the context of smart sensing in a clinical sample as the included predictors explained a great amount of the variance of acceptance. This finding aligns with previous findings (30). The results also emphasize that the most critical factor for the acceptance of smart sensing is the expected personal benefit to the patient. Also consistent with prior research, the second most influential predictor was effort expectancy, albeit with a significantly greater impact on acceptance than could be expected based on previous findings (27, 30). One possible reason for this could be that a common symptom in mental disorders, particularly in cases of depressive symptoms, is aversion or a lack of motivation (50). Therefore, any additionally perceived effort is likely to have a more negative impact on acceptance in psychotherapy patients compared to other populations. Thus, the perceived minimal effort appears to be of importance for the acceptance of smart sensing in this context. While social influence showed a significant positive correlation with acceptance, social influence did not remain a predictor of acceptance in the structural equation model. This could be attributed to its contribution to the explained variance in acceptance, which was already accounted for by performance expectancy and effort expectancy, both of which were also significantly correlated with social influence.
Hence, we strongly recommend to focus on the performance expectancy when aiming to successfully implement smart sensing in clinical practice as the influence is almost twice as strong than effort expectancy. For instance, this could be done by highlighting the benefits of smart sensing and how the psychotherapeutic process can benefit from it (e.g., trajectory modeling, early-warning systems). Besides, the already outlined potential to increase the feasibility of smart sensing in clinical practice, AFI may also hold the potential to increase the adherence to smart sensing sample protocols in research to counteract missingness and increase data quality (18, 19, 51).
4.3 Exploratory analyses
The Subgroup analyses are of particular interest when it comes to the question for which subgroups the AFI had the largest impact. However, these analyses must be interpreted in light of the fact that the group sizes were neither large nor balanced with respect to key individual variables. Therefore, they can only provide a hint for future research questions of interest. The results indicate that the AFI particularly enhanced acceptance among females, older patients, and patients with lower education. This appears to be due to lower baseline acceptance of smart sensing in these groups compared to their respective counterparts. This suggests that those are the groups that should especially be provided with an AFI when smart sensing is recommended. Future research is necessary to replicate those findings and might test if AFIs that target specific needs or concerns of those groups could further enhance the acceptance of smart sensing.
Neither stress, anxiety, depression, nor overall psychological distress exhibited a significant association with acceptance. This finding contrasts with other studies reporting a positive relationship between symptom severity and the acceptance of modern technologies in treatment (31, 35, 52). However, it should be noted that the studies by Lin et al. (35) and Baumeister et al. (31) examined different patient groups (pain patients and diabetes patients) and focused on Internet- and mobile-based interventions.
4.5 Limitations
It is important to address certain limitations when interpreting the results. (1) The present study was designed to investigate the acceptance of smart sensing in psychotherapy patients, but did not make a differentiation between mental diagnoses, which could have an impact on the acceptance. To infer to moderation effects on the symptomology level, we conducted exploratory correlation analyses between depression, anxiety, stress, and distress, which yielded non-significant findings. Besides, a clinical discussion for which patient groups the technology might be suited at all, future studies should explore the acceptance in more detail in specific patient groups. (2) Despite contacting every potentially available patient at the site twice, only 149 patients could be recruited for randomization. This means that the final recruitment target of 156 patients could not be reached. At this point, the recruitment capacity at the site was exhausted in terms of patients. Future studies should aim for a confirmatory study and could base their calculations on an effect size of d = 0.4. (3) The active control condition might also have had an effect on the acceptability towards mental health interventions in general, which might carry over to smart sensing. This would mean that baseline acceptance might be even lower, which could be investigated in future studies. (4) Future studies should follow-up with a closer investigation of the acceptance towards specific sensor modalities, such as screen usage, location, biophysiological data or language usage. For instance, Nicholas and colleagues (53) found differences in the acceptance towards health information (e.g., sleep, mood data), and personal data (e.g., communication logs, or location features). To which extent the acceptance might vary across sensors in psychotherapy patients is currently unknown. (5), the present sample showed an imbalance in gender and education leaning towards a female highly educated population. While this may reflect imbalances in prevalence rates for some disorders (e.g., increased prevalence of depression in women) and help seeking behavior to some extent, it also limits the generalizability of the present findings highlighting the need for replication studies. The slight imbalances between the IG and CG concerning gender and education were due to the randomization process but did not influence the overall effect of the AFI on acceptance (please compare Supplementary Material S5). Lastly, like many previous studies, this research primarily assessed acceptance and attitudes towards new technology by predicting behavioral intentions. While behavioral intentions are widely recognized as a proximal indicator of actual behavior, a gap often exists between intention and behavior (54). Therefore, future research should take into account the volitional aspect and incorporate actual smart sensing use, such as uptake rates, as an outcome measure (30, 36, 55).
5 Conclusion
In summary, our study provides evidence that acceptance of smart sensing among psychotherapy patients can be significantly increased by an AFI based on a time- and location independent video format. The low to moderate baseline acceptance level in the CG simultaneously emphasizes the importance of such interventions to potentially ensure technology usage and compliance.
Our IG exhibited high levels of performance expectancy, effort expectancy, and facilitating conditions after exposure to the AFI. This outcome is particularly promising from a scalability perspective, as such videos offer a versatile means of dissemination through various communication channels, including waiting rooms, the Internet, or television. This widespread distribution can significantly contribute to the adoption of these innovative digital health applications.
The study demonstrates that the UTAUT model is applicable within the context of smart sensing in a clinical sample. The findings highlight that the most critical factor for the acceptance of smart sensing is performance expectancy. Therefore, when recommending smart sensing to patients, the focus should be on their expected personal benefits. Exploratory findings suggest that this approach may be especially beneficial for increasing acceptance among females, older patients, and those with lower levels of education.
Data availability statement
Data requests should be directed to the corresponding author (FR). Data can be shared with researchers who provide a methodologically sound proposal, which is not already covered by other researchers. Data can only be shared for projects if the General Data Protection Regulation is met. Requestors may need to sign additional data access agreements. Support depends on available resources.
Ethics statement
The studies involving humans were approved by Ethikkommission der Friedrich-Schiller-Universität Jena (Ethics Committee of the Friedrich Schiller University Jena). The studies were conducted in accordance with the local legislation and institutional requirements. The participants provided their written informed consent to participate in this study.
Author contributions
FR: Conceptualization, Data curation, Investigation, Methodology, Project administration, Supervision, Writing – original draft, Writing – review & editing, Software. EB: Data curation, Formal Analysis, Investigation, Software, Writing – review & editing. GW: Conceptualization, Writing – review & editing. IC: Project administration, Writing – review & editing. HB: Supervision, Writing – review & editing. YT: Conceptualization, Investigation, Methodology, Supervision, Validation, Writing – original draft, Writing – review & editing.
Funding
The author(s) declare financial support was received for the research, authorship, and/or publication of this article.
This study was supported by the initial phase of the German Center for Mental Health [Deutsches Zentrum für Psychische Gesundheit (DZPG), grant: 01EE2303A, 01EE2305B].
Acknowledgments
We want to thank all psychotherapy patients of the outpatient department of the Friedrich-Schiller University Jena who took part in the survey.
Conflict of interest
The authors declare that the research was conducted in the absence of any commercial or financial relationships that could be construed as a potential conflict of interest.
The author(s) declared that they were an editorial board member of Frontiers, at the time of submission. This had no impact on the peer review process and the final decision.
Publisher's note
All claims expressed in this article are solely those of the authors and do not necessarily represent those of their affiliated organizations, or those of the publisher, the editors and the reviewers. Any product that may be evaluated in this article, or claim that may be made by its manufacturer, is not guaranteed or endorsed by the publisher.
Supplementary material
The Supplementary Material for this article can be found online at: https://www.frontiersin.org/articles/10.3389/fdgth.2024.1335776/full#supplementary-material.
References
1. Mohr DC, Zhang M, Schueller SM. Personal sensing: understanding mental health using ubiquitous sensors and machine learning. Annu Rev Clin Psychol. (2017) 13(1):23–47. doi: 10.1146/annurev-clinpsy-032816-044949
2. Moshe I, Terhorst Y, Philippi P, Domhardt M, Cuijpers P, Cristea I, et al. Digital interventions for the treatment of depression: a meta-analytic review. Psychol Bull. (2021) 147(8):749. doi: 10.1037/bul0000334
3. Terhorst Y, Knauer J, Baumeister H. Smart sensing enhanced diagnostic expert systems. In: Montag C., Baumeister H, (Hrsg.). Digital Phenotyping and Mobile Sensing. Cham: Springer International Publishing (2023). S. 413–25. doi: 10.1007/978-3-030-98546-2_24
4. Andrade LH, Alonso J, Mneimneh Z, Wells JE, Al-Hamzawi A, Borges G, et al. Barriers to mental health treatment: results from the WHO world mental health surveys. Psychol Med. (2014) 44(6):1303–17. doi: 10.1017/S0033291713001943
5. Henriques Franca M, Bharat C, Novello E, Hwang I, Medina-Mora ME, Benjet C, et al. Towards measuring effective coverage: critical bottlenecks in quality- and user-adjusted coverage for major depressive disorder in São Paulo metropolitan area, Brazil. Int J Ment Health Syst. (2023) 17(1):19. doi: 10.1186/s13033-023-00583-w
6. Herrman H, Patel V, Kieling C, Berk M, Buchweitz C, Cuijpers P, et al. Time for united action on depression: a lancet–world psychiatric association commission. Lancet. (2022) 399(10328):957–1022. doi: 10.1016/S0140-6736(21)02141-3
7. Garatva P, Terhorst Y, Messner E-M, Karlen W, Pryss R, Baumeister H. Smart sensors for health research and improvement. In: Montag C., Baumeister H, (Hrsg.). Digital Phenotyping and Mobile Sensing. Cham: Springer International Publishing (2023). S. 395–411. doi: 10.1007/978-3-030-98546-2_23
8. Onnela J-P, Rauch SL. Harnessing smartphone-based digital phenotyping to enhance behavioral and mental health. Neuropsychopharmacology. (2016) 41(7):1691–6. doi: 10.1038/npp.2016.7
9. Can YS, Arnrich B, Ersoy C. Stress detection in daily life scenarios using smart phones and wearable sensors: a survey. J Biomed Inform. (2019) 92:103139. doi: 10.1016/j.jbi.2019.103139
10. Levine LJ, Safer MA. Sources of bias in memory for emotions. Curr Dir Psychol Sci. (2002) 11(5):169–73. doi: 10.1111/1467-8721.00193
11. Taylor SE, Brown JD. Positive illusions and well-being revisited: separating fact from fiction. Psychol Bull. (1994) 116(1):21–7. doi: 10.1037/0033-2909.116.1.21
12. Clough BA, Casey LM. The smart therapist: a look to the future of smartphones and mHealth technologies in psychotherapy. Prof Psychol Res Pract. (2015) 46(3):147. doi: 10.1037/pro0000011
13. Abd-Alrazaq A, AlSaad R, Shuweihdi F, Ahmed A, Aziz S, Sheikh J. Systematic review and meta-analysis of performance of wearable artificial intelligence in detecting and predicting depression. NPJ Digit Med. (2023) 6(1):84. doi: 10.1038/s41746-023-00828-5
14. Cornet VP, Holden RJ. Systematic review of smartphone-based passive sensing for health and wellbeing. J Biomed Inform. (2018) 77:120–32. doi: 10.1016/j.jbi.2017.12.008
15. Kulkarni P, Kirkham R, McNaney R. Opportunities for smartphone sensing in E-health research: a narrative review. Sensors. (2022) 22(10):3893. doi: 10.3390/s22103893
16. Harari GM, Gosling SD, Wang R, Chen F, Chen Z, Campbell AT. Patterns of behavior change in students over an academic term: a preliminary study of activity and sociability behaviors using smartphone sensing methods. Comput Human Behav. (2017) 67:129–38. doi: 10.1016/j.chb.2016.10.027
17. Abujrida H, Agu E, Pahlavan K. Machine learning-based motor assessment of Parkinson’s disease using postural sway, gait and lifestyle features on crowdsourced smartphone data. Biomed Phys Eng Express. (2020) 6(3):035005. doi: 10.1088/2057-1976/ab39a8
18. Moshe I, Terhorst Y, Opoku Asare K, Sander LB, Ferreira D, Baumeister H, et al. Predicting symptoms of depression and anxiety using smartphone and wearable data. Front Psychiatry. (2021) 12:625247. doi: 10.3389/fpsyt.2021.625247
19. Opoku Asare K, Terhorst Y, Vega J, Peltonen E, Lagerspetz E, Ferreira D. Predicting depression from smartphone behavioral markers using machine learning methods, hyperparameter optimization, and feature importance analysis: exploratory study. JMIR Mhealth Uhealth. (2021) 9(7):e26540. doi: 10.2196/26540
20. Rohani DA, Faurholt-Jepsen M, Kessing LV, Bardram JE. Correlations between objective behavioral features collected from mobile and wearable devices and depressive mood symptoms in patients with affective disorders: systematic review. JMIR Mhealth Uhealth. (2018) 6(8):e165. doi: 10.2196/mhealth.9691
21. Benoit J, Onyeaka H, Keshavan M, Torous J. Systematic review of digital phenotyping and machine learning in psychosis spectrum illnesses. Harv Rev Psychiatry. (2020) 28(5):296–304. doi: 10.1097/HRP.0000000000000268
22. Faurholt-Jepsen M, Frost M, Vinberg M, Christensen EM, Bardram JE, Kessing LV. Smartphone data as objective measures of bipolar disorder symptoms. Psychiatry Res. (2014) 217(1–2):124–7. doi: 10.1016/j.psychres.2014.03.009
23. Messner E-M, Sariyska R, Mayer B, Montag C, Kannen C, Schwerdtfeger A, et al. Insights: future implications of passive smartphone sensing in the therapeutic context. Verhaltenstherapie. (2019) 29(3):155–65. doi: 10.1159/000501735
24. Pratap A, Atkins DC, Renn BN, Tanana MJ, Mooney SD, Anguera JA, et al. The accuracy of passive phone sensors in predicting daily mood. Depress Anxiety. (2019) 36(1):72–81. doi: 10.1002/da.22822
25. Venkatesh V, Morris MG, Davis GB, Davis FD. User acceptance of information technology: toward a unified view. MIS Q. (2003) 27(3):425. doi: 10.2307/30036540
26. Blut M, Chong A, Tsiga Z, Venkatesh V. Meta-analysis of the unified theory of acceptance and use of technology (UTAUT): challenging its validity and charting a research agenda in the red ocean. J Assoc Inform Syst. (2021) forthcoming. Available online at: https://papers.ssrn.com/sol3/papers.cfm?abstract_id=3834872
27. Philippi P, Baumeister H, Apolinário-Hagen J, Ebert DD, Hennemann S, Kott L, et al. Acceptance towards digital health interventions—model validation and further development of the unified theory of acceptance and use of technology. Internet Interv. (2021) 26:100459. doi: 10.1016/j.invent.2021.100459
28. Sok Foon Y, Chan Yin Fah B. Internet banking adoption in Kuala Lumpur: an application of UTAUT model. Int J Bus Manag. (2011) 6(4):161. doi: 10.5539/ijbm.v6n4p161
29. Wills MJ, El-Gayar OF, Bennett D. Examining Healthcare Professionals’ Acceptance of Electronic Medical Records Using UTAUT. (2008). Available online at: https://scholar.dsu.edu/bispapers/190/ (Accessed October 12, 2023).
30. Terhorst Y, Weilbacher N, Suda C, Simon L, Messner E-M, Sander LB, et al. Acceptance of smart sensing: a barrier to implementation—results from a randomized controlled trial. Front Digit Health. (2023) 5. doi: 10.3389/fdgth.2023.1075266
31. Baumeister H, Nowoczin L, Lin J, Seifferth H, Seufert J, Laubner K, et al. Impact of an acceptance facilitating intervention on diabetes patients’ acceptance of internet-based interventions for depression: a randomized controlled trial. Diabetes Res Clin Pract. (2014) 105(1):30–9. doi: 10.1016/j.diabres.2014.04.031
32. Baumeister H, Seifferth H, Lin J, Nowoczin L, Lüking M, Ebert D. Impact of an acceptance facilitating intervention on patients’ acceptance of internet-based pain interventions: a randomized controlled trial. Clin J Pain. (2015) 31(6):528–35. doi: 10.1097/AJP.0000000000000118
33. Baumeister H, Terhorst Y, Grässle C, Freudenstein M, Nübling R, Ebert DD. Impact of an acceptance facilitating intervention on psychotherapists’ acceptance of blended therapy. PLoS One. (2020) 15(8):e0236995. doi: 10.1371/journal.pone.0236995
34. Ebert DD, Berking M, Cuijpers P, Lehr D, Pörtner M, Baumeister H. Increasing the acceptance of internet-based mental health interventions in primary care patients with depressive symptoms. A randomized controlled trial. J Affect Disord. (2015) 176:9–17. doi: 10.1016/j.jad.2015.01.056
35. Lin J, Faust B, Ebert DD, Krämer L, Baumeister H. A web-based acceptance-facilitating intervention for identifying patients’ acceptance, uptake, and adherence of internet- and mobile-based pain interventions: randomized controlled trial. J Med Internet Res. (2018) 20(8):e244. doi: 10.2196/jmir.9925
36. Schwarzer R. Modeling health behavior change: how to predict and modify the adoption and maintenance of health behaviors. Appl Psychol. (2008) 57(1):1–29. doi: 10.1111/j.1464-0597.2007.00325.x
37. Nilges P, Essau C. Die Depressions-Angst-Stress-Skalen: Der DASS – ein Screeningverfahren nicht nur für Schmerzpatienten. Der Schmerz. (2015) 29(6):649–57. doi: 10.1007/s00482-015-0019-z
39. Kühner C, Bürger C, Keller F, Hautzinger M. Reliabilität und Validität des revidierten Beck-Depressionsinventars (BDI-II): Befunde aus deutschsprachigen Stichproben. Nervenarzt. (2007) 78(6):651–6. doi: 10.1007/s00115-006-2098-7
40. Klepsch M, Seufert T. Making an effort versus experiencing load. Front Educ. (2021) 6:645284. doi: 10.3389/feduc.2021.645284/full
41. Mayer RE. Multimedia learning. In: Ross BH, editor. Psychology of Learning and Motivation. Elsevier (2002). p. 85–139. Available online at: https://www.sciencedirect.com/science/article/pii/S0079742102800056
42. Schneider S, Krieglstein F, Beege M, Rey GD. Successful learning with whiteboard animations–a question of their procedural character or narrative embedding? Heliyon. (2023) 9(2). doi: 10.1016/j.heliyon.2023.e13229
43. Gesundheitsportal Bund (Regisseur). Was ist eine Depression?(2020). Available online at: https://www.youtube.com/watch?v=vWEALfbZYZk (Accessed October 11, 2023).
44. Gesundheitsportal Bund (Regisseur). Was ist eine generalisierte Angststörung? (2022). Available online at: https://www.youtube.com/watch?v=Du3QR0Ykdus (Accessed October 11, 2023).
45. Browne MW, Cudeck R. Alternative ways of assessing model fit. Sociol Methods Res. (1992) 21(2):230–58. doi: 10.1177/0049124192021002005
46. Moshagen M. The model size effect in SEM: inflated goodness-of-fit statistics are due to the size of the covariance matrix. Struct Equ Model Multidiscip J. (2012) 19(1):86–98. doi: 10.1080/10705511.2012.634724
47. Moshagen M, Erdfelder E. A new strategy for testing structural equation models. Struct Equ Model Multidiscip J. (2016) 23(1):54–60. doi: 10.1080/10705511.2014.950896
48. Hu L, Bentler PM. Cutoff criteria for fit indexes in covariance structure analysis: conventional criteria versus new alternatives. Struct Equ Model Multidiscip J. (1999) 6(1):1–55. doi: 10.1080/10705519909540118
49. Flückiger C, Del Re AC, Wampold BE, Horvath AO. The alliance in adult psychotherapy: a meta-analytic synthesis. Psychotherapy. (2018) 55(4):316. doi: 10.1037/pst0000172
50. Kennedy SH. Core symptoms of major depressive disorder: relevance to diagnosis and treatment. Dialogues Clin Neurosci. (2008) 10(3):271–7. doi: 10.31887/DCNS.2008.10.3/shkennedy
51. De Angel V, Lewis S, White K, Oetzmann C, Leightley D, Oprea E, et al. Digital health tools for the passive monitoring of depression: a systematic review of methods. NPJ Digit Med. (2022) 5(1):3. doi: 10.1038/s41746-021-00548-8
52. Arjadi R, Nauta MH, Bockting CLH. Acceptability of internet-based interventions for depression in Indonesia. Internet Interv. (2018) 13:8–15. doi: 10.1016/j.invent.2018.04.004
53. Nicholas J, Shilton K, Schueller SM, Gray EL, Kwasny MJ, Mohr DC. The role of data type and recipient in individuals’ perspectives on sharing passively collected smartphone data for mental health: cross-sectional questionnaire study. JMIR Mhealth Uhealth. (2019) 7(4):e12578. doi: 10.2196/12578
54. Bhattacherjee A, Sanford C. The intention–behaviour gap in technology usage: the moderating role of attitude strength. Behav Inf Technol. (2009) 28(4):389–401. doi: 10.1080/01449290802121230
Keywords: smart sensing, psychotherapy, acceptance, digital health, implementation, unified theory of acceptance and use of technology
Citation: Rottstädt F, Becker E, Wilz G, Croy I, Baumeister H and Terhorst Y (2024) Enhancing the acceptance of smart sensing in psychotherapy patients: findings from a randomized controlled trial. Front. Digit. Health 6:1335776. doi: 10.3389/fdgth.2024.1335776
Received: 9 November 2023; Accepted: 2 April 2024;
Published: 18 April 2024.
Edited by:
Silvia Simbula, University of Milano-Bicocca, ItalyReviewed by:
Rainer Leonhart, University of Freiburg, GermanyMarkus Wolf, University of Zurich, Switzerland
© 2024 Rottstädt, Becker, Wilz, Croy, Baumeister and Terhorst. This is an open-access article distributed under the terms of the Creative Commons Attribution License (CC BY). The use, distribution or reproduction in other forums is permitted, provided the original author(s) and the copyright owner(s) are credited and that the original publication in this journal is cited, in accordance with accepted academic practice. No use, distribution or reproduction is permitted which does not comply with these terms.
*Correspondence: Fabian Rottstädt ZmFiaWFuLnJvdHRzdGFlZHRAdW5pLWplbmEuZGU=