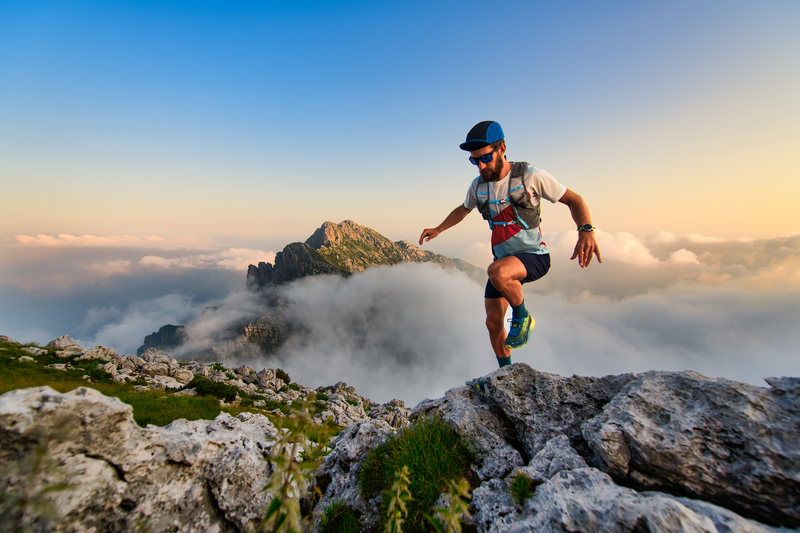
95% of researchers rate our articles as excellent or good
Learn more about the work of our research integrity team to safeguard the quality of each article we publish.
Find out more
OPINION article
Front. Digit. Health , 23 January 2024
Sec. Health Communications and Behavior Change
Volume 6 - 2024 | https://doi.org/10.3389/fdgth.2024.1330160
This article is part of the Research Topic Reliable AI and Imaging Methods View all 5 articles
Health disparities are one of the most pressing health issues we face today, transcending the boundaries of any single profession or discipline despite many decades of research and novel interventions (1) This issue has shown stark divisions in equitable access to high-quality healthcare based on race, ethnicity, gender, language preference, disabilities, socioeconomic status, environment, and other factors reflecting systemic biases and structural health inequalities. Addressing these social determinants of health is crucial to reducing health disparities and achieving health equity.
Health disparities are a complex issue, as there are multiple contributing factors that extend well beyond individuals’ behaviors and choices (2). This multidimensional issue is based on historical, societal, and economic conditions. As a result, disparate health outcomes are seen among various population groups, including minorities or marginalized people or communities, and those without access to resources. The US healthcare system can perpetuate inequality by allowing differential access to quality healthcare, diagnostic delays, treatment inaccessibility, and unequal treatment between populations.
Technological tools arising from artificial intelligence (AI) have the potential to unravel the difficulties of addressing social determinants of health and mitigating health disparities (3). Mitigating health disparities is not just a mere assertion of AI's potential. Still, it is based on the robust body of literature affirming that AI is a transformative force that can be used to dissect the multifactorial social, genetic, and environmental factors of health disparities (3). We argue that using AI to address health disparities is not just a simple choice but a scientific imperative (4–8). As this discourse continues, we must recognize the pressing need for AI as an important aspect in our collective endeavor to alleviate health disparities. As such, we present a call to action to position AI as a critical tool in the pursuit of health equity.
AI is a rapidly developing field of study with incredible potential to change how we deliver care and provide health services (9). AI is already being used to address some of the most pressing health challenges and healthcare obstacles that we face as a society, from improving diagnostic accuracy to supporting precision medicine (10). Given the context of AI's preexisting utility in healthcare, AI technologies have revolutionary potential as a disruptive partner in addressing health disparities.
By taking advantage of these powerful tools, we can become more efficient and comprehensive, creating a paradigm shift revolutionizing how we measure, understand, and develop interventions to address disparities. Traditional approaches to address health disparities often fail to capture the issue's complexity and leave many gaps unresolved (11). By analyzing large datasets for patterns, correlations, or predictive markers that are difficult to identify through traditional analyses, AI can help us uncover hidden mechanisms and underpinnings of health disparities (12, 13). AI has many advantages compared with traditional strategies for addressing health disparities, notably in its ability to uncover unexpected correlations and relationships that have remained unidentified in human-driven analyses, offering new insights. By examining variables like genetic markers, environmental exposures, social determinants, and zip codes, AI can uncover novel connections that challenge current paradigms, revealing new areas for research and interventions (14).
Though AI holds great promise as a tool to combat health disparities, it also presents some unique challenges that need to be considered.
First, AI is limited to the quality of data it’s trained on (5, 15, 16). Populations that have been historically marginalized and experience barriers to care are underrepresented in historical datasets, leading to them being negatively impacted when biased data and algorithms are deployed in real-world applications (15, 17, 18). If AI is implemented without regard for these biases, it can reinforce or even worsen existing disparities. There is a need for more inclusive datasets that accurately reflect the health experiences of various marginalized social, racial, and ethnic groups.
Second, equity must be considered during all stages of AI use and processes (7); it is critical to design algorithms with equity in mind. Technical approaches to mitigating discrimination and bias in algorithm development, like the open-source AI Fairness Project, should be used when applicable (https://aif360.res.ibm.com/). When health disparities are not considered during all stages of AI development and implementation, AI can perpetuate existing biases and inequalities within healthcare, which can disproportionately impact marginalized populations.
Third, there is a lack of diversity and representation within the teams developing and deploying AI algorithms (19). Greater representation from various stakeholders from diverse backgrounds, including policymakers, researchers, clinicians, and ethicists, must be included to ensure different voices and perspectives are included in the research team (20–22). Diverse research teams are more able to identify potential disparities, inequities, or underrepresentation that could lead to biased (15) AI algorithms (22).
Fourth, ethical standards and guidelines must be established around the use of AI. As previously mentioned, some critics contend that AI's dependence on historical data may perpetuate biases present in past healthcare practices (23); as such, it will be important to address this concern so that we avoid reproducing patterns that could be seen as discriminatory. Even if the models are built and algorithms developed a way that mitigates bias, there should still be a focus on ensuring that the algorithm output's use does not exacerbate disparities. Ethical standards should focus on mitigating bias, increasing transparency, and ensuring accountability regarding the creation and usage of AI algorithms.
Fifth and finally, there are concerns that becoming overly reliant on AI may undermine the clinician-patient relationship (14). Though algorithms cannot and should not replace human interactions in healthcare, incorporating AI as a decision support tool rather than a replacement for humans can harness and balance both the physician's expertise and machine's insights. Also, AI models or algorithms may potentially help enhance the patient-provider relationship by identifying key subject areas or talking points based on individualized patient factors, leading to better conversations and outcomes (24). AI should be designed to make the jobs of humans in healthcare better and easier. By recognizing concerns around AI, we can employ rigorous strategies, such as debiasing the data, optimizing development of algorithms, and providing ethical oversight, to ensure the integrity of both the data and the output before deploying AI strategies.
AI will present new challenges when it is used to address health disparities, but its potential is immense. Combining human ingenuity with AI offers a new roadmap towards an equitable healthcare system and overall health ecosystem. For us to realize AI's maximum impact for all key stakeholders within the healthcare system, we must be fully willing to leverage these new tools by harmonizing data standards, refining algorithms, and creating a more inclusive culture within this space. We suggest the following actionable recommendations.
Researchers must advocate for the development and utilization of more comprehensive, diverse, and inclusive datasets that accurately represent marginalized communities and various socioeconomic backgrounds (15, 22, 25–28). There must be collaborations among healthcare institutions, community organizations, and AI developers to ensure the incorporation of diverse perspectives (8, 22, 29, 30).
There is a need to establish stringent ethical guidelines and frameworks for designing AI algorithms. Prioritization of transparency, fairness, and accountability throughout the AI development and implementation process is key (8, 13, 18, 31, 32). We must leverage tools like the AI Fairness Project to mitigate biases and discrimination in AI algorithms used within healthcare.
We must encourage and facilitate greater diversity within AI research and development teams. Inclusive representation from various stakeholders-such as policymakers, healthcare professionals, ethicists, and community representatives- will aid in identifying biases and disparities, ensuring a broader perspective in algorithms development (13, 15, 16, 20, 22, 31, 33–35).
There is a need to formulate and implement clear ethical standards specific to AI applications in healthcare. These standards should focus on reducing biases in AI-driven models, ensuring transparency, and overseeing the responsible development and deployment of AI algorithms to avoid exacerbating disparities (13, 18, 21, 22, 36–39).
There should be an emphasis on the complementary role of AI as a decision support tool rather than a replacement for human interaction in healthcare settings. AI should be designed to enhance, not replace, the clinician-patient relationship, utilizing machine learning and insights to facilitate more informed conversations and personalized care (40).
Mechanisms must be developed for ongoing evaluation and improvement of AI models. Protocols for regularly auditing and monitoring AI systems must be established to ensure they remain unbiased, transparent, and aligned to reduce health disparities (8, 20, 31, 34, 41–43).
Leveraging AI in addressing health disparities necessitates a concerted effort to navigate the challenges while capitalizing on the immense potential these tools offer. By prioritizing inclusive data practices, ethical guidelines, diverse representation, and maintaining a human-centered approach to healthcare, we can harness AI's transformative power to mitigate health disparities and move closer toward achieving health equity for all.
We must implement AI with care, integrity, and an unyielding dedication to equity. Through collaborative action and audacious vision, we can pave the way towards a future where health disparities become less prominent and widespread.
BG: Conceptualization, Writing – original draft, Writing – review & editing. AM: Writing – original draft, Writing – review & editing. ER: Writing – review & editing.
The author(s) declare that no financial support was received for the research, authorship, and/or publication of this article.
Editorial assistance was provided by the Moffitt Cancer Center's Office of Scientific Publishing by DW and GH; no compensation was given beyond their regular salaries.
The authors declare that the research was conducted in the absence of any commercial or financial relationships that could be construed as a potential conflict of interest.
All claims expressed in this article are solely those of the authors and do not necessarily represent those of their affiliated organizations, or those of the publisher, the editors and the reviewers. Any product that may be evaluated in this article, or claim that may be made by its manufacturer, is not guaranteed or endorsed by the publisher.
1. Institute of medicine (US) committee on understanding and eliminating racial and ethnic disparities in health care. In: Smedley BD, Stith AY, Nelson AR, editors. Unequal Treatment: Confronting Racial and Ethnic Disparities in Health Care. Washington, DC: National Academies Press (US) (2003). 25032386
3. National Healthcare Quality & Disparities Reports. 2021 National Healthcare Quality and Disparities Report. Rockville, MD: Agency for Healthcare Research and Quality (US) (2021).
4. Ferryman K. Addressing health disparities in the food and drug administration’s artificial intelligence and machine learning regulatory framework. J Am Med Inform Assoc. (2020) 27(12):2016–9. doi: 10.1093/jamia/ocaa133
5. Thomasian NM, Eickhoff C, Adashi EY. Advancing health equity with artificial intelligence. J Public Health Policy. (2021) 42(4):602–11. doi: 10.1057/s41271-021-00319-5
6. Obermeyer Z, Powers B, Vogeli C, Mullainathan S. Dissecting racial bias in an algorithm used to manage the health of populations. Science. (2019) 366(6464):447–53. doi: 10.1126/science.aax2342
7. d'Elia A, Gabbay M, Rodgers S, Kierans C, Jones E, Durrani I, et al. Artificial intelligence and health inequities in primary care: a systematic scoping review and framework. Fam Med Community Health. (2022) 10(Suppl 1):e001670. doi: 10.1136/fmch-2022-001670
8. Rajkomar A, Hardt M, Howell MD, Corrado G, Chin MH. Ensuring fairness in machine learning to advance health equity. Ann Intern Med. (2018) 169(12):866–72. doi: 10.7326/M18-1990
9. Corti C, Cobanaj M, Dee EC, Criscitiello C, Tolaney SM, Celi LA, et al. Artificial intelligence in cancer research and precision medicine: applications, limitations and priorities to drive transformation in the delivery of equitable and unbiased care. Cancer Treat Rev. (2023) 112:102498. doi: 10.1016/j.ctrv.2022.102498
10. Sahni NR, Carrus B. Artificial intelligence in U.S. health care delivery. N Engl J Med. (2023) 389(4):348–58. doi: 10.1056/NEJMra2204673
11. Topol EJ. High-performance medicine: the convergence of human and artificial intelligence. Nat Med. (2019) 25(1):44–56. doi: 10.1038/s41591-018-0300-7
12. Purnell TS, Calhoun EA, Golden SH, Halladay JR, Krok-Schoen JL, Appelhans BM, et al. Achieving health equity: closing the gaps in health care disparities, interventions, and research. Health Aff (Millwood). (2016) 35(8):1410–5. doi: 10.1377/hlthaff.2016.0158
13. Basu S, Faghmous JH, Doupe P. Machine learning methods for precision medicine research designed to reduce health disparities: a structured tutorial. Ethn Dis. (2020) 30(Suppl 1):217–28. doi: 10.18865/ed.30.S1.217
14. Dankwa-Mullan I, Weeraratne D. Artificial intelligence and machine learning technologies in cancer care: addressing disparities, bias, and data diversity. Cancer Discov. (2022) 12(6):1423–7. doi: 10.1158/2159-8290.Cd-22-0373
15. Obermeyer Z, Emanuel EJ. Predicting the future—big data, machine learning, and clinical medicine. N Engl J Med. (2016) 375(13):1216–9. doi: 10.1056/NEJMp1606181
16. Parikh RB, Teeple S, Navathe AS. Addressing bias in artificial intelligence in health care. JAMA. (2019) 322(24):2377–8. doi: 10.1001/jama.2019.18058
17. Adamson AS, Smith A. Machine learning and health care disparities in dermatology. JAMA Dermatol. (2018) 154(11):1247–8. doi: 10.1001/jamadermatol.2018.2348
18. Gianfrancesco MA, Tamang S, Yazdany J, Schmajuk G. Potential biases in machine learning algorithms using electronic health record data. JAMA Intern Med. (2018) 178(11):1544–7. doi: 10.1001/jamainternmed.2018.3763
19. Nordling L. A fairer way forward for AI in health care. Nature. (2019) 573(7775):S103–s5. doi: 10.1038/d41586-019-02872-2
20. Panch T, Mattie H, Atun R. Artificial intelligence and algorithmic bias: implications for health systems. J Glob Health. (2019) 9(2):010318. doi: 10.7189/jogh.09.020318
21. Johnson SLJ. AI, machine learning, and ethics in health care. J Legal Med. (2019) 39(4):427–41. doi: 10.1080/01947648.2019.1690604
22. Cho MK. Rising to the challenge of bias in health care AI. Nat Med. (2021) 27(12):2079–81. doi: 10.1038/s41591-021-01577-2
23. Hague DC. Benefits, pitfalls, and potential bias in health care AI. N C Med J. (2019) 80(4):219–23. doi: 10.18043/ncm.80.4.219
24. Kluge E-HW. Artificial intelligence in healthcare: ethical considerations. Healthcare Management Forum. (2020) 33(1):47–9. doi: 10.1177/0840470419850438
25. Sauerbrei A, Kerasidou A, Lucivero F, Hallowell N. The impact of artificial intelligence on the person-centred, doctor-patient relationship: some problems and solutions. BMC Med Inform Decis Mak. (2023) 23(1):73. doi: 10.1186/s12911-023-02162-y
26. Plana D, Shung DL, Grimshaw AA, Saraf A, Sung JJY, Kann BH. Randomized clinical trials of machine learning interventions in health care: a systematic review. JAMA Netw Open. (2022) 5(9):e2233946. doi: 10.1001/jamanetworkopen.2022.33946
27. Brault N, Saxena M. For a critical appraisal of artificial intelligence in healthcare: the problem of bias in mhealth. J Eval Clin Pract. (2021) 27(3):513–9. doi: 10.1111/jep.13528
28. Zaidi D, Miller T. Implicit bias and machine learning in health care. South Med J. (2023) 116(1):62–4. doi: 10.14423/smj.0000000000001489
29. Byrne MD. Reducing bias in healthcare artificial intelligence. J Perianesth Nurs. (2021) 36(3):313–6. doi: 10.1016/j.jopan.2021.03.009
30. Sood A, Sangari A, Chen JY, Stoff BK. The ethics of using biased artificial intelligence programs in the clinic. J Am Acad Dermatol. (2022) 87(4):935–6. doi: 10.1016/j.jaad.2021.11.031
31. Rojas JC, Fahrenbach J, Makhni S, Cook SC, Williams JS, Umscheid CA, et al. Framework for integrating equity into machine learning models: a case study. Chest. (2022) 161(6):1621–7. doi: 10.1016/j.chest.2022.02.001
32. Rajkomar A, Dean J, Kohane I. Machine learning in medicine. N Engl J Med. (2019) 380(14):1347–58. doi: 10.1056/NEJMra1814259
33. Uche-Anya E, Anyane-Yeboa A, Berzin TM, Ghassemi M, May FP. Artificial intelligence in gastroenterology and hepatology: how to advance clinical practice while ensuring health equity. Gut. (2022) 71(9):1909–15. doi: 10.1136/gutjnl-2021-326271
34. Chen IY, Szolovits P, Ghassemi M. Can AI help reduce disparities in general medical and mental health care? AMA J Ethics. (2019) 21(2):E167–79. doi: 10.1001/amajethics.2019.167
35. Gervasi SS, Chen IY, Smith-McLallen A, Sontag D, Obermeyer Z, Vennera M, et al. The potential for bias in machine learning and opportunities for health insurers to address it. Health Aff (Millwood). (2022) 41(2):212–8. doi: 10.1377/hlthaff.2021.01287
36. McCradden MD, Joshi S, Anderson JA, Mazwi M, Goldenberg A, Zlotnik Shaul R. Patient safety and quality improvement: ethical principles for a regulatory approach to bias in healthcare machine learning. J Am Med Inform Assoc. (2020) 27(12):2024–7. doi: 10.1093/jamia/ocaa085
37. Timmons AC, Duong JB, Simo Fiallo N, Lee T, Vo HPQ, Ahle MW, et al. A call to action on assessing and mitigating bias in artificial intelligence applications for mental health. Perspect Psychol Sci. (2023) 18(5):1062–96. doi: 10.1177/17456916221134490
38. Crigger E, Reinbold K, Hanson C, Kao A, Blake K, Irons M. Trustworthy augmented intelligence in health care. J Med Syst. (2022) 46(2):12. doi: 10.1007/s10916-021-01790-z
39. Wiens J, Price WN 2nd, Sjoding MW. Diagnosing bias in data-driven algorithms for healthcare. Nat Med (2020) 26(1):25–6. doi: 10.1038/s41591-019-0726-6
40. Matheny ME, Whicher D, Thadaney Israni S. Artificial intelligence in health care: a report from the national academy of medicine. JAMA. (2020) 323(6):509–10. doi: 10.1001/jama.2019.21579
41. Chen Y, Clayton EW, Novak LL, Anders S, Malin B. Human-centered design to address biases in artificial intelligence. J Med Internet Res. (2023) 25:e43251. doi: 10.2196/43251
42. Sikstrom L, Maslej MM, Hui K, Findlay Z, Buchman DZ, Hill SL. Conceptualising fairness: three pillars for medical algorithms and health equity. BMJ Health Care Inform. (2022) 29(1):e100459. doi: 10.1136/bmjhci-2021-100459
Keywords: health disparities, artificial intelligence, machine learning, equity, bias
Citation: Green BL, Murphy A and Robinson E (2024) Accelerating health disparities research with artificial intelligence. Front. Digit. Health 6:1330160. doi: 10.3389/fdgth.2024.1330160
Received: 3 November 2023; Accepted: 10 January 2024;
Published: 23 January 2024.
Edited by:
Maurizio Caon, University of Applied Sciences and Arts of Western Switzerland, SwitzerlandReviewed by:
Karthik Adapa, University of North Carolina at Chapel Hill, United States© 2024 Green, Murphy and Robinson. This is an open-access article distributed under the terms of the Creative Commons Attribution License (CC BY). The use, distribution or reproduction in other forums is permitted, provided the original author(s) and the copyright owner(s) are credited and that the original publication in this journal is cited, in accordance with accepted academic practice. No use, distribution or reproduction is permitted which does not comply with these terms.
*Correspondence: Anastasia Murphy YW5hc3Rhc2lhLm11cnBoeUBtb2ZmaXR0Lm9yZw==
Disclaimer: All claims expressed in this article are solely those of the authors and do not necessarily represent those of their affiliated organizations, or those of the publisher, the editors and the reviewers. Any product that may be evaluated in this article or claim that may be made by its manufacturer is not guaranteed or endorsed by the publisher.
Research integrity at Frontiers
Learn more about the work of our research integrity team to safeguard the quality of each article we publish.