- 1Sport and Physical Activity Research Institute, School of Health and Life Sciences, University of the West of Scotland, Glasgow, United Kingdom
- 2Future Health Technologies, Singapore-ETH Centre, Campus for Research Excellence and Technological Enterprise (CREATE), Singapore, Singapore
- 3Saw Swee Hock School of Public Health, National University of Singapore, Singapore, Singapore
Background: With the rise of smartphone ownership and increasing evidence to support the suitability of smartphone usage in healthcare, the light source and smartphone camera could be utilized to perform photoplethysmography (PPG) for the assessment of vital signs, such as heart rate (HR). However, until rigorous validity assessment has been conducted, PPG will have limited use in clinical settings.
Objective: We aimed to conduct a scoping review assessing the validity of resting heart rate (RHR) acquisition from PPG utilizing contact-based smartphone devices. Our four specific objectives of this scoping review were to (1) conduct a systematic search of the published literature concerning contact-based smartphone device-derived PPG, (2) map study characteristics and methodologies, (3) identify if methodological and technological advancements have been made, and (4) provide recommendations for the advancement of the investigative area.
Methods: ScienceDirect, PubMed and SPORTDiscus were searched for relevant studies between January 1st, 2007, and November 6th, 2022. Filters were applied to ensure only literature written in English were included. Reference lists of included studies were manually searched for additional eligible studies.
Results: In total 10 articles were included. Articles varied in terms of methodology including study characteristics, index measurement characteristics, criterion measurement characteristics, and experimental procedure. Additionally, there were variations in reporting details including primary outcome measure and measure of validity. However, all studies reached the same conclusion, with agreement ranging between good to very strong and correlations ranging from r = .98 to 1.
Conclusions: Smartphone applications measuring RHR derived from contact-based smartphone PPG appear to agree with gold standard electrocardiography (ECG) in healthy subjects. However, agreement was established under highly controlled conditions. Future research could investigate their validity and consider effective approaches that transfer these methods from laboratory conditions into the “real-world”, in both healthy and clinical populations.
Introduction
Rationale
Photoplethysmography (PPG) can provide important clinical outcome measures and has been used for the diagnosis, monitoring, and screening of various diseases and disorders (1). “Photoplethysmography” consists of “photo,” meaning light; “plethysmo,” meaning volume; and “graphy” meaning recording (2). PPG was first suggested as a technique for measuring blood volume changes by Hertzman in 1937 (3, 4). PPG is a measurement of light either absorbed (transmissive photoplethysmography) or reflected (reflective photoplethysmography) by human tissues (1), and is based on optical properties such as absorption, scattering and transmission (5). Transmissive PPG measures light that passes through the various human tissues and is mainly used at the distal parts of the body where those tissues are thin, for example at the fingers, toes, and earlobes. Reflective PPG measures scattered light that irradiates skin tissue and produces a reduced light intensity (6). While transmissive PPG exhibits more stable PPG performance (7) since the reflective type of signal is degraded, the latter has the advantage of a greater number of measurement sites such as the forehead, wrist, carotid artery, and esophagus, where transmissive PPG would be difficult (8, 9).
As such, PPG data is explained by Beer-Lambert's Law which defines resultant light intensity by the extinction coefficient, concentration, and optical path length of a medium when light passes through it (10). The Beer–Lambert law can be described by:
Whereby: the transmitted light intensity (I) through a medium will decrease exponentially in irradiated light intensity (I0) in relation to the absorption coefficient (ε), where (λ) is the specific absorptivity, characteristic of the traversed tissue and dependent on the light wavelength λ, ρ is the density of the tissue, and d is the light pathlength (6).
Since most of these factors are constant for a given tissue the signal quality is mainly impacted through the later part of the equation, through manipulation of λ, ρ and d, which can be modified through measurement site selection, wavelength selection and contact pressure, resulting in a reduced ε, which could explain why fingers and earlobes are preferred.
Various PPG devices have been utilized in clinical practice (1). However, since the release of the first iPhone in 2007, smartphones have been widely adopted globally (11) and are now considered a tool with high utility, avoiding some major pitfalls of traditional data collection techniques. The traditional approach where an individual's health is monitored periodically, often by appointment, may not be an accurate representation of the possible variations in physiological measurements that occur longitudinally (12, 13). Moreover, smartphone technology and embedded cameras allow PPG acquisition without the need for additional, potentially costly, external devices (14) and could be suitable for targeting populations in traditionally underserved groups (15) particularly those whose demographic, geographic, or economic characteristics negatively affect health care access and delivery (16, 17). Therefore, telemedicine technologies are becoming more widely adopted in practice, especially since the recent COVID-19 pandemic, which highlighted the need for vital signs evaluated using telemonitoring (14, 18, 19). As a result, the proliferation of smartphone-based telemedicine appears to be here to stay and could address the United Nations Sustainable Development Goals (UN SDGs) (20), in particular UN SDG 3 (21).
Smartphone PPG has been previously utilized to estimate resting heart rate (RHR) through the measurement of distal pulse rate (PR) at rest, during exercise, and whilst completing mental tasks (1). However, at the time of writing, there is no consensus on what metric should be used to establish the validity of smartphone-based PPG or under what conditions. Another issue is that to convert the PPG signal, a mathematical algorithm is required which not only affects smartphone performance but also validity and reliability. This is problematic given the proliferation of telemedicine, and it is therefore essential mobile health (mHealth) technologies are considered reliable and valid compared to gold standard measurements before universal adoption (22). In this context, De Ridder et al. (23) conducted a meta-analysis of articles published between 1st January 2009 and 7th December 2016 investigating the use of smartphones to measure PR by performing PPG in comparison with a range of methods, including ECG, pulse oximetry and radial pulse. Although these methods suffer various pitfalls, comparisons with multiple validation methods could strengthen smartphone device and application validity. Results revealed good agreement between smartphone-derived (HR-PPG) and validated method-derived RHR. These authors therefore concluded that RHR obtained from a smartphone PPG signal could be used as an alternative to traditional methods, such as ECG, in an adult population, in the right context. However, De Ridder et al. (23) highlighted several limitations to the included studies. Firstly, there was high statistical heterogeneity between studies, ostensibly due to participant characteristics, measurement conditions, and the smartphone devices utilized (23). Secondly, the latest IOS device reviewed was the iPhone 5 (released 2012) and the latest android was the Samsung Galaxy S4 (released 2013). Emerging evidence suggests advancements in technology, such as the availability of various camera positions (i.e., front-facing vs. rear-facing) and the advent of multiple lenses, could result in improvements in PPG acquisition (14).
These technological enhancements are promising for the telemedicine sphere as HR-PPG could be considered a population-level biomarker, utilized for screening, surveillance, and to monitor responses to policy interventions in epidemiology and public health. Population-level biomarkers are easy to measure in the real-world, low-cost and scalable (24). RHR has considerable population-level applicability and can predict adverse outcomes and the development of disease. As smartphone ownership is increasing [80% of over 65-year-olds own a smartphone in the UK (25, 26)], and smartphone HR-PPG removes the barrier to scalability of “wearable” ownership, valid contact-based HR-PPG from a smartphone device has significant scope for public health surveillance. However, before that goal is reached, it is imperative to consider the existing literature in terms of HR-PPG validity.
Two approaches of measuring PR via PPG are known: contact and non-contact. With contact PPG, PR is measured by placing a finger on the phone rear camera, while in non-contact, imaging photoplethysmography (iPPG) is extracted from the face, without the need for direct skin contact. iPGG has some advantages over contact-based PPG, such as detecting PR in crowds and at long-distance (27, 28). However, in general, contact PPG exhibits better accuracy than non-contact PPG (29). Considering that contact-based PPG is generally more accurate than non-contact PPG, and our group's interest in this methodology, we were interested in the validity of RHR acquisition from PPG utilizing contact-based smartphone devices.
Objectives
As a result of the importance of using validated PPG for telemedicine, and the rapidly improving technology, we aimed to conduct a scoping review assessing the validity of RHR acquisition from PPG (referred to as HR-PPG) utilizing contact-based smartphone devices against gold standard ECG (referred to as HR-ECG). Our four specific objectives of this scoping review were to 1) conduct a systematic search of the published literature concerning contact-based smartphone device-derived PPG, 2) map study characteristics and methodologies, 3) identify if methodological and technological advancements have been made, and 4) provide recommendations for the advancement of the investigative area.
Methods
Protocol and registration
The review was not preregistered, as scoping reviews are not. This review was conducted and reported in accordance with the Preferred reporting items for systematic reviews and meta-analyses extension for scoping reviews (PRISMA-ScR) guidelines (30).
Eligibility criteria
Studies were included if the measurement of HR-PPG was conducted via the front or rear facing camera of a smartphone by contact-based PPG. Only studies compared with the gold standard measurement [electrocardiography (ECG)], were included. Studies were excluded if the index measurement was conducted with a device connected to a smartphone, such as a mobile sensor, medical device or wearable device; the paper did not include validity assessment of HR-PPG and HR-ECG as an outcome measurement; the study used a clinical population (we assumed healthy population unless stated otherwise); the paper was not an original article (i.e., utilized a database from a secondary source); the paper was a review; there was no abstract or full text available.
Literature search
We conducted a systematic literature search of ScienceDirect, PubMed and SPORTDiscus from January 1st, 2007, to November 6th, 2022, with the following search key: (((((“validity”) AND (“mobile”)) AND (“photoplethysmography”)) OR (“PPG”)) AND (“heart rate”)) NOT (“wearable”) AND [2007:2022(pdat)], which were developed through examination of previously published original and review articles. Filters were applied to ensure only literature written in English were included. Reference lists of included studies were manually searched for additional eligible studies.
Study selection
Studies were identified by the first author and evaluated by JDM and LDH independently and compared in an unblinded and standardized manner. Once database searches were complete, all studies were downloaded to a single reference list [utilizing Zotero software (version 6.0.26)] and duplicates were removed. First, titles and abstracts were screened for eligibility (JDM). Full text articles were then read and coded in relation to exclusion criteria, utilizing “tags” in Zotero [version 6.0.26], which was reviewed by the second author (LDH). This process involved a thorough assessment of all eligibility criteria with authors JDM and LDH confirming inclusion and exclusion. Additionally, disagreements were addressed by a third reviewer (NFS).
Data extraction
Data extracted from each study included author(s), sample size, participant sex, country of study, age, skin pigmentation, if participants were considered healthy, smartphone model, name of application utilized, whether the application was commercially available, index measurement sampling rate, camera position and resolution, flash (torch) settings, channel used for computations, ECG device utilized, electrode placement, ECG processing information, instructions given to participants, dietary control, participant posture, region of interest, breathing pattern, environmental conditions, stabilization period, duration of measurement, number of attempts or trials, primary outcome measures and measures of validity.
Outcome measures
Our primary interests were measurements of validity and mean differences between heart rate via gold standard ECG measurement (HR-ECG), and pulse rate measured by contact-based smartphone PPG (HR-PPG). Additionally, issues that arose regarding the reporting and conducting of HR-PPG validity assessment were compiled into a checklist (Table 1).
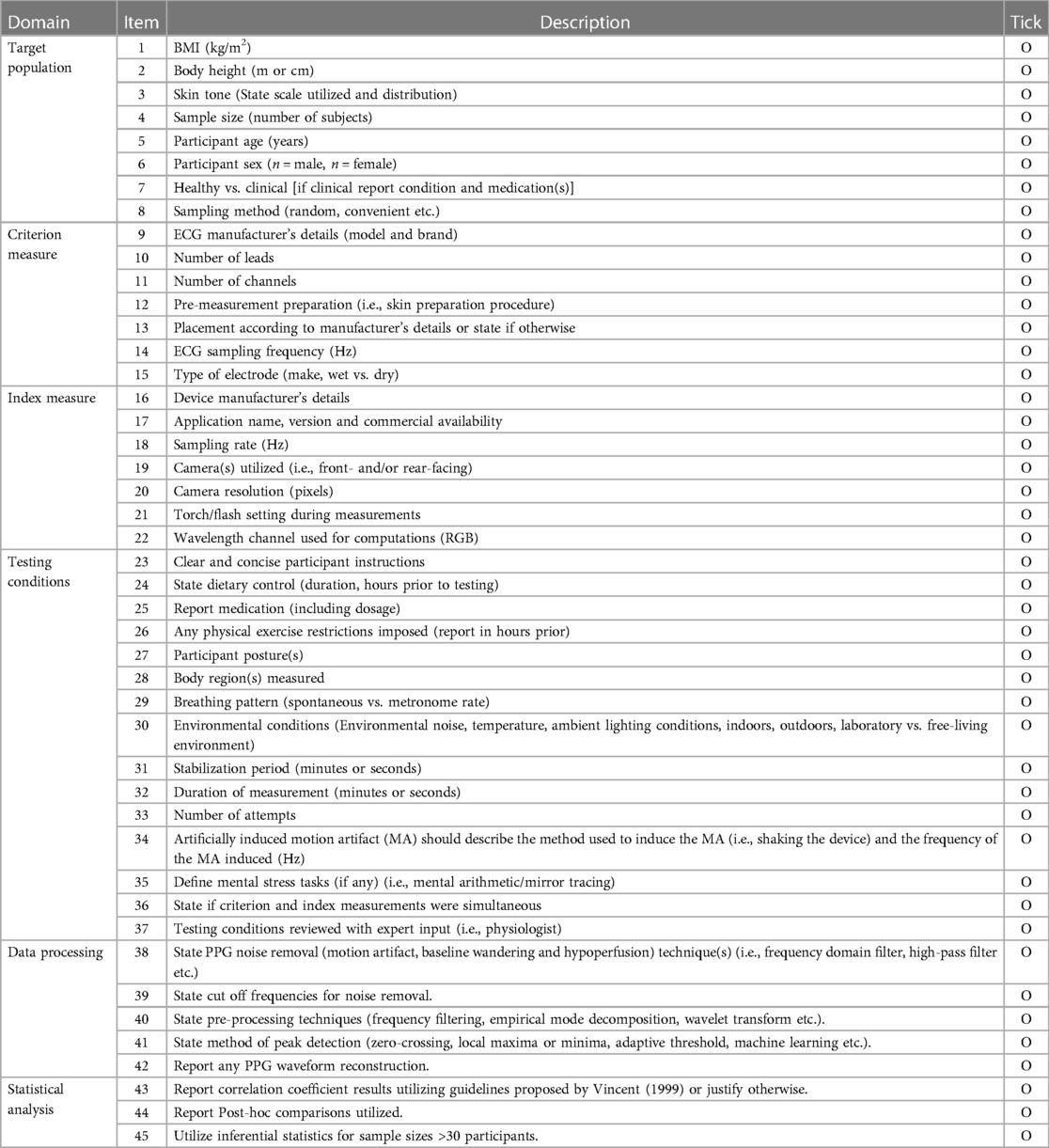
Table 1. Items to consider when reporting validity protocols for the acquisition of RHR via contact-based PPG, using smartphone devices.
Results
Study selection
Following initial database searches, 1,401 articles were identified, and 1,365 titles and abstracts were screened once duplicates (n = 36) were removed. These were screened for inclusion, resulting in 251 full text articles being screened. Of these 247 were excluded and four remained. A further six articles were manually identified by consulting reference lists of the included four articles, resulting in a further six articles, and therefore a total of 10 articles were included in analysis (Figure 1).
Study characteristics
Of the ten studies included in the review, all (100%) reported the country of study, which were upper-middle to high income countries. Sample sizes were reported in all studies (100%) (11, 31–39) and ranged from one to 50 participants. Seven (70%) reported the number of male and female participants, of which most were male, and seven (70%) studies reported participant age (33–39). Only one study (10%) reported participant skin colour (36) and five (50%) reported participants’ health status (11, 34–37) (Table 2).
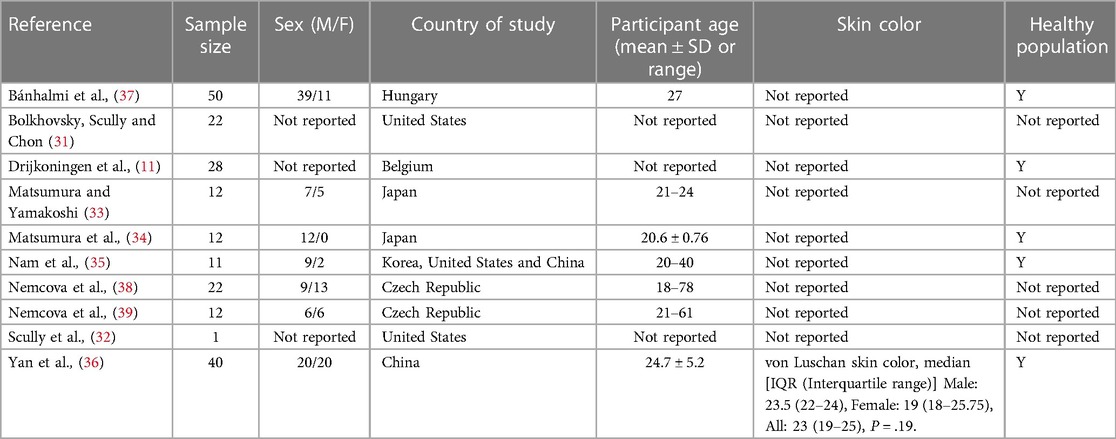
Table 2. General study information of investigations concerning smartphone rear-facing PPG measurement and ECG for the determination of heart rate (pulse rate) and descriptive statistics of participants.
Index measurement characteristics
Index measurement characteristics are displayed in Figure 2A and Table 3 (measurement settings) and Table 4 (device hardware specifications). Eight studies (80%) used a single smartphone for data collection (11, 32–37, 39) and two studies (20%) utilized two or more devices (31, 38). Four articles (40%) stated the name of the smartphone application (33, 34, 36, 38), three (30%) of which were commercially available (33, 34, 38). In the remaining seven studies (70%), commercial availability was not reported (11, 31, 32, 35–37, 39). No studies (0%) reported beat detection algorithm. Eight studies (80%) reported which camera recorded smartphone PPG measurements (32–39) of which the rear-facing camera was utilized for all with torch (flash) turned on. Two studies (20%) failed to report camera location and torch (flash) settings (11, 31). Camera resolution was reported in six studies (60%) (32–35, 37, 39) and varied in resolution. In the remaining studies resolution was not reported (40%) (11, 31, 36, 38). Smartphone sampling rate was reported in nine studies (90%) (11, 31–35, 37–39) and one study (10%) did not report sampling rate (36). Of the nine studies that did report sampling rate six (60%) recorded at 30 Hz (11, 33–35, 38, 39) one (10%) recorded at 30 Hz and 20 Hz, which was dependent on smartphone device (31), one study (10%) recorded at 24.99 Hz (32) and the final study (10%) that reported sampling rate recorded in “slow-motion” capture mode at 240 Hz (37). Nine studies (90%) reported the color channel used during analysis (11, 31–35, 37–39), and in one study (10%) it was not reported (36). Of these studies two studies (20%) utilized red, green and blue color channels (34, 38). Three studies (30%) utilized green only (32, 33, 35). One study (10%) utilized red and green depending on smartphone used (31). Two studies (20%) utilized red only (37, 39). Finally, one study (10%) converted to a single grey value (11).
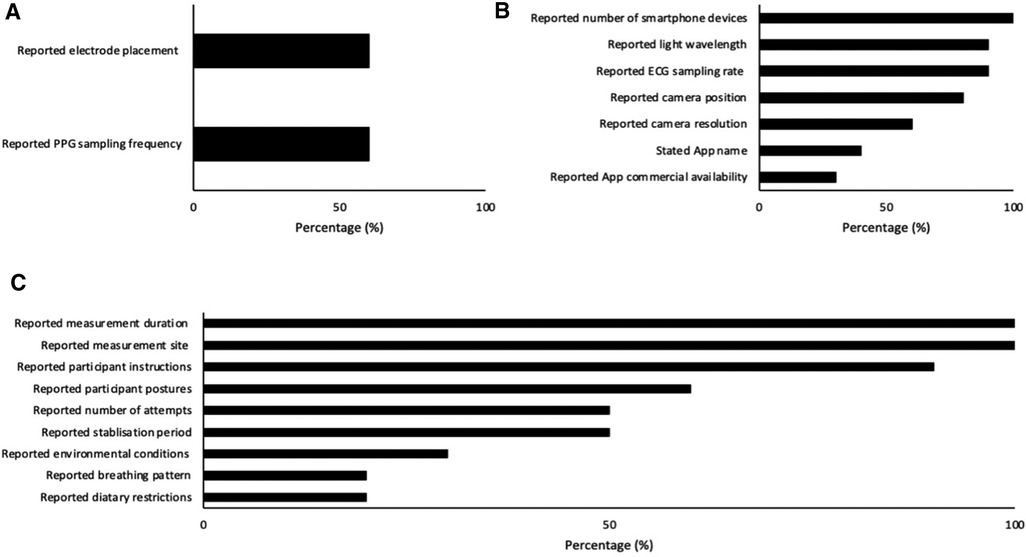
Figure 2. Number of papers reporting index (A), criterion (B) and environmental (C) characteristics.
Criterion measurement characteristics
Criterion measurement characteristics are reported in Figure 2B and Table 5. 12-Lead ECG was used in two studies (20%) (11, 36), three studies (30%) used 5-lead (31, 32, 35), one study (10%) used 4-lead (37), two studies (20%) used 2-lead (33, 34), and two studies (20%) used 1-lead (38, 39). Six studies (60%) reported ECG electrode placement and sampling frequency (32, 34, 35, 37–39).
Experimental procedure characteristics
Environmental procedure characteristics are reported in Figure 2C and Table 6. Nine studies (90%) provided participant instructions (11, 31–38) and two studies (20%) had dietary restrictions (33, 34). Participant postures were stated in six studies (60%) (31, 33–35, 37, 39). Of these studies four (40%) were measured in seated posture (33–35, 37) and two studies (20%) were measured participants in two or more postures (31, 39). All studies (100%) reported measurement site (11, 31–39). Of these studies four (40%) were measured at the index finger (left) (32–35), two (20%) at the index finger (right) (11, 31), and four (40%) at the index finger (left or right not reported) (36–39). Two studies (20%) reported breathing pattern (32, 35) and participants were instructed to breathe at various metronome rates. Environmental conditions were reported in three studies (30%) (33, 34, 36) and not reported in the remaining seven (70%) (11, 31, 32, 35, 37–39). Stabilization period was reported in five studies (50%) (33–37). Of these studies one (10%) allowed participants 10 min for stabilization (33), two studies (20%) allowed participants 5 min (34, 36), one (10%) was permitted 20 s (37), and one (10%) was given an unspecified stabilization period (35). All other studies (50%) did not report stabilization period (11, 31, 32, 38, 39). Measurement duration was reported in all ten studies (100%) (11, 31–39). Finally, the number of attempts allowed per participant was reported in five studies (50%) (31–35). No study reported skin temperature and one study (38) measured contact pressure using the strain gauge array under the screen (3D Touch) but this was for the estimate of BP, and the actual force values were not reported.
Primary outcome measure(s) and results of included studies
Seven studies (70%) reported mean and standard deviation for HR acquisition via criterion ECG (31–36, 38) and eight studies (80%) reported mean and standard deviation for HR acquisition via smartphone PPG measurement (31–36, 38, 39). Only one study (10%) reported the mean difference and its significance between criterion and index measurement (36) (Table 7).
Measures of validity
Correlations were reported in seven studies (70%) (11, 31, 34–38), Limits of agreement (LoA) (Bland-Altman method) were calculated in seven studies (70%) (11, 31, 33–37), ANOVA, Tukey HSD and geometric mean regression (GMR) were utilized concurrently in one study (10%) (34), Wilcoxon ranked sum test, were reported in two studies (20%) (36, 38), paired student t test were reported in one study (10%) (36), one study (10%) reported mean ± SD only (32) and one study (10%) utilized a non-numeric technical validation method of five expert reviewers (39). All studies (100%) (11, 31, 33–39) reported agreement, ranging from good to very strong and correlations ranging from r = .98 to 1, between HR-PPG and HR-ECG utilizing the methods outlined above (Table 8).
Discussion
Principle findings
This scoping review provided an overview of existing literature regarding the acquisition and validity of HR-PPG, in healthy subjects at rest utilizing smartphone devices, with the aim of facilitating improvements in future research and clinical practice. In relation to our objective of assessing the validity of HR-PPG acquisition from PPG measurement utilizing contact-based smartphone devices, this review highlighted several methodological and reporting discrepancies between studies which can lead to different results that do not reflect outcome of comparison (22). As there is currently no consensus on what metric should be used to establish the validity of smartphone-based PPG or under what conditions, the reviewed research appears to have utilized an exploratory approach. However, with the rapid development in technology and an improved understanding of this research area, we have highlighted key considerations for reporting contact-based PPG RHR acquisition with smartphones (Table 1).
Target population considerations
With regards to the general study information reported (Table 2) results revealed only one study (10%) (37) met the suggested guidelines for validating heart rate devices (albeit wearables) suggested by Mühlen et al. (40). Overall reporting was poor with small and unjustified sample sizes, and few studies adequately reported sex, skin color, or age of participants. An expert consensus suggested that studies validating HR-PPG should determine sample size based on an expected mean absolute difference, expected SD of differences and a pre-defined clinical maximum difference needed to obtain a power of 80% or 90% to assess agreement with sufficient precision (41). If no a priori level of “in agreement” is specified a sample size of 45 is recommended (42). However, all but one study had a sample size of n < 45, and therefore results could be under powered (43). We suggest sample sizes should be carefully calculated during study design utilising current guidelines (43) and these calculations should be presented in the methods section.
Comorbidities were poorly reported, particularly those that might affect pulse rate or amplitude, such as arterial stiffening or conditions affecting cardiac electrophysiology (33, 44). At a minimum, studies should report either that participants were free from such health conditions, or clearly state their health conditions, if their aim is to validate HR-PPG in a particular population.
Additionally, there was inadequate reporting of participant skin color within this review. Felix von Luschan chromatic scale (VLCS) (range 1–36) was utilized, which is a validated method of skin color evaluation (45). Skin color is an important consideration during PPG acquisition as skin tone may affect the accuracy of measurements (40). However, a recent systematic review of wrist-word devices, which utilize reflective PPG, stated evidence is inconclusive possibly due to small sample sizes and the requirement for a more objective way of identifying participants' skin tone (46). Nevertheless, authors suggested HR-PPG detection may be less accurate in darker skin tones (46). Since the papers in this review failed to adequately report skin tone this cannot be corroborated with regards to camera-based methods and although Yan at al. (36) measured skin tone (Table 2), participants fell within the mid-range of the skin tone spectrum (range 19–25), with one representing light skin and 36 representing dark skin (45). Consequently, it is not clear if participants' skin tone influenced the results of the studies in the present review. Therefore, human factors such as skin color should be recorded (47) and appropriate light wavelength should be selected (48). Moreover, it is evident that more research is required investigating the effect of darker skin tones on signal quality.
Index measurement considerations
Interestingly, the majority of studies reported the use of a single smartphone device (11, 32–37, 39). This of course maximizes internal validity of each study, but does somewhat hamper ecological validity and generalizability, given the vast options in terms of smartphones at the time of writing. Additionally, since a major advantage of mHealth technologies are their reach, it is advisable to assess the index measurements validity cross-platform, at a minimum of one phone from each. Moreover, the most recent article was Nemcova et al. published in 2021 (28), suggesting future measurements could improve through the utilization of newer technology (14).
As highlighted in the results, heterogeneity existed between smartphone model and application utilized and although authors reported the name of the smartphone application, zero studies reported the specific programming code utilized for beat detection. This could be due to financial, security and/or privacy reasons, as some applications were commercially available. This makes direct comparisons between apps and devices difficult as there is no guarantee two apps used the same code. Additionally, around half of the studies stated the application utilized was developed specifically for the intended research, therefore the algorithm could have been described or the code made available. Consequently, validation of specific algorithms within this review was not possible, this could be feasible in future if algorithms and build versions were explicit (49). Moreover, it is difficult to extrapolate these data to the real world without testing the efficacy of those applications outside stringent conditions of a laboratory. Identification of certain smartphones or applications which produce better PPG signals could lead to improvements in HR measures (23). However, this is difficult as there is currently no consensus on what metric should be used to establish the validity of smartphone-based PPG or under what conditions, therefore protocols vary dramatically. Identifying optimal device(s) and application(s) is difficult. Therefore, we present a checklist (Table 1) to facilitate superior acquisition of HR-PPG via smartphone devices.
Although there has been a considerable increase in the number of mobile apps, many have been designed without regulation regarding development, risk mitigation, and quality control. Therefore, we advise future developers to adhere to the guidelines proposed by Llorens-Vernet and Miro (50), which consist of 36 important criteria and outline standards for mobile health-related applications. These criteria are grouped into eight categories including usability, privacy, security, appropriateness and suitability, transparency and content, safety, technical support and updates, and technology.
Most studies reported which camera recorded smartphone PPG measurements (32–39) of which the rear-facing camera was utilized for all with torch (flash) turned on. However, recent research investigating rear- vs. front-facing PPG smartphone measurement revealed the front-facing camera to be more advantageous when considering greater control over the emitted light and finger detection. It is possible that previous research has not utilized this method as smartphone devices with front-facing camera capabilities are a newer technology that is still under development (14). However, regardless of the camera selected it is advisable to state this as camera selection clearly influences PPG signal quality.
Over half of the studies reported camera resolution (32–35, 37, 39). However, it was not clear if the reported resolution was referring to the smartphone cameras hardware settings or if the resolution was selected through the applications capture settings. Raposo et al., (14) suggest resolution should be set to its minimum value to reduce computational load. Moreover, implementation of interpolation techniques can be used to increase fiducial point detection through improvements in temporal resolution (51). This could influence device selection as future research could utilize devices with theoretically suboptimal resolution. For example, a device that, without adjustment of capture resolution would have high computational load, yet have other PPG performance advantages, we could then manually determine the resolution to the desired level within capture settings (i.e., reducing the capture resolution within the app), potentially improving PPG signal quality, and reducing computational load. For this reason, it is important to report what the resolution is and how it was acquired since newer devices often provide multiple rear-facing lenses, of which some have “slow-motion” technology, providing potentially enhanced sampling rate capabilities.
Smartphone sampling rate was reported in most studies (11, 31–35, 37–39). Sampling rate can be as high as 1,000 Hz for medical equipment (52) however, for most smartphone cameras, it is typically less than 30 Hz (53), which can result in inaccurate waveform analysis (54). As outlined in our results, sampling rate was generally 20–30 Hz. For context, the latest smartphone model in the reviewed studies was the iPhone 6s (released 2015), which has a sampling rate of 30, 60 or 240 Hz, depending on the resolution settings during recording. Implications of inappropriate sampling frequency selection could result in inaccurate waveform analysis (54) and HR-PPG determination. Beres and Hejjel (51) investigated the minimum sampling frequency requirements for HR-PPG parameters in healthy individuals and concluded a minimum of 5 Hz is sufficient without interpolation, for pulse rate determination. However, although lower sampling frequencies minimize the computational load and, as a result, the power consumption consequently extending battery life (51), they can also deteriorate the accuracy of fiducial point detection in HR-PPG and/or HR-ECG, decreasing signal accuracy. Moreover, applications intending on measuring other parameters, for example those related to HRV, would require higher sampling rates with possible interpolation (51). As sampling rate is largely determined by smartphone make/model, we advise future research to utilise devices with higher sampling rate capabilities and/or implement interpolation techniques. When designing an application, it is important to consider the parameter being measured (higher sampling rates required for HRV in comparison to HR analysis) and the target demographic, as applications that are compatible with newer and older smartphone models could provide for broader scope, especially for those in low- and middle-income countries (LMIC) that may not have access to adequate healthcare.
As various wavelengths interact differently with blood and tissues (55), important consideration must be had with regards to wavelength selection (56) (i.e., red, green or blue colour channels). Emerging research suggests green wavelengths demonstrated stronger cardiac pulse signals in comparison with red or blue bands during remote PPG imaging (37). However, this was demonstrated in wrist-worn devices and more research is required in smartphone-derived PPG. Finally, improvements in pulse signal could be attained through optimization of the pixel averaging region (32), whereby the video area closest to the light source is analysed increasing the overall gain of the signal and therefore improving signal quality (14).
Experimental procedure considerations
Firstly, when describing the experimental procedure, studies described the technical computer science methods well. However, their relationship to physiology (i.e., what variable they are measuring and the relationship between the signal capture and the underpinning physiology) was not described in as much detail. Nearly all studies provided participant instructions (11, 31–38), however, some study designs were hard to follow and not enough detail was provided to allow accurate replication. Studies that provided sufficient detail utilized schematic diagrams and detailed subsections within the methods as to index and criterion measurements, experimental procedure, and participant instructions.
Over half the studies reported participant postures with the seated posture being the most frequently utilized measurement position. Postural changes can result in deviations in cardiovascular measurements, such as HR (57, 58). Therefore, participant measurement posture should be reported when describing the experimental procedure. In addition to measurement posture, measurement site is also an important consideration. Hartmann et al. (59) investigated the effect of measurement site on HR-PPG waveform characteristics utilizing a reflective PPG sensor with a peak wavelength of 880 nm, comparable with reflective wavelengths utilized in smartphone devices that utilize an infrared light wavelength (880–940 nm) (6). Authors determined that under normal and deep breathing conditions the finger produced the most analyzable waveforms (95% and 86% analyzable, respectively) in terms of mean amplitude, pulse peak time (Tp), dicrotic notch time (Tn), and the reflection index (RI) (all p < 0.001), which could be due to higher sensitivity to volumetric fluctuations in the cutaneous vascular walls of the finger compared with other measurement sites (59).
The application of pressure at the measurement site is something to be considered, as this is the fundamental of blood pressure measurement (i.e., an increase in pressure eventually results in occlusion). Variations in contact pressure can result in changes in several waveform characteristics (60). Increased contact pressure decreases the optical path length through the tissue, increasing AC amplitude. AC amplitude reaches its maximum when transmural pressure, defined as the difference between intraarterial pressure on the vessel wall and contact pressure, reach zero (61, 62). Additional pressure beyond this begins to occlude the vessel reducing amplitude until no signal is visible. Conversely, contact pressure applied too softly increases the optical path length through the tissue, decreasing AC amplitude. Considering this, applying enough pressure to create conditions where transmural pressure is zero could be beneficial for RHR determination, as this could make peaks more easily identifiable. While this paragraph briefly outlines the underlying physiology and AC amplitude changes form varying contact pressures, from a technical standpoint, Apple stopped incorporating the strain gauge array under the screen (3D Touch) from ∼2017 onwards. Therefore, no force measures can be obtained directly from the device. For this reason, our in-house pilot testing has suggested that providing the app user with the real-time PPG signal (i.e., visual feedback) can enhance the quality of the PPG signal. This approach has been previously conducted by Nemcova et al. (38) who reported they provided app feedback (visual peaks presented on the smartphone display) to enhance signal quality during measurement conditions. These authors stated that quality was evaluated visually by the users; quasi-periodic peaks/spikes must be seen in the signals. A flat signal or a signal with many peaks/spikes with the absence of quasi-periodicity represents a low-quality signal. The user should iteratively change the position of the smartphone according to the feedback of the application. Therefore, applying contact pressure which allows a signal which displays key pulse wave fiducial points, that has many quasi-periodic peaks would be considered ideal.
Previous research stated environmental conditions such as ambient light or motion can influence HR measurement (49, 63). In addition, careful consideration of the environmental temperature has the benefit of reducing possible HR-ECG and HR-PPG noise due to shivering (64). Of course, these environmental conditions ultimately influence participant temperature, and temperature of the measurement site (i.e., skin temperature). However, no study included in this review reported skin temperature. From a technical standpoint, the device temperature sensors are only designed for management of the CPU and battery, so measurement of environmental or skin temperature is beyond the scope of those sensors. Thus, skin temperature reporting would require an additional device such as a skin thermometer. From our in-house pilot testing, we have observed that having cold hands can reduce the quality of the PPG signal (by “quality” we mean a signal which displays key pulse wave fiducial points, that has many quasi-periodic peaks would be considered ideal). This in-house pilot testing in our lab is supported by previous work suggesting that both increased and decreased skin temperature can alter the increased PPG amplitude and total signal, PPG waveform amplitude, and PPT (60, 65–68). Research suggests ambient light may also affect light sensitive diodes; however, the size of the effect is currently unknown (40). Allen (47) suggests correct positioning of the device and the use of light modulation filtering can reduce ambient light interference.
We identified HR-ECG and HR-PPG were generally recorded simultaneously for short durations (<3 min), which is acceptable. Nemcova et al. (39) suggest ultrashort- (< 5 min) and short-term (∼ 5 min) measurements have several advantages over longer term measurements, including minimal risk of data loss during measurement, subject comfort (including flash/torch burn risk) and reduced computational demands that influence battery capacity and memory. Definitions of short- and ultra-short vary depending on the intended research, 10 s duration is commonly cited as the most appropriate duration within the literature, for HR-PPG acquisition. However, although all studies in the current review reported measurement duration (Table 6), no study compared the effect of increased or reduced measurement duration on signal quality. Therefore, the impact of measurement duration in the present review is unclear.
The time taken for a pulse wave to travel along a fixed arterial length is considered the pulse transit time (PTT). When that pulse arrives, known as pulse arrival time (PAT), it is represented by a peak in the HR-PPG signal, however due to the PTT, there is misalignment, or “time lag”, between the R wave of the HR-ECG signal and the HR-PPG peak (69). A recent review of open-source beat detection algorithms describes a method of time alignment where HR-ECG and HR-PPG derived beats within the range of <150 ms were determined to be correctly identified. The time lag between beats was manipulated by offsetting the beats in increments of 20 ms. The time lag that resulted in the most correctly identified beats (the most HR-ECG and HR-PPG beats within the range of <150 ms) was considered the “true lag” (70). Time alignment allows for direct beat comparison and ensures that not only are the same time frames are being analysed but also the same beats, improving validity assessment. However, only Bolkhovsky et al. (31) explicitly stated that HR-ECG and HR-PPG were aligned during post-recording data analysis.
Finally, the number of attempts allowed per participant was inadequately reported (Table 6). Holmes et al. (71) suggest number of attempts should be limited to three as additional measurements would counteract the advantages of ultrashort-term measures outlined above. We argue that there is a compromise to be made between end-user burden/acceptability and reliability/precision. Whilst it is likely that more trials per participant will increase the chances of acquiring a good signal and therefore improve validity, the more trials a user completes the greater the data entry burden (72), which could reduce usability and adherence.
Primary outcome and statistical measures of validity considerations
Results of this scoping review highlight the agreement between HR-PPG and HR-ECG (Table 8). In this scoping review CIs, LoA, or bias [from which LoA can be derived (LoA = bias ± 1.96 SD)] were not reported in all studies. Yet Mühlen et al. (40) state 95% confidence intervals (CIs) and LoAs should be provided for between-device comparisons. Interestingly, given the large number of studies reporting correlation coefficients, zero papers defined guidelines utilized to determine strength of coefficients (73, 74). We conducted post hoc interpretation and six articles (11, 31, 34, 36–38) exceeded the minimum requirements for “high” or “strong” correlation using previously reported guidelines (73, 74). However, it was unclear whether these studies examined mean HR agreement, rather than time alignment and beat to beat agreement.
Nam et al. (35) stated PPG measured from the green wavelength (HR-Green) demonstrated “good agreement” (Table 8) in comparison with HR-ECG, however, neither the coefficient itself, nor the criteria for this qualitative assessment was provided. In summary, statistical interpretation could be improved in future research, utilizing the Bland-Altman method (75) for testing agreement between HR-ECG and HR-PPG, rather than relationship between the two (as agreement and relationship are different concepts). We also propose greater transparency in statistical reporting, including precise coefficients, a priori thresholds for interpretation (i.e., “poor”, “good”, “very good”) etc.
Effects of mobile platform (iOS and android)
It is worth noting that there are technical and practical issues related to the platform (iOS vs. Android) used to collect PPG data. A common approach is splitting the captured image into its primary colour components since red or green channels often provide a better signal. This technique largely hinges on the sensor's colour sensitivity and its colour filter array (CFA) precision. Given the stricter manufacturing control, Apple's iOS devices have a more uniform sensor technology and will likely offer a consistent baseline for PPG measurements between devices. The wider range of Android manufacturers means devices will use sensors from different producers, resulting in a broader range of sensor metrics between devices. The distinct approaches to sensor integration and image processing algorithms may further compound these differences. Apple's control over hardware and software typically results in predictable sensor performance. In contrast, Android devices might exhibit significant variability in sensor behaviour, potentially impacting homogeneity of PPG measurements across devices.
While these differences mean different phones may have different magnitudes of sensor values, the degree to which this impacts peak detection or other variables (such as frequency domain HRV) has yet to be widely investigated. More broadly, both platforms offer frame rates that enable sampling at 30 Hz. While this is suitable for peak detection, more nuanced analyses, such as wave morphology and feature identification, are challenging at this frame rate. Both manufacturers have started to include higher framerate video capture, such as “slow motion” modes with frame rates between 120 and 240 Hz. While these modes may reveal more significant detail in the collected wave data, the validity and reliability of this higher framerate regarding critical variables (e.g., the consistency of the period between frames) is not known.
There are other more practical issues regarding using phones for widescale HR monitoring. While not a significant feature of this review, in our testing (our unpublished observation) we have found that the flash on some Android phones gets uncomfortably hot when used in bulb mode (necessary to generate the PPG data). Similarly, the trend for integrating more lenses into the phone has, in some cases, moved the lenses further from the flash, resulting in less consistent lighting across the tissue in contact with the lens (our unpublished observation).
Conclusions and practical recommendations
To ensure validity and comparability with previous research, we have proposed a framework for optimal reporting (Table 1). This was based on the “Towards Intelligent Health and Well-Being: Network of Physical Activity Assessment” (INTERLIVE) best-practice recommendations (40). We took the INTERLIVE statement for wearable devices and adapted it for phone camera-based PPG. The validation process should consider six domains: the target population, criterion measure, index measure, testing conditions, data processing and the statistical analysis (40). Adherence to the checklist will result in superior acquisition of HR-PPG via smartphone devices, facilitating improvements in research and clinical practice. Future research could investigate validity with consideration towards effective approaches that transfer these methods from laboratory conditions into the “real-world”, in both healthy and clinical populations.
Data availability statement
The original contributions presented in the study are included in the article/Supplementary Material, further inquiries can be directed to the corresponding author.
Author contributions
JDM: Conceptualization, Data curation, Formal Analysis, Investigation, Methodology, Project administration, Resources, Software, Validation, Visualization, Writing – original draft, Writing – review & editing. LDH: Conceptualization, Data curation, Formal Analysis, Investigation, Methodology, Project administration, Resources, Software, Validation, Visualization, Writing – original draft, Writing – review & editing. JLM: Writing – review & editing. NFS: Conceptualization, Data curation, Formal Analysis, Funding acquisition, Investigation, Methodology, Project administration, Resources, Software, Supervision, Validation, Writing – original draft, Writing – review & editing.
Funding
The author(s) declare financial support was received for the research, authorship, and/or publication of this article.
This research was partially funded by the National Research Foundation, Prime Minister's Office, Singapore under its Campus for Research Excellence and Technological Enterprise (CREATE) program and by Future Health Technologies grant money.
Conflict of interest
The authors declare that the research was conducted in the absence of any commercial or financial relationships that could be construed as a potential conflict of interest.
Publisher's note
All claims expressed in this article are solely those of the authors and do not necessarily represent those of their affiliated organizations, or those of the publisher, the editors and the reviewers. Any product that may be evaluated in this article, or claim that may be made by its manufacturer, is not guaranteed or endorsed by the publisher.
References
1. Almarshad MA, Islam MS, Al-Ahmadi S, BaHammam AS. Diagnostic features and potential applications of PPG signal in healthcare: a systematic review. Healthcare. (2022) 10:547. doi: 10.3390/healthcare10030547
2. Alnaeb ME, Crabtree VP, Boutin A, Mikhailidis DP, Seifalian AM, Hamilton G. Prospective assessment of lower-extremity peripheral arterial disease in diabetic patients using a novel automated optical device. Angiology. (2007) 58:579–85. doi: 10.1177/0003319707305685
3. Hertzman AB. Photoelectric plethysmography of the fingers and toes in man. Exp Biol Med. (1937) 37:529–34. doi: 10.3181/00379727-37-9630
4. Hertzman AB. The blood supply of various skin areas as estimated by the photoelectric plethysmograph. Am J Physiol-Leg Content. (1938) 124:328–40. doi: 10.1152/ajplegacy.1938.124.2.328
5. Challoner AVJ, Ramsay CA. A photoelectric plethysmograph for the measurement of cutaneous blood flow. Phys Med Biol. (1974) 19:317–28. doi: 10.1088/0031-9155/19/3/003
6. Park J, Seok HS, Kim S-S, Shin H. Photoplethysmogram analysis and applications: an integrative review. Front Physiol. (2022) 12:808451. doi: 10.3389/fphys.2021.808451
7. Li K, Zhang S, Yang L, Jiang H, Chi Z, Wang A, et al. Changes of arterial pulse waveform characteristics with gestational age during normal pregnancy. Sci Rep. (2018) 8:15571. doi: 10.1038/s41598-018-33890-1
8. Venema B, Blanik N, Blazek V, Gehring H, Opp A, Leonhardt S. Advances in reflective oxygen saturation monitoring with a novel in-ear sensor system: results of a human hypoxia study. IEEE Trans Biomed Eng. (2012) 59:2003–10. doi: 10.1109/TBME.2012.2196276
9. Wannenburg J, Malekian R. Body sensor network for mobile health monitoring, a diagnosis and anticipating system. IEEE Sens J. (2015) 15:6839–52. doi: 10.1109/JSEN.2015.2464773
10. Mayerhöfer TG, Pahlow S, Popp J. The bouguer-beer-lambert law: shining light on the obscure. ChemPhysChem. (2020) 21:2029–46. doi: 10.1002/cphc.202000464
11. Drijkoningen L, Lenaerts F, Vandervoort P, Grieten L. Validation of a smartphone based photoplethysmographic beat detection algorithm for normal and ectopic complexes. a smartphone based photoplethysmographic beat.
12. Goërtz YMJ, Braamse AMJ, Spruit MA, Janssen DJA, Ebadi Z, Van Herck M, et al. Fatigue in patients with chronic disease: results from the population-based lifelines cohort study. Sci Rep. (2021) 11:20977. doi: 10.1038/s41598-021-00337-z
13. Mair JL, Hayes LD, Campbell AK, Sculthorpe N. Should we use activity tracker data from smartphones and wearables to understand population physical activity patterns? J Meas Phys Behav. (2022) 1:1–5. doi: 10.1123/jmpb.2021-0012
14. Raposo A, da Silva HP, Sanches J. Camera-based photoplethysmography (cbPPG) using smartphone rear and frontal cameras: an experimental study. 2021 43rd Annu Int Conf IEEE Eng Med Biol Soc EMBC. Mexico: IEEE; 2021. p.7091–4. DOI: 10.1109/EMBC46164.2021.9630847.
15. Kim BH, Glanz K. Text messaging to motivate walking in older African Americans. Am J Prev Med. (2013) 44:71–5. doi: 10.1016/j.amepre.2012.09.050
17. Weitz TA, Freund KM, Wright L. Identifying and caring for underserved populations: experience of the national centers of excellence in women’s health. J Womens Health Gend Based Med. (2001) 10:937–52. doi: 10.1089/152460901317193521
18. Hayes LD, Ingram J, Sculthorpe NF. More than 100 persistent symptoms of SARS-CoV-2 (long COVID): a scoping review. Front Med. (2021) 8. doi: 10.3389/fmed.2021.750378
19. McLaughlin M, Cerexhe C, Macdonald E, Ingram J, Sanal-Hayes NEM, Hayes LD, et al. A cross-sectional study of symptom prevalence, frequency, severity, and impact of long-COVID in Scotland: part I. Am J Med. (2023). doi: 10.1016/j.amjmed.2023.07.004
20. Goal 3: Department of Economic and Social Affairs. Available online at: https://sdgs.un.org/goals/goal3 (cited Aug 19, 2023).
21. Asi YM, Williams C. The role of digital health in making progress toward sustainable development goal (SDG) 3 in conflict-affected populations. Int J Med Inf. (2018) 114:114–20. doi: 10.1016/j.ijmedinf.2017.11.003
22. Vandenberk T, Stans J, Mortelmans C, Van Haelst R, Van Schelvergem G, Pelckmans C, et al. Clinical validation of heart rate apps: mixed-methods evaluation study. JMIR MHealth UHealth. (2017) 5:e129. doi: 10.2196/mhealth.7254
23. De Ridder B, Van Rompaey B, Kampen JK, Haine S, Dilles T. Smartphone apps using photoplethysmography for heart rate monitoring: meta-analysis. JMIR Cardio. (2018) 2:e4. doi: 10.2196/cardio.8802
24. Gonzales TI, Jeon JY, Lindsay T, Westgate K, Perez-Pozuelo I, Hollidge S, et al. Resting heart rate is a population-level biomarker of cardiorespiratory fitness: the fenland study. PloS One. (2023). doi: 10.1371/journal.pone.0285272
25. Smartphone ownership by age 2012-2023. Statista. Available online at: https://www.statista.com/statistics/271851/smartphone-owners-in-the-united-kingdom-uk-by-age/ (cited Jan 25, 2024).
26. Silver L. Smartphone ownership is growing rapidly around the world, but not always equally. Pew Res Cent Glob Attitudes Proj. (2019). Available online at: https://www.pewresearch.org/global/2019/02/05/smartphone-ownership-is-growing-rapidly-around-the-world-but-not-always-equally/
27. Mercuri M, Lorato IR, Liu Y-H, Wieringa F, Hoof CV, Torfs T. Vital-sign monitoring and spatial tracking of multiple people using a contactless radar-based sensor. Nat Electron. (2019) 2:252–62. doi: 10.1038/s41928-019-0258-6
28. Amelard R, Scharfenberger C, Kazemzadeh F, Pfisterer KJ, Lin BS, Clausi DA, et al. Feasibility of long-distance heart rate monitoring using transmittance photoplethysmographic imaging (PPGI). Sci Rep. (2015) 5:14637. doi: 10.1038/srep14637
29. Coppetti T, Brauchlin A, Müggler S, Attinger-Toller A, Templin C, Schönrath F, et al. Accuracy of smartphone apps for heart rate measurement. Eur J Prev Cardiol. (2017) 24:1287–93. doi: 10.1177/2047487317702044
30. Tricco AC, Lillie E, Zarin W, O’Brien KK, Colquhoun H, Levac D, et al. PRISMA extension for scoping reviews (PRISMA-ScR): checklist and explanation. Ann Intern Med. (2018) 169:467–73. doi: 10.7326/M18-0850
31. Bolkhovsky JB, Scully CG, Chon KH. Statistical analysis of heart rate and heart rate variability monitoring through the use of smart phone cameras. 2012 Annu Int Conf IEEE Eng Med Biol Soc. San Diego, CA: IEEE; 2012. p. 1610–3. DOI: 10.1109/EMBC.2012.6346253.
32. Scully CG, Selvaraj N, Romberg FW, Wardhan R, Ryan J, Florian JP, et al. Using time-frequency analysis of the photoplethysmographic waveform to detect the withdrawal of 900 ml of blood. Anesth Analg. (2012) 115:74–81. doi: 10.1213/ANE.0b013e318256486c
33. Matsumura K, Yamakoshi T. Iphysiometer: a new approach for measuring heart rate and normalized pulse volume using only a smartphone. Behav Res Methods. (2013) 45:1272–8. doi: 10.3758/s13428-012-0312-z
34. Matsumura K, Rolfe P, Lee J, Yamakoshi T. Iphone 4s photoplethysmography: which light color yields the most accurate heart rate and normalized pulse volume using the iPhysioMeter application in the presence of motion artifact? PloS One. (2014) 9:e91205. doi: 10.1371/journal.pone.0091205
35. Nam Y, Kong Y, Reyes B, Reljin N, Chon KH. Monitoring of heart and breathing rates using dual cameras on a smartphone. PloS One. (2016) 11:e0151013. doi: 10.1016/j.ifacol.2015.10.166
36. Yan BP, Chan CK, Li CK, To OT, Lai WH, Tse G, et al. Resting and postexercise heart rate detection from fingertip and facial photoplethysmography using a smartphone camera: a validation study. JMIR MHealth UHealth. (2017) 5:e33. doi: 10.2196/mhealth.7275
37. Bánhalmi A, Borbás J, Fidrich M, Bilicki V, Gingl Z, Rudas L. Analysis of a pulse rate variability measurement using a smartphone camera. J Healthc Eng. (2018) 2018:4038034. doi: 10.1155/2018/4038034
38. Nemcova A, Jordanova I, Varecka M, Smisek R, Marsanova L, Smital L, et al. Monitoring of heart rate, blood oxygen saturation, and blood pressure using a smartphone. Biomed Signal Process Control. (2020) 59:101928. doi: 10.1016/j.bspc.2020.101928
39. Nemcova A, Vargova E, Smisek R, Marsanova L, Smital L, Vitek M. Brno university of technology smartphone PPG database (BUT PPG): annotated dataset for PPG quality assessment and heart rate estimation. BioMed Res Int. (2021) 2021:3453007. doi: 10.1155/2021/3453007
40. Mühlen JM, Stang J, Lykke Skovgaard E, Judice PB, Molina-Garcia P, Johnston W, et al. Recommendations for determining the validity of consumer wearable heart rate devices: expert statement and checklist of the INTERLIVE network. Br J Sports Med. (2021) 55:767–79. doi: 10.1136/bjsports-2020-103148
41. Lu M-J, Zhong W-H, Liu Y-X, Miao H-Z, Li Y-C, Ji M-H. Sample size for assessing agreement between two methods of measurement by bland−altman method. Int J Biostat. (2016) 12:20150039. doi: 10.1515/ijb-2015-0039
42. Carstensen B. Comparing Clinical Measurement Methods: A Practical Guide. Chichester: Wiley (2010).
43. Serdar CC, Cihan M, Yücel D, Serdar MA. Sample size, power and effect size revisited: simplified and practical approaches in pre-clinical, clinical and laboratory studies. Biochem Medica. (2021) 31:27–53. doi: 10.11613/BM.2021.010502
44. Allen J, Murray A. Age-related changes in peripheral pulse timing characteristics at the ears, fingers and toes. J Hum Hypertens. (2002) 16:711–7. doi: 10.1038/sj.jhh.1001478
45. Treesirichod A, Chansakulporn S, Wattanapan P. Correlation between skin color evaluation by skin color scale chart and narrowband reflectance spectrophotometer. Indian J Dermatol. (2014) 59:339–42. doi: 10.4103/0019-5154.135476
46. Koerber D, Khan S, Shamsheri T, Kirubarajan A, Mehta S. Accuracy of heart rate measurement with wrist-worn wearable devices in Various skin tones: a systematic review. J Racial Ethn Health Disparities. (2022) 10:2676–84. doi: 10.1007/s40615-022-01446-9
47. Allen J. Photoplethysmography and its application in clinical physiological measurement. Physiol Meas. (2007) 28:R1–39. doi: 10.1088/0967-3334/28/3/R01
48. Fallow BA, Tarumi T, Tanaka H. Influence of skin type and wavelength on light wave reflectance. J Clin Monit Comput. (2013) 27:313–7. doi: 10.1007/s10877-013-9436-7
49. Bruining N, Caiani E, Chronaki C, Guzik P, Van Der Velde E. Acquisition and analysis of cardiovascular signals on smartphones: potential, pitfalls and perspectives: by the task force of the e-cardiology working group of European society of cardiology. Eur J Prev Cardiol. (2014) 21:4–13. doi: 10.1177/2047487314552604
50. Llorens-Vernet P, Miró J. Standards for mobile health–related apps: systematic review and development of a guide. JMIR MHealth UHealth. (2020) 8:e13057. doi: 10.2196/13057
51. Béres S, Hejjel L. The minimal sampling frequency of the photoplethysmogram for accurate pulse rate variability parameters in healthy volunteers. Biomed Signal Process Control. (2021) 68:102589. doi: 10.1016/j.bspc.2021.102589
52. Kok BE, Coffey KA, Cohn MA, Catalino LI, Vacharkulksemsuk T, Algoe SB, et al. How positive emotions build physical health: perceived positive social connections account for the upward spiral between positive emotions and vagal tone. Psychol Sci. (2013) 24:1123–32. doi: 10.1177/0956797612470827
53. Peng R-C, Zhou X-L, Lin W-H, Zhang Y-T. Extraction of heart rate variability from smartphone photoplethysmograms. Comput Math Methods Med. (2015) 2015:516826.25685174
54. Liu I, Ni S, Peng K. Enhancing the robustness of smartphone photoplethysmography: a signal quality Index approach. Sensors. (2020) 20:E1923. doi: 10.3390/s20071923
55. Biswas D, Simoes-Capela N, Van Hoof C, Van Helleputte N. Heart rate estimation from wrist-worn photoplethysmography: a review. IEEE Sens J. (2019) 19:6560–70. doi: 10.1109/JSEN.2019.2914166
56. Vizbara V. Comparison of green, blue and infrared light in wrist and forehead photoplethysmography. Biomed Eng OnLine. (2013) 17.
57. Verkruysse W, Svaasand LO, Nelson JS. Remote plethysmographic imaging using ambient light. Opt Express. (2008) 16:21434. doi: 10.1364/OE.16.021434
58. Wang W, den Brinker AC. Camera-based respiration monitoring. In: Wang W, Wang X, editors. Contactless Vital Signs Monitoring. Amsterdam: Elsevier (2022). p. 79–97. doi: 10.1016/B978-0-12-822281-2.00012-3
59. Hartmann V, Liu H, Chen F, Qiu Q, Hughes S, Zheng D. Quantitative comparison of photoplethysmographic waveform characteristics: effect of measurement site. Front Physiol. (2019) 10:00198. doi: 10.3389/fphys.2019.00198
60. Fine J, Branan KL, Rodriguez AJ, Boonya-ananta T, Ajmal Ramella-Roman JC, et al. Sources of inaccuracy in photoplethysmography for continuous cardiovascular monitoring. Biosensors. (2021) 11:126. doi: 10.3390/bios11040126
61. Yamakoshi K, Shimazu H, Shibata M, Kamiya A. New oscillometric method for indirect measurement of systolic and mean arterial pressure in the human finger. Part 1: model experiment. Med Biol Eng Comput. (1982) 20:307–13. doi: 10.1007/BF02442797
62. Forster FK, Turney D. Oscillometric determination of diastolic, mean and systolic blood pressure–a numerical model. J Biomech Eng. (1986) 108:359–64. doi: 10.1115/1.3138629
63. Boulos M, Wheeler S, Tavares C, Jones R. How smartphones are changing the face of mobile and participatory healthcare: an overview, with example from eCAALYX. Biomed Eng OnLine. (2011) 10:24. doi: 10.1186/1475-925X-10-24
64. Sattar Y, Chhabra L. Electrocardiogram. Treasure Island (FL): StatPearls Publishing (2023). Available online at: http://www.ncbi.nlm.nih.gov/books/NBK549803/
65. Allen J, Frame JR, Murray A. Microvascular blood flow and skin temperature changes in the fingers following a deep inspiratory gasp. Physiol Meas. (2002) 23:365. doi: 10.1088/0967-3334/23/2/312
66. Lindberg LG, Tamura T, Öberg PÅ. Photoplethysmography. Med Biol Eng Comput. (1991) 29:40–7. doi: 10.1007/BF02446294
67. Khan M, Pretty CG, Amies AC, Elliott R, Shaw GM, Chase JG. Investigating the effects of temperature on photoplethysmography. IFAC-Pap. (2015) 48:360–5. doi: 10.1016/j.ifacol.2015.10.166
68. Zhang X-Y, Zhang Y-T. The effect of local mild cold exposure on pulse transit time. Physiol Meas. (2006) 27:649. doi: 10.1088/0967-3334/27/7/008
69. Zhou Z-B, Cui T-R, Li D, Jian J-M, Li Z, Ji S-R, et al. Wearable continuous blood pressure monitoring devices based on pulse wave transit time and pulse arrival time: a review. Materials (Basel). (2023) 16:2133. doi: 10.3390/ma16062133
70. Charlton PH, Kotzen K, Mejía-Mejía E, Aston PJ, Budidha K, Mant J, et al. Detecting beats in the photoplethysmogram: benchmarking open-source algorithms. Physiol Meas. (2022) 43:085007. doi: 10.1088/1361-6579/ac826d
71. Holmes CJ, Fedewa MV, Winchester LJ, MacDonald HV, Wind SA, Esco MR. Validity of smartphone heart rate variability pre- and post-resistance exercise. Sensors. (2020) 20:E5738. doi: 10.3390/s20205738
72. Mathews SC, McShea MJ, Hanley CL, Ravitz A, Labrique AB, Cohen AB. Digital health: a path to validation. Npj Digit Med. (2019) 2:38. doi: 10.1038/s41746-019-0111-3
Keywords: photoplethysmography, PPG, mobile, heart rate, validity
Citation: Mather JD, Hayes LD, Mair JL and Sculthorpe NF (2024) Validity of resting heart rate derived from contact-based smartphone photoplethysmography compared with electrocardiography: a scoping review and checklist for optimal acquisition and reporting. Front. Digit. Health 6:1326511. doi: 10.3389/fdgth.2024.1326511
Received: 23 October 2023; Accepted: 8 February 2024;
Published: 29 February 2024.
Edited by:
Francesco Onorati, Takeda Pharmaceuticals, United StatesReviewed by:
Kausik Basak, JIS Institute of Advanced Studies and Research, IndiaXiaoman Xing, Chinese Academy of Sciences (CAS), China
© 2024 Mather, Hayes, Mair and Sculthorpe. This is an open-access article distributed under the terms of the Creative Commons Attribution License (CC BY). The use, distribution or reproduction in other forums is permitted, provided the original author(s) and the copyright owner(s) are credited and that the original publication in this journal is cited, in accordance with accepted academic practice. No use, distribution or reproduction is permitted which does not comply with these terms.
*Correspondence: Jacqueline L. Mair jacqueline.mair@sec.ethz.ch