- School of Nursing and Public Health, The University of Dodoma, Dodoma, Tanzania
Background: Tanzania has shown some improvements in the adoption of electronic medical record (EMR) systems in public health facilities; however, the rate of utilization of data generated from EMRs among health managers is not well documented. This study aims to assess the use of electronic medical record systems data in decision-making among health managers at public primary health facilities in Dodoma Region, Central Tanzania.
Methods: A facility-based quantitative cross-sectional analytical study was conducted among 308 randomly selected health managers. A self-administered questionnaire supplemented with documentary review was used. Descriptive summary statistics and bivariable and multivariable logistic regression analyses (crude and adjusted odds ratios) were used. A P-value of <0.05 was used to declare statistically significant associations.
Results: Overall, more than a third (40.6%) of the health managers, that is 174 of the 308 included in the study, reported using data generated by EMR systems in decision-making. One-third (33.4%) of the health managers were adequately using data generated by EMR systems, of which 39.3% used data to support continuous quality improvement initiatives. Among the facilities visited, only nine (30%) had good documented EMR systems data use. Access to computers [adjusted odds ratio (AOR) = 4.72, 95% confidence interval (CI): 1.65, 13.48, p-value (p) = 0.004] and discussions on EMRs during meetings (AOR = 2.77, 95% CI: 1.01, 7.58, p = 0.047) were independent predictors of EMR system data use. Those who reported having EMR systems in all working areas were seven times more likely to use EMR system data (AOR = 7.23, 95% CI: 3.15, 16.59, p = 0.001). The respondents with good perceived EMR system information quality were more likely to use EMR system data (AOR = 2.84, 95% CI: 1.50, 5.39, p = 0.001) than those with poor perception. Furthermore, health managers who had excellent knowledge of computers and data use had higher odds of using EMR system data (AOR = 1.84, 95% CI: 3.38, 10.13, p = 0.001) compared with their counterparts.
Conclusions: The findings of this study indicate that utilization of EMR system data in decision-making among health managers was optimal. It was found that training in itself is insufficient to improve use of EMR, which points to more organizational aspects of work routine as a challenge. Hence, a comprehensive approach that addresses these factors is essential for maximizing EMR system data use in decision-making.
1. Introduction
Electronic medical record (EMR) system, which is an integral part of the larger health management information system (HMIS), is a software that records health-related information of patients on computers. It is a program that collects, manages, and generates data that are used by authorized workers within health facilities. EMR systems provide substantial benefits to health facilities and organizations in patient management and are quickly replacing paper-based systems. They solve many of the limitations of paper-based systems and have proved to be cost-effective in improving the quality of health services (1). They improve the accessibility of health records as well as the quality and accuracy of patient information. Given these benefits, institutions have widely adopted EMR systems in healthcare globally (2).
Previously, all tasks related to the administration and management of patients, such as human resources, procurement, and clinical management, were managed using paper-based record-keeping systems, which proved to be inefficient in terms of information retrieval, security, and data quality and did not allow concurrent data access. However, paper-based systems are still used by health facilities largely because many facilities have not installed EMR systems and the health workers are more familiar with paper-based records due to the long-term use and the fact that they do not require a high level of technical knowledge and skills compared with the electronic systems (1).
An EMR system is a powerful tool for improving clinical and administrative/managerial decision-making by providing access to accurate and relevant information (3). It provides a comprehensive way to manage patients and streamline administrative tasks, and is one of the best healthcare analytics solutions as it collects and analyzes patient data to identify trends and patterns and develop predictive models (4). The increasing integration of highly diversified technologies in the healthcare field has resulted in the need for such an organized gathering of accurate data for informed decision-making in the health sector (5).
Globally there is an increasing volume of health-related data being generated, accelerating the trend towarddigitalization in health. However, there are persistent data gaps and fragmented approaches to the governance of health data in different contexts, which has contributed to inadequate data use (6). EMR systems have begun to be widely used in healthcare facilities worldwide as a data collection and aggregation tool (7). A systematic review of publications from 15 Sub-Saharan African countries revealed that about 91% reported the use of open-source healthcare software. However, the use of EMR systems in low-income countries remains in its initial stages (8, 9).
In developed countries like the USA, 94% of the hospitals use electronic health records (EHR) data in decision-making, such as for quality improvement (82%), monitoring patient safety (81%), and measuring organizational performance (77%). EHR data were least commonly used to develop approaches to query for patient data (51%), assess adherence to clinical practice guidelines (59%), and identify care gaps for specific patient populations (60%). While EHRs are used by multiple care providers and health organizations, hospital characteristics (public, private, rural, and urban) significantly impact the use of EMR data. However, there was substantial variation in the use of EHR data (10).
In Sub-Saharan Africa, a study conducted in a Malawian hospital revealed that utilization of EMR functionalities varied among departments as well as among users. Health facility workers used half of the system functions, and the most commonly used were capturing demographics (82.9%) and capturing and assessing clinical data (68.8%). These functions were frequently used because they applied to almost all the patients who visited the hospital. Gender, age, and previous computer use did not influence EMR systems usage. However, education and employment levels were predictors of EMR systems use (1).
In Tanzania, public health facilities use different EMR systems; some of the EMR systems used include the government of Tanzania hospital management information system (GoTHOMIS), Jeeva, Afya Care, and CTC2 database (11, 12). An EMR system is intended to improve and strengthen electronic data capture at the point of care to improve patient clinical care and facility management. The use of electronic data systems has led to improvements in the quality of health services delivery, including improvement in revenue collection, human resource management, supply chain management, health information management, and improved planning and decision-making at different levels of the health system (13, 14).
A study conducted in Tanzania on paper-based HMIS data use in decision-making revealed that about 56.9% of the facilities had functional HMIS, 18% of the facilities had used their data for planning and services improvement, 26.3% had disseminated data, and about 9.1% of the facilities had proper medical records. The level of the facility was associated with the use of data, with hospitals and health centers showing higher use (15). Another study (16) on paper-based data use in decision-making revealed that 60% of the respondents reported using HMIS data in decision-making, of which data was most used to compare service coverage (53%).
The government of Tanzania, through its fifth Health Sector Strategic Plan (HSSP V), has set the priority to improve the application of digital health technologies. The aim is to facilitate the attainment of high-quality health services to all citizens. Despite this, very few health facilities have installed and made use of EMR systems as data gathering and aggregation tools. Expansion of the system to all government-owned facilities is a priority (14). Owing to the limited resources, most electronic systems are still being used side by side with paper documentation, which is creating a burden on the healthcare workers (2, 17).
HSSP V prioritizes the application of digital health technologies, including the use of EMR systems in public health facilities (14). The government, through support from implementing partners, started the development and implementation of comprehensive electronic medical record systems in 2015 (18). As of now, the AfyA Care and GoT-HoMIS have been approved for scale-up country wide (19). Currently, the GoT-HoMIS system has been deployed in 1,424 healthcare facilities (20). Despite EMR systems supporting data synthesis and visualizations, the use of EMR systems in decision-making in Tanzania is still inadequate (13, 21).
The increasing adoption of the EMR system in Tanzania as a data collection and aggregation tool should be implemented together with improvements in the capacity of health managers to harness its potential. The weakness in general managerial capacity of the health system has been cited as one of the contributory factors in failing to scale up effective health interventions. Hence, understanding how health facilities are currently using data generated by EMR systems in decision-making is important as one of the policy initiatives (14). A study (16) on paper-based systems revealed that 60% of health facility workers and 38.5% of district officials use routine health information for decision-making, with the majority using it in comparing service coverage and monitoring disease trends over time and health promotion activities.
In 2023, the government introduced a data use toolkit for the health sector that insists that the availability of timely, retrievable, and accessible quality data is a cornerstone of all health systems. The health facilities are advised to conduct regular data review meetings (weekly or monthly) to understand their data, identify challenges, and set action items to improve health services. The health facility management team (HMT) has a role in conducting data use review meetings, monitoring the use of data, ensuring evidence-based planning and documentation of data use activities, and increasing the capacity of health workers in data use skills. One of the data sources insisted on in this guideline is EMR systems such as GoT-HoMIS (22).
Despite large investments to support the adoption of EMR systems in Tanzania, so far limited studies have been conducted to give an insight into the acceptance and use of data generated by EMR systems in decision-making in the study area. Therefore, this study aims to fill the knowledge gap related to EMR data utilization in decision-making and its associated factors among health facility managers in the public primary health facilities in Dodoma Region, Central Tanzania.
1.1. Conceptual framework
In this study, the Performance of Routine Information System Management Series (PRISM) framework was conceptualized and adapted in establishing the association between the individual, organizational, behavioral, and technical factors related with EMR systems and the utilization of the systems data in decision-making. The PRISM framework was modified to include individual factors that can influence data use in decision-making, such as age, sex, work experience, level of education, and managerial experience.
Other determinants of data use in decision-making such as organizational (governance, planning, training, supervision, quality, promotion of culture of information, availability of resources), technical [complexity of reporting forms, health information system (HIS) design, computer software], and behavioral (level of knowledge of contents of EMRs, data quality checking skills, problem solving for HIS tasks, competence in HIS tasks, confidence) factors were adopted as per the original PRISM model (Figure 1).
The PRISM framework was adapted because it has been used by other scholars to study EMR system data use in Ethiopia (2) and Malawi (1).
2. Methods
2.1. Study design and setting
This is a facility-based cross-sectional analytical study design that uses a quantitative approach. This study was supplemented by document review to ascertain the existing EMR system data use practices in decision-making at the facility level. It was conducted in Dodoma Region, which is among the regions with an increasing trend in coverage of facilities utilizing EMR systems (18). Dodoma Region is one of the 31 administrative regions of Tanzania and it is located in Central Tanzania. It has a total of eight district councils. The region also has a total of 497 health facilities, including 26 hospitals, 69 health centers, and 402 dispensaries (23). Among the health facilities, 35 public primary health facilities have installed and use EMR systems, of which seven are district hospitals, 24 are health centers, and four are dispensaries (24).
2.2. Study participants
The study participants of this study were health facility managers randomly selected across 30 public primary health facilities with functional EMR systems. A sample size of 315 was estimated with an assumption of a 95% confidence interval, a 5% significance level, and a standard normal deviation of 1.96. The proportion of EMR utilization was set at 26.6% (2). A total of 308 health managers were eventually included in the data collection process. The eligibility criteria for participation were as follows: (1) position: health managers working in public primary health facilities with functional EMR systems, and (2) age: 18 years or older. The exclusion criteria included (1) not consenting to participate in the study, (2) using program-specific EMR systems such as CTC2 database, and (3) being on leave during the period of data collection.
A stratified sampling technique with proportionate allocation to each facility was employed. To recruit 315 participants from the selected facilities, Neyman's allocation formula as cited by Mathew (25) was used:
where nh is the sample size for stratum h, n is the total sample size, Nh is the population size per facility, and N is the total population.
Samples were distributed according to their respective strata (hospital, health centre, or dispensary). The sample size per facility, Nh, was determined by using the recommended number of HMT members, which for the hospital and health center is 14 members each and for the dispensary is nine members (26). Hence, the population of participants was sampled as shown (Figure 2).
2.3. Data collection methods
A structured self-administered questionnaire supplemented with documentary review was used to collect data on sociodemographic, organizational, behavioral, and technical factors as well as knowledge and attitudes regarding the use of EMRs. The tool was adapted from and constructed using PRISM (27) and was based on previous studies (2). The questionnaire was administered in English. Before administration, the questionnaire was designed using Kobo toolbox software, and diploma holder nurses were involved in administering the tool after 2 days of training.
A document review checklist was used to assess the existing EMR use in decision-making at the facility level over the past 6 months. The documents reviewed included HMT meeting minutes, commodity audit reports, commodity procurement reports, and annual facility planning reports. To ensure good data quality and avoid problems of missing data, the recruitment team were trained for 2 days. The respondents who were absent during data collection were repeatedly visited to minimize the rate of high non-responses.
2.4. Variable measurements
2.4.1. Knowledge of computer and data use
This was assessed by using a 10 question score as follows: internet browsing, making calculations, sending email communication, managing the EMR database, checking accuracy of data, plotting data by months or years, computing trends from bar charts, explaining findings and their implications, using data for identifying gaps and setting targets, using data for making various decisions, and providing feedback. A mean score above or equal to 90 denoted excellent, 80–<90, very good, 60–<80, good, 50–<60, fair, and less than or equal to 50, poor (2).
2.4.2. Attitude
This was assessed by using eight questions that could be answered on a scale (strongly disagree, disagree, neutral, agree, strongly agree). Those who scored an aggregate of above 32 indicated a positive attitude (good), and those who scored below 32 were considered as having a negative attitude (bad) (2).
2.4.3. Perceived EMR system quality
This was assessed by using five questions that could be answered on a scale (strongly disagree, disagree, neutral, agree, strongly agree). Those who scored an aggregate score of above or equal to 20 indicated a positively perceived EMR system quality (good), and those who scored below 20 were considered as perceiving a negative EMR system quality (poor) (2).
2.4.4. Perceived EMR service quality
This was assessed by using nine questions that could be answered on a scale (strongly disagree, disagree, neutral, agree, strongly agree). Those who scored an aggregate score of above or equal to 36 indicated a positively perceived EMR service quality (good) and those who scored below 36 were considered as perceiving a negative EMR system service quality (poor) (2).
2.4.5. Perceived EMR information quality
This was assessed by using seven questions that could be answered on a scale (strongly disagree, disagree, neutral, agree, strongly agree). Those who scored an aggregate score of above or equal to 28 indicated a positively perceived EMR information quality (good) and those who scored below 28 were considered as perceiving a negative EMR system information quality (bad) (2).
2.4.6. EMR use in decision-making (health manager)
This was defined as having ever used EMR system data in decision-making, and the extent of use was assessed by a question with 10 items on the participants use of the EMR to perform one or more of the following clinical and administrative/managerial functions: (1) support quality improvement (QIT), (2) assess adherence to clinical practice guidelines, (3) create a dashboard with measures of organizational performance (e.g., revenue collection), (4) identify high risk patients, (5) create individual provider performance profiles, (6) create dashboards with unit performance (e.g., trend in revenue collection), (7) generate reports to inform strategic planning, (8) identify care gaps for patients, (9) assess adherence to guidelines, (10) create an approach for clinicians to query for data (10). A mean score above 5 denoted adequate data use, and a mean score below 5denoted inadequate data use.
2.5. Data analysis
Data analysis was conducted by using the statistical software SPSS version 25. All filled out questionnaires were carefully reviewed by the data collectors for clarity and completeness. Data were coded, and data cleaning was performed before entry into the SPSS software. For this study, we conducted two main analyses corresponding to our objectives. The first was a descriptive analysis, to describe the proportion of study participants and the proportion of facilities using EMR data in decision-making. The aim of the second analysis was to determine the associations between technical, behavioral, organizational, technical, and demographic factors and EMR data use in decision-making by using bivariable and multivariable logistic regression analyses. All variables were subjected to chi-square test and then predictor variables with outcome at a p-value below 0.2 were considered for the logistic regression analyses. All statistics with a P-value < 0.05 were regarded as significant.
3. Results
3.1. Sociodemographic characteristics of study participants
A total of 308 health managers from different categories and educational levels out of 315 sampled participants with a mean age of 33 years were interviewed. The majority (53.9%) of the respondents were men. Among the study participants, 118 (38.3%) were aged less than 30 years, 141 (45.8%) were aged 30–40 years, and 49 (15.9%) were aged 40 years and above. In addition, 75 (24%) had attended undergraduate degrees. Approximately 39.9% of the study participants had work experience of more than 6 years. In addition, most of the respondents (42.9%) were nurses compared with other professions (Table 1). However, the majority 204 (66.2%) of the facility health managers were from health centers, and only 33 (11%) were from dispensaries (Figure 3).
3.2. EMR system data use practices in decision-making among health managers
EMR use in decision-making among health managers was measured if a participant reported use of the EMR data in decision-making and clarified the purpose of EMR data use. The majority of the participants, 174 (56.5%), reported that they currently used EMR in their facility, and 128 (73.6%) used it on a daily basis. Approximately 142 (46.1%) of the participants had used the EMR system before. Among the respondents, approximately 125 (40.6%) reported the use of EMR system data in decision-making, and among them, approximately 103 (33.4%) had adequate EMR data use practices (Table 2).
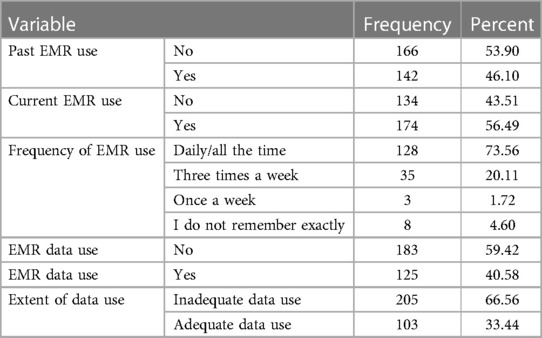
Table 2. EMR system data use practices among health managers in public primary health facilities, Dodoma Region (n = 308).
The EMR data were most commonly used for continuous quality improvement (121,39.29%) and were least commonly used for identifying high-risk patients (78, 25.32%) (Figure 4).
Furthermore, approximately 168 (54%) of the managers did not use EMR in decision-making frequently, and the main reported reason for not using the system frequently in decision-making was the unavailability of the EMR system in some units/departments (150, 89.3%) (Figure 5).
3.2.1. EMR system data use and related organizational characteristics
Overall, 184 (59.7%) of the participants had access to at least one computer, and among them, 19 (10.4%) shared the computer with more than four people. Furthermore, 120 (39%) of the participants had been trained on HMIS, while 152 (49.4%) had been trained on EMR use. However, 174 (56.5%) of the health managers are currently using the EMR system.
Most of the participants, 263 (85.4%), held regular discussions on EMR during facility meetings, and 180 (60.0%) reported that there was facility management support in the use of EMR system data. Approximately 109 (35.4%) of the participants mentioned that there was a person assigned to the facility to facilitate EMR use within the institution as an EMR champion (Table 3).
3.2.2. EMR system data use in decision-making and related behavioral characteristics
Preference of the EMR system compared with the paper-based system, attitude, and knowledge of computer and data use were assessed. Among the participants, 288 (93.51%) reported that they prefer the EMR system over the paper-based system. Knowledge of computer and data use ranged from 67.53% to 93.83% in all 10 areas assessed. Furthermore, 267 (86.69%) of the health managers had a good attitude toward the EMR system (Table 4).
3.2.3. EMR system data use and related technical characteristics
Almost all the participants (289, 93.8%) reported that they were able to use a computer. A total of 134 (43.35%) had positively perceived EMR system quality but only 22 (7.14%) had positively perceived EMR system service quality (Table 5).
3.3. Factors associated with EMR system data use in decision-making
Binary logistic regression analysis was used to determine how the independent variables influenced the dependent variable collectively. The analysis was also meant to establish the extent to which each independent variable affected the dependent variable and which factors were more significant.
The following factors were found to independently influence EMR data use: age of respondent, education level, access to a computer, motivation to use data, functionality of the computer, perceived EMR system information quality, and computer and data use skills as provided in Table 6.
3.3.1. Sociodemographic factors
Health managers with a diploma and a degree or above were three and five times more likely to use the EMR system than certificate holders, respectively [AOR = 3.24 with 95% CI of (1.29, 8.15), p = 0.012 and AOR = 5.26 with 95% CI of (1.84, 7.15), p = 0.002]. In addition, specialists/general practitioners were 2.64-fold more likely to use EMR system data than other cadres [AOR = 2.64 with 95% CI of (1.17, 5.94), p = 0.019]. Those who were working in rural areas were less likely to use EMR data than those working in urban areas [AOR = 0.46 with 95% CI of (0.26, 0.81), p = 0.008]. Those having managerial experience of ≤1 year were less likely to be associated with EMR system data use [AOR = 0.38 with 95% CI of (0.19, 0.74), p = 0.004]. However, other factors, such as age, sex, and type of facility did not have significant findings.
3.3.2. Organizational factors
Those who reported having access to computers and discussions on EMR during meetings were 4.72 and 2.77 more likely to use EMR system data adequately compared with others, respectively [AOR = 4.72 with 95% CI of (1.65, 13.48), p = 0.004 and AOR = 2.77, 95% CI: 1.01, 7.58, p = 0.047]. Those who reported having EMR systems in all working areas were seven times more likely to use EMR system data in decision-making than those who reported having EMR systems in some units [AOR = 7.23 with 95% CI of (3.15, 16.59), p = 0.001]. Other factors did not have significant findings.
3.3.3. Behavioral factors
Respondents with good perceived EMR system information quality were three times more likely to use EMR systems than those with poor perception of system quality [AOR = 2.84 with 95% CI of (1.50, 5.39), p = 0.001]. However, preference for the EMR system and attitude toward EMR did not have significant findings.
3.3.4. Technical factors
Health managers who had excellent knowledge of computer and data use were two times more likely to use EMR system data than those with poor knowledge of computer and data use [AOR = 1.84 with 95% CI of (3.38, 10.13), p = 0.001]. Interestingly, those who reported being able to use computers were less likely to use EMR system data adequately [AOR = 0.12 with 95% CI of (0.019, 0.79), p = 0.028].
4. Discussion
This study set out to assess the EMR system data use in decision-making and its associated factors in public primary health facilities. We assessed variables related to (1) sociodemographic, (2) organizational, (3) technical, and (4) behavioral factors.
The findings of this study revealed that approximately half (56.5%) of health managers were current users of the EMR system, of whom 73.6% used the system on a daily basis. Among them, approximately 125 (40.6%) used EMR data in decision-making. This rate was higher than that in a study conducted in Ethiopia, which revealed that EMR system data were used by 26.6% of the healthcare workers (2). Most of the facilities were partially electronic and partially paper-based. This finding is the same as those revealed in studies conducted in Ethiopia and Malawi (1, 2, 28).
The relatively high use of EMR system data may be attributed to the fact that most of the users preferred EMRs over paper-based systems (93.5%). In addition, respondents also had good computer and data use skills ranging from 50.93% to 93.83%, which might have contributed to this level of implementation. This is supported by a study conducted in Dire Dawa (2) and Eastern Ethiopia (28).
Health professionals with excellent knowledge of computers and data use skills were more likely to use EMR systems than others. This finding is similar to that from a study conducted in Tanzania, which shows that the knowledge gap and skills among healthcare workers significantly influence EMR system use and the data management process (16, 29). In a study conducted in Ethiopia, it was revealed that staff with good data analysis skills were more likely to use health information system data than others (30). Moreover, a study conducted at Muhimbili National Hospital revealed that one of the challenges in the implementation of EMRs was inadequate skills of healthcare workers in the use of computers, which was because most of the old staff were aging and had inadequate basic skills in the use of computers, and the new staff turnover at the facility was high. All this resulted in poor quality of clinical note documentation. Refresher trainings and interfacility forums to share real practical experience were recommended (31).
In this study, the age of the health managers did not significantly impact their likelihood of using EMR data. This was different from a study conducted in Ethiopia that revealed that health professionals in the age group of 26–30 years were 1.61 times more likely to use the EMR system than those whose age was more than 40 years (32). However, another study in Ethiopia revealed that health professionals with a higher age, above 35 years, were more likely to use EMR systems than others (28). The different outcomes might be influenced by contextual factors such as the availability of training programs, the complexity of the EMR system, and the overall technological environment in the healthcare setting.
In this study, it was further revealed that motivation (managerial support) for the use of EMR system data was less likely to be associated with improved use of EMR data. This is different from a study conducted in Bahir Dar City, Ethiopia, which revealed that healthcare workers with managerial support were more willing to use EMR systems than others (33). However, another study (34) revealed that good governance (leadership, participatory monitoring, regular review of data) was associated with improved data use practices. In addition, performance-based financing was also found to have a role in promoting data use. In another study too, conducted at Mount Meru Hospital, EMR use was limited by low knowledge and skills of healthcare workers on information and communication technology (ICT) and inadequate management support (35). Therefore, the differences in findings could be due to variations in the healthcare settings, organizational cultures, levels of managerial support, and overall readiness of healthcare workers to adopt and utilize EMRs.
Those who were working in rural areas were less likely to use EMR data compared with those working in urban areas. This is congruent with a study conducted in the USA (10) in which hospital characteristics influenced use of EHR data, with the small, rural, state/local government run, and non-teaching hospitals having the lowest rates of EHR data use. This might be because EMR adoption rates in rural areas are significantly lower than in urban areas. This could be attributed to factors such as technology access, limited resources, and lower EMR adoption rates in rural settings.
Furthermore, respondents with good perceived EMR information quality were three times more likely to use EMR system data than those with poor perceived information quality. However, in this study, perceived system quality and service quality did not have a significant influence on EMR use. This is different from a study conducted in Ethiopia in which EMR system service quality and system quality were independent predictors of EMR use (2). Another study conducted in Gabon revealed that perceived EMR system quality and information quality were 1.7 times more likely to impact use of the system (36). This may be because data use is influenced by how users perceive the quality of the data produced.
The degree of EMR implementation was also a predictor of EMR use. Those who had EMR systems in all departments/units were more likely to use EMR systems than others. This is congruent with a study conducted in Ayder Hospital in Ethiopia in which 95% of the units were using the EMR system; hence, EMR use was higher (37). Another study conducted in Kenya revealed that EMR use was not adequate due to infrastructure challenges (38). In addition, another study revealed that the availability of health information system resources, such as EMR systems, organizational structure, and training, potentially influences data use (34). This may be because the availability of the EMR system in all departments increases the chance that in hospital discussions, all staff will be discussing data generated from the EMR system rather than a mixture of sources of data.
Training on EMR use had no influence on EMR system data use in this study. This was in line with a study conducted in Dire Dawa, Ethiopia (2). However, this finding is in contrast with findings from other studies conducted in different regions of Ethiopia (28), Malawi (1), and Kenya (38). It is well known that training has great potential to improve EMR use, as it can improve the attitude, knowledge, and skills of health managers. While training is a crucial component, its effectiveness in promoting EMR data use is intertwined with other factors, such as managerial support, cultural considerations, and resource availability. Hence, a comprehensive approach that addresses these factors is essential for maximizing the benefits of EMRs implementation.
A higher education level was also associated with EMR system data use, in which those with a diploma and a degree or above were 3 and 5 times, respectively, more likely to use EMR system data than those with a certificate. This is congruent with a study conducted in Ethiopia in which health professionals with an educational level of first degree and above were 1.92 times more likely to use the EMR system than diploma holders (32). This finding suggests that providing higher education and training opportunities to healthcare professionals could enhance their ability to engage with EMR systems and leverage the data they provide. This could lead to improved decision-making processes and patient care.
Overall, the study identified several factors that influenced the use of EMR system data among health managers. These factors included education level, professional role, managerial experience, access to computers, discussions about EMR, system implementation status, perceived system quality, and computer and data use skills.
5. Conclusions
The electronic medical records system has a proven advantage of enabling easy retrieval of data, including data analysis and use. This can potentially lead to more informed decision-making in the health sector. However, in this study, almost half of the respondents were current users of the EMR system, and 40.6% of the health managers were using EMR data in decision-making. This indicates that although many health managers are using EMR systems, a notable portion of decision makers have not fully integrated EMR data into their decision-making practices. Thus, for improvement in EMR system data use, future investments in issues such as training, installation of the system in all units/departments, education, and integration of EMR data into established decision-making workflows should be advocated. Since it was found that training in itself is insufficient to improve use of EMR, other organizational aspects of work routine that may serve as a challenge must be considered. Many health projects focus on training and technical aspects rather than improving organizational culture. Therefore, a comprehensive approach that addresses these factors is essential for maximizing EMR use in decision-making.
Data availability statement
The raw data supporting the conclusions of this article will be made available by the authors, without undue reservation.
Ethics statement
The studies involving humans were approved by The University of Dodoma Institutional Review Ethics Committee (IRREC). The studies were conducted in accordance with the local legislation and institutional requirements. The participants provided their written informed consent to participate in this study.
Author contributions
EK: Conceptualization, Data curation, Formal analysis, Funding acquisition, Methodology, Writing – original draft. JN: Supervision, Writing – review and editing. SK: Supervision, Writing – review and editing.
Funding
The author(s) declare no external financial support was received for the research, authorship, and/or publication of this article. This work was funded by the main researcher.
Acknowledgments
I would like to acknowledge the University of Dodoma Research Committee through the Department of Nursing and Public Health for their tireless support during the development of this article. In addition, my sincere gratitude to SK and JN for their support during the preparation of this research article and protocol as student coordinators.
Conflict of interest
The authors declare that the research was conducted in the absence of any commercial or financial relationships that could be construed as a potential conflict of interest.
Publisher's note
All claims expressed in this article are solely those of the authors and do not necessarily represent those of their affiliated organizations, or those of the publisher, the editors and the reviewers. Any product that may be evaluated in this article, or claim that may be made by its manufacturer, is not guaranteed or endorsed by the publisher.
References
1. Msiska KEM, Kumitawa A, Kumwenda B. Factors affecting the utilisation of electronic medical records system in Malawian central hospitals. Malawi Med J. (2017) 29(3):247–53. doi: 10.4314/mmj.v29i3.4
2. Tolera A, Oljira L, Dingeta T, Abera A, Roba HS. Electronic medical record use and associated factors among healthcare professionals at public health facilities in Dire Dawa, eastern Ethiopia: a mixed-method study. Front Digit Health. (2022) 4:935945. doi: 10.3389/fdgth.2022.935945
3. Mukred M, Yusof ZM, Asma’ Mokhtar U, Sadiq AS, Hawash B, Ahmed WA. Improving the decision-making process in the higher learning institutions via electronic records management system adoption. KSII Trans Internet Inf Syst. (2021) 15(1):90–113. doi: 10.3837/tiis.2021.01.006
4. Kovalenko P. 8 Ways EMR systems improve the quality of hospital services. Fort Lee: Langate (2023). p. 1–9.
5. Health Systems Strengthening Practice Spotlight. Ethiopia’s Information Revolution: Using Digitalization to Improve the Health System. Washington, DC: USAID (2021).
6. ODI, Boyd M, Tennison J. Health data governance summit. In: Pre-read: Health data as a global public good. Geneva: World Health Organization (2021). p. 19–23. Available at: https://cdn.who.int/media/docs/default-source/world-health-data-platform/events/health-data-governance-summit/preread-2-who-data-governance-summit_health-data-as-a-public-good.pdf?sfvrsn=2d1e3ad8_8
7. Feyzabadi VY, Emami M, Mehrolhassani MH. Health information system in primary health care: the challenges and barriers from local providers’ perspective of an area in Iran. Int J Prev Med. (2015) 2015. doi: 10.4103/2008-7802.160056
8. Aminpour F, Sadoughi F, Ahamdi M. Utilization of open source electronic health record around the world: a systematic review. J Res Med Sci. (2014) 19(1):57–64.24672566
9. Akanbi MO, Ocheke AN, Agaba PA, Daniyam CA, Agaba EI, Okeke EN, et al. Use of electronic health records in sub-Saharan Africa: progress and challenges. J Med Trop. (2014) 14(1):1–6.
10. Parasrampuria S, Henry J. Hospitals’ use of electronic health records data, 2015–2017. Hospitals (Lond). (2015) 46:2015–7.
11. Peltola J. On adoption and use of hospital information systems in developing countries: experiences of health care personnel and hospital management in Tanzania (master’s thesis). Tampere: Tampere University (2019). p. 35. Available online at: https://trepo.tuni.fi/bitstream/handle/10024/118773/PeltolaJohanna.pdf?sequence=2&isAllowed=y
12. Kikoba BR, Kalinga E, Lungo J. Integrating electronic medical records data into national health reporting system to enhance health data reporting and use at the facility level. In: Nielsen P, Kimaro HC, editors. Information and communication technologies for development. Strengthening southern-driven cooperation as a catalyst for ICT4D. ICT4D 2019. IFIP advances in information and communication technology. Vol. 551. Cham: Springer (2019). doi: 10.1007/978-3-030-18400-1_44
13. Ministry of Health, Community Development, Gender, Elderly and Children TDS. Digital health strategy: July 2019–June 2024. (2019) 1:98.
14. Ministry of Health, Community Development, Gender, Elderly and Children. Tanzania Health sector strategic plan 2021–2026. (2021) 2026:104.
15. German CC, Kinyenje ES, Yahya TA, Hokororo JC, Nungu S, Mohamed MA, et al. The use of data for planning and services improvement in Tanzanian primary healthcare facilities: experience from star rating assessment. J Serv Sci Manag. (2023) 16(2):144–60. doi: 10.4236/jssm.2023.162010
16. Mboera LEG, Rumisha SF, Mbata D, Mremi IR, Lyimo EP, Joachim C. Data utilisation and factors influencing the performance of the health management information system in Tanzania. BMC Health Serv Res. (2021) 21(1):4–11. doi: 10.1186/s12913-020-05979-9
17. Saleem JJ, Russ AL, Justice CF, Hagg H, Ebright PR, Woodbridge PA, et al. Exploring the persistence of paper with the electronic health record. Int J Med Inform. (2009) 78(9):618–28. doi: 10.1016/j.ijmedinf.2009.04.001
19. Ministry of Health, Community Development, Gender, Elderly and Children. Health data collaborative (HDC) implementation report. Popul Policy Compend. (2020) 1:1–6.
20. PO-RALG. Orodha ya vituo vyenye mfumo mpaka wa GOTHOMIS kufikia mei 31, 2022. President's Office, Regional Administration and Local Government Tanzania [Swahili]. (2022).
21. PO-RALG. Data use and quality improvement in Tanzania. President's Office, Regional Administration and Local Government Tanzania (2021).
24. Dodoma Region. Names of public primary health facilities with EMR system, Dodoma. (2022) 2(8.5.2017):2003–5.
25. Mathew OO, Sola AF, Oladiran BH, Amos AA. Efficiency of Neyman allocation procedure over other allocation procedures in stratified random sampling. Am J Theor Appl Stat. (2013) 2(5):122. doi: 10.11648/j.ajtas.20130205.12
26. PO-RALG M. Council health management system guideline. President's Office, Regional Administration and Local Government Tanzania. (2019). p. 36.
27. Aqil A, Lippeveld T, Traore M, Barry A. PRISM Tools: user guide. Meas Eval. (2012) 1:1–174. Available at: https://www.measureevaluation.org/resources/publications/ms-12-51
28. Oumer A, Muhye A, Dagne I, Ishak N, Ale A, Bekele A. Utilization, determinants, and prospects of electronic medical records in Ethiopia. Biomed Res Int. (2021) 2021:2230618. doi: 10.1155/2021/2230618
29. Yilma TM, Tilahun B, Mamuye A, Kerie H, Nurhussien F, Zemen E, et al. Organizational and health professional readiness for the implementation of electronic medical record system: an implication for the current EMR implementation in northwest Ethiopia. BMJ Health Care Inform. (2023) 30(1):1–7. doi: 10.1136/bmjhci-2022-100723
30. Dagnew E, Woreta SA, Shiferaw AM. Routine health information utilization and associated factors among health care professionals working at public health institution in North Gondar, Northwest Ethiopia. BMC Health Serv Res. (2018) 18(1):1–8. doi: 10.1186/s12913-018-3498-7
31. Mashoka RJ, Murray B, George U, Lobue N, Mfinanga J, Sawe H, et al. Implementation of electronic medical records at an emergency medicine department in Tanzania: the information technology perspective. Afr J Emerg Med. (2019) 9(4):165–71. doi: 10.1016/j.afjem.2019.07.002
32. Mekonnen G, Gobena T, Bekele Z, Mariam ZT, Taddese A, Hawulte B. Utilization and determinants of electronic medical record system among health professionals in public health facilities of Harari Regional State. East Ethiopia. (2021) 12:10.
33. Berihun B, Atnafu DD, Sitotaw G. Willingness to use electronic medical record (EMR) system in healthcare facilities of Bahir Dar City, Northwest Ethiopia. Biomed Res Int. (2020) 2020:3827328. doi: 10.1155/2020/3827328
34. Rendell N, Lokuge K, Rosewell A, Field E. Factors that influence data use to improve health service delivery in low- and middle-income countries. Glob Health Sci Pract. (2020) 8(3):566–81. doi: 10.9745/GHSP-D-19-00388
35. Edward L, Sukums F. Adoption and users experience of government of Tanzania-hospital management information system in Meru district hospital, Arusha, Tanzania: a qualitative study. SSRN [preprint]. (2022) 1:16–8. https://ssrn.com/abstract=4147066
36. Bagayoko CO, Tchuente J, Traoré D, Moukoumbi Lipenguet G, Ondzigue Mbenga R, Koumamba AP, et al. Implementation of a national electronic health information system in Gabon: a survey of healthcare providers’ perceptions. BMC Med Inform Decis Mak. (2020) 20(1):1–9. doi: 10.1186/s12911-020-01213-y
37. Bisrat A, Minda D, Assamnew B, Abebe B, Abegaz T. Implementation challenges and perception of care providers on electronic medical records at St. Paul’s and Ayder hospitals, Ethiopia. BMC Med Inform Decis Mak. (2021) 21(1):1–12. doi: 10.1186/s12911-021-01670-z
Keywords: electronic medical records, data use, decision-making, health managers, public primary health facilities, cross-sectional analytical study
Citation: Kessy EC, Kibusi SM and Ntwenya JE (2024) Electronic medical record systems data use in decision-making and associated factors among health managers at public primary health facilities, Dodoma region: a cross-sectional analytical study. Front. Digit. Health 5:1259268. doi: 10.3389/fdgth.2023.1259268
Received: 15 July 2023; Accepted: 20 November 2023;
Published: 12 February 2024.
Edited by:
Vijayakumar Varadarajan, University of New South Wales, AustraliaReviewed by:
Manisha Mantri, Center for Development of Advanced Computing (C-DAC), IndiaAervina Misron, UNITAR International University, Malaysia
© 2024 Kessy, Kibusi and Ntwenya. This is an open-access article distributed under the terms of the Creative Commons Attribution License (CC BY). The use, distribution or reproduction in other forums is permitted, provided the original author(s) and the copyright owner(s) are credited and that the original publication in this journal is cited, in accordance with accepted academic practice. No use, distribution or reproduction is permitted which does not comply with these terms.
*Correspondence: Eusebi Cornelius Kessy kessydr1982@gmail.com
†Present Address: Eusebi Cornelius Kessy, Department of Public Health, University of Dodoma, Dodoma, Tanzania