- 1Institute of Biomedical Ethics and History of Medicine, University of Zurich (UZH), Zürich, Switzerland
- 2Clinical Ethics Unit, University Hospital of Basel (USB), Basel, Switzerland
- 3Clinical Ethics Unit, University Psychiatric Clinics Basel (UPK), Basel, Switzerland
- 4Department of Internal Medicine, University Hospital Zurich (USZ), Zürich, Switzerland
- 5Medical Faculty, University of Geneva, Geneva, Switzerland
- 6Medical Faculty, University of Basel, Basel, Switzerland
Introduction: Patient decision aids (PDAs) are important tools to empower patients and integrate their preferences and values in the decision-making process. Even though patients with mental health problems have a strong interest in being more involved in decision making about their treatment, research has mainly focused on PDAs for somatic conditions. In this scoping review, we focus on patients suffering from depression and the role of PDAs for this patient group. The review offers an overview of digital and analog PDAs, their advantages and disadvantages as well as recommendations for further research and development.
Methods: A systematic search of the existing literature guided by the Cochrane Handbook for Systematic Reviews and the Preferred Reporting Items for Systematic Reviews and Meta-Analyses - extension for scoping reviews (PRISMA-ScR) was conducted. Three electronic literature databases with the appropriate thematic focus were searched (PubMed, PsycInfo, and Web of Science). The search strategy used controlled and natural language to search for the key concepts decision aids and depression. The articles were selected in a two-step process guided by predefined inclusion and exclusion criteria. We narratively synthetized information extracted from 40 research articles.
Results: We included 40 articles in our review. Our review revealed that there is more focus on digital PDAs in research than in clinical practice. Digitalization can enhance the benefits of PDAs by developing tools that are more efficient, interactive, and personalized. The main disadvantages of both types of PDAs for the treatment of depression are related to time, dissemination, and capacity building for the health care providers. Digital PDAs need to be regularly updated, effective strategies for their dissemination and acceptance need to be identified, and clinicians need sufficient training on how to use digital PDAs. There is more research needed to study which forms of PDAs are most appropriate for various patient groups (e.g., older adults, or patients with comorbidities), and to identify the most effective ways of PDAs' integration in the clinical workflow. The findings from our review could be well aligned with the International Patient Decision Aids Standards.
Discussion: More research is needed regarding effective strategies for the implementation of digital PDAs into the clinical workflow, ethical issues raised by the digital format, and opportunities of tailoring PDAs for diverse patient groups.
1. Introduction
According to the World Health Organization (WHO), about 280 million people worldwide suffer from a depressive disorder rendering it a leading cause of disability in the world (1). Depression as a public health issue becomes even more challenging when we consider therapeutic options, such as different types of psychotherapy and psychiatric medication (2, 3). Even though there are effective, evidence-based treatments, only about one third of patients receive adequate therapy (4, 5). Some of the reasons for this problem are poor clinician-patient communication, insufficient knowledge of treatment options as well as insufficient inclusion of patients' views and preferences into the decision-making process (6–8). Therefore, the better inclusion of patient values and preferences into treatment decisions may play an important role in addressing the public health issue of depression (9, 10) by possibly enhancing the quality of treatment outcomes, through enhanced patient satisfaction and therapeutic adherence (11). Furthermore, the importance of involving patients and their values and preferences is consistent with best practices and clinical guidelines for mental health services (9, 12–14).
Patient decision aids (PDAs) are important tools for facilitating and achieving this aim. PDAs are evidence-based tools that inform patients about treatment options and go beyond mere informational materials by helping patients elicit their preferences, and thus preparing them for a consultation with a healthcare professional to engage in a shared decision making (SDM) process (15–17). PDAs aim at empowering patients by presenting the available evidence in an understandable manner, thereby encouraging the patients to be more involved in the decision making, reducing their decisional conflict, and aligning treatment decisions with patients' preferences and values (15, 16, 18, 19). These are important factors both for patient's empowerment and SDM that is based not only on information exchange but also on creation of a trusting relationship between a mental health care provider and a patient (17).
PDAs can be designed as stand-alone tools, as facilitators during the SDM process, or as a combination of both. There are several types of PDAs: they can be either developed in analog forms, e.g., as fact sheets, or in a digital format, such as websites or applications. Best practice standards for developing PDAs have been defined in the International Patient Decision Aid Standards (20, 21).
As in many other areas, novel IT technologies hold many promises also in the health care sector. This does not only apply to diagnostic tools or treatment options but also to decision making. Digitalization offers the opportunity to design PDAs in an interactive, personalized, and possibly more effective way to better engage patients and facilitate SDM for decisions regarding current and future care (22–24). Hence, digital PDAs have the potential to empower patients and orient healthcare towards patient- and value-oriented practice (10, 22, 24).
Previous research on PDAs has mainly focused on somatic conditions (18, 25, 26). A Cochrane review (18) showed the effectiveness of PDAs in terms of increased patient knowledge, decreased decisional conflict, and clarity about personal values. However, the majority of the 105 included studies in the review focused on health decisions related to somatic conditions (18). A similar pattern can be seen in a recent review focusing on users' involvement in design and development of PDAs; also here, only about 2% of the included PDA projects belong to mental health (27). These reviews are used to formulate guidelines and checklists for development of PDAs (28, 29), but the patient groups with major depressive disorder and generally with mental disorders are underrepresented. The inclusion of patients and their preferences and values in the decision making process is at least as important in mental healthcare. Nevertheless, PDAs have only recently been researched in mental healthcare, even though patients with mental disorders in general and depression in particular are interested in taking a more active role in decision making (30, 31). The (qualitative) research specifically focusing on patients' experiences with PDAs and their involvement in the development of PDAs for the treatment of depression deserves more attention (30, 32). Furthermore, recent reviews have focused on quantitative results of randomized-controlled studies, thus neglecting a large part of the available evidence (33, 34). Current research on SDM in mental health care can provide valuable insights for future studies on PDAs as it offers a crucial context for understanding their utilization (11, 17, 35). Additionally, such research highlights important factors that contribute to the empowerment and active involvement of patients with mental health conditions in their treatment (17, 35).
To follow the current development driven by digital revolution and its potential, the aim of this scoping review was to characterize and compare digital and analog PDAs for patients with major depressive disorders by portraying both qualitative and quantitative evidence of their main advantages and disadvantages. Thereby, we define digital PDAs as tools that can be used with computers, mobile devices, or other digital devices. Analog PDAs are tools that are not in digital electronic formats. Instead, they typically use paper-based materials, such as flyers or booklets. This evidence synthesis provides a comprehensive understanding of the role of PDAs for patients' decision making as well as recommendations for further research and development.
2. Methods
As we intended to portray the existing literature on key characteristics of PDAs in depression (rather than provide a definitive, quantitative answer to a narrow question such as the effect of PDAs on decisional conflict in patients with depression), scoping review methodology was most appropriate for our study (36). As standard registries such as PROSPERO do not currently accept Scoping Reviews we did not pre-register the review (37). A systematic search of the existing literature guided by the Cochrane Handbook for Systematic Reviews (38) and the Preferred Reporting Items for Systematic Reviews and Meta-Analyses—extension for scoping reviews (PRISMA-ScR) was conducted (see the PRISMA Flow Diagram in Figure 1) (39). Three electronic literature databases with the appropriate thematic focus were searched (PubMed, PsycInfo, and Web of Science). The search strategy used controlled and natural language to search for the key concepts decision aids and depression (see Supplementary Table S1). The database search closed on December 31st, 2022. To compensate for eventual shortcomings of the database search, it was complemented with a search on Google Scholar, and, for all included articles, a search for citing articles on Web of Science and a hand search of the reference lists.
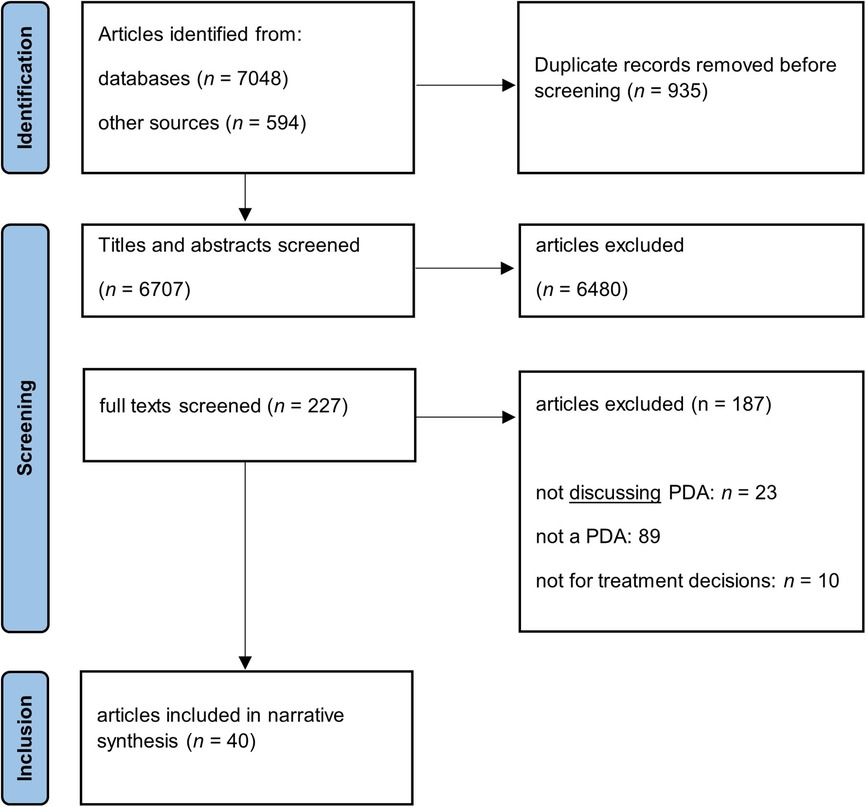
Figure 1. PRISMA flowchart of the literature search. MDE, major depressive episode, PDA, patient decision aid.
The articles were selected in a two-step process guided by predefined inclusion and exclusion criteria (see Table 1). To broaden the scope of our review, we did not restrict our search by publication date and included a variety of article types such as study protocols and reviews. Screening was done independently by ALW and JS. In both screening steps, ALW screened all records, while JS screened 20% including all references marked as “unsure” by ALW and a random selection of references. Disagreements were resolved by discussion among ALW, JS, and, if necessary, MT.
Data extraction was aligned with the aims of the study: summarizing advantages and disadvantages of using PDAs in general and digital vs. analog PDAs. During the extraction process, it became clear that it was not possible to differentiate between benefits and advantages or risks, challenges, and disadvantages, because the included articles did not differentiate clearly between this terminology. Besides, the terminology used in the selected articles was not unified. That is why we decided to use general terms of advantages and disadvantages to refer to the potential positive and negative aspects of the use of digital and analog PDAs. The method of narrative synthesis (40) was chosen to gather a broad scope of knowledge to create an overview of PDAs for depression and guide further research. This method is well suitable since there are not many studies about PDAs for depression and given the variety of the chosen articles. To compare the digital and analog PDAs and provide an overview on the digital PDAs, the information extraction was clustered in accordance with the topics of advantages, disadvantages, and recommendations for both digital and analog PDAs. Data extraction was done by JS and checked by ALW.
This narrative synthesis was supplemented by tabulating the quantitative results. For ease of comparison across publications, effect sizes are presented wherever possible. For included studies reporting neither effect sizes nor sufficient information to calculate them although this should be possible given the study design, the corresponding authors were contacted with a request for additional information. Cohen's d was chosen because it is appropriately widely used for continuous outcomes (such as decisional conflicts) independent of the scale on which the outcome was measured (41). Cohen's d is computed as the difference of the two means divided by the pooled SD with values of 0.20, 0.50, and 0.80 signifying small, medium, and large effects, respectively (42). For publications not reporting Cohen's d, it was computed as follows: when raw data or descriptive statistics such as M and SD were reported, d was derived from these statistics. From CIs, the SD was computed as proposed by Higgins (43). From F statistics with one degree of freedom from t statistics, and from odds ratios, d was computed according to Borenstein (2019) (41), and from z and χ2 statistics according to Rosenthal and DiMatteo (44). For pre-post-control between-subject designs, d was computed according to Morris (45).
3. Results
After two-step screening and applying our inclusion and exclusion criteria, 40 articles remained (see in Figure 1) that were used to synthetize evidence and information on disadvantages and advantages of the use of analog and digital PDAs as well as recommendations for their development. Table 2 shows the main characteristics of the 40 included publications for this review (15, 25, 26, 30–33, 46–78). More than one third of the articles focused on digital PDAs for patients with depression without any focus on a particular patient group (e.g., older adults or young adults). Very often, the articles included not only the patients' but also the clinicians' perspective on PDAs. Most PDAs were developed in interdisciplinary teams and in line with the International Patient Decision Aid Standards (61, 69, 70, 72).
3.1. Advantages
3.1.1. Advantages of both digital and analog PDAs
The mentioned advantages of PDAs and positive roles that PDAs can have for patients seeking treatment of depression were increased knowledge (25, 32, 46, 48, 50, 51, 54, 58, 62, 69, 70, 73, 75, 77), reduced decisional conflict (32, 55, 58, 62, 70, 72, 73, 77, 78), supporting decision-making (56, 62, 72, 76), elicitation of and treatment alignment with patients’ preferences and values (26, 32, 46, 51, 54, 72, 76, 77), better preparation for and involvement in SDM (15, 26, 46, 48–51, 55, 63, 64, 69, 71, 72, 78), patients' satisfaction (46, 49, 51, 54, 62–64, 72), and more realistic expectations (51, 72). Furthermore, some studies pointed out that the use of PDAs in SDM did not increase the consultation time (25, 46, 49, 62, 63). Finally, an inclusion of personal stories was considered to be beneficial for patients' elicitation of their preferences because they could relate to people with similar experiences (48, 59, 68).
Supplementary Table S2 displays an overview of quantitative results from the included studies. These provide preliminary evidence for good acceptability, a reduction of decisional conflict, increase of patient involvement, adherence, and satisfaction by PDAs, without increase in consultation time. Data on other outcomes such as patient knowledge and clinical outcomes are inconsistent and/or scarce.
3.1.2. Advantages specific to digital PDAs
A variety of advantages or positive aspects specific to digital PDAs for the treatment of depression were discussed: digital PDAs are effective, easy and quick to use and access (50, 51, 54, 67). They can give patients enough flexibility and time to use them when it is most suitable and comfortable for them without being rushed (54, 55, 75, 77). This should enable patients to be better prepared for the consultation with clinicians, formulate questions, or use the waiting time efficiently if the tools are used in the waiting room (54, 55). Furthermore, audio and visual components can be implemented in digital PDAs that can be particularly important for low literacy users (56). Digital PDAs can also offer more privacy, for example, when the tool is secured with a password (51, 75). They can be important for particular groups such as young patients as they are comfortable with this technology (67, 71, 72). Furthermore, digitalization allows for personalization and tailoring of PDAs (15, 30, 50, 69). Digitalization also allows greater scalability and adaptability of the PDAs in terms of both content (e.g., updates in the light of new evidence or personalized content) and form (e.g., information online or in printable format) (50, 57, 75, 76). The scalability and adaptability can lead to further implementation of PDAs in other countries (57). More specific advantages, which are mentioned, were the possibility to include exercises that will prepare patients for decision making and help them understand how their decision making is influenced by relatives or friends (57, 75).
Finally, from the clinicians’ perspective, a great advantage of digital PDAs used in SDM settings is the possibility to link them with electronic health systems, e.g., electronic health records or screening assessment tools (56). This will create better efficiency and allow real-time decisions (50, 54). Integration of digital PDAs can also lead to support evidence-based and patient-centered care (54, 72).
3.1.3. Advantages specific to analog PDAs
The only mentioned advantages specific to analog PDAs for the treatment of depression were that printed materials are important for patients without access to computers or the internet (46, 51), and that they are freely available from public and non-profit organizations (71). An overview of all advantages is in Table 3.
3.2. Disadvantages
3.2.1. Disadvantages related to both digital and analog PDAs
The challenges, which were discussed either in the general context of PDAs or for both digital and analog PDAs for the treatment of depression, were mainly related to the information provided in the PDAs. Both clinicians and patients raised concerns about PDAs that appeared to be too technical and too overwhelming, for example, in terms of content or wording that they provide (32, 48, 50, 51, 68). However, some patients and clinicians also reported that the material was insufficient (49, 50) or the presentation of treatment was conflicting information provided by a clinician (58). Patients' literacy needed for using PDAs that might present a challenging issue (50, 68). Finally, the use of PDAs might pose an additional burden for patients (58, 59) and increase their anxiety (58).
3.2.2. Disadvantages specific to digital PDAs
The common challenges discussed with digital PDAs for the treatment of depression were mainly connected with PDAs integration and implementation in the clinicians' workflow (50, 51). PDAs can play an important role to support SDM and hence, clinicians need sufficient training on how to properly integrate PDAs into their consultation (50). Another problematic issue might be the dissemination of PDAs (51). Finally, digital PDAs might not be suitable and easy to use for all groups of patients (54).
3.2.3. Specific to analog PDAs
In analog PDAs for the treatment of depression, it might be particularly challenging to regularly update evidence about the treatment (26). This challenge is intensified considering scarce resources—such as finances—for creating analog materials (26). Table 4 displays an overview of all disadvantages.
3.3. Recommendations
3.3.1. Recommendations for both digital and analog PDAs
In the papers included in this analysis, several recommendations have been identified, which relate to both analog and digital PDAs for the treatment of depression (Table 5 display an overview of recommendations and their relevance) and correspond with the International Patient Decision Aids Standards: information in PDAs should be written in clear, understandable, concise, and simple language (46, 50, 51, 54, 57). Furthermore, careful wording about potential risks should be used (54, 70) as well as balancing them with positive effects (46). It was recommended that clear instructions or even education should be provided on how to use PDAs (48), particularly digital PDAs (54). Specific recommendations about the visual side of PDAs included recommendation for bright and attractive colors (46, 50), and the use of more visuals for risks and expected benefits of treatment options (46, 70). The inclusion of important stakeholders for designing and developing PDAs was also recommended (55, 58).
In terms of the content of PDAs for the treatment of depression, it was suggested to include both pharmacological as well as psychotherapeutic treatment options (69), and to include a broad range of questions regarding possible treatments (51), update the content regularly (e.g., every 2 years) or, alternatively, determine the “expiration date” of PDAs (50). One study recommended to consider a bias possibly inflicted by the order for which treatment options are presented (69). Furthermore, the inclusion of patients' values and preferences as well as personalized information based on their current social situation, religious and cultural beliefs, and prior knowledge was highlighted several times (26, 31, 74). This can be expected to encourage patients to raise issues, which are important for them and which they would not raise otherwise in the consultation with a clinician (26).
Tailoring PDAs to the needs of the target group was another recurring topic (31, 33, 51, 60). In the context of older adults, it was recommended to tailor PDAs for the treatment of depression in a way that the influence of cognitive impairment is minimized (31). Several studies highlighted the importance of designing PDAs for the treatment of depression for different subgroups of patients (51), such as specific age groups, ethnicities, educational level, and patients with medical comorbidities and other disabilities (31, 62, 70).
In terms of research, it was also recommended to include a variety of population groups as well as considerations of factors related to age and involvement of caregivers (72). Future research should replicate existing studies and focus in more detail on the extent to which PDAs are effective for the treatment of depression and adherence to treatment, and whether PDAs have uniquely positive effects on SDM (33, 48, 69, 72). Furthermore, more research is needed to determine the precise role of PDAs in SDM, to identify the optimal amount of information provided in PDAs (69), and PDAs' accessibility for different health literacy levels (49, 50). Finally, it was recommended to conduct more research on implementation of PDAs (77), more specifically on facilitators and barriers for implementing PDAs (33, 46, 67, 68) as well as on cost-effectiveness of implementing and developing PDAs (58, 62).
In terms of use of PDAs for the treatment of depression, it was recommended to make PDAs interactive, use them on a regular basis (26), make them available at an early stage of decision making (69), include healthcare professionals such as clinicians or nurses to use PDAs with patients (31, 74), and to also include caregivers and relatives (26, 48). Particularly in the context of the last point, it was recommended to provide patients with PDAs before a consultation so that family and other important people for patients can be included in the decision process (49).
3.3.2. Recommendations specific to digital PDAs
Recommendations specific to digital PDAs for the treatment of depression mainly concerned their implementation in the clinical workflow (50, 51, 54, 68): PDAs could be delivered and accessed by patients directly at the clinic by using electronic tablets (50, 68). If linked with screening assessment, the waiting time could be used effectively, and this would allow real-time decision support (54). From a clinicians' perspective, it was recommended to implement PDAs into electronic medical record systems and make them accessible within a shared network (e.g., electronic charts) (50, 54). More research on how exactly such implementation can be reached is needed (54, 68). Finally, it was recommended to further study what forms of digital PDAs (e.g., mobile applications or websites) are most powerful for both patients and healthcare providers (51).
4. Discussion
This scoping review aimed at comparing digital and analog PDAs for patients with major depressive disorder by collecting evidence and information regarding advantages and disadvantages of their use as well as recommendations for their development. The main finding was that analog and digital PDAs increased the patients' satisfaction with the tool, enhanced knowledge, reduced decisional conflict and better preparation for SDM. There was only one advantage specific to the analog PDAs, namely that these PDAs are more suitable for people without access to technology such as computers or smartphones. Digital PDAs were deemed to be more efficient, flexible, more easily accessible and with the opportunity of personalization. The main disadvantages of both types of PDAs for the treatment of depression are related to time, dissemination, and capacity building for the health care providers. Digital PDAs need to be regularly updated, effective strategies for their dissemination and acceptance need to be identified, and clinicians need sufficient training on how to use digital PDAs. Furthermore, there is more research needed to study which forms of PDAs are most appropriate for various patient groups (e.g., older adults, or patients with comorbidities), and to identify the most effective ways of PDAs' integration in the clinical workflow.
The findings from our review could be well aligned with the International Patient Decision Aids Standards (20, 21) that was often used as guidance in the development of PDAs. In both, there is a strong emphasis on presenting treatment options based on evidence that is regularly updated, presenting options in understandable language, including patients' values, and developing them in a way that can guide patients in SDM. The specific topic that was not discussed in our findings is the presentation of probabilities of outcomes. Our findings offer additional insights regarding tailoring PDAs to different patients' subgroups, need for specifying dissemination and implementation process of PDAs and inclusion of the role of families and significant persons in the decision making.
The review of the literature documents the growing interest in digital PDAs for patients with depression. These patients deem it important to be more involved in the decision-making process and have more information about the available treatment options (25, 26, 32). Digitalization offers greater scalability, flexibility, and personalization of PDAs, which would allow for a possibly more effective and tailored inclusion of patients' values and preferences into the decisional framework for therapeutic choices. The easy access, flexibility and personalization of digital PDAs might be especially beneficial considering patients' possible cognitive deficits such as lower motivation or poor concentration. Furthermore, an advantage of digital PDAs for the treatment of depression is its effective inclusion in the clinical workflow, which should facilitate evidence-based and patient-centered healthcare.
4.1. Research gaps and recommendations for further research
In general, more research is needed to systematically study the clinical effectiveness and possibly adverse effects of digital PDAs for the treatment of depression. The present review shows a high level of heterogeneity of approaches and measures of digital PDAs. Some studies were designed with a narrow focus on a specific patient group; other studies had a broad focus on PDAs from both clinicians' and patients' perspective. In addition, many studies used unvalidated instruments to measure the impact of the intervention, such as treatment adherence, patient knowledge, and goal concordance of care. This is in part explained by a lack of validated measurement instruments, e.g., for concordance of care with patient preferences and values (32). Another challenge for interpreting the existing evidence is the different study designs that ranged from purely descriptive studies and pilot testing in focus groups with baseline measures to randomized control trials (RCTs). This is in line with other recent recommendations for further research on decision aids, which call for more RCTs in this field using checklists to ensure that all relevant factors are measured (79).
In the present scoping review, only 40 articles qualified for inclusion, although inclusion criteria were deliberately broad. This is a small number given the impact of depression on public health as well as on patients, who often wish to be included to a greater extent in the decision-making process about their treatment. This research gap is accentuated by the fact that half of the articles had to be excluded because the main (or the only) focus was on the use of decision aids by clinicians (see Figure 1). Even though clinicians' experience can offer valuable insight particularly regarding the role of PDAs in SDM (60), a stronger focus of future research should be on patients' experience. Interestingly, among the articles after the first screening step, PDAs powered by artificial intelligence (AI) were mainly developed for diagnostic or screening purposes (80, 81) even though the potential of AI could be used to contribute to personalized and tailored PDAs as well.
The development, challenges and research of PDAs for patients with depression can gain from taking inspiration from research on PDAs for somatic conditions such as osteoarthritis and cancer and for other mental disorders such as schizophrenia (18). Research in this area has shown that patients' experiences, understanding as well as quality of SDM was improved when digital PDAs contained visual aids such as icons and bar charts (82, 83). Another helpful feature in this context might be that information is presented in different formats such as writing, video and audio (84, 85). Assessing PDAs for readability and cultural sensitivity of different patient groups can be a strategy for ensuring proper personalization of digital PDAs (85). In terms of interaction with digital PDAs, more research is needed to establish an appropriate framework for personalized design that would also take into consideration emotional aspects of decision-making (86). Another suggestion related to improved interaction with digital PDAs was developing them with a flexible, dynamic design that would enable them to choose questions and topics depending on the patient's individual needs and preferences as opposed to having algorithmic predefined structure and questions (87). This can be particularly helpful for patients with depression that might suffer from cognitive deficits. However, more research is needed to identify the necessary features in this context.
The implementation challenges might be approached by the following strategies. Clinicians need training on how to effectively use PDAs with patients in the SDM process (84, 88). In addition, PDAs can have incorporated communication aids (84) and instruction sheets for their implementation in the clinical workflow (88). Finally, an efficient strategy might be to align PDAs and their development directly with clinical practice guidelines so that the PDAs reflect guideline content and guidelines contain passages on SDM and PDAs (82, 87, 89). This effort might be strengthened by collaborating with initiatives and stakeholders focusing on clinical practice guidelines, SDM and implementation of best available evidence (82, 87). Multistakeholder teams can also help with regular evidence update of information provided in PDAs (90). The quality of evidence in PDAs was identified as an important issue in several included studies in this review (26, 50, 75, 77). Furthermore, there was a strong recommendation to strengthen the evidence in a recent evaluation of Ottawa Decision Support Framework for PDAs (80). Research on formulating guidance for evidence selection and summarization might be particularly helpful in this context (90).
In future studies, it is of importance to further improve the inclusion of preferences, values, and experiences of patients with depression in PDAs and SDM. More scientific studies, particularly qualitative studies, are needed to learn about patients' views, experience, and factors influencing acceptance and implementation of PDAs (91). Furthermore, the topics of personalization and appropriateness of different forms of PDAs for different groups deserve more attention as well as the potential for serious adverse events, such as suicide. Both could be achieved by following user-centered designs by developing PDAs to ensure that important stakeholders (55) and preferences and values of different patient groups are included (27). Gibson et al. (92) suggested giving patients the option to decide how much they want to be involved in the SDM process as this preference might change from patient to patient. It would be also interesting to study the precise role of PDAs in the SDM process; particularly, if there is a change in acceptance and effectiveness depending on the phase in the SDM process in which PDAs are used. Finally, even though privacy was listed as a positive aspect of digital PDAs in our results, more research from an ethical perspective is needed as digitalization in health care raises many challenges such as privacy issues, equality of access, or security. The fact that such ethical issues were not discussed is an important research gap.
4.2. Strengths and limitations
Strengths of this review are the systematic literature search with broad inclusion criteria, capturing the full scope of scholarly articles on advantages and disadvantages of PDAs for the treatment of depression. However, this review did not include research on self-help groups, online fora, or patient versions of clinical guidelines, that are probably often used as PDA although not meeting our PDA definition. Also, while we did not exclude articles based on the age of the target group of the respective PDA, due to differences in diagnostic categories between child and adolescent and adult mental health care, our search strategy was likely less sensitive towards articles on child and adolescent depression. The final limitation is that even though we included articles in German, we did not specifically search for articles in German databases.
5. Conclusion
The present scoping review suggests that in the field of PDAs for the treatment of patients with depression, more systematic and comprehensive research is needed to study the role of PDAs in the SDM process and to address the potential benefits as well as challenges that digitalized PDAs can offer. More research is also needed regarding effective strategies for the implementation of digital PDAs into the clinical workflow, ethical and equity issues raised by the digital format, and opportunities of tailoring PDAs for diverse patient groups.
Data availability statement
The original contributions presented in the study are included in the article/Supplementary Material, further inquiries can be directed to the corresponding author.
Author contributions
JS, AW, and MT conceived the study including its methods. AW developed the search strategy, and screened all retrieved records, while JS screened 20% of the records and MT acted as arbiter in the case of disagreement between AW and JS. JS extracted the qualitative data and undertook the narrative synthesis. AW extracted the quantitative data, computed effect sizes and tabulated them. JS drafted the article apart from the methods section, which was drafted by AW. All authors contributed to the article and approved the submitted version.
Acknowledgments
The authors would like to thank Jacqueline Huber (liaison librarian at University of Zurich) for expert support in developing the search strategy.
Conflict of interest
The authors declare that the research was conducted in the absence of any commercial or financial relationships that could be construed as a potential conflict of interest.
Publisher's note
All claims expressed in this article are solely those of the authors and do not necessarily represent those of their affiliated organizations, or those of the publisher, the editors and the reviewers. Any product that may be evaluated in this article, or claim that may be made by its manufacturer, is not guaranteed or endorsed by the publisher.
Supplementary material
The Supplementary Material for this article can be found online at: https://www.frontiersin.org/articles/10.3389/fdgth.2023.1208889/full#supplementary-material
References
1. World Health Organization. Depressive disorder (depression). World Health Organization (2023). Available at: https://www.who.int/news-room/fact-sheets/detail/depression (Accessed July 15, 2023).
2. 2019 Clinical practice guideline for the treatment of depression across three age cohorts. Available at: https://www.apa.org/depression-guideline (Accessed July 15, 2023).
3. Bayes AJ, Parker GB. Comparison of guidelines for the treatment of unipolar depression: a focus on pharmacotherapy and neurostimulation. Acta Psychiatr Scand Juni. (2018) 137(6):459–71. doi: 10.1111/acps.12878
4. Wang PS, Aguilar-Gaxiola S, Alonso J, Angermeyer MC, Borges G, Bromet EJ, et al. Use of mental health services for anxiety, mood, and substance disorders in 17 countries in the WHO world mental health surveys. Lancet Lond Engl. (2007) 370(9590):841–50. doi: 10.1016/S0140-6736(07)61414-7
5. Radez J, Reardon T, Creswell C, Lawrence PJ, Evdoka-Burton G, Waite P. Why do children and adolescents (not) seek and access professional help for their mental health problems? A systematic review of quantitative and qualitative studies. Eur Child Adolesc Psychiatry. (2021) 30(2):183–211. doi: 10.1007/s00787-019-01469-4
6. Puyat JH, Kazanjian A, Goldner EM, Wong H. How often do individuals with major depression receive minimally adequate treatment? A population-based, data linkage study. Can J Psychiatry Rev Can Psychiatr. (2016) 61(7):394–404. doi: 10.1177/0706743716640288
7. Wei Y, McGrath PJ, Hayden J, Kutcher S. Mental health literacy measures evaluating knowledge, attitudes and help-seeking: a scoping review. BMC Psychiatry. (2015) 17(15):291. doi: 10.1186/s12888-015-0681-9
8. Swift JK, Callahan JL, Cooper M, Parkin SR. The impact of accommodating client preference in psychotherapy: a meta-analysis. J Clin Psychol. (2018) 74(11):1924–37. doi: 10.1002/jclp.22680
9. Clever SL, Ford DE, Rubenstein LV, Rost KM, Meredith LS, Sherbourne CD, et al. Primary care patients’ involvement in decision-making is associated with improvement in depression. Med Care. (2006) 44:398–405. doi: 10.1097/01.mlr.0000208117.15531.da
10. Menear M, Girard A, Dugas M, Gervais M, Gilbert M, Gagnon MP. Personalized care planning and shared decision making in collaborative care programs for depression and anxiety disorders: a systematic review. PloS One. (2022) 17(6):e0268649. doi: 10.1371/journal.pone.0268649
11. Marshall T, Stellick C, Abba-Aji A, Lewanczuk R, Li XM, Olson K, et al. The impact of shared decision-making on the treatment of anxiety and depressive disorders: systematic review - CORRIGENDUM. BJPsych Open. (2021) 7(6):e212. doi: 10.1192/bjo.2021.1050
12. Joosten EAG, DeFuentes-Merillas L, de Weert GH, Sensky T, van der Staak CPF, de Jong CAJ. Systematic review of the effects of shared decision-making on patient satisfaction, treatment adherence and health status. Psychother Psychosom. (2008) 77(4):219–26. doi: 10.1159/000126073
13. Swift JK, Mullins RH, Penix EA, Roth KL, Trusty WT. The importance of listening to patient preferences when making mental health care decisions. World Psychiatry. (2021) 20(3):316–7. doi: 10.1002/wps.20912
14. National Institute for Health and Clinical Excellence. Overview | Depression in adults: treatment and management | Guidance | NICE. NICE (2022). Available at: https://www.nice.org.uk/guidance/ng222 (Accessed July 15, 2023).
15. Stacey D, Menard P, Gaboury I, Jacobsen M, Sharif F, Ritchie L, et al. Decision-making needs of patients with depression: a descriptive study. J Psychiatr Ment Health Nurs. (2008) 15(4):287–95. doi: 10.1111/j.1365-2850.2007.01224.x
16. Joseph-Williams N, Abhyankar P, Boland L, Bravo P, Brenner AT, Brodney S, et al. What works in implementing patient decision aids in routine clinical settings? A rapid realist review and update from the international patient decision aid standards collaboration. Med Decis Mak. (2021) 41(7):907–37. doi: 10.1177/0272989X20978208
17. Zisman-Ilani Y, Barnett E, Harik J, Pavlo A, O’Connell M. Expanding the concept of shared decision making for mental health: systematic search and scoping review of interventions. Ment Health Rev J. (2017) 22(3):191–213. doi: 10.1108/MHRJ-01-2017-0002
18. Stacey D, Légaré F, Lewis K, Barry MJ, Bennett CL, Eden KB, et al. Decision aids for people facing health treatment or screening decisions. Cochrane Database Syst Rev. (2017) 4:CD001431. doi: 10.1002/14651858.CD001431.pub5
19. Elwyn G, Frosch D, Volandes AE, Edwards A, Montori VM. Investing in deliberation: a definition and classification of decision support interventions for people facing difficult health decisions. Med Decis Mak. (2010) 30(6):701–11. doi: 10.1177/0272989X10386231
20. Elwyn G, O’Connor A, Stacey D, Volk R, Edwards A, Coulter A, et al. Developing a quality criteria framework for patient decision aids: online international Delphi consensus process. Br Med J. (2006) 333(7565):417. doi: 10.1136/bmj.38926.629329.AE
21. Elwyn G, O’Connor AM, Bennett C, Newcombe RG, Politi M, Durand MA, et al. Assessing the quality of decision support technologies using the international patient decision aid standards instrument (IPDASi). PLoS One. (2009) 4(3):e4705. doi: 10.1371/journal.pone.0004705
22. Lopez-Olivo MA, Suarez-Almazor ME. Digital patient education and decision aids. Rheum Dis Clin North Am. (2019) 45(2):245–56. doi: 10.1016/j.rdc.2019.01.001
23. Hassan N, Slight RD, Bimpong K, Weiand D, Vellinga A, Morgan G, et al. Clinicians’ and patients’ perceptions of the use of artificial intelligence decision aids to inform shared decision making: a systematic review. Lancet. (2021) 398:S80. doi: 10.1016/S0140-6736(21)02623-4
24. Biller-Andorno N, Biller A. The advance care compass–a new mechanics for digitally transforming advance directives. Front Digit Health. (2021) 3:753747. doi: 10.3389/fdgth.2021.753747
25. Hopwood M. The shared decision-making process in the pharmacological management of depression. Patient. (2020) 13(1):23–30. doi: 10.1007/s40271-019-00383-w
26. Simmons MB, Hetrick SE, Jorm AF. Experiences of treatment decision making for young people diagnosed with depressive disorders: a qualitative study in primary care and specialist mental health settings. BMC Psychiatry. (2011) 11:194. doi: 10.1186/1471-244X-11-194
27. Vaisson G, Provencher T, Dugas M, Trottier MÈ, Chipenda Dansokho S, Colquhoun H, et al. User involvement in the design and development of patient decision aids and other personal health tools: a systematic review. Med Decis Mak. (2021) 41(3):261–74. doi: 10.1177/0272989X20984134
28. Witteman HO, Maki KG, Vaisson G, Finderup J, Lewis KB, Steffensen KD, et al. Systematic development of patient decision aids: an update from the IPDAS collaboration. Med Decis Mak. (2021) 41(7):736–54. doi: 10.1177/0272989X211014163
29. Sepucha KR, Abhyankar P, Hoffman AS, Bekker HL, LeBlanc A, Levin CA, et al. Standards for universal reporting of patient decision aid evaluation studies: the development of SUNDAE checklist. BMJ Qual Saf. (2018) 27(5):380–8. doi: 10.1136/bmjqs-2017-006986
30. Hetrick S, Simmons M, Merry S. SSRIs and depression in children and adolescents: the imperative for shared decision-making. Australas Psychiatry. (2008) 16(5):354–8. doi: 10.1080/10398560802189888
31. Raue PJ, Schulberg HC, Lewis-Fernandez R, Boutin-Foster C, Hoffman AS, Bruce ML. Shared decision-making in the primary care treatment of late-life major depression: a needed new intervention? Int J Geriatr Psychiatry. (2010) 25(11):1101–11. doi: 10.1002/gps.2444
32. Perestelo-Perez L, Rivero-Santana A, Sanchez-Afonso JA, Perez-Ramos J, Castellano-Fuentes CL, Sepucha K, et al. Effectiveness of a decision aid for patients with depression: a randomized controlled trial. Health Expect. (2017) 20(5):1096–105. doi: 10.1111/hex.12553
33. Alarcon-Ruiz CA, Zafra-Tanaka JH, Diaz-Barrera ME, Becerra-Chauca N, Toro-Huamanchumo CJ, Pacheco-Mendoza J, et al. Effects of decision aids for depression treatment in adults: systematic review. BJPsych Bull. (2022) 46(1):42–51. doi: 10.1192/bjb.2020.130
34. Samalin L, Genty JB, Boyer L, Lopez-Castroman J, Abbar M, Llorca PM. Shared decision-making: a systematic review focusing on mood disorders. Curr Psychiatry Rep. (2018) 20(4):23. doi: 10.1007/s11920-018-0892-0
35. Matthews EB, Savoy M, Paranjape A, Washington D, Hackney T, Galis D, et al. Shared decision making in primary care based depression treatment: communication and decision-making preferences among an underserved patient population. Front Psychiatry. (2021) 12:681165. doi: 10.3389/fpsyt.2021.681165
36. Munn Z, Peters MDJ, Stern C, Tufanaru C, McArthur A, Aromataris E. Systematic review or scoping review? Guidance for authors when choosing between a systematic or scoping review approach. BMC Med Res Methodol. (2018) 18(1):143. doi: 10.1186/s12874-018-0611-x
37. Peters MDJ, Godfrey C, McInerney P, Khalil H, Larsen P, Marnie C, et al. Best practice guidance and reporting items for the development of scoping review protocols. JBI Evid Synth. (2022) 20(4):953–68. doi: 10.11124/JBIES-21-00242
38. Higgins JPT, Thomas J, Chandler J, Cumpston M, Li T, Page MJ, et al. Cochrane handbook for systematic reviews of interventions. 2nd Edition. Chichester, UK: John Wiley & Sons (2019).
39. Tricco AC, Lillie E, Zarin W, O’Brien KK, Colquhoun H, Levac D, et al. PRISMA extension for scoping reviews (PRISMA-ScR): checklist and explanation. Ann Intern Med. (2018) 169(7):467–73. doi: 10.7326/M18-0850
40. Lisy K, Porritt K. Narrative synthesis. Int J Evid Based Healthc. (2016) 14(4):201. doi: 10.1097/01.XEB.0000511348.97198.8c
41. Borenstein M, Hedges LV, Higgins JPT, Rothstein HR. Chapter 7: converting among effect sizes. In: Borenstein M, Hedges LV, Higgins JPT, Rothstein HR, editors. Introduction to meta-analysis. Chichester, UK: Wiley (2009). p. 45–9. doi: 10.1002/9780470743386.ch7
43. Higgins JPT, Thomas J, Chandler J, Cumpston M, Li T, Page MJ, Welch VA. Choosing effect measures and computing estimates of effect. In: Higgins JPT, Thomas J, Chandler J, Cumpston M, Li T, Page MJ, Welch VA, editors. Cochrane handbook for systematic reviews of interventions 2nd Edition. Chichester (UK): John Wiley & Sons (2019). p. 143–76.
44. Rosenthal R, DiMatteo MR. Meta-analysis: recent developments in quantitative methods for literature reviews. Annu Rev Psychol. (2001) 52:59–82. doi: 10.1146/annurev.psych.52.1.59
45. Morris SB. Estimating effect sizes from pretest-posttest-control group designs. Organ Res Methods. (2008) 11(2):364–86. doi: 10.1177/1094428106291059
46. Abousheishaa AA, Lazim NHM, Tang SL, Sulaiman AH, Huri HZ, Guan NC. Antidepressant decision aid for major depressive disorder patients (ADAM): development and pilot testing. Patient Educ Couns. (2022) 105(7):2466–74. doi: 10.1016/j.pec.2021.11.007
47. Aljumah K, Hassali MA. Impact of pharmacist intervention on adherence and measurable patient outcomes among depressed patients: a randomised controlled study. BMC Psychiatry. (2015) 15:219. doi: 10.1186/s12888-015-0605-8
48. Aoki Y, Takaesu Y, Baba H, Iga JI, Hori H, Inoue T, et al. Development and acceptability of a decision aid for major depressive disorder considering discontinuation of antidepressant treatment after remission. Neuropsychopharmacol Rep. (2022) 42(3):306–14. doi: 10.1002/npr2.12269
49. Aoki Y, Takaesu Y, Inoue M, Furuno T, Kobayashi Y, Chiba H, et al. Seven-day shared decision making for outpatients with first episode of mood disorders among university students: a randomized controlled trial. Psychiatry Res. (2019) 281:112531. doi: 10.1016/j.psychres.2019.112531
50. Barr PJ, Forcino RC, Dannenberg MD, Mishra M, Turner E, Zisman-Ilani Y, et al. Healthcare options for people experiencing depression (HOPE*D): the development and pilot testing of an encounter-based decision aid for use in primary care. BMJ Open. (2019) 9(4):e025375. doi: 10.1136/bmjopen-2018-025375
51. Beaulac J, Westmacott R, Walker JR, Vardanyan G. Primary care provider views about usefulness and dissemination of a web-based depression treatment information decision aid. J Med Internet Res. (2016) 18(6):e153. doi: 10.2196/jmir.5458
52. Brodney S, Valentine KD, Sepucha K. Psychometric evaluation of a decision quality instrument for medication decisions for treatment of depression symptoms. BMC Med Inform Decis Mak. (2021) 21(1):252. doi: 10.1186/s12911-021-01611-w
53. Broughton LC, Medlicott NJ, Smith AJ. Effectiveness of patient decision aids in women considering psychotropic medication use during pregnancy: a literature review. Arch Womens Ment Health. (2021) 24(4):569–78. doi: 10.1007/s00737-021-01118-3
54. Dannenberg MD, Bienvenida JCM, Bruce ML, Nguyen T, Hinn M, Matthews J, et al.. End-user views of an electronic encounter decision aid linked to routine depression screening. Patient Educ Couns. (2019) 102(3):555–63. doi: 10.1016/j.pec.2018.10.002
55. Fisher A, Marel C, Teesson M, Mills K. Assessing patient information and decision-support needs in problematic alcohol use and co-occurring depression to inform shared decision-making interventions. Bull Menninger Clin. (2021) 85(2):143–76. doi: 10.1521/bumc.2021.85.2.143
56. Gordon M, Henderson R, Holmes JH, Wolters MK, Bennett IM. Participatory design of ehealth solutions for women from vulnerable populations with perinatal depression. J Am Med Inform Assoc. (2016) 23(1):105–9. doi: 10.1093/jamia/ocv109
57. Hussain-Shamsy N, Somerton S, Stewart DE, Grigoriadis S, Metcalfe K, Oberlander TF, et al. The development of a patient decision aid to reduce decisional conflict about antidepressant use in pregnancy. BMC Med Inform Decis Mak. (2022) 22(1):130. doi: 10.1186/s12911-022-01870-1
58. Khalifeh H, Molyneaux E, Brauer R, Vigod S, Howard LM. Patient decision aids for antidepressant use in pregnancy: a pilot randomised controlled trial in the UK. BJGP Open. (2019) 3(4). doi: 10.3399/bjgpopen19X101666
59. Kivelitz L, Härter M, Mohr J, Melchior H, Goetzmann L, Warnke MH, et al. Choosing the appropriate treatment setting: which information and decision-making needs do adult inpatients with mental disorders have? A qualitative interview study. Patient Prefer Adherence. (2018) 12:823–33. doi: 10.2147/PPA.S164160
60. Kroenke K. The role of decision aids in depression care. JAMA Intern Med. (2015) 175(11):1770–2. doi: 10.1001/jamainternmed.2015.5243
61. LeBlanc A, Bodde AE, Branda ME, Yost KJ, Herrin J, Williams MD, et al. Translating comparative effectiveness of depression medications into practice by comparing the depression medication choice decision aid to usual care: study protocol for a randomized controlled trial. Trials. (2013) 14:127. doi: 10.1186/1745-6215-14-127
62. LeBlanc A, Herrin J, Williams MD, Inselman JW, Branda ME, Shah ND, et al. Shared decision making for antidepressants in primary care: a cluster randomized trial. JAMA Intern Med. (2015) 175(11):1761–70. doi: 10.1001/jamainternmed.2015.5214
63. Loh A, Simon D, Wills CE, Kriston L, Niebling W, Härter M. The effects of a shared decision-making intervention in primary care of depression: a cluster-randomized controlled trial. Patient Educ Couns. (2007) 67(3):324–32. doi: 10.1016/j.pec.2007.03.023
64. Loh A, Simon D, Härter M. Effekte der patientenbeteiligung in der grundversorgung depressiver patienten - höhere therapietreue und bessere behandlungsergebnisse. Klin. (2007) 37(01):38–41.
65. Raue PJ, Schulberg HC, Bruce ML, Banerjee S, Artis A, Espejo M, et al. Effectiveness of shared decision-making for elderly depressed minority primary care patients. Am J Geriatr Psychiatry. (2019) 27(8):883–93. doi: 10.1016/j.jagp.2019.02.016
66. Raue PJ, Sirey JA. Designing personalized treatment engagement interventions for depressed older adults. Psychiatr Clin North Am. (2011) 34(2):489–500. doi: 10.1016/j.psc.2011.02.011
67. Reis J. A case report of college Students’ rating of a shared decision-making tool for taking antidepression medication. J Patient Exp. (2021) 8:23743735211007350. doi: 10.1177/23743735211007352
68. Reuter K, Genao K, Callanan EM, Cannone DE, Giardina EG, Rollman BL, et al. Increasing uptake of depression screening and treatment guidelines in cardiac patients: a behavioral and implementation science approach to developing a theory-informed, multilevel implementation strategy. Circ Cardiovasc Qual Outcomes. (2022) 15(11):e009338. doi: 10.1161/CIRCOUTCOMES.122.009338
69. Rogojanski J, Zeifman RJ, Antony MM, Walker JR, Monson CM. Evaluation of a decision aid for the treatment of depression among college students. J Am Coll Health. (2022) 70(6):1634–43. doi: 10.1080/07448481.2020.1817034
70. Shillington AC, Langenecker SA, Shelton RC, Foxworth P, Allen L, Rhodes M, et al. Development of a patient decision aid for treatment resistant depression. J Affect Disord. (2020) 275:299–306. doi: 10.1016/j.jad.2020.07.014
71. Simmons MB, Hetrick SE, Jorm AF. Making decisions about treatment for young people diagnosed with depressive disorders: a qualitative study of clinicians’ experiences. BMC Psychiatry. (2013) 13:335. doi: 10.1186/1471-244X-13-335
72. Simmons MB, Elmes A, McKenzie JE, Trevena L, Hetrick SE. Right choice, right time: evaluation of an online decision aid for youth depression. Health Expect. (2017) 20(4):714–23. doi: 10.1111/hex.12510
73. Simon D, Kriston L, von Wolff A, Buchholz A, Vietor C, Hecke T, et al. Effectiveness of a web-based, individually tailored decision aid for depression or acute low back pain: a randomized controlled trial. Patient Educ Couns. (2012) 87(3):360–8. doi: 10.1016/j.pec.2011.10.009
74. Starks H, Shaw JL, Hiratsuka V, Dillard DA, Robinson R. Engaging stakeholders to develop a depression management decision support tool in a tribal health system. Qual Life Res. (2015) 24(5):1097–105. doi: 10.1007/s11136-014-0810-9
75. Vigod S, Hussain-Shamsy N, Grigoriadis S, Howard LM, Metcalfe K, Oberlander TF, et al. A patient decision aid for antidepressant use in pregnancy: study protocol for a randomized controlled trial. Trials. (2016) 17(1):110. doi: 10.1186/s13063-016-1233-4
76. Vigod SN, Wilson CA, Howard LM. Authors’ reply to braillon and bewley. Br Med J. (2016) 353:i2583. doi: 10.1136/bmj.i2583
77. Vigod SN, Hussain-Shamsy N, Stewart DE, Grigoriadis S, Metcalfe K, Oberlander TF, et al.. A patient decision aid for antidepressant use in pregnancy: pilot randomized controlled trial. J Affect Disord. (2019) 251:91–9. doi: 10.1016/j.jad.2019.01.051
78. Weiss F, Vietor C, Hecke TL. Use of routine data for evaluation purposes in sickness funds - evaluation of the TK-patientendialog. Gesundheitswesen. (2010) 72(6):371–8. doi: 10.1055/s-0030-1249702
79. Herrmann A, Hall A, Zdenkowski N, Sanson-Fisher R. Heading in a new direction? Recommendations for future research on patient decision aids. Patient Educ Couns Mai. (2019) 102(5):1029–34. doi: 10.1016/j.pec.2018.12.006
80. Hoefel L, Lewis KB, O’Connor A, Stacey D. 20th Anniversary update of the Ottawa decision support framework: part 2 subanalysis of a systematic review of patient decision aids. Med Decis Mak. (2020) 40(4):522–39. doi: 10.1177/0272989X20924645
81. Benrimoh D, Tanguay-Sela M, Perlman K, Israel S, Mehltretter J, Armstrong C, et al. Using a simulation centre to evaluate preliminary acceptability and impact of an artificial intelligence-powered clinical decision support system for depression treatment on the physician-patient interaction. BJPsych Open. (2021) 7(1):e22. doi: 10.1192/bjo.2020.127
82. Ramos-García V, Perestelo-Pérez L, Rivero-Santana A, Peñate-Castro W, Duarte-Díaz A, Álvarez-Pérez Y, et al. Decision aids linked to the recommendations in clinical practice guidelines: results of the acceptability of a decision aid for patients with generalized anxiety disorder. BMC Med Inform Decis Mak. (2022) 22(1):171. doi: 10.1186/s12911-022-01899-2
83. Vromans R, Tenfelde K, Pauws S, van Eenbergen M, Mares-Engelberts I, Velikova G, et al. Assessing the quality and communicative aspects of patient decision aids for early-stage breast cancer treatment: a systematic review. Breast Cancer Res Treat. (2019) 178(1):1–15. doi: 10.1007/s10549-019-05351-4
84. Jayakumar P, Moore MG, Furlough KA, Uhler LM, Andrawis JP, Koenig KM, et al. Comparison of an artificial intelligence-enabled patient decision aid vs educational material on decision quality, shared decision-making, patient experience, and functional outcomes in adults with knee osteoarthritis: a randomized clinical trial. JAMA Netw Open. (2021) 4(2):e2037107. doi: 10.1001/jamanetworkopen.2020.37107
85. Thomson MD, Hoffman-Goetz L. Readability and cultural sensitivity of web-based patient decision aids for cancer screening and treatment: a systematic review. Med Inform Internet Med. (2007) 32(4):263–86. doi: 10.1080/14639230701780408
86. Motorny S, Sarnikar S, Noteboom C. Design of an intelligent patient decision aid based on individual decision-making styles and information need preferences. Inf Syst Front. (2021) 24:1249–64. doi: 10.1007/s10796-021-10125-9
87. Heen AF, Vandvik PO, Brandt L, Achille F, Guyatt GH, Akl EA, et al. Decision aids linked to evidence summaries and clinical practice guidelines: results from user-testing in clinical encounters. BMC Med Inform Decis Mak. (2021) 21(1):202. doi: 10.1186/s12911-021-01541-7
88. Keikes L, de Vos-Geelen J, de Groot JWB, Punt CJA, Simkens LHJ, Trajkovic-Vidakovic M, et al. Implementation, participation and satisfaction rates of a web-based decision support tool for patients with metastatic colorectal cancer. Patient Educ Couns. (2019) 102(7):1331–5. doi: 10.1016/j.pec.2019.02.020
89. van der Weijden T, Boivin A, Burgers J, Schünemann HJ, Elwyn G. Clinical practice guidelines and patient decision aids. An inevitable relationship. J Clin Epidemiol. (2012) 65(6):584–9. doi: 10.1016/j.jclinepi.2011.10.007
90. Scalia P, Saunders CH, Dannenberg M, Mc Giguere A, Alper BS, Hoffmann T, et al. Processes for evidence summarization for patient decision aids: a Delphi consensus study. Health Expect. (2021) 24(4):1178–86. doi: 10.1111/hex.13244
91. Tong WT, Ng CJ, Lee YK, Lee PY. What will make patients use a patient decision aid? A qualitative study on patients’ perspectives on implementation barriers and facilitators. J Eval Clin Pract Juni. (2020) 26(3):755–64. doi: 10.1111/jep.13161
92. Gibson A, Cooper M, Rae J, Hayes J. Clients’ experiences of shared decision making in an integrative psychotherapy for depression. J Eval Clin Pract. (2020) 26(2):559–68. doi: 10.1111/jep.13320
93. Whybrow R, Webster LM, Seed PT, Sandall J, Chappell LC. The effectiveness of decision aids for pregnancy related decision-making in women with pre-pregnancy morbidity; systematic review and meta-analysis. BMC Pregnancy Childbirth. (2022) 22(1):81. doi: 10.1186/s12884-022-04402-x
94. Zhu A, Phuong M, Giacobbe P. The story of ECT: behind the scenes of a controversial yet effective treatment. Comics Grid. (2018) 8(1). doi: 10.16995/cg.129
95. Arandjelovic K, Eyre HA, Lenze E, Singh AB, Berk M, Bousman C. The role of depression pharmacogenetic decision support tools in shared decision making. J Neural Transm Vienna Austria. (2019) 126(1):87–94. doi: 10.1007/s00702-017-1806-8
96. Sanchez K, Killian MO, Eghaneyan BH, Cabassa LJ, Trivedi MH. Culturally adapted depression education and engagement in treatment among hispanics in primary care: outcomes from a pilot feasibility study. BMC Fam Pract. (2019) 20(1):140. doi: 10.1186/s12875-019-1031-7
97. Bell RA, Taylor LD, Kravitz RL. Do antidepressant advertisements educate consumers and promote communication between patients with depression and their physicians? Patient Educ Couns. (2010) 81(2):245–50. doi: 10.1016/j.pec.2010.01.014
98. Wicks P, Massagli M, Frost J, Brownstein C, Okun S, Vaughan T, et al. Sharing health data for better outcomes on PatientsLikeMe. J Med Internet Res. (2010) 12(2):e19. doi: 10.2196/jmir.1549
99. White JH, Towers SE, Turner A, Hambridge J. Electronic screening and decision support for poststroke depression: an exploration of doctors’ and patients’ perceptions of acceptability. Arch Phys Med Rehabil. (2013) 94(4):788–90. doi: 10.1016/j.apmr.2012.09.009
100. Goodyear-Smith F, Warren J, Elley CR. The eCHAT program to facilitate healthy changes in New Zealand primary care. J Am Board Fam Med. (2013) 26(2):177–82. doi: 10.3122/jabfm.2013.02.120221
101. Bonfils KA, Dreison KC, Luther L, Fukui S, Dempsey AE, Rapp CA, et al. Implementing CommonGround in a community mental health center: lessons in a computerized decision support system. Psychiatr Rehabil J. (2018) 41(3):216–23. doi: 10.1037/prj0000225
102. Fisher A, Sharpe L, Anderson J, Manicavasagar V, Juraskova I. Development and pilot of a decision-aid for patients with bipolar II disorder and their families making decisions about treatment options to prevent relapse. PloS One. (2018) 13(7):e0200490. doi: 10.1371/journal.pone.0200490
103. Al Jumah K. The impact of a decision aid on depressed patients’ involvement in shared decision-making: a pilot randomized controlled double-blinded study. Value Health. (2016) 19(3):A19. doi: 10.1016/j.jval.2016.03.409
104. O’Connor AM, Bennett CL, Stacey D, Barry M, Col NF, Eden KB, et al. Decision aids for people facing health treatment or screening decisions. Chichester, UK: John Wiley & Sons, Ltd (2009).
Keywords: patient decision aids, shared decision making, autonomy, depression, mental health, digitalization
Citation: Sedlakova J, Westermair AL, Biller-Andorno N, Meier CA and Trachsel M (2023) Comparison of analog and digital patient decision aids for the treatment of depression: a scoping review. Front. Digit. Health 5:1208889. doi: 10.3389/fdgth.2023.1208889
Received: 19 April 2023; Accepted: 18 August 2023;
Published: 1 September 2023.
Edited by:
Bárbara Oliván Blázquez, University of Zaragoza, SpainReviewed by:
Graciela Rojas, University of Chile, ChileYaara Zisman-Ilani, Temple University, United States
© 2023 Sedlakova, Westermair, Biller-Andorno, Meier and Trachsel. This is an open-access article distributed under the terms of the Creative Commons Attribution License (CC BY). The use, distribution or reproduction in other forums is permitted, provided the original author(s) and the copyright owner(s) are credited and that the original publication in this journal is cited, in accordance with accepted academic practice. No use, distribution or reproduction is permitted which does not comply with these terms.
*Correspondence: Manuel Trachsel bWFudWVsLnRyYWNoc2VsQHVzYi5jaA==
†These authors have contributed equally to this work