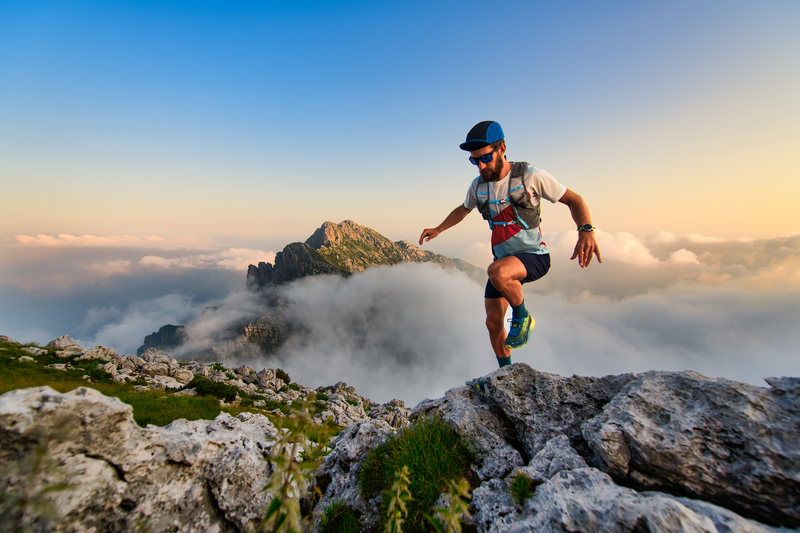
94% of researchers rate our articles as excellent or good
Learn more about the work of our research integrity team to safeguard the quality of each article we publish.
Find out more
SYSTEMATIC REVIEW article
Front. Digit. Health , 22 May 2023
Sec. Digital Mental Health
Volume 5 - 2023 | https://doi.org/10.3389/fdgth.2023.1170002
This article is part of the Research Topic Intelligent Approaches to Smart Mental Health Monitoring and Management: Implementation, Validation and Promotion View all 8 articles
Introduction: Personalization is a much-discussed approach to improve adherence and outcomes for Digital Mental Health interventions (DMHIs). Yet, major questions remain open, such as (1) what personalization is, (2) how prevalent it is in practice, and (3) what benefits it truly has.
Methods: We address this gap by performing a systematic literature review identifying all empirical studies on DMHIs targeting depressive symptoms in adults from 2015 to September 2022. The search in Pubmed, SCOPUS and Psycinfo led to the inclusion of 138 articles, describing 94 distinct DMHIs provided to an overall sample of approximately 24,300 individuals.
Results: Our investigation results in the conceptualization of personalization as purposefully designed variation between individuals in an intervention's therapeutic elements or its structure. We propose to further differentiate personalization by what is personalized (i.e., intervention content, content order, level of guidance or communication) and the underlying mechanism [i.e., user choice, provider choice, decision rules, and machine-learning (ML) based approaches]. Applying this concept, we identified personalization in 66% of the interventions for depressive symptoms, with personalized intervention content (32% of interventions) and communication with the user (30%) being particularly popular. Personalization via decision rules (48%) and user choice (36%) were the most used mechanisms, while the utilization of ML was rare (3%). Two-thirds of personalized interventions only tailored one dimension of the intervention.
Discussion: We conclude that future interventions could provide even more personalized experiences and especially benefit from using ML models. Finally, empirical evidence for personalization was scarce and inconclusive, making further evidence for the benefits of personalization highly needed.
Systematic Review Registration: Identifier: CRD42022357408.
At an estimated lifetime prevalence of more than 10% (1, 2), major depressive disorder (MDD) is the second leading cause of years lived in disability (3). While this makes efficient treatments urgently needed, traditional approaches such as face-to-face psychotherapy are difficult to access for a significant part of patients (4–6). However, providing treatment through digital channels such as mobile applications and online formats (7) is effective in reducing depressive symptoms (8, 9) in a cost-effective way (10). Since most of the world population has access to the internet (11) and/or a smartphone (12), digital mental health interventions (DMHIs) bypass barriers to traditional treatment.
Despite their potential, DMHIs inherit some of the general problems in depression treatment: Estimates for treatment dropout, as observed in RCTs, are up to 50% when considering publication bias (13). Moreover, response rates are unsatisfactory at less than 50% (14). Therefore, improving outcomes and reducing dropouts in DMHIs are expected to be highly impactful in facing the burden of depression.
Luckily, DMHIs' unique delivery channel provides new opportunities to improve the treatment of those suffering from depressive symptoms. Specifically, digital applications can efficiently be individualized to improve users' experience and outcomes, as observable across many other domains, ranging from e-commerce (15) over e-learning (16) towards social media (17). Simultaneously, the importance of accommodating patients’ preferences for treatment outcomes in mental healthcare has been well established (18). Hence, the personalization of interventions to adapt treatment to individual needs is a promising approach to improving care, for depressive symptoms and beyond (19–22).
In line with that idea, a meta-analysis from 2013 showed that algorithm-based tailoring of DMHIs is associated with better outcomes (23). A review from 2022 found that none of the 26 reviewed apps for depression used just-in-time (JIT) adaptations, a mechanism for personalizing the timing of content delivery based on the individual or the situation (24). Another current systematic review investigated tailored interventions for workplace mental health (25), finding benefits on several outcomes when content or feedback was tailored towards the individual. Finally, a component network analysis examined the benefits of common internet-based cognitive behavioral therapy (iCBT) packages for depression, discovering small interactions between treatment components and patient characteristics (26).
While these publications are unified in their call for more personalization in DMHIs, they do not add up to a satisfactory empirical and theoretical ground for it. Firstly, the fragmented use of vocabulary fails to demarcate personalization from other distinct phenomena related to variability in DMHIs. For example, the term “tailoring” is used across various scopes and foci (23, 25, 27), while similar mechanisms are elsewhere called “individualized” (28) or “personalized” (26, 29). This diversity in vocabulary is shared with non-digital settings, as for traditional psychotherapy, 15 different terms for the same phenomena of varying treatment between individuals were reported (30). Secondly, in contrast to the breadth of used vocabulary, the focus of mechanisms within studies seems to be relatively narrow, focusing on specific mechanisms (23, 24) or areas (25, 28) of personalization. This potentially leads to an underestimation of variability already in place. Finally, while two of the mentioned reviews investigated the benefits of personalization through direct comparisons, they did so without a specific focus on depression and, related to the aforementioned narrow conceptualizations of personalization, with few studies being included. In conclusion, the concept, prevalence, and efficacy of personalization in DMHIs for depressive symptoms are not adequately delineated. Therefore, a disorder-specific review developing a conceptual framework for personalization and reviewing a wide span of interventions seems needed.
This systematic review aims to reduce the gap between the potential of personalization and its actual implementation by performing a comprehensive review of DMHIs for depressive symptoms with the following purposes:
1. Extract a conceptual framework that allows a clear and meaningful way of investigating, discussing, and classifying personalization.
2. Apply this framework to the available literature and report current use and mechanisms.
3. Evaluate the available evidence by identifying studies that directly compare interventions with different degrees of personalization.
This review was planned and reported following the Preferred Reporting Items for Systematic Reviews and Meta-Analyses (PRISMA) guidelines (31). The protocol of this review was registered in the International Prospective Register of Systematic Reviews of the National Institute for Health Research (PROSPERO) under the ID CRD42022357408. The protocol was updated once after initial piloting to improve the alignment of the inclusion criteria and data extraction method with the scope of the review. Specifically, a new classification dimension for personalization was added that occurred in the literature and did not fit the pre-defined schema and the exclusion of e.g., prenatal depression was added to improve the comparability between included interventions. The final version of the protocol can be found in the Supplementary Appendix S1.
In the first step, a search was performed in three major databases (SCOPUS, PubMed, PsycInfo) to identify all published studies on DMHIs for depressive symptoms. The full search strings can be found in Supplementary Appendix S2. Additionally, three related reviews (13, 14, 32) were screened, and studies not yet included were added. Finally, papers brought to the author's awareness by being discussed in our included articles, not included yet but fulfilling our selection criteria, were added.
We included empirical studies on DMHIs specifically targeting depressive symptoms, determining the interventions target by authors' self-report. This covered both, patients with diagnosed major depressive disorder (MDD), as well as with subclinical levels of symptoms. To be considered a DMHI, interventions needed to be delivered through the internet and/or a smartphone. We included only empirical, peer-reviewed, English studies and conference articles with original data and patient cohort. To ensure a focus on the most relevant interventions for current use, we start our search from 2015 onwards.
To narrow down the focus of this review, studies on interventions targeting comorbid disorders such as anxiety were excluded. The same applied to those targeting a specific subtype of depression (e.g., prenatal depression), a single sub-symptom (e.g., rumination), or adolescent or elderly people (below 18 years or >64 years). Finally, those studies using digital technologies exclusively as a means of communication, such as one-on-one psychotherapy delivered via the web, were excluded as well.
One of the researchers (S.H.) performed an initial screening based on the title and abstract of the studies identified through the search strategy. A second researcher (K.Z.) conducted the same procedure for a randomly chosen subset of 100 studies, resulting in excellent interrater reliability (0.94). The full description of the intervention was then read by both reviewers for all remaining papers to determine the final selection, extract interventions and code the variables of interest. Disagreements on any aspect of this process were solved by discussion between the reviewers until a consensus was reached. If full texts were unavailable, they were requested from the corresponding author. This occurred 12 times, with 8 of the articles made available on request.
During the initial screening and before the update of the PROSPERO registration, we developed the proposed framework in an iterative process, considering usability, conceptual literature, and the observed interventions. Specifically, we discussed how we could classify personalization in a way that allows us to not just cover all mechanisms in the literature but also maximize usability by defining the dimensions as distinct as possible. We did this as we needed a method to classify personalization mechanisms during the systematic review and we could not find a satisfactory framework in the literature yet.
We departed from a common dictionary definition defining personalization as “the action of designing or producing something that meets someone's individual requirement” (33). Based on that, we intended to classify personalization in DMHIs in a broad enough way to cover the diversity of mechanisms present in related reviews and studies. At the same time, we intended to narrow down the concept to those mechanisms affecting the therapeutic content and structure, setting it apart from superficial sources of variability. Therefore, we excluded interactivity (34), the sole replay of user input as part of the app experience. For example, showing each patient their previously set goal might be a powerful tool, but it does not change the underlying therapeutic elements delivered. Additionally, we factored out customization (35), minor aesthetic adaptation such as users ability to change the color of an avatar. Finally, seeing personalization as referring to the level of the individual patient, we excluded group-based variability, such as cultural adaptation of the entire intervention (36).
Numerous screened interventions used a structured session-based approach to deliver their intervention—a common approach among manualized mental health interventions (37). Therefore, we identified (a) content (what is delivered during a session) and (b) order (how sessions are ordered) as potential areas of personalization. Since (c) guidance (level of human contact) is a highly relevant and variable aspect of DMHIs (38) we added it as another dimension. Finally, as we discovered prompts and mechanisms targeting the timing of interventions not being sufficiently represented in these three categories, we appended (d) communication as another dimension.
While, as mentioned above, we intended to exclude customization as minor user-choice-based adaptations of the intervention, we did not exclude user choice per se from our concept. This differs from the use in fields like marketing, where anything done by the user is defined as customization, not personalization (35). However, we saw the inclusion of actively designed user choice being justified for the following reasons: Firstly, user choice was a common mechanism described in the included interventions. Secondly, those mechanisms seem easily implementable and therefore highly relevant for practitioners interested in personalizing their intervention. Finally, user agency has been shown to be particularly relevant in mental healthcare (18). We also identified provider choice as another mechanism for guided and blended interventions. For data-driven personalization mechanisms, we saw rule-based and ML as distinct mechanisms applying static or learning criteria for personalization.
The framework developed above was applied to all identified interventions, coding the presence of personalization for each of the four (a–d) dimensions and classifying the underlying mechanism. For this, interventions had to be extracted from the included studies, and information from several studies on the same intervention had to be merged. If more than one distinct intervention was presented in a study, they were coded separately. Intervention versions in different languages were not coded separately if not reported to be clearly distinct in their content. If more than one study was available, a single observation of personalization resulted in a positive coding, but conflicting information was noted. Additionally, cited material such as older papers, weblinks, or appendices were consulted in the refrained from additional free-hand research on the reported interventions. In case information was indicative of personalization but insufficient for our coding, we contacted the corresponding author and asked for clarification. For this, we provided a four-week response window, including one reminder. Out of the seven authors contacted, six responded by providing additional information. In the single case where authors did not respond (39) we decided to code restrictively and assume the simpler of the potential mechanisms involved (rule-based instead of ML). Finally, for evaluating the evidence for personalization, we included every study that directly compared intervention versions that differed in their degree of personalization, according to our framework. We extracted effect sizes, dependent variables, and sample sizes for those.
Overall, we identified 3.143 potentially relevant publications and screened the title and abstract. For 213 of those, the full intervention description was reviewed, resulting in the final inclusion of N = 138 papers describing k = 94 distinct DMHIs for depressive symptoms (see Figure 1) (39–175).
While mostly one study per intervention was included, for some up to seven publications on distinct trials were present and kept for further analysis. Across all studies, the reviewed interventions were deployed to approximately 24.300 participants, with an average sample size of 259 participants per intervention (range 1–2964). 75 of the interventions were used in a randomized controlled trial, with the remaining evidence coming from feasibility studies, naturalistic routine care data, and other study designs.
Most interventions had a duration between 6 and 12 weeks, and around 40 of the interventions report a structured module-/ session-based design, delivering the content in pre-defined blocks. Finally, 38 interventions were unguided (no human contact within the intervention), 32 guided (including guidance from clinician or coach), and 14 blended (combining face-to-face and digital treatment), with the remaining 10 covering more than one of those categories. An overview of all characteristics can be found in Supplementary Appendix S3.
A conceptual framework of personalization in DMHIs was synthesized from the reviewed DMHIs and theoretical considerations. In summary, an understanding of personalization as purposefully designed variation between individuals in an intervention's therapeutic elements or its structure emerged. As such, personalization is differentiated from customization, usage, interactivity, and group-based adaptations. Customization describes minor adjustments, such as visual aspects, leaving the actual therapeutic ingredients unchanged. Usage refers to possible user-induced differences in app usage that were not actively or purposefully designed. For example, variability in the time spent on a module is usage, the offering of short and long versions of a module qualifies as personalization. Interactivity, the mere replay of user input as for example commonly used for goal-setting exercises, as this leaves the actual therapeutic elements and structure unchanged. Finally, as we understand personalization as referring to the level of the individual, we see it as being distinct from group-based variability, such as the adaptation for a particular cultural context (see Figure 2).
Figure 2. Personalization in comparison to the terms usage, customization, interactivity and group-based adaption.
Within our definition of personalization, four personalizable intervention dimensions emerged, namely content, guidance level, order, and communication, as summarized in Figure 3. Content describes all variability in the delivered intervention material, such as exercises, psychoeducative material or topics presented. Order includes cases when patients receive the same content but in different order. Guidance refers to the extent of therapeutic support offered. Communication concerns the channel, timing, and content of actively offered information outside of the intervention's content. This primarily includes prompts or reminder messages. Mechanisms regarding the frequency and timing of the intervention, such as JIT mechanisms, also fall under communication.
Further, four different mechanisms beyond personalization emerged: user choice, provider choice, rule-based and ML-based personalization (see Figure 4). User choice covers intentionally designed personalization based on the direct choice of the participant. For provider choice, either the individual providing guidance, or the clinician involved in a blended setting makes the personalization decision. Among automated personalization mechanisms, rule-based (if-then-decision rules) from Machine Learning (decisions with “learned” decision criteria) personalization mechanisms are gathered.
Applying the proposed framework for classifying variability in DMHIs, personalization was reported for 62 of the 94 interventions (66%). Most prominently, personalization mechanisms were used in the content for 30 of the interventions (32%). This was followed by personalized communication (30%), type (25%), and order (4%). 43 of the 62 (69%) interventions with a reported personalization mechanism did so for only a single dimension, while one DMHI reported a mechanism for all four subdomains of their intervention (60–66).
Across the 107 reported personalization mechanisms, rule-based was most prominent, being used in 51 cases (48%). User choice was observed in 39 cases (36%), and providers were involved in personalization 14 times (13%). The use of machine learning was reported three times (3%). Rule-based personalization was particularly prominent in the communication domain, accounting for 21 occurrences. Similarly, human guidance was personalized using decision rules 16 times. For content, user choice had a more prominent role, being reported 15 times. The use of personalization is summarized in Figure 5, with examples of the 3 most strategies being presented in Table 1. The share of interventions applying at least one personalization mechanism was the highest for guided interventions (72%), followed by unguided (63%) and tailed by blended (57%) interventions. Generally, the dimensions of personalization were equally spread across guidance levels. However, provider choice was nearly twice as common for blended than for guided interventions.
Among the 55 automated mechanisms used, most were rule-based mechanisms of personalization. Here, activity data was heavily utilized, for example, for reminders in case of inactivity. Another common pattern was the use of symptom scores like the PHQ to step up care in the form of additional guidance (57) or the change from guided to blended care (169). While those approaches mostly used overall symptom severity, one exemption was the personalization based on suicide risk as e.g., in the form of additional prompts (146).
We identified three clear use cases of ML techniques for personalization. Firstly, EmoRecorder (70) used an activity recommendation system based on diverse data sources like app activity, sensor data and past recommendations. However, the intervention was at an early stage, being tested on a sample of only 15 healthy individuals. Secondly, the intervention MOSS (136) built on a JIT framework to assign intervention content depending on users' context and preferences. As such, it tested a recommender system with a sample of 126 adults. A third recommender system approach, so-called MUBS (137), applied a combination of ML and user choice by providing the 17 patients with a set of content recommendations.
Among the 138 papers in the final review, we identified two papers that included a direct comparison of a more and a less personalized version of an intervention. One study had participants fill out a questionnaire on motivational schemata and either matched them with an intervention arm to fit their motivational preference or a general one (40). Results showed effects for one of the two included motives (“being supported”) on anticipated adherence, working alliance, and satisfaction; however, the overall sample size of this trial was just 55 participants. Secondly, a study compared a program version including JIT prompts with one without those prompts, therefore, differing the personalization in the communication domain between trial arms (93). While both versions showed significant effects compared to the waitlist, no effects were reported between the arms. Again, this should be interpreted with caution, considering the sample size of around 60 individuals per group.
In recent years, personalization has been widely discussed as a promising avenue to improve DMHI adherence and outcomes. Nevertheless, it remains unclear what it entails and how it is used. In this review, we address this need for the case of depressive symptoms, by defining personalization as purposefully designed variation in intervention content, order, guidance, or communication. As possible mechanisms to operationalize personalization, we extract user choice, provider choice, decision rules, and ML. Applying this framework to 94 interventions for depressive symptoms reveals that two-thirds use at least one technique for personalization. Especially rule-based personalization of communication and guidance and user choice-based personalization of content is common. However, among interventions applying personalization, a majority does so just for one out of four dimensions of the intervention. Also, the use of ML models is scarce and limited to feasibility studies. Additionally, just two of the included studies investigated the benefits of personalization, both having small samples and just one finding supporting evidence.
Arguably, the biggest contrast between the proposed potentials in the personalization of DMHIs (19–22) and the existing literature is the lack of implemented ML mechanisms. Several of the implemented non-learning algorithms and decision rules were well designed. Yet, literature on ML in DMHIs reveals ample further promising and feasible use cases. Firstly, a notable body of research provides encouraging results in outcome (176, 177) and dropout (178, 179) predictions in DMHIs. Adapting the interventions for assumed non-responders is a low-hanging fruit and has already been successful for other disorders (180). Secondly, a prominent algorithmic approach to personalization in digital products is recommender systems (181–183). While all included ML approaches were such recommender systems, they were in early stages and deployed to very small sample sizes. Finally, all included ML approaches focused on the content of the intervention. However, ML also is a promising approach to personalize guidance, communication and order.
Contrasting theory and observations in another dimension, the data used for personalization just samples a fraction of the technically possible. While app usage patterns are an obvious data option, smartphones can also measure sleep patterns (184), physical activity (185), social interactions (186), and many other data points known to be relevant for depressive symptoms. Readily available toolkits like Apple's health kit (187) reduce the effort for implementation significantly. However, particularly passive sensing was rarely utilized in the reviewed interventions. Notably, the potential of ML-based personalization is heavily intertwined with the quality of the data available to them. Beyond that, aspects such as ethical responsibility in health care and privacy rights must be strongly considered, especially when investigating automated decisions (188).
Several interventions used self-reported symptoms for the personalization of the intervention. Noticeably, these mechanisms mostly used overall symptom severity. This approach disregards that symptom profiles can vary massively between patients with the same overall score (189). Some evidence points toward distinct symptom patterns being associated with different optimal treatment procedures (190). Therefore, while overall severity seems reasonable for varying guidance or communication, the sub-symptoms might be a promising ground for personalizing content and order.
The two included trials that manipulated personalization did so with small sample sizes and inconclusive results. Subsequently, one barrier to implementing personalization might be the lack of clear evidence for its benefits. However, RCTs investigating personalization are likely costly and require large sample sizes when assuming smaller effect sizes than for waitlist-controlled studies. Luckily, meta-analytic approaches allow summarizing evidence across studies, even when personalization is rarely directly manipulated. While we mentioned one such approach investigating interactions between individuals and benefits of iCBT packages (26), we consider similar approaches for other personalization mechanisms as very promising. However the identification and comparison of relevant studies in meta-analyses requires shared vocabulary and a common framework. We believe that such future work will benefit from the shared conceptual framework proposed in this article.
There are some limitations of this review that should be considered. Firstly, published studies are just one marker of what interventions are in use. While several included interventions originated in a commercial setting, those from academic settings will likely still be overrepresented in this review. Secondly, we focused on personalization within an intervention, excluding the personalization of interventions themselves. For example, past approaches investigated the data-driven personalization of therapy school (191) or the decision between medication and CBT (192). Thirdly, identifying interventions for depressive symptoms while excluding those addressing comorbid disorders, particularly anxiety, has proven challenging. One example is when anxiety was mentioned as intervention target in a cited study, but not in the original paper. While this seems understandable in light of the well-established comorbidity of depression and anxiety (193), this resulted in several edge cases of inclusion. Fourthly, we took interactivity, customization, and group-based adaptions out of the scope of this review due to their difference in nature to personalization. This should not be misunderstood as an assumed inferiority, and we call for the further investigation of these approaches to complement or even substitute personalization. Fifthly, we did not evaluate our framework by any methods besides the literature review. Approaches like expert interviews could help to determine and improve the usability of the proposed conceptualization. Sixthly, to provide a wide and less biased picture of the state of personalization, a broad search strategy was used. However, studies using more specific terminologies might be underrepresented. For example, a study on ecological momentary interventions (EMI) was not identified by our search strategy (194) as EMI was not used as a search term. Also, as pointed out by one of the reviewers, the mesh term “Telemedicine” was not used. Future approaches could therefore benefit from the application of additional techniques for iterating on the search strategy, such as the wider use of sentinel articles. Finally, as we developed our framework exclusively with studies on depressive symptoms, it remains unclear whether there are more aspects to consider with other disorders. However, we expect this framework to provide value beyond the use case of depressive symptoms and encourage future studies to investigate personalization strategies in other domains.
In conclusion, our conceptual development and empirical evaluation holistically characterizes the current use of personalization for DMHIs for depressive symptoms. A broad conceptualization of personalization reveals that most interventions incorporate personalization mechanisms. However, we conclude that we are barely scratching the surface of what is technically possible and already gold standard in other research and business areas. At the same time, we see the thin empirical ground as a barrier to implementation and call for more direct and meta-analytic evidence to delineate the benefits personalization has over an “one size fits all”-approach. Finally, as we see this question as equally pressing for other disorders, we hope for similar-minded approaches for those in the future.
A file with all included studies as well as the related coding regarding the variables of interest can be found in Appendix 3. A file with the full search strings used can be found in Appendix 2. A file containing all studies screened during the selection process is available on request.
All authors designed the review and contributed to the reviews protocol. SH and KZ performed the literature search and data extraction. All authors contributed to the analysis of results and synthesis of insights. SH and KZ wrote the initial draft of the paper. All authors reviewed the draft and contributed to the final paper. All authors contributed to the article and approved the submitted version.
The article processing charge was funded by the Deutsche Forschungsgemeinschaft (DFG, German Research Foundation) - 491192747 and the Open Access Publication Fund of Humboldt-Universität zu Berlin.
Two of the authors declare no Competing Non-Financial Interests but the following Competing Financial Interests. SH is currently employed as Data Scientist by Elona Health, a digital mental health start-up building blended mental healthcare solutions for the German market. SH worked for Meru Health, a digital mental health company developing interventions, in the past. BF is a shareholder at HelloBetter, a digital mental health company developing digital interventions, and PersonalAIze, an AI consulting company.
The remaining authors declare that the research was conducted in the absence of any commercial or financial relationships that could be construed as a potential conflict of interest.
All claims expressed in this article are solely those of the authors and do not necessarily represent those of their affiliated organizations, or those of the publisher, the editors and the reviewers. Any product that may be evaluated in this article, or claim that may be made by its manufacturer, is not guaranteed or endorsed by the publisher.
The Supplementary Material for this article can be found online at: https://www.frontiersin.org/articles/10.3389/fdgth.2023.1170002/full#supplementary-material.
1. Lim GY, Tam WW, Lu Y, Ho CS, Zhang MW, Ho RC. Prevalence of depression in the community from 30 countries between 1994 and 2014. Sci Rep. (2018) 8:2861. doi: 10.1038/s41598-018-21243-x
2. Hasin DS, Sarvet AL, Meyers JL, Saha TD, Ruan WJ, Stohl M, et al. Epidemiology of adult DSM-5 major depressive disorder and its specifiers in the United States. JAMA Psychiatry. (2018) 75:336. doi: 10.1001/jamapsychiatry.2017.4602
3. Ferrari AJ, Charlson FJ, Norman RE, Patten SB, Freedman G, Murray CJL, et al. Burden of depressive disorders by country, sex, age, and year: findings from the global burden of disease study 2010. PLOS Med. (2013) 10:e1001547. doi: 10.1371/journal.pmed.1001547
4. Wang PS, Demler O, Kessler RC. Adequacy of treatment for serious mental illness in the United States. Am J Public Health. (2002) 92:92–8. doi: 10.2105/ajph.92.1.92
5. Singer S, Engesser D, Wirp B, Lang K, Paserat A, Kobes J, et al. Effects of a statutory reform on waiting times for outpatient psychotherapy: a multicentre cohort study. Couns Psychother Res. (2022) 22:982–97. doi: 10.1002/capr.12581
6. Moroz N, Moroz I, D’Angelo MS, editors. Mental health services in Canada: barriers and cost-effective solutions to increase access. Healthc Manag Forum. (2020) 33:282–7. doi: 10.1177/0840470420933911
7. Tal A, Torous J. The digital mental health revolution: opportunities and risks. Psychiatr Rehabil J. (2017) 40:263–5. doi: 10.1037/prj0000285
8. Josephine K, Josefine L, Philipp D, David E, Harald B. Internet- and mobile-based depression interventions for people with diagnosed depression: a systematic review and meta-analysis. J Affect Disord. (2017) 223:28–40. doi: 10.1016/j.jad.2017.07.021
9. Moshe I, Terhorst Y, Philippe P, Domhardt M, Cuijpers P, Cristea I, et al. Digital interventions for the treatment of depression: a meta-analytic review. Psychol Bull. (2021) 147:749–86. doi: 10.1037/bul0000334
10. Donker T, Blankers M, Hedman E, Ljotsson B, Petrie K, Christensen H. Economic evaluations of internet interventions for mental health: a systematic review. Psychol Med. (2015) 45:3357–76. doi: 10.1017/S0033291715001427
11. International Telecommunication Union. Measuring digital development Facts and figures. ITU. (2019). https://www.itu.int/en/ITU-D/Statistics/Documents/facts/ (Accessed December 15, 2022).
12. O’Dea S. Number of smartphone users worldwide from 2016 to 2021. Statista. (2021). https://www.statista.com/statistics/330695/number-of-smartphone-usersworldwide/ (Accessed December 15, 2022).
13. Torous J, Lipschitz J, Ng M, Firth J. Dropout rates in clinical trials of smartphone apps for depressive symptoms: a systematic review and meta-analysis. J Affect Disord. (2020) 263:413–9. doi: 10.1016/j.jad.2019.11.167
14. Karyotaki E, Efthimiou O, Miguel C, Bermpohl FMG, Furukawa TA, Cuijper P, et al. Internet-based cognitive behavioral therapy for depression: a systematic review and individual patient data network meta-analysis. JAMA Psychiatry. (2021) 78:361–71. doi: 10.1001/jamapsychiatry.2020.4364
15. Kaptein M, Parvinen P. Advancing e-commerce personalization: process framework and case study. Int J Electron Commer. (2015) 19:7–33. doi: 10.1080/10864415.2015.1000216
16. Zheng L, Long M, Zhong L, Gyasi JF. The effectiveness of technology-facilitated personalized learning on learning achievements and learning perceptions: a meta-analysis. Edu Inf Tech. (2022) 27:11807–30. doi: 10.1007/s10639-022-11092-7
17. Shanahan T, Tran TP, Taylor EC. Getting to know you: social media personalization as a means of enhancing brand loyalty and perceived quality. J Retail Consum Serv. (2019) 47:57–65. doi: 10.1016/j.jretconser.2018.10.007
18. Swift JK, Callahan JL, Cooper M, Parkin SR. The impact of accommodating client preference in psychotherapy: a meta-analysis. J Clin Psychol. (2018) 74:1924–37. doi: 10.1002/jclp.22680
19. Aung MH, Matthews M, Choudhury T. Sensing behavioral symptoms of mental health and delivering personalized interventions using mobile technologies. Depress Anxiety. (2017) 34:603–9. doi: 10.1002/da.22646
20. D’Alfonso S. AI In mental health. Curr Opin Psychol. (2020) 36:112–7. doi: 10.1016/j.copsyc.2020.04.005
21. Chawla NV, Davis DA. Bringing big data to personalized healthcare: a patient-centered framework. J Gen Intern Med. (2013) 28:660–5. doi: 10.1007/s11606-013-2455-8
22. Andrews G, Williams AD. Internet psychotherapy and the future of personalized treatment. Depress Anxiety. (2014) 31:912–5. doi: 10.1002/da.22302
23. Lustria ML, Noar SM, Cortese J, Van Stee SK, Glueckauf RL, Lee J. A meta-analysis of web-delivered tailored health behavior change interventions. J Health Commun. (2013) 18(9):1039–69. doi: 10.1080/10810730.2013.768727
24. Teepe GW, Da Fonseca A, Kleim B, Jacobson NC, Salamanca Sanabria A, Tudor Car L, et al. Just-in-time adaptive mechanisms of popular mobile apps for individuals with depression: systematic app search and literature review. J Med Internet Res. (2021) 23:e2941. doi: 10.2196/29412:9
25. Moe-Byrne T, Shepherd J, Merecz-Kot D, Sinokki M, Naumanen P, Hakkaart-van Roijen L, et al. Effectiveness of tailored digital health interventions for mental health at the workplace: a systematic review of randomised controlled trials. PLOS Dig Heal. (2022) 1:e0000123. doi: 10.1371/journal.pdig.0000123
26. Furukawa TA, Suganuma A, Ostinelli EG, Andersson G, Beevers CG, Shumake J, et al. Dismantling, optimising, and personalising internet cognitive behavioural therapy for depression: a systematic review and component network meta-analysis using individual participant data. Lancet Psychiat. (2021) 8:500–11. doi: 10.1016/S2215-0366(21)00077-8
27. Ta Park VM, Ton V, Yeo G, Tiet QQ, Vuong Q, Gallagher-Thompson D. Vietnamese American dementia caregivers’ perceptions and experiences of a culturally tailored, evidence-based program to reduce stress and depression. J Gerontol Nurs. (2019) 45:39–50. doi: 10.3928/00989134-20190813-05
28. Zagorscak P, Heinrich M, Bohn J, Stein J, Knaevelsrud C. How individuals change during internet-based interventions for depression: a randomized controlled trial comparing standardized and individualized feedback. Brain Behav. (2020) 10:e01484. doi: 10.1002/brb3.1484
29. Lau Y, Chee DGH, Chow XP, Cheng LJ, Wong SN. Personalised eHealth interventions in adults with overweight and obesity: a systematic review and meta-analysis of randomised controlled trials. Prev Med. (2020) 132:106001. doi: 10.1016/j.ypmed.2020.106001
30. Captari LE, Hook JN, Hoyt W, Davis DE, McElroy-Heltzel SE, Worthington EL Jr. Integrating clients’ religion and spirituality within psychotherapy: a comprehensive meta-analysis. J Clin Psychol. (2018) 74:1938–51. doi: 10.1002/jclp.22681
31. Moher D, Liberati A, Tetzlaff J, Altman DG, PRISMA Group. Preferred reporting items for systematic reviews and meta-analyses: the PRISMA statement. PLoS Med. (2009) 6(7):e1000097. doi: 10.1371/journal.pmed.1000097
32. Himle JA, Weaver A, Zhang A, Xiang X. Digital mental health interventions for depression. Cogn Behav Pract. (2022) 29(1):50–9. doi: 10.1016/j.cbpra.2020.12.009
33. Suprenant CF, Solomon MR. Predictability and personalization in the service encounter. J Mark. (1987) 51(2):86–96. doi: 10.1177/002224298705100207
34. Deighton J, Sorrell M. The future of interactive marketing. Harv Bus Rev. (1996) 74(6):151–60. https://hbr.org/1996/11/the-future-of-interactive-marketing
35. Sundar SS, Marathe SS. Personalization versus customization: the importance of agency, privacy, and power usage. Hum Commun Res. (2010) 36(3):298–322. doi: 10.1111/j.1468-2958.2010.01377.x
36. Spanhel K, Balci S, Feldhahn F, Bengel J, Baumeister H, Sander LB. Cultural adaptation of internet-and mobile-based interventions for mental disorders: a systematic review. NPJ Digit Med. (2021) 4(1):1–18. doi: 10.1038/s41746-021-00498-1
37. Luborsky L, DeRubeis RJ. The use of psychotherapy treatment manuals: a small revolution in psychotherapy research style. Clin Psychol Rev. (1984) 4(1):5–14. doi: 10.1016/0272-7358(84)90034-5
38. Karyotaki E, Efthimiou O, Miguel C, Bermpohl FMG, Furukawa TA, Cuijpers P, et al. Internet-based cognitive behavioral therapy for depression: a systematic review and individual patient data network meta-analysis. JAMA Psychiatry. (2021) 78(4):361–71. doi: 10.1001/jamapsychiatry.2020.4364
39. Burton C, Szentagotai Tatar A, McKinstry B, Matheson C, Matu S, Moldovan R, et al. Pilot randomised controlled trial of Help4Mood, an embodied virtual agent-based system to support treatment of depression. J Telemed Telecare. (2016) 22(6):348–55. doi: 10.1177/1357633X15609793
40. Bücker L, Berger T, Bruhns A, Westermann S. Motive-oriented, personalized, internet-based interventions for depression: nonclinical experimental study. JMIR Form Res. (2022) 6(9):e37287. doi: 10.2196/37287
41. Schuster R, Leitner I, Carlbring P, Laireiter AR. Exploring blended group interventions for depression: randomised controlled feasibility study of a blended computer-and multimedia-supported psychoeducational group intervention for adults with depressive symptoms. Internet Interv. (2017) 8:63–71. doi: 10.1016/j.invent.2017.04.001
42. Six SG, Byrne KA, Aly H, Harris MW. The effect of mental health app customization on depressive symptoms in college students: randomized controlled trial. JMIR Ment Health. (2022) 9(8):e39516. doi: 10.2196/39516
43. Dahne J, Collado A, Lejuez CW, Risco CM, Diaz VA, Coles L, et al. Pilot randomized controlled trial of a Spanish-language Behavioral Activation mobile app (¡ Aptívate!) for the treatment of depressive symptoms among United States Latinx adults with limited English proficiency. J Affect Disord. (2019) 250:210–7. doi: 10.1016/j.jad.2019.03.009
44. Dahne J, Lejuez CW, Diaz VA, Player MS, Kustanowitz J, Felton JW, et al. Pilot randomized trial of a self-help behavioral activation mobile app for utilization in primary care. Behav Ther. (2019) 50(4):817–27. doi: 10.1016/j.beth.2018.12.003
45. Pérez JC, Fernández O, Cáceres C, Carrasco ÁE, Moessner M, Bauer S, et al. An adjunctive internet-based intervention to enhance treatment for depression in adults: randomized controlled trial. JMIR Ment Health. (2021) 8(12):e26814. doi: 10.2196/26814
46. Lüdtke T, Pult LK, Schröder J, Moritz S, Bücker L. A randomized controlled trial on a smartphone self-help application (be good to yourself) to reduce depressive symptoms. Psychiatry Res. (2018) 269:753–62. doi: 10.1016/j.psychres.2018.08.113
47. Forand NR, Barnett JG, Strunk DR, Hindiyeh MU, Feinberg JE, Keefe JR. Efficacy of guided iCBT for depression and mediation of change by cognitive skill acquisition. Behav Ther. (2018) 49(2):295–307. doi: 10.1016/j.beth.2017.04.004
48. Pfeiffer PN, Pope B, Houck M, Benn-Burton W, Zivin K, Ganoczy D, et al. Effectiveness of peer-supported computer-based CBT for depression among veterans in primary care. Psychiatr Serv. (2020) 71(3):256–62. doi: 10.1176/appi.ps.201900283
49. Gupta SK, Slaven JE, Liu Z, Polanka BM, Freiberg MS, Stewart JC. Effects of internet cognitive-behavioral therapy on depressive symptoms and surrogates of cardiovascular risk in human immunodeficiency virus: a pilot, randomized, controlled trial. Open Forum Infect Dis. (2020) 7(7):ofaa280. doi: 10.1093/ofid/ofaa280
50. Xiang X, Kayser J, Sun Y, Himle J. Internet-based psychotherapy intervention for depression among older adults receiving home care: qualitative study of participants’ experiences. JMIR Aging. (2021) 4(4):e27630. doi: 10.2196/27630
51. Littlewood E, Duarte A, Hewitt C, Knowles S, Palmer S, Walker S, et al. A randomised controlled trial of computerised cognitive behaviour therapy for the treatment of depression in primary care: the randomised evaluation of the effectiveness and acceptability of computerised therapy (REEACT) trial. Health Technol Assess. (2015) 19(101):viii–171. doi: 10.3310/hta191010
52. Fuller-Tyszkiewicz M, Richardson B, Klein B, Skouteris H, Christensen H, Austin D. A mobile app-based intervention for depression: end-user and expert usability testing study. JMIR Ment Health. (2018) 5(3):e54. doi: 10.2196/mental.9445
53. Stiles-Shields C, Montague E, Kwasny MJ, Mohr DC. Behavioral and cognitive intervention strategies delivered via coached apps for depression: pilot trial. Psychol Serv. (2019) 16(2):233–38. doi: 10.1037/ser0000261
54. Blackwell SE, Browning M, Mathews A, Pictet A, Welch J, Davies J, et al. Positive imagery-based cognitive bias modification as a web-based treatment tool for depressed adults: a randomized controlled trial. Clin Psychol Sci. (2015) 3(1):91–111. doi: 10.1177/2167702614560746
55. Williams AD, O’Moore K, Blackwell SE, Smith J, Holmes EA, Andrews G. Positive imagery cognitive bias modification (CBM) and internet-based cognitive behavioral therapy (iCBT): a randomized controlled trial. J Affect Disord. (2015) 178:131–41. doi: 10.1016/j.jad.2015.02.026
56. Pictet A, Jermann F, Ceschi G. When less could be more: investigating the effects of a brief internet-based imagery cognitive bias modification intervention in depression. Behav Res Ther. (2016) 84:45–51. doi: 10.1016/j.brat.2016.07.008
57. Callan JA, Dunbar Jacob J, Siegle G, Dey A, Thase M, DeVito Dabbs A, et al. CBT Mobilework©: user-centered development and testing of a mobile mental health application for depression. Cogn Ther Res. (2021) 45:287–302. doi: 10.1007/s10608-020-10159-4
58. Ritvo P, Knyahnytska Y, Pirbaglou M, Wang W, Tomlinson G, Zhao H, et al. Online mindfulness-based cognitive behavioral therapy intervention for youth with major depressive disorders: randomized controlled trial. J Med Internet Res. (2021) 23(3):e24380. doi: 10.2196/24380
59. Eriksson MCM, Kivi M, Hange D, Petersson EL, Ariai N, Häggblad P, et al. Long-term effects of internet-delivered cognitive behavioral therapy for depression in primary care–the PRIM-NET controlled trial. Scand J Prim Health Care. (2017) 35(2):126–36. doi: 10.1080/02813432.2017.1333299
60. Beevers CG, Pearson R, Hoffman JS, Foulser AA, Shumake J, Meyer B. Effectiveness of an internet intervention (Deprexis) for depression in a United States adult sample: a parallel-group pragmatic randomized controlled trial. J Consult Clin Psychol. (2017) 85(4):367–80. doi: 10.1037/ccp0000171
61. Zwerenz R, Becker J, Knickenberg RJ, Siepmann M, Hagen K, Beutel ME. Online self-help as an add-on to inpatient psychotherapy: efficacy of a new blended treatment approach. Psychother Psychosom. (2017) 86(6):341–50. doi: 10.1159/000481177
62. Richter LE, Machleit-Ebner A, Scherbaum N, Bonnet U. How effective is a web-based mental health intervention (Deprexis) in the treatment of moderate and major depressive disorders when started during routine psychiatric inpatient treatment as an adjunct therapy? A pragmatic parallel-group randomized controlled trial. Fortschr Neurol Psychiatr. (2022) 04:10.1055/a-1826-2888. doi: 10.1055/a-1826-2888
63. Klein JP, Berger T, Schröder J, Späth C, Meyer B, Caspar F, et al. Effects of a psychological internet intervention in the treatment of mild to moderate depressive symptoms: results of the EVIDENT study, a randomized controlled trial. Psychother Psychosom. (2016) 85(4):218–28. doi: 10.1159/000445355
64. Gräfe V, Moritz S, Greiner W. Health economic evaluation of an internet intervention for depression (deprexis), a randomized controlled trial. Health Econ Rev. (2020) 10(1):19. doi: 10.1186/s13561-020-00273-0
65. Meyer B, Bierbrodt J, Schröder J, Berger T, Beevers CG, Weiss M, et al. Effects of an internet intervention (Deprexis) on severe depression symptoms: randomized controlled trial. Internet Interv. (2015) 2(1):48–59. doi: 10.1016/j.invent.2014.12.003
66. Fischer A, Schröder J, Vettorazzi E, Wolf OT, Pöttgen J, Lau S, et al. An online programme to reduce depression in patients with multiple sclerosis: a randomised controlled trial. Lancet Psychiatry. (2015) 2(3):217–23. doi: 10.1016/S2215-0366(14)00049-2
67. Crisp DA, Griffiths KM. Reducing depression through an online intervention: benefits from a user perspective. JMIR Ment Health. (2016) 3(1):e4. doi: 10.2196/mental.4356
68. Iacoviello BM, Murrough JW, Hoch MM, Huryk KM, Collins KA, Cutter GR, et al. A randomized, controlled pilot trial of the emotional faces memory task: a digital therapeutic for depression. NPJ Digit Med. (2018) 1:21. doi: 10.1038/s41746-018-0025-5
69. Hoch MM, Doucet GE, Moser DA, Hee Lee W, Collins KA, Huryk KM, et al. Initial evidence for brain plasticity following a digital therapeutic intervention for depression. Chronic Stress. (2019) 3:2470547019877880. doi: 10.1177/2470547019877880
70. Hung GC, Yang PC, Wang CY, Chiang JH. A smartphone-based personalized activity recommender system for patients with depression. In: Proceedings of the 5th EAI international conference on wireless mobile communication and healthcare; 2015 Oct 14–16; London, Great Britain. Belgium: Institute for Computer Sciences, Social-Informatics and Telecommunications Engineering (2015). p. 253–7. doi: 10.4108/eai.14-10-2015.2261655
71. Pinto MD, Greenblatt AM, Hickman RL, Rice HM, Thomas TL, Clochesy JM. Assessing the critical parameters of eSMART-MH: a promising avatar-based digital therapeutic intervention to reduce depressive symptoms. Perspect Psychiatr Care. (2016) 52(3):157–68. doi: 10.1111/ppc.12112
72. Arjadi R, Nauta MH, Scholte WF, Hollon SD, Chowdhary N, Suryani AO, et al. Internet-based behavioural activation with lay counsellor support versus online minimal psychoeducation without support for treatment of depression: a randomised controlled trial in Indonesia. Lancet Psychiatry. (2018) 5(9):707–16. doi: 10.1016/S2215-0366(18)30223-2
73. Buntrock C, Ebert D, Lehr D, Riper H, Smit F, Cuijpers P, et al. Effectiveness of a web-based cognitive behavioural intervention for subthreshold depression: pragmatic randomised controlled trial. Psychother Psychosom. (2015) 84(6):348–58. doi: 10.1159/000438673
74. Reins JA, Boß L, Lehr D, Berking M, Ebert DD. The more I got, the less I need? Efficacy of internet-based guided self-help compared to online psychoeducation for major depressive disorder. J Affect Disord. (2019) 246:695–705. doi: 10.1016/j.jad.2018.12.065
75. Ebert DD, Buntrock C, Lehr D, Smit F, Riper H, Baumeister H, et al. Effectiveness of web-and mobile-based treatment of subthreshold depression with adherence-focused guidance: a single-blind randomized controlled trial. Behav Ther. (2018) 49(1):71–83. doi: 10.1016/j.beth.2017.05.004
76. Braun L, Titzler I, Terhorst Y, Freund J, Thielecke J, Ebert DD, et al. Are guided internet-based interventions for the indicated prevention of depression in green professions effective in the long run? Longitudinal analysis of the 6-and 12-month follow-up of a pragmatic randomized controlled trial (PROD-A). Internet Interv. (2021) 26:100455. doi: 10.1016/j.invent.2021.100455
77. Ofoegbu TO, Asogwa U, Otu MS, Ibenegbu C, Muhammed A, Eze B. Efficacy of guided internet-assisted intervention on depression reduction among educational technology students of Nigerian universities. Medicine (Baltimore). (2020) 99(6):e18774. doi: 10.1097/MD.0000000000018774
78. Thase ME, Wright JH, Eells TD, Barrett MS, Wisniewski SR, Balasubramani GK, et al. Improving the efficiency of psychotherapy for depression: computer-assisted versus standard CBT. Am J Psychiatry. (2018) 175(3):242–50. doi: 10.1176/appi.ajp.2017.17010089
79. Wright JH, Owen J, Eells TD, Antle B, Bishop LB, Girdler R, et al. Effect of computer-assisted cognitive behavior therapy vs usual care on depression among adults in primary care: a randomized clinical trial. JAMA Netw Open. (2022) 5(2):e2146716. doi: 10.1001/jamanetworkopen.2021.46716
80. Deady M, Johnston D, Milne D, Glozier N, Peters D, Calvo R, et al. Preliminary effectiveness of a smartphone app to reduce depressive symptoms in the workplace: feasibility and acceptability study. JMIR Mhealth Uhealth. (2018) 6(12):e11661. doi: 10.2196/11661
81. Strauss C, Dunkeld C, Cavanagh K. Is clinician-supported use of a mindfulness smartphone app a feasible treatment for depression? A mixed-methods feasibility study. Internet Interv. (2021) 25:100413. doi: 10.1016/j.invent.2021.100413
82. Fish MT, Saul AD. The gamification of meditation: a randomized-controlled study of a prescribed mobile mindfulness meditation application in reducing college students’ depression. Simul Gaming. (2019) 50(4):419–35. doi: 10.1177/1046878119851821
83. Ying Y, Ji Y, Kong F, Wang M, Chen Q, Wang L, et al. Efficacy of an internet-based cognitive behavioral therapy for subthreshold depression among Chinese adults: a randomized controlled trial. Psychol Med. (2022):1–11. doi: 10.1017/S0033291722000599
84. Beiwinkel T, Eißing T, Telle NT, Siegmund-Schultze E, Rössler W. Effectiveness of a web-based intervention in reducing depression and sickness absence: randomized controlled trial. J Med Internet Res. (2017) 19(6):e6546. doi: 10.2196/jmir.6546
85. Bur OT, Krieger T, Moritz S, Klein JP, Berger T. Optimizing the context of support of web-based self-help in individuals with mild to moderate depressive symptoms: a randomized full factorial trial. Behav Res Ther. (2022) 152:104070. doi: 10.1016/j.brat.2022.104070
86. Gili M, Castro A, García-Palacios A, Garcia-Campayo J, Mayoral-Cleries F, Botella C, et al. Efficacy of three low-intensity, internet-based psychological interventions for the treatment of depression in primary care: randomized controlled trial. J Med Internet Res. (2020) 22(6):e15845. doi: 10.2196/15845
87. Zhao C, Wampold BE, Ren Z, Zhang L, Jiang G. The efficacy and optimal matching of an internet-based acceptance and commitment therapy intervention for depressive symptoms among university students: a randomized controlled trial in China. J Clin Psychol. (2022) 78(7):1354–375. doi: 10.1002/jclp.23329
88. Oehler C, Scholze K, Driessen P, Rummel-Kluge C, Görges F, Hegerl U. How are guide profession and routine care setting related to adherence and symptom change in iCBT for depression?—an explorative log-data analysis. Internet Interv. (2021) 26:100476. doi: 10.1016/j.invent.2021.100476
89. Varga A, Czeglédi E, Tóth MD, Purebl G. Effectiveness of iFightDepression® online guided self-help tool in depression–a pilot study. J Telemed Telecare. (2022) 0(0):1357633X221084584. doi: 10.1177/1357633X221084584
90. Schuster R, Fischer E, Jansen C, Napravnik N, Rockinger S, Steger N, et al. Blending internet-based and tele group treatment: acceptability, effects, and mechanisms of change of cognitive behavioral treatment for depression. Internet Interv. (2022) 29:100551. doi: 10.1016/j.invent.2022.100551
91. Oehler C, Scholze K, Reich H, Sander C, Hegerl U. Intervention use and symptom change with unguided internet-based cognitive behavioral therapy for depression during the COVID-19 pandemic: log data analysis of a convenience sample. JMIR Ment Health. (2021) 8(7):e28321. doi: 10.2196/28321
92. Paganini S, Terhorst Y, Sander LB, Lin J, Schlicker S, Ebert DD, et al. Internet-and mobile-based intervention for depression in adults with chronic back pain: a health economic evaluation. J Affect Disord. (2022) 308:607–15. doi: 10.1016/j.jad.2022.04.004
93. Everitt N, Broadbent J, Richardson B, Smyth JM, Heron K, Teague S, et al. Exploring the features of an app-based just-in-time intervention for depression. J Affect Disord. (2021) 291:279–87. doi: 10.1016/j.jad.2021.05.021
94. El Alaoui S, Ljótsson B, Hedman E, Svanborg C, Kaldo V, Lindefors N. Predicting outcome in internet-based cognitive behaviour therapy for major depression: a large cohort study of adult patients in routine psychiatric care. PLoS One. (2016) 11(9):e0161191. doi: 10.1371/journal.pone.0161191
95. Anguera JA, Jordan JT, Castaneda D, Gazzaley A, Areán PA. Conducting a fully mobile and randomised clinical trial for depression: access, engagement and expense. BMJ Innov. (2016) 2(1):14–21. doi: 10.1136/bmjinnov-2015-000098
96. Pratap A, Renn BN, Volponi J, Mooney SD, Gazzaley A, Arean PA, et al. Using mobile apps to assess and treat depression in Hispanic and Latino populations: fully remote randomized clinical trial. J Med Internet Res. (2018) 20(8):e10130. doi: 10.2196/10130
97. Mol M, Dozeman E, Provoost S, van Schaik A, Riper H, Smit JH. Behind the scenes of online therapeutic feedback in blended therapy for depression: mixed-methods observational study. J Med Internet Res. (2018) 20(5):e174. doi: 10.2196/jmir.9890
98. Noguchi R, Sekizawa Y, So M, Yamaguchi S, Shimizu E. Effects of five-minute internet-based cognitive behavioral therapy and simplified emotion-focused mindfulness on depressive symptoms: a randomized controlled trial. BMC Psychiatry. (2017) 17(1):1–14. doi: 10.1186/s12888-017-1248-8
99. Sweet AM, Pearlstein SL, Paulus MP, Stein MB, Taylor CT. Computer-delivered behavioural activation and approach-avoidance training in major depression: proof of concept and initial outcomes. Br J Clin Psychol. (2021) 60(3):357–74. doi: 10.1111/bjc.12287
100. Murillo LA, Follo E, Smith A, Balestrier J, Bevvino DL. Evaluating the effectiveness of online educational modules and interactive workshops in alleviating symptoms of mild to moderate depression: a pilot trial. J Prim Care Community Health. (2020) 11:2150132720971158. doi: 10.1177/2150132720971158
101. Kingston J, Becker L, Woeginger J, Ellett LA. Randomised trial comparing a brief online delivery of mindfulness-plus-values versus values only for symptoms of depression: does baseline severity matter? J Affect Disord. (2020) 276:936–44. doi: 10.1016/j.jad.2020.07.087
102. Lüdtke T, Westermann S, Pult LK, Schneider BC, Pfuhl G, Moritz S. Evaluation of a brief unguided psychological online intervention for depression: a controlled trial including exploratory moderator analyses. Internet Interv. (2018) 13:73–81. doi: 10.1016/j.invent.2018.06.004
103. Ly KH, Topooco N, Cederlund H, Wallin A, Bergström J, Molander O, et al. Smartphone-supported versus full behavioural activation for depression: a randomised controlled trial. PLoS One. (2015) 10(5):e0126559. doi: 10.1371/journal.pone.0126559
104. Kenter RM, Cuijpers P, Beekman A, van Straten A. Effectiveness of a web-based guided self-help intervention for outpatients with a depressive disorder: short-term results from a randomized controlled trial. J Med Internet Res. (2016) 18(3):e4861. doi: 10.2196/jmir.4861
105. Nyström MB, Stenling A, Sjöström E, Neely G, Lindner P, Hassmén P, et al. Behavioral activation versus physical activity via the internet: a randomized controlled trial. J Affect Disord. (2017) 215:85–93. doi: 10.1016/j.jad.2017.03.018
106. Johansson O, Bjärehed J, Andersson G, Carlbring P, Lundh LG. Effectiveness of guided internet-delivered cognitive behavior therapy for depression in routine psychiatry: a randomized controlled trial. Internet Interv. (2019) 17:100247. doi: 10.1016/j.invent.2019.100247
107. Nordgreen T, Blom K, Andersson G, Carlbring P, Havik OE. Effectiveness of guided internet-delivered treatment for major depression in routine mental healthcare—an open study. Internet Interv. (2019) 18:100274. doi: 10.1016/j.invent.2019.100274
108. Jakobsen H, Andersson G, Havik OE, Nordgreen T. Guided internet-based cognitive behavioral therapy for mild and moderate depression: a benchmarking study. Internet Interv. (2017) 7:1–8. doi: 10.1016/j.invent.2016.11.002
109. Nygren T, Brohede D, Koshnaw K, Osman SS, Johansson R, Andersson G. Internet-based treatment of depressive symptoms in a Kurdish population: a randomized controlled trial. J Clin Psychol. (2019) 75(6):985–98. doi: 10.1002/jclp.22753
110. Tulbure BT, Andersson G, Sălăgean N, Pearce M, Koenig HG. Religious versus conventional internet-based cognitive behavioral therapy for depression. J Relig Health. (2018) 57(5):1634–648. doi: 10.1007/s10943-017-0503-0
111. Hallgren M, Kraepelien M, Öjehagen A, Lindefors N, Zeebari Z, Kaldo V, et al. Physical exercise and internet-based cognitive–behavioural therapy in the treatment of depression: randomised controlled trial. Br J Psychiatry. (2015) 207(3):227–34. doi: 10.1192/bjp.bp.114.160101
112. Wong VWH, Ho FY, Shi NK, Tong JT, Chung KF, Yeung WF, et al. Smartphone-delivered multicomponent lifestyle medicine intervention for depressive symptoms: a randomized controlled trial. J Consult Clin Psychol. (2021) 89(12):970–84. doi: 10.1037/ccp0000695
113. Salisbury C, O’Cathain A, Edwards L, Thomas C, Gaunt D, Hollinghurst S, et al. Effectiveness of an integrated telehealth service for patients with depression: a pragmatic randomised controlled trial of a complex intervention. Lancet Psychiatry. (2016) 3(6):515–25. doi: 10.1016/S2215-0366(16)00083-3
114. Pots WT, Fledderus M, Meulenbeek PA, ten Klooster PM, Schreurs KM, Bohlmeijer ET. Acceptance and commitment therapy as a web-based intervention for depressive symptoms: randomised controlled trial. Br J Psychiatry. (2016) 208(1):69–77. doi: 10.1192/bjp.bp.114.146068
115. Kelders SM, Bohlmeijer ET, Pots WT, van Gemert-Pijnen JE. Comparing human and automated support for depression: fractional factorial randomized controlled trial. Behav Res Ther. (2015) 72:72–80. doi: 10.1016/j.brat.2015.06.014
116. Rauen K, Vetter S, Eisele A, Biskup E, Delsignore A, Rufer M, et al. Internet cognitive behavioral therapy with or without face-to-face psychotherapy: a 12-weeks clinical trial of patients with depression. Front Digit Health. (2020) 2:4. doi: 10.3389/fdgth.2020.00004
117. Addington EL, Cheung EO, Bassett SM, Kwok I, Schuette SA, Shiu E, et al. The MARIGOLD study: feasibility and enhancement of an online intervention to improve emotion regulation in people with elevated depressive symptoms. J Affect Disord. (2019) 257:352–64. doi: 10.1016/j.jad.2019.07.049
118. Moskowitz JT, Addington EL, Shiu E, Bassett SM, Schuette S, Kwok I, et al. Facilitator contact, discussion boards, and virtual badges as adherence enhancements to a web-based, self-guided, positive psychological intervention for depression: randomized controlled trial. J Med Internet Res. (2021) 23(9):e25922. doi: 10.2196/25922
119. Visser DA, Tendolkar I, Schene AH, van de Kraats L, Ruhe HG, Vrijsen JN, et al. A pilot study of smartphone-based memory bias modification and its effect on memory bias and depressive symptoms in an unselected population. Cognit Ther Res. (2020) 44(1):61–72. doi: 10.1007/s10608-019-10042-x
120. Bruhns A, Lüdtke T, Moritz S, Bücker L. A mobile-based intervention to increase self-esteem in students with depressive symptoms: randomized controlled trial. JMIR Mhealth Uhealth. (2021) 9(7):e26498. doi: 10.2196/26498
121. Lukas CA, Eskofier B, Berking M. A gamified smartphone-based intervention for depression: randomized controlled pilot trial. JMIR Ment Health. (2021) 8(7):e16643. doi: 10.2196/16643
122. Lukas CA, Berking M. Blending group-based psychoeducation with a smartphone intervention for the reduction of depressive symptoms: results of a randomized controlled pilot study. Pilot Feasibility Stud. (2021) 7(1):1–8. doi: 10.1186/s40814-021-00799-y
123. Raevuori A, Vahlberg T, Korhonen T, Hilgert O, Aittakumpu-Hyden R, Forman-Hoffman V. A therapist-guided smartphone app for major depression in young adults: a randomized clinical trial. J Affect Disord. (2021) 286:228–38. doi: 10.1016/j.jad.2021.02.007
124. Economides M, Lehrer P, Ranta K, Nazander A, Hilgert O, Raevuori A, et al. Feasibility and efficacy of the addition of heart rate variability biofeedback to a remote digital health intervention for depression. Appl Psychophysiol Biofeedback. (2020) 45(2):75–86. doi: 10.1007/s10484-020-09458-z
125. Forman-Hoffman VL, Nelson BW, Ranta K, Nazander A, Hilgert O, de Quevedo J. Significant reduction in depressive symptoms among patients with moderately-severe to severe depressive symptoms after participation in a therapist-supported, evidence-based mobile health program delivered via a smartphone app. Internet Interv. (2021) 25:100408. doi: 10.1016/j.invent.2021.100408
126. Bücker L, Schnakenberg P, Karyotaki E, Moritz S, Westermann S. Diminishing effects after recurrent use of self-guided internet-based interventions in depression: randomized controlled trial. J Med Internet Res. (2019) 21(10):e14240. doi: 10.2196/14240
127. Titzler I, Egle V, Berking M, Gumbmann C, Ebert DD. Blended psychotherapy: treatment concept and case report for the integration of internet-and mobile-based interventions into brief psychotherapy of depressive disorders. Verhaltenstherapie. (2019) 32(1):1–15. doi: 10.1159/000503408
128. Wang F, Feng F, Zhang J, Cooper A, Hong L, Wang W, et al. Outcomes of an online computerized cognitive behavioral treatment program for treating Chinese patients with depression: a pilot study. Asian J Psychiatr. (2018) 38:102–7. doi: 10.1016/j.ajp.2017.11.007
129. Brabyn S, Araya R, Barkham M, Bower P, Cooper C, Duarte A, et al. The second randomised evaluation of the effectiveness, cost-effectiveness and acceptability of computerised therapy (REEACT-2) trial: does the provision of telephone support enhance the effectiveness of computer-delivered cognitive behaviour therapy? A randomised controlled trial. Health Technol Assess. (2016) 20(89):1–64. doi: 10.3310/hta20890
130. Silverstone PH, Rittenbach K, Suen VY, Moretzsohn A, Cribben I, Bercov M, et al. Depression outcomes in adults attending family practice were not improved by screening, stepped-care, or online CBT during a 12-week study when compared to controls in a randomized trial. Front Psychiatry. (2017) 8:32. doi: 10.3389/fpsyt.2017.00032
131. McDermott R, Dozois DJ. A randomized controlled trial of internet-delivered CBT and attention bias modification for early intervention of depression. J Exp Psychopathol. (2019) 10(2):2043808719842502. doi: 10.1177/2043808719842502
132. Gilbody S, Littlewood E, Hewitt C, Brierley G, Tharmanathan P, Araya R, et al. Computerised cognitive behaviour therapy (cCBT) as treatment for depression in primary care (REEACT trial): large scale pragmatic randomised controlled trial. Br Med J. (2015) 351:i195. doi: 10.1136/bmj.h5627
133. Löbner M, Pabst A, Stein J, Dorow M, Matschinger H, Luppa M, et al. Computerized cognitive behavior therapy for patients with mild to moderately severe depression in primary care: a pragmatic cluster randomized controlled trial (@ktiv). J Affect Disord. (2018) 238:317–26. doi: 10.1016/j.jad.2018.06.008
134. Birney AJ, Gunn R, Russell JK, Ary DV. Moodhacker mobile web app with email for adults to self-manage mild-to-moderate depression: randomized controlled trial. JMIR Mhealth Uhealth. (2016) 4(1):e8. doi: 10.2196/mhealth.4231
135. Rohani DA, Tuxen N, Lopategui AQ, Faurholt-Jepsen M, Kessing LV, Bardram JE. Personalizing mental health: a feasibility study of a mobile behavioral activation tool for depressed patients. Proceedings of the 13th EAI international conference on pervasive computing technologies for healthcare (PervasiveHealth'19). p. 282–91. doi: 10.1145/3329189.3329214
136. Wahle F, Kowatsch T, Fleisch E, Rufer M, Weidt S. Mobile sensing and support for people with depression: a pilot trial in the wild. JMIR Mhealth Uhealth. (2016) 4(3):e111. doi: 10.2196/mhealth.5960
137. Rohani DA, Quemada Lopategui A, Tuxen N, Faurholt-Jepsen M, Kessing LV, Bardram JE. MUBS: a personalized recommender system for behavioral activation in mental health. Proceedings of the 2020 CHI conference on human factors in computing systems (CHI ‘20). p. 1–13. doi: 10.1145/3313831.3376879
138. Young CL, Mohebbi M, Staudacher HM, Kay-Lambkin F, Berk M, Jacka FN, et al. Optimizing engagement in an online dietary intervention for depression (my food & mood version 3.0): cohort study. JMIR Ment Health. (2021) 8(3):e24871. doi: 10.2196/24871
139. Hirsch A, Luellen J, Holder JM, Steinberg G, Dubiel T, Blazejowskyj A, et al. Managing depressive symptoms in the workplace using a web-based self-care tool: a pilot randomized controlled trial. JMIR Res Protoc. (2017) 6(4):e51. doi: 10.2196/resprot.7203
140. Stawarz K, Preist C, Tallon D, Thomas L, Turner K, Wiles N, et al.. Integrating the digital and the traditional to deliver therapy for depression: lessons from a pragmatic study. In Proceedings of the 2020 CHI conference on human factors in computing systems (CHI ‘20). pp. 1–14. doi: 10.1145/3313831.3376510
141. Schlosser DA, Campellone TR, Truong B, Anguera JA, Vergani S, Vinogradov S, et al. The feasibility, acceptability, and outcomes of PRIME-D: a novel mobile intervention treatment for depression. Depress Anxiety. (2017) 34(6):546–54. doi: 10.1002/da.22624
142. Mehrotra S, Sudhir P, Rao G, Thirthalli J, Srikanth TK. Development and pilot testing of an internet-based self-help intervention for depression for Indian users. Behav Sci. (2018) 8(4):36. doi: 10.3390/bs8040036
143. Sakata M, Toyomoto R, Yoshida K, Luo Y, Nakagami Y, Uwatoko T, et al. Components of smartphone cognitive-behavioural therapy for subthreshold depression among 1093 university students: a factorial trial. Evid Based Ment Health. (2022) 25(e1):e18–e25. doi: 10.1136/ebmental-2022-300455
144. Jelinek L, Arlt S, Moritz S, Schröder J, Westermann S, Cludius B. Brief web-based intervention for depression: randomized controlled trial on behavioral activation. J Med Internet Res. (2020) 22(3):e15312. doi: 10.2196/15312
145. Haller N, Lorenz S, Pfirrmann D, Koch C, Lieb K, Dettweiler U, et al. Individualized web-based exercise for the treatment of depression: randomized controlled trial. JMIR Ment Health. (2018) 5(4):e10698. doi: 10.2196/10698
146. Zagorscak P, Heinrich M, Sommer D, Wagner B, Knaevelsrud C. Benefits of individualized feedback in internet-based interventions for depression: a randomized controlled trial. Psychother Psychosom. (2018) 87(1):32–45. doi: 10.1159/000481515
147. Mayer G, Hummel S, Oetjen N, Gronewold N, Bubolz S, Blankenhagel K, et al. User experience and acceptance of patients and healthy adults testing a personalized self-management app for depression: a non-randomized mixed-methods feasibility study. Digit Health. (2022) 8:20552076221091353. doi: 10.1177/20552076221091353
148. Montero-Marín J, Araya R, Pérez-Yus MC, Mayoral F, Gili M, Botella C, et al. An internet-based intervention for depression in primary care in Spain: a randomized controlled trial. J Med Internet Res. (2016) 18(8):e5695. doi: 10.2196/jmir.5695
149. Mira A, Bretón-López J, García-Palacios A, Quero S, Baños RM, Botella C. An internet-based program for depressive symptoms using human and automated support: a randomized controlled trial. Neuropsychiatr Dis Treat. (2017) 13:987–1006. doi: 10.2147/NDT.S130994
150. Richards D, Timulak L, O’Brien E, Hayes C, Vigano N, Sharry J, et al. A randomized controlled trial of an internet-delivered treatment: its potential as a low-intensity community intervention for adults with symptoms of depression. Behav Res Ther. (2015) 75:20–31. doi: 10.1016/j.brat.2015.10.005
151. Richards D, Murphy T, Viganó N, Timulak L, Doherty G, Sharry J, et al. Acceptability, satisfaction and perceived efficacy of “space from depression” an internet-delivered treatment for depression. Internet Interv. (2016) 5:12–22. doi: 10.1016/j.invent.2016.06.007
152. Salamanca-Sanabria A, Richards D, Timulak L, Connell S, Mojica Perilla M, Parra-Villa Y, et al. A culturally adapted cognitive behavioral internet-delivered intervention for depressive symptoms: randomized controlled trial. JMIR Ment Health. (2020) 7(1):e13392. doi: 10.2196/13392
153. Salamanca-Sanabria A, Richards D, Timulak L. Adapting an internet-delivered intervention for depression for a Colombian college student population: an illustration of an integrative empirical approach. Internet Interv. (2019) 15:76–86. doi: 10.1016/j.invent.2018.11.005
154. Kageyama K, Kato Y, Mesaki T, Uchida H, Takahashi K, Marume R, et al. Effects of video viewing smartphone application intervention involving positive word stimulation in people with subthreshold depression: a pilot randomized controlled trial. J Affect Disord. (2021) 282:74–81. doi: 10.1016/j.jad.2020.12.104
155. Takahashi K, Takada K, Hirao K. Feasibility and preliminary efficacy of a smartphone application intervention for subthreshold depression. Early Interv Psychiatry. (2019) 13(1):133–6. doi: 10.1111/eip.12540
156. Cuijpers P, Heim E, Abi Ramia J, Burchert S, Carswell K, Cornelisz I, et al. Effects of a WHO-guided digital health intervention for depression in Syrian refugees in Lebanon: a randomized controlled trial. PLoS Med. (2022) 19(6):e1004025. doi: 10.1371/journal.pmed.1004025
157. Cuijpers P, Heim E, Ramia JA, Burchert S, Carswell K, Cornelisz I, et al. Guided digital health intervention for depression in Lebanon: randomised trial. Evid Based Ment Health. (2022) 25(e1):e34–e40. doi: 10.1136/ebmental-2021-300416
158. Carswell K, Harper-Shehadeh M, Watts S, Van’t Hof E, Abi Ramia J, Heim E, et al. Step-by-Step: a new WHO digital mental health intervention for depression. Mhealth. (2018) 4:34. doi: 10.21037/mhealth.2018.08.01
159. Heim E, Ramia JA, Hana RA, Burchert S, Carswell K, Cornelisz I, et al. Step-by-step: feasibility randomised controlled trial of a mobile-based intervention for depression among populations affected by adversity in Lebanon. Internet Interv. (2021) 24:100380. doi: 10.1016/j.invent.2021.100380
160. Piera-Jiménez J, Etzelmueller A, Kolovos S, Folkvord F, Lupiáñez-Villanueva F. Guided internet-based cognitive behavioral therapy for depression: implementation cost-effectiveness study. J Med Internet Res. (2021) 23(5):e27410. doi: 10.2196/27410
161. Roepke AM, Jaffee SR, Riffle OM, McGonigal J, Broome R, Maxwell B. Randomized controlled trial of SuperBetter, a smartphone-based/internet-based self-help tool to reduce depressive symptoms. Games Health J. (2015) 4(3):235–46. doi: 10.1089/g4h.2014.0046
162. Hatcher S, Whittaker R, Patton M, Miles WS, Ralph N, Kercher K, et al. Web-based therapy plus support by a coach in depressed patients referred to secondary mental health care: randomized controlled trial. JMIR Ment Health. (2018) 5(1):e5. doi: 10.2196/mental.8510
163. Titov N, Dear BF, Staples LG, Terides MD, Karin E, Sheehan J, et al. Disorder-specific versus transdiagnostic and clinician-guided versus self-guided treatment for major depressive disorder and comorbid anxiety disorders: a randomized controlled trial. J Anxiety Disord. (2015) 35:88–102. doi: 10.1016/j.janxdis.2015.08.002
164. Rosso I, Killgore WD, Olson EA, Webb CA, Fukunaga R, Auerbach RPM, et al. Internet-based cognitive behavior therapy for major depressive disorder: a randomized controlled trial. Depress Anxiety. (2017) 34(3):236–45. doi: 10.1002/da.22590
165. Smith J, Newby JM, Burston N, Murphy MJ, Michael S, Mackenzie A, et al. Help from home for depression: a randomised controlled trial comparing internet-delivered cognitive behaviour therapy with bibliotherapy for depression. Internet Interv. (2017) 9:25–37. doi: 10.1016/j.invent.2017.05.001
166. O’moore KA, Newby JM, Andrews G, Hunter DJ, Bennell K, Smith J, et al. Internet cognitive–behavioral therapy for depression in older adults with knee osteoarthritis: a randomized controlled trial. Arthritis Care Res (Hoboken). (2018) 70(1):61–70. doi: 10.1002/acr.23257
167. Mewton L, Andrews G. Cognitive behaviour therapy via the internet for depression: a useful strategy to reduce suicidal ideation. J Affect Disord. (2015) 170:78–84. doi: 10.1016/j.jad.2014.08.038
168. Mohr DC, Lattie EG, Tomasino KN, Kwasny MJ, Kaiser SM, Gray EL, et al. A randomized noninferiority trial evaluating remotely-delivered stepped care for depression using internet cognitive behavioral therapy (CBT) and telephone CBT .Behav Res Ther. (2019) 123:103485. doi: 10.1016/j.brat.2019.103485
169. Schueller SM, Mohr DC. Initial field trial of a coach-supported web-based depression treatment. Proceedings of the 9th international conference on pervasive computing technologies for healthcare (PervasiveHealth ‘15) (2015). p. 25–8
170. Stiles-Shields C, Montague E, Lattie EG, Schueller SM, Kwasny MJ, Mohr DC. Exploring user learnability and learning performance in an app for depression: usability study. JMIR Hum Factors. (2017) 4(3):e7951. doi: 10.2196/humanfactors.7951
171. Schure MB, Lindow JC, Greist JH, Nakonezny PA, Bailey SJ, Bryan WL, et al. Use of a fully automated internet-based cognitive behavior therapy intervention in a community population of adults with depression symptoms: randomized controlled trial. J Med Internet Res. (2019) 21(11):e14754. doi: 10.2196/14754
172. Stuart R, Fischer H, Leitzke AS, Becker D, Saheba N, Coleman KJ. The effectiveness of internet-based cognitive behavioral therapy for the treatment of depression in a large real-world primary care practice: a randomized trial. Perm J. (2022) 26(3):53–60. doi: 10.7812/TPP/21.183
173. Lu SHX, Assudani HA, Kwek TRR, Ng SWH, Teoh TEL, Tan GCY. A randomised controlled trial of clinician-guided internet-based cognitive behavioural therapy for depressed patients in Singapore. Front Psychol. (2021) 12:668384. doi: 10.3389/fpsyg.2021.668384
174. Imamura K, Kawakami N, Tsuno K, Tsuchiya M, Shimada K, Namba K. Effects of web-based stress and depression literacy intervention on improving symptoms and knowledge of depression among workers: a randomized controlled trial. J Affect Disord. (2016) 203:30–7. doi: 10.1016/j.jad.2016.05.045
175. Chee W, Kim S, Ji X, Park S, Chee E, Tsai H, et al. The effect of a culturally tailored web-based physical activity promotion program on Asian American midlife women’s depressive symptoms. Asian Pac Isl Nurs J. (2016) 1(4):162–73. doi: 10.9741/23736658.1044
176. Vieira S, Liang X, Guiomar R, Mechelli A. Can we predict who will benefit from cognitive-behavioural therapy? A systematic review and meta-analysis of machine learning studies. Clin Psychol Rev. (2022) 97:102193. doi: 10.1016/j.cpr.2022.102193
177. Hornstein S, Forman-Hoffman V, Nazander A, Ranta K, Hilbert K. Predicting therapy outcome in a digital mental health intervention for depression and anxiety: a machine learning approach. Digit Health. (2021) 7:1–11. doi: 10.1177/20552076211060659
178. Bremer V, Chow PI, Funk B, Thorndike FP, Ritterband LM. Developing a process for the analysis of user journeys and the prediction of dropout in digital health interventions: machine learning approach. J Med Internet Res. (2020) 22(10):e17738. doi: 10.2196/17738
179. Bennemann B, Schwartz B, Giesemann J, Lutz W. Predicting patients who will drop out of out-patient psychotherapy using machine learning algorithms. Br J Psychiatry. (2022) 220(4):192–201. doi: 10.1192/bjp.2022.17
180. Forsell E, Jernelöv S, Blom K, Kraepelien M, Svanborg C, Andersson G, et al. Proof of concept for an adaptive treatment strategy to prevent failures in internet-delivered CBT: a single-blind randomized clinical trial with insomnia patients. Am J Psychiatry. (2019) 176(4):315–23. doi: 10.1176/appi.ajp.2018.18060699
181. Alamdari PM, Navimipour NJ, Hosseinzadeh M, Safaei AA, Darwesh AA. Systematic study on the recommender systems in the e-commerce. IEEE Access. (2020) 8:115694–716. doi: 10.1109/ACCESS.2020.3002803
182. Liu Z, Zou L, Zou X, Wang C, Zhang B, Tang D, et al. Monolith: real time recommendation system with collisionless embedding table. arXiv. (2022):2209.07663. 48550/arXiv.2209.07663
183. Kulkarni PV, Rai S, Kale R. Recommender system in elearning: a survey. Proceeding of international conference on computational science and applications (2020). p. 119–26. doi: 10.1007/978-981-15-0790-8_13
184. Ong AA, Gillespie MB. Overview of smartphone applications for sleep analysis. World J Otorhinolaryngol Head Neck Surg. (2016) 2(1):45–9. doi: 10.1016/j.wjorl.2016.02.001
185. Bort-Roig J, Gilson ND, Puig-Ribera A, Contreras RS, Trost SG. Measuring and influencing physical activity with smartphone technology: a systematic review. Sports Med. (2014) 44(5):671–86. doi: 10.1007/s40279-014-0142-5
186. Boukhechba M, Daros AR, Fua K, Chow PI, Teachman BA, Barnes LE. Demonicsalmon: monitoring mental health and social interactions of college students using smartphones. Smart Health. (2018) 9-10:192–203. doi: 10.1016/j.smhl.2018.07.005
187. North F, Chaudhry R. Apple healthkit and health app: patient uptake and barriers in primary care. Telemed J E Health. (2016) 22(7):608–13. doi: 10.1089/tmj.2015.0106
188. Carr S. “AI gone mental”: engagement and ethics in data-driven technology for mental health. J Ment Health. (2020) 29(2):125–30. doi: 10.1080/09638237.2020.1714011
189. Fried EI, Nesse RM. Depression sum-scores don’t add up: why analyzing specific depression symptoms is essential. BMC Med. (2015) 13:1–11. doi: 10.1186/s12916-015-0325-4
190. Boschloo L, Bekhuis E, Weitz ES, Reijnders M, DeRubeis RJ, Dimidjian S, et al. The symptom-specific efficacy of antidepressant medication vs. Cognitive behavioral therapy in the treatment of depression: results from an individual patient data meta-analysis. World Psychiatry. (2019) 18(2):183–91. doi: 10.1002/wps.20630
191. Delgadillo J, Duhne PGS. Targeted prescription of cognitive–behavioral therapy versus person-centered counseling for depression using a machine learning approach. J Consult Clin Psychol. (2020) 88(1):14. doi: 10.1037/ccp0000476
192. Gunlicks-Stoessel M, Klimes-Dougan B, VanZomeren A, Ma S. Developing a data-driven algorithm for guiding selection between cognitive behavioral therapy, fluoxetine, and combination treatment for adolescent depression. Transl Psychiatry. (2020) 10:321. doi: 10.1038/s41398-020-01005-y
193. Lamers F, van Oppen P, Comijs HC, Smit JH, Spinhoven P, van Balkom AJ, et al. Comorbidity patterns of anxiety and depressive disorders in a large cohort study: the Netherlands study of depression and anxiety (NESDA). J Clin Psychiatry. (2011) 72(3):3397. doi: 10.4088/JCP.10m06176blu
Keywords: depression, digital mental health, personalization, precision care, iCBT, machine learning
Citation: Hornstein S, Zantvoort K, Lueken U, Funk B and Hilbert K (2023) Personalization strategies in digital mental health interventions: a systematic review and conceptual framework for depressive symptoms. Front. Digit. Health 5:1170002. doi: 10.3389/fdgth.2023.1170002
Received: 20 February 2023; Accepted: 5 May 2023;
Published: 22 May 2023.
Edited by:
Kwok Leung Tsui, Virginia Tech, United StatesReviewed by:
Rüdiger Christoph Pryss, Julius Maximilian University of Würzburg, Germany© 2023 Hornstein, Zantvoort, Lueken, Funk and Hilbert. This is an open-access article distributed under the terms of the Creative Commons Attribution License (CC BY). The use, distribution or reproduction in other forums is permitted, provided the original author(s) and the copyright owner(s) are credited and that the original publication in this journal is cited, in accordance with accepted academic practice. No use, distribution or reproduction is permitted which does not comply with these terms.
*Correspondence: Silvan Hornstein c2lsdmFuLmhvcm5zdGVpbkBodS1iZXJsaW4uZGU=
Disclaimer: All claims expressed in this article are solely those of the authors and do not necessarily represent those of their affiliated organizations, or those of the publisher, the editors and the reviewers. Any product that may be evaluated in this article or claim that may be made by its manufacturer is not guaranteed or endorsed by the publisher.
Research integrity at Frontiers
Learn more about the work of our research integrity team to safeguard the quality of each article we publish.