- 1Department of Medicine, Stanford University School of Medicine, Stanford, CA, United States
- 2Computational & Mathematical Engineering, Stanford University, Stanford, CA, United States
- 3United States Department of Veterans Affairs, Palo Alto Healthcare System, Palo Alto, CA, United States
- 4Department of Psychiatry, Stanford University School of Medicine, Stanford, CA, United States
- 5Department of Radiology, Stanford University School of Medicine, Stanford, CA, United States
- 6Department of Surgery, VA Palo Alto Health Care System, Menlo Park, CA, United States
- 7Department of Surgery, Stanford University School of Medicine, Stanford, CA, United States
- 8Department of Biomedical Data Science, Stanford University, Stanford, CA, United States
Objective: The opioid crisis brought scrutiny to opioid prescribing. Understanding how opioid prescribing patterns and corresponding patient outcomes changed during the epidemic is essential for future targeted policies. Many studies attempt to model trends in opioid prescriptions therefore understanding the temporal shift in opioid prescribing patterns across populations is necessary. This study characterized postoperative opioid prescribing patterns across different populations, 2010–2020.
Data Source: Administrative data from Veteran Health Administration (VHA), six Medicaid state programs and an Academic Medical Center (AMC).
Data extraction: Surgeries were identified using the Clinical Classifications Software.
Study Design: Trends in average daily discharge Morphine Milligram Equivalent (MME), postoperative pain and subsequent opioid prescription were compared using regression and likelihood ratio test statistics.
Principal Findings: The cohorts included 595,106 patients, with populations that varied considerably in demographics. Over the study period, MME decreased significantly at VHA (37.5–30.1; p = 0.002) and Medicaid (41.6–31.3; p = 0.019), and increased at AMC (36.9–41.7; p < 0.001). Persistent opioid users decreased after 2015 in VHA (p < 0.001) and Medicaid (p = 0.002) and increase at the AMC (p = 0.003), although a low rate was maintained. Average postoperative pain scores remained constant over the study period.
Conclusions: VHA and Medicaid programs decreased opioid prescribing over the past decade, with differing response times and rates. In 2020, these systems achieved comparable opioid prescribing patterns and outcomes despite having very different populations. Acknowledging and incorporating these temporal distribution shifts into data learning models is essential for robust and generalizable models.
Introduction
There are 53 million surgeries performed in the United States every year and the majority of patients experience moderate to severe postoperative pain (1–3). Opioids are often a first-line treatment of postoperative pain and perioperative opioid exposure is considered a gateway to opioid misuse and addiction (4–6). The increased prescription of opioid medications in the United States over the past several decades, including those for postoperative pain, led to widespread dependence and misuse (7). In 2017, more than 191 million opioid prescriptions were written in the United States and these prescription opioids were involved in more than 35% of all opioid overdose deaths (8). Therefore many health care systems and levels of government attempted to promote more judicious prescribing.
In 2010, the Drug Enforcement Administration (DEA) finalized a policy that required 2-factor authentication for electronic opioid prescribing to increase security and improve monitoring and tracking (9). In 2013, the Veterans' Health Administration (VHA) launched the ambitious Opioid Safety Initiative (OSI) to help decrease opioid prescribing practices through data audits and provide feedback (10). In 2016, two federal initiatives targeting opioid prescribing emerged: the Center for Disease Control (CDC) opioid prescribing guidelines (11) and the Surgeon General's “Call to Action” (12). In addition, many states initiated laws, such as the mandatory enrollment in prescription drug monitoring programs and payor rules (13). In addition, prescription drug monitoring programs were developed, such as the Controlled Substance Utilization Review and Evaluation System (CURES), which was mandated in California in 2018 (13). Finally, quality measures were modified to remove opioid incentives (14).
The rush of policies to curtail opioid over-prescribing had to balance the need for adequate pain management. Further complicating the issue is the diversity and non-comparability of populations, both regarding vulnerabilities and pain levels. Often, opioid-prescribing assessments are conducted in single health systems, which limits the generalizability of those insights gained. There is a need to assess the impacts of recent changes in opioid-related policies over a diverse set of patients.
The aim of this study was to characterize opioid prescribing and associated patient outcomes across three diverse healthcare systems over this period of change: 2010–2020. We hypothesized that opioid prescribing at surgical discharge would decrease over the study period and that patient pain outcomes would not change. The study included two closed systems, one with an integrated response to the epidemic [the Veterans Health Administration (VHA)] and another with a comparable population, but less integrated (Medicaid) and an open academic medical center (AMC), serving a different population. The study provides insight on the effectiveness of changing prescribing patterns on patient outcomes.
Study data and methods
This is a retrospective analysis of postoperative opioid prescribing patterns among adult patients at VHA (01/2010–06/2020), in six diverse Medicaid state programs (01/2016–06/2020), and an AMC (01/2010–06/2020). The years included are based on data availability and data use agreement contracts.
The investigation focused on surgical patients because their pain level is comparable to other opioid indications. Surgeries were categorized using the Clinical Classifications Software (online Supplementary Appendix S1A) and only the most recent surgery was considered. Patients with a length of stay greater than 13 days and patients without continuous healthcare encounters (Medicaid and AMC) were excluded. Finally, patients were excluded with no record of an opioid prescription at discharge. This study received the approval from the institutes' Institutional Review Board (IRB).
To standardize opioid strength assessment, we converted opioid medications to daily Morphine Milligram Equivalents (MME) according to CDC calculation (15, 16). Persistent opioid prescriptions (POP) were defined as a new outpatient opioid prescription between 3 and 6 months after surgery (17). Trends were calculated using the Joinpoint regression algorithm (version 4.9) (18), based on the likelihood ratio test statistic. We identified the best-fitting regression models for each outcome over time and significant changes in the trends. For each slope, the annual percentage change (APC) was calculated.
Study results
A total of 460,653 VHA, 92,147 Medicaid, and 42,306 AMC patients were included in the study (Figure 1). Table 1 shows patient demographic characteristics stratified by population. Overall, VHA prescribed weaker opioid medications at discharge compared to Medicaid and the AMC, with an average MME of 32.2 ± 21.1, 35.5 ± 20.7, and 40.0 ± 19.5 respectively. Yet, both VHA and Medicaid had significantly higher rates of POP compared to the AMC (20.5%, 24.6%, 12.2%, respectively).
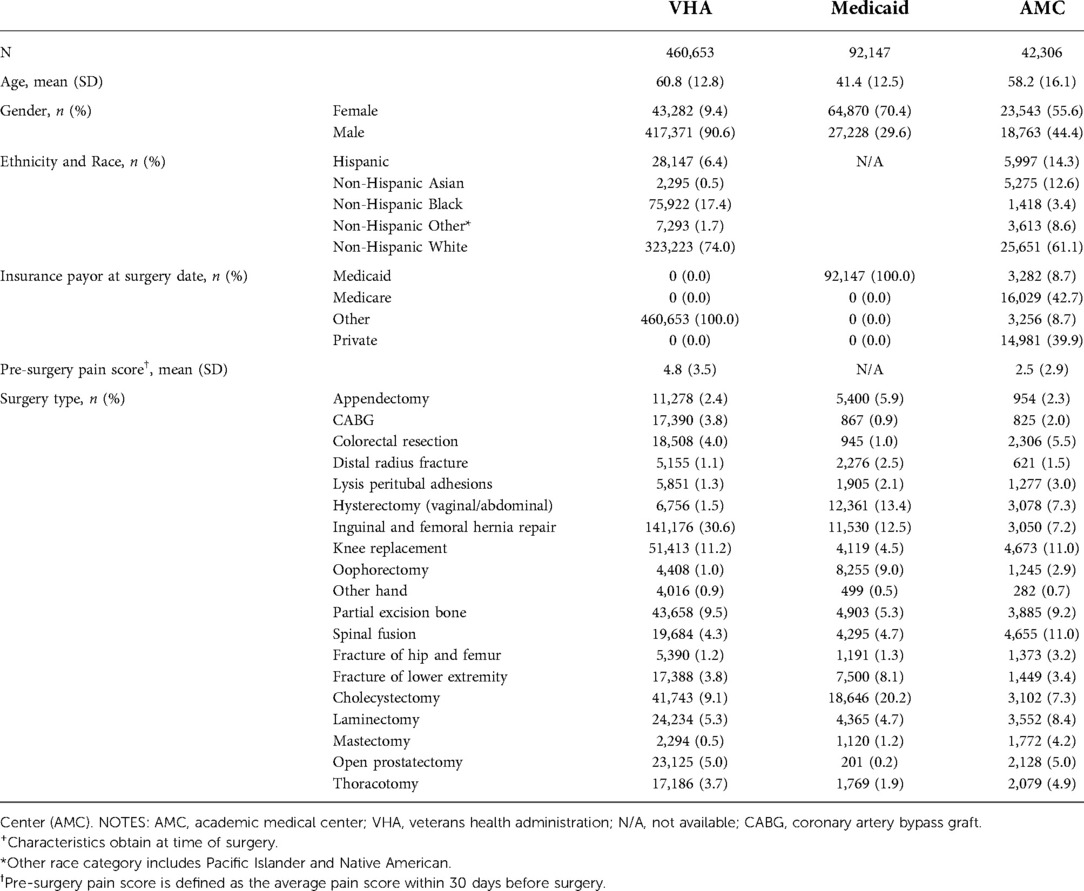
Table 1. Demographic and clinical characteristics of surgical patients stratified by healthcare system+.
At VHA, the average MME prescribed at discharge decreased from 37.5 in 2010 to 30.1 in 2014 (APC: 5.4%, p = 0.002) and remained constant at 31.2 thereafter (p = 0.799) (Figure 2A). The average discharge MME in Medicaid decreased from 41.6 in 2016 to 31.3 in 2020 (APC: 8.4%, p = 0.019). In the AMC, average MME increased from 36.9 in 2010 to 41.7 in 2020 (APC: 1.2%, p < 0.001).
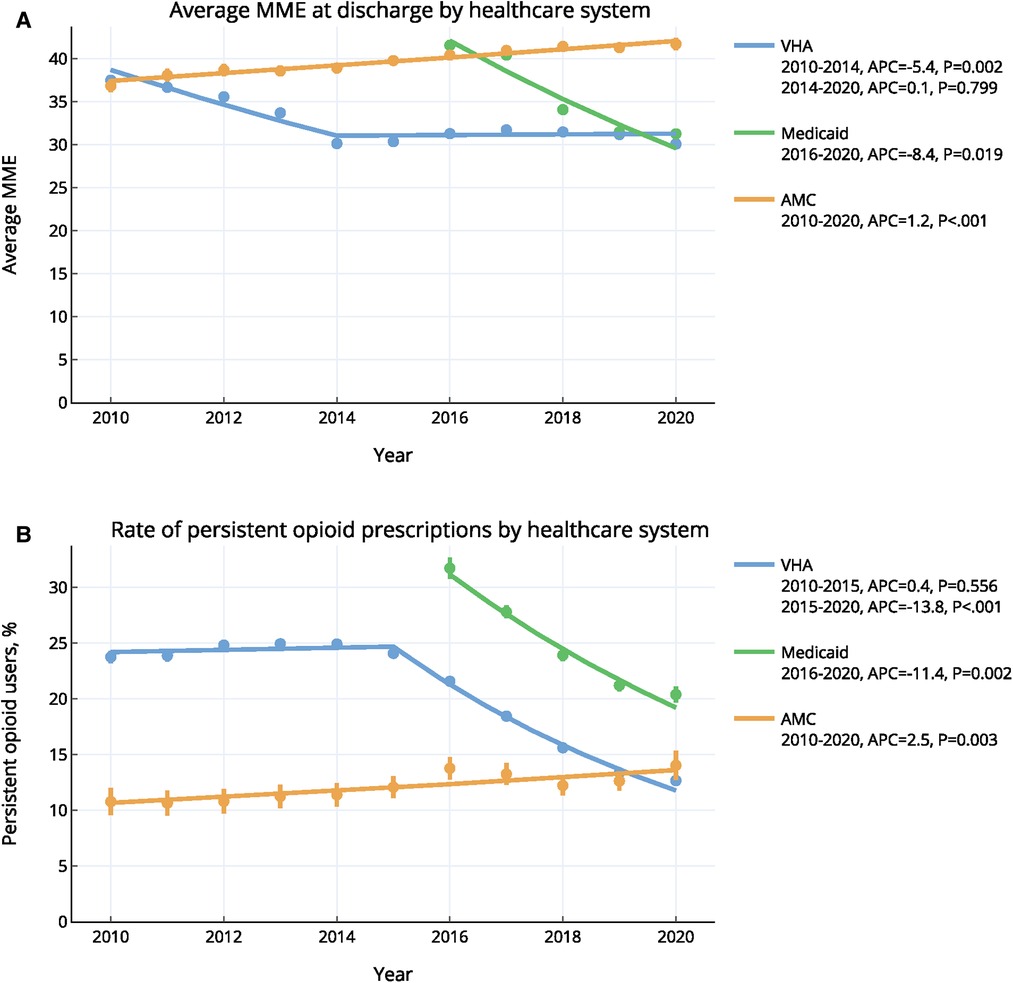
Figure 2. Annual trends of (A) opioid prescribing and (B) persistent opioid prescriptions in three healthcare systems, 2010–2020 *error bars indicate 95% confidence intervals. Trends were calculated using the ioinpoint regression algorithm. VHA, veterans health administration; AMC, academic medical center; MME, morphine milligram equivalent.
From 2010 to 2015, the rate of POP for VHA remained constant (24.4%; p = 0.556), and decreased significantly after 2015 (APC: 13.8%, p < 0.001) to reach a rate of 12.7% in 2020 (Figure 2B). For the Medicaid cohort, the rate of POP decreased significantly from 31.7% in 2016 to 20.4% in 2020 (APC: 11.4%, p = 0.002). Finally, the rate of POP for AMC increased significantly, rising 2.5% annually from 10.8% in 2010 to 14.0% in 2020 (p = 0.003).
Pain scores were available for VHA and AMC. From 2010 to 2020, the average 3-week postoperative pain score decreased from 3.0 to 2.6 for VHA (p = 0.004), while remained constant at AMC (avg: 2.1; p = 0.068). Other outcomes, such as 30-day readmissions and emergency visits, remained relatively constant over the study period in all healthcare systems (online Supplementary Table S2A).
Discussion
Multiple policies directed at opioid prescribing patterns have been initiated over the last decade. Understanding the temporal changes of opioid prescriptions and related outcomes in the real-world setting is important for future policy initiatives. We found VHA rapidly decreased average MMEs prescribed at discharge early in the study period and Medicaid followed soon thereafter. In contrast, average MME slightly increased at the AMC. Correspondingly, persistent opioid prescription (POP) rates significantly decreased at VHA and Medicaid, while rates at the AMC slightly increased. However, rates of POP at the AMC started significantly lower than the other two closed systems. Although MME decreased at VHA and Medicaid, patient outcomes remained constant, including pain scores at VHA.
These findings demonstrate important changes in provider prescribing behavior and opioid-related adverse events. Previously, providers were strongly encouraged to minimize pain and opioid prescriptions dramatically increased (19). Here, we demonstrate a pendulum effect, with providers greatly reducing opioid strength, likely in response to policies promoting judicious prescribing, similar to other studies focused on chronic pain. Both VHA and Medicaid had high rates of POP at the start of the study, highlighting the opioid problem in these populations. In 2013, the VHA implemented the OSI program to address to the opioid epidemic. This program was multipronged, targeting education, risk mitigation, pain management and addiction arms (20). The VHA informatics infrastructure allowed for the system to follow individual prescribing provide benchmarks for providers and facilities across the country. Medicaid opioid prescriptions also decreased in 2016, following the multiple state and federal incentives. However, previous studies analyzing opioid prescriptions prior to 2016 in the Medicaid population have shown either consistent or increased opioid prescriptions before 2015 (21–23). In the AMC where individual providers are generally less regulated, MME prescribing increased over the study period. This work suggests providers are responsive to changing landscapes and polices, especially within the tighter controls in closed systems.
Even as opioid prescriptions strengths were reduced, other patient outcomes did not significantly change, as measured by 3-week postoperative pain scores, 30-day readmissions, and emergency visits. This work supports policies reducing opioid consumption, at least from the extremely high levels in 2010 (24). As opioid prescribing declines, proposals to further reduce use must ensure that patient outcomes continue to be maintained, with focus on to avoiding a decline in quality of life due to avoidable pain (25).
Our study has limitations. This is an observational retrospective study and cannot be interpreted as causal, although our findings were robust and represent large population-based cohorts. As we limit prescription data to that which is associated with surgeries, we provide a limited view to opioid prescribing and utilization dynamics. However, as surgery is considered a gateway to prescription opioids (6), this work provides important insights into an important pathway to initial opioid exposure. We could not confirm whether additional prescriptions were related to the index surgery. However, we exclude patients with a second surgery during the follow-up period, in an attempt to mitigate this limitation. In addition, we do not know if the patient used the prescribed medication, therefore the overall POP rates could be erroneous. It is possible that some patients may be represented in multiple service groups (e.g., VHA and AMC) and as data are de-identified we are not able to identify patients in multiple groups, however this number is likely small relative to the overall cohort sizes. Finally, results from three specific populations may not be generalizable to all.
Conclusion
In this population-based study, we found significant decreases in opioid prescribing patterns in both closed systems (VHA and Medicaid) and corresponding improvements in patient outcomes. The AMC had different trends, likely reflecting the stark difference in patient populations and underlying medical conditions. These temporal shifts will affect the performance and generalizability of associated AI technologies. This work provides insight into the effect of federal initiatives targeting opioid reduction strategies, and highlights reduced postoperative opioid prescribing does not impair patient outcomes. Further research is needed to confirm these findings.
Data availability statement
The data analyzed in this study is subject to the following licenses/restrictions: The data used in this study contain patient identifiers therefore they are not publicly available. Requests to access these datasets should be directed toYm91c3NhcmRAc3RhbmZvcmQuZWR1.
Ethics statement
The studies involving human participants were reviewed and approved by Stanford University. Written informed consent for participation was not required for this study in accordance with the national legislation and the institutional requirements.
Author contributions
THB: has full access to all of the data in the study and takes responsibility for the integrity of the data and the accuracy of the data analysis. Study concept and design: THB, CMC, TO. Acquisition of data: JC, AZ, OEH. Analysis and interpretation of data: all authors. Drafting of the manuscript: JC, THB. Critical revision of the manuscript for important intellectual content: all authors. Statistical analysis: JC. Administrative, technical, or material support: THB. Study supervision: THB. All authors contributed to the article and approved the submitted version.
Funding
This material is the result of work supported with resources and the use of facilities at the Palo Alto Veterans Hospital. Research reported in this publication was supported by the National Library of Medicine of the National Institutes of Health under Award Number R01LM013362. Data and support for this work were provided by HMS (now part of Gainwell Technologies) as part of the Digital Health CRC (Cooperative Research Centre) Limited. The DHCRC is established and supported under the Australian Government's Cooperative Research Centres Program. The content is solely the responsibility of the authors and does not necessarily represent the official views of the National Institutes of Health or the Department of Veterans Affairs. The Funding sources had no role in the design and conduct of the study; collection, management, analysis, and interpretation of the data; preparation, review, or approval of the manuscript; and decision to submit the manuscript for publication.
Conflict of interest
The authors declare that the research was conducted in the absence of any commercial or financial relationships that could be construed as a potential conflict of interest.
Publisher's note
All claims expressed in this article are solely those of the authors and do not necessarily represent those of their affiliated organizations, or those of the publisher, the editors and the reviewers. Any product that may be evaluated in this article, or claim that may be made by its manufacturer, is not guaranteed or endorsed by the publisher.
Supplementary material
The Supplementary Material for this article can be found online at: https://www.frontiersin.org/articles/10.3389/fdgth.2022.995497/full#supplementary-material.
References
1. Apfelbaum JL, Chen C, Mehta SS, Gan TJ. Postoperative pain experience: results from a national survey suggest postoperative pain continues to be undermanaged. Anesth Analg. (2003) 97(2):534–40; table of contents. doi: 10.1213/01.ANE.0000068822.10113.9E
2. DeFrances CJ, Podgornik MN. National hospital discharge survey. Adv Data Vital Health Stat Natl Health Stat. (2004) 2006(371):1–19. Available at: https://stacks.cdc.gov/view/cdc/83543. PUBMED: 16703980
3. Adamson SJ, Deering DE, Sellman JD, Sheridan J, Henderson C, Robertson R. An estimation of the prevalence of opioid dependence in New Zealand. Int J Drug Policy. (2012) 23(1):87–9. doi: 10.1016/j.drugpo.2011.05.013
4. Wunsch H, Wijeysundera DN, Passarella MA, Neuman MD. Opioids prescribed after low-risk surgical procedures in the United States, 2004–2012. JAMA. (2016) 315(15):1654–7. doi: 10.1001/jama.2016.0130
5. Alam A, Gomes T, Zheng H, Mamdani MM, Juurlink DN, Bell CM. Long-term analgesic use after low-risk surgery: a retrospective cohort study. Arch Intern Med Mar. (2012) 172(5):425–30. doi: 10.1001/archinternmed.2011.1827
6. Waljee JF, Li L, Brummett CM, Englesbe MJ. Iatrogenic opioid dependence in the United States: are surgeons the gatekeepers? Ann Surg. (2017) 265(4):728–30. doi: 10.1097/SLA.0000000000001904
7. Han B, Compton WM, Jones CM, Cai R. Nonmedical prescription opioid use and use disorders among adults aged 18 through 64 years in the United States, 2003–2013. JAMA. (2015) 314(14):1468–78. doi: 10.1001/jama.2015.11859
8. Centers for Disease Control and Prevention. Annual Surveillance Report of Drug-Related Risks and Outcomes — United States. Surveillance Special Report 2. Centers for Disease Control and Prevention, U.S. Department of Health and Human Services. Published August 31, 2018 (2018).
9. Gawande AA. It’s time to adopt electronic prescriptions for opioids. Ann Surg. (2017) 265(4):693–4. doi: 10.1097/SLA.0000000000002133
10. Lin LA, Bohnert ASB, Kerns RD, Clay MA, Ganoczy D, Ilgen MA. Impact of the opioid safety initiative on opioid-related prescribing in veterans. Pain. (2017) 158(5):833–9. doi: 10.1097/j.pain.0000000000000837
11. Frieden TR, Houry D. Reducing the risks of relief–the CDC opioid-prescribing guideline. N Engl J Med. Apr. (2016) 374(16):1501–4. doi: 10.1056/NEJMp1515917
12. Murthy VH. Ending the opioid epidemic - a call to action. N Engl J Med. Dec. (2016) 375(25):2413–5. doi: 10.1056/NEJMp1612578
13. Phan MT, Wong C, Tomaszewski DM, Kain ZN, Jenkins B, Donaldson C, et al. Evaluating opioid dispensing rates among pediatrics and young adults based on CURES data reporting in California from 20152019. J Contemp Pharm Prac. (2021) 67(4):23–32. doi: 10.37901/jcphp20-00012
14. Ducoffe AR, York A, Hu DJ, Perfetto D, Kerns RD. National action plan for adverse drug event prevention: recommendations for safer outpatient opioid use. Pain Med. (2016) 17(12):2291–304. doi: 10.1093/pm/pnw106
15. Lossio-Ventura JA, Song W, Sainlaire M, Dykes PC, Hernandez-Boussard T. Opioid2MME: standardizing opioid prescriptions to morphine milligram equivalents from electronic health records. Int J Med Inform. (2022) 162:104739. doi: 10.1016/j.ijmedinf.2022.104739
16. Control NCfIPa. CDC Compilation of opioid analgesic formulations with morphine milligram equivalent conversion factors, 2015 version. GA: Centers for Disease Control and Prevention Atlanta (2015).
17. Jivraj NK, Raghavji F, Bethell J, Wijeysundera DN, Ladha KS, Bateman BT, et al. Persistent postoperative opioid use: a systematic literature search of definitions and population-based cohort study. Anesthesiology. (2020) 132(6):1528–39. doi: 10.1097/ALN.0000000000003265
18. Kim HJ, Fay MP, Feuer EJ, Midthune DN. Permutation tests for joinpoint regression with applications to cancer rates. Stat Med. (2000) 19(3):335–51. doi: 10.1002/(SICI)1097-0258(20000215)19:3%3C335::AID-SIM336%3E3.0.CO;2-Z
19. Tedesco D, Asch SM, Curtin C, Hah J, McDonald KM, Fantini MP, et al. Opioid abuse and poisoning: trends in inpatient and emergency department discharges. Health Aff. (2017) 36(10):1748–53. doi: 10.1377/hlthaff.2017.0260
20. Gellad WF, Good CB, Shulkin DJ. Addressing the opioid epidemic in the United States: lessons from the department of veterans affairs. JAMA Intern Med. (2017) 177(5):611–2. doi: 10.1001/jamainternmed.2017.0147
21. Sullivan MD, Bauer AM, Fulton-Kehoe D, Garg RK, Turner JA, Wickizer T, et al. Trends in opioid dosing among Washington State medicaid patients before and after opioid dosing guideline implementation. J Pain. (2016) 17(5):561–8. doi: 10.1016/j.jpain.2015.12.018
22. Tehrani AB, Henke RM, Ali MM, Mutter R, Mark TL. Trends in average days’ supply of opioid medications in medicaid and commercial insurance. Addict Behav. (2018) 76:218–22. doi: 10.1016/j.addbeh.2017.08.005
23. Keast SL, Kim H, Deyo RA, Middleton L, McConnell KJ, Zhang K, et al. Effects of a prior authorization policy for extended-release/long-acting opioids on utilization and outcomes in a state medicaid program. Addiction. (2018) 113(9):1651–60. doi: 10.1111/add.14248
24. Humphreys K. Avoiding globalisation of the prescription opioid epidemic. Lancet. (2017) 390(10093):437–9. doi: 10.1016/S0140-6736(17)31918-9
Keywords: opioid, temporal pattern mining, surgery, postoperative pain, prescribing patterns
Citation: Coquet J, Zammit A, Hajouji OE, Humphreys K, Asch SM, Osborne TF, Curtin CM and Hernandez-Boussard T (2022) Changes in postoperative opioid prescribing across three diverse healthcare systems, 2010–2020. Front. Digit. Health 4:995497. doi: 10.3389/fdgth.2022.995497
Received: 15 July 2022; Accepted: 27 October 2022;
Published: 6 December 2022.
Edited by:
Alex Mariakakis, University of Toronto, CanadaReviewed by:
Terri Elizabeth Workman, George Washington University, United StatesDi Liang, Fudan University, China
© 2022 Coquet, Zammit, Hajouji, Humphryes, Asch, Osborne, Curtin and Hernandez-Boussard. This is an open-access article distributed under the terms of the Creative Commons Attribution License (CC BY). The use, distribution or reproduction in other forums is permitted, provided the original author(s) and the copyright owner(s) are credited and that the original publication in this journal is cited, in accordance with accepted academic practice. No use, distribution or reproduction is permitted which does not comply with these terms.
*Correspondence: Tina Hernandez-Boussard Ym91c3NhcmRAc3RhbmZvcmQuZWR1
Specialty Section: This article was submitted to Health Informatics, a section of the journal Frontiers in Digital Health