Commentary: Artificial Intelligence and Statistics: Just the Old Wine in New Wineskins?
- 1Medical Retina Department, Moorfields Eye Hospital NHS Foundation Trust, London, United Kingdom
- 2Medignition Inc., Research Consultants, Zurich, Switzerland
- 3Health Data Research UK, London, United Kingdom
- 4National Institute for Health Research (NIHR) Biomedical Research Centre for Ophthalmology, Moorfields Eye Hospital National Health Service (NHS) Foundation Trust and University College London (UCL) Institute of Ophthalmology, London, United Kingdom
- 5Julius Center for Health Science and Primary Care, University Medical Center Utrecht, University of Utrecht, Utrecht, Netherlands
- 6Department of Biostatistics, Epidemiology, Biostatistics and Prevention Institute, University of Zurich, Zurich, Switzerland
- 7Department of Clinical Epidemiology, Biostatistics and Bioinformatics, Amsterdam Public Health Research Institute, Amsterdam University Medical Centers, Amsterdam, Netherlands
Introduction
We are witnessing a tremendous increase in scientific studies in the medical literature using Artificial Intelligence (AI) and its branch Machine Learning (ML) methods in particular. A recent systematic review comparing the classification performance of healthcare professionals vs. AI retrieved over 20,000 records of study reports published since January 2012. In 2020 alone, over 7,000 new records were found in medical electronic databases (1). Simply by searching the Medline database using the Medical Subject Heading (MeSH) “Artificial Intelligence,” which was introduced in 1986, we find a continued increase of records over the last two decades (Figure 1). The total number of records currently indexed with the term adds up to 120,000 in Medline alone. Several issues beside the sheer number become apparent when reading through those papers.
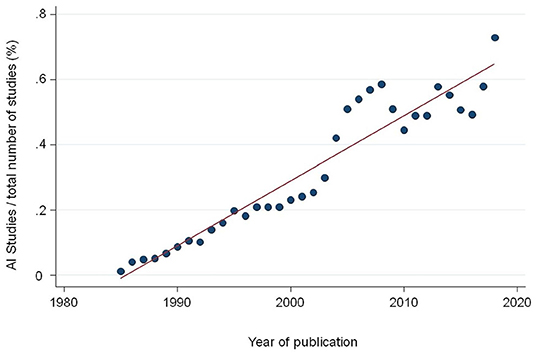
Figure 1. Proportion of studies indexed in Medline with the Medical Subject Heading (MeSH) term “Artificial Intelligence” divided by the total number of publications per year.
What Is the Difference Between AI and Statistics?
The general readership of medical journals, including clinicians, researchers, statisticians, and methodologists have experienced confusion with some of the terms they encounter in papers on AI. Table 1 shows a collection of terms found in the statistics world and its typical counterparts in the ML/AI field (2–4). A lack of consensus regarding terminology makes the comparability of studies and study results difficult, or even impossible. For example, in medical applications, diagnostic accuracy is usually reported using statistics as sensitivity, specificity, and area under the receiver operating characteristic curve. Studies using traditional statistical methods should report their results following the relevant reporting guidelines, such as STARD for diagnostic accuracy studies (5) and TRIPOD for prognostic models (6). In ML applications, models are commonly reported with other terms (i.e., recall and confusion matrix) but also different metrics (i.e., F1 score and dice coefficient). For example, reporting two by two tables of results for clinically relevant thresholds would achieve a higher comparability of studies by simple means.
Another conflict relates to the connection between AI and statistics. A growing number of researchers from various disciplines have expressed the view that many of the research questions are not too different in both disciplines (7). In fact, it may be argued that a large number of the differences in the analytical approach are only superficial and caused more by differences in terminology and scientific culture than from genuine dissimilarities (8). Differences may exist in terminology (not intentional) as they evolved in different scientific cultures with their legacy, nomenclature, notation, and philosophical perspectives (7, 9, 10).
The Challenge of Developing a Common Scientific Language
Recently, several initiatives have been launched to advance the quality of reporting and the consistency of terminology in AI studies. It has been recognized that arriving at a consensus about a set of terms that could be used interchangeably between disciplines would reduce some of the unnecessary complexities which, for example, systematic reviewers might face when assessing different studies. Addressing these concerns, the Cochrane collaboration initiated the Cochrane Prognosis Methods Group (11), since “methodological development and refinement” was seen as crucial for future systematic reviews of prognostics studies. Also, guidelines for reporting development and validation of research using AI methodology (TRIPOD-AI and PROBAST-AI) are currently being developed by Collins et al. (12). Reporting guidelines for the early evaluation of AI systems (DECIDE-AI), performance evaluation (STARD-AI), and the evaluation in randomized controlled trials (CONSORT-AI) are also being developed (13, 14). The guidelines, using a principled approach including a consensus process among computer scientists, mathematicians, statisticians, healthcare professionals, epidemiologists, and clinicians, aim at improving completeness of reporting and shall assist researchers and policymakers when critically appraising the design, conduct, and analysis of ML based prediction model studies.
The Chaos of Humans and Healthcare
At present, many of the algorithms frequently cited in the literature are hardly applicable in clinical practice (15). This is for two reasons: first, these AI innovations by themselves do not re-engineer the incentives that govern existing ways of working. A complex web of ingrained political and economic factors as well as the proximal influence of medical practice norms and commercial interests determine the way healthcare is delivered (16). Regulations and guidelines currently in use are not sufficient for AI methods to be reported in such detail that they can be reproduced and safely implemented in clinical practice for classification or prediction in new patients (17).
Perhaps with the exception of AI incorporated in Computer Aided Design (CAD) systems in radiology, simply adding AI applications to a fragmented system will not necessarily create sustainable change (18). However, by embedding AI applications into key drivers of a healthcare system, as was done within the National Health Service (NHS) in UK AI/automation could have real impact, if applied in a staged approach (19, 20).
Second, most healthcare organizations lack the data infrastructure required to collect the data that are needed for training algorithms so they can be (a) updated to the local population and/or the local practice patterns (a requirement prior to deployment that is rarely highlighted by current AI publications) and (b) for investigating the potential for biases, to guarantee that the algorithms perform consistently across patient cohorts, especially those who may not have been adequately represented in the training cohort (18). Additionally, the key regulators are still in consultation phase on how AI applications will be regulated, and what the level of validation needed is. There is also no assurance on how AI models will be monitored and audited in the event of adverse outcomes. Recent publications from the fields of sports medicine and oncology reflect these complexities (21, 22).
The Ambitious Search for Suitable Areas of Application
Artificial intelligence and its branch ML have had their greatest successes in high signal/noise situations, e.g., visual and sound recognition, language translation, and playing games with concrete rules (23–28). What distinguishes these is rapid feedback while training, and availability of the correct answer. Things are different in the low signal/noise world and small datasets that typically prevail in diagnostic and descriptive prognostic research in medicine (29). A recent systematic review comparing AI with traditional statistical approaches found no advantages in terms of predictive accuracy between models developed with AI over logistic regression (9). Artificial intelligence can very well be applied in pattern recognition, to mimic or improve expert image interpretations. For estimating the probability of a positive biopsy given symptoms, signs, risk factors, and demographics, usefulness seems limited.
Currently, the most promising fields for AI applications are likely to be found in circumstances where the interpretation of estimated regression coefficients is not an issue, like in the context of triage based on image analysis. Efficient automated triage could reduce the burden of health services as it would identify a set of patients requiring timely care. Another area is AI application in less affluent countries where the lack of medical experts is a major impediment for an efficient delivery of healthcare (30, 31).
An essential difference between human and AI is that humans can learn efficiently even from small amounts of data. The neuronal processes involved are little known. A small child, for example, can recognize a leopard as a cat after looking at a few cat pictures. Machines generally need much more data to accomplish the same task. In addition, machines have no common sense. Although ML generally requires a large sample size, it is not clear how this can make accurate and unbiased predictions in erroneous data typically found in electronic medical records. Simply increasing the amount of data does not solve fundamental data quality problems (32).
On the other hand, AI algorithms can learn from huge amounts of data. While an AI model can be trained using a large amount of patient data from electronic patient files, a physician's ability to learn from experience is limited. Throughout his or her career, he or she will probably see only a fraction of the number of patients that can be offered to a ML model. Consequently substantial progress was made in clinical fields with large amounts of structured data such as pathology, radiology, and cardiovascular imaging. Results of classical comparative efficacy research and pragmatic studies have provided important insights for clinical practice by means of observational data. Recent attempts to use ML for this purpose have shown, however, that this is difficult because of the insufficient quality of the data sets. Moreover, the models need updating when new insights make this necessary. The claim to adapt these models due to regional differences in prescription practice is also hardly successful. The vision of automatically extracting the relevant variables from the electronic health record systems is promising but not yet a reality (33).
A promise of AI in health care is the avoidance of biases in arriving at diagnosis and in assigning medical treatment; a computer algorithm could objectively synthesize and interpret the data in the medical record. Integration of ML with clinical decision support tools, such as computerized alerts or diagnostic support, may offer physicians and others who provide health care targeted and timely information that can improve clinical decisions. The appropriateness of study data in terms of quality and representativeness is also of great importance in the world of ML. It is an unrealistic dream to believe that maximizing the amount of data automatically increases the quality of the data. The larger the proportion of errors in a data set, the more likely it is that the erroneous data and decisions will be represented in the model. The validity of data should always be distinguished from the quantity. While the former allows valid conclusions to be drawn, the latter only increases the precision of the potentially biased result (34, 35).
Artificial intelligence can be helpful in completing difficult tasks, such as the assessment of large amounts of imaging data. In order to develop its full potential, several steps need to be taken: First, joining the forces of various disciplines and stakeholders, and concerted efforts to reach a consensus about the terminology are needed. Second, once the various fields start using the same language, consolidation of the body of evidence will be feasible and specific requirements for sound research can be depicted. Third, results from AI research should be reported in way that directly inform clinical practice. Fourth, studies reporting the results of AI research should be methodologically comparable and accessible to meta-epidemiological assessment and economic evaluations.
Discussion
It should be acknowledged that by the start of the twentieth century, medicine had moved from the empirical observation of individual cases to the scientific applications of today's research culture (36). Based on the fundamental findings of Pierre Simon Laplace, Ronald Fisher, Austin Bradford Hill, and other brilliant scientists, the body of knowledge that characterizes clinical research today was created (37). Fundamental work on ML goes back to the 1960s and was developed on the basis of mathematical and statistical principles to which traditional statistics also refers (38). It is worth remembering its roots.
The scientific community is called upon to make its contribution in knowledge development and cultivation. The recently launched initiatives will lay important foundations to make the findings from studies using these new forms of analysis more understandable, comparable, and critically assessable in the medium term (17). Ultimately, this goal is essential. Clinical research should never be an end in itself but should be at the service of improving medical care for the benefit of all.
Author Contributions
LF and LB have drafted the manuscript. LF has created tables and figures. All authors have contributed to the conception to this opinion article, have reviewed, and edited the manuscript.
Conflict of Interest
LB is employed by Medignition Inc., Research Consultants.
The remaining authors declare that the research was conducted in the absence of any commercial or financial relationships that could be construed as a potential conflict of interest.
Publisher's Note
All claims expressed in this article are solely those of the authors and do not necessarily represent those of their affiliated organizations, or those of the publisher, the editors and the reviewers. Any product that may be evaluated in this article, or claim that may be made by its manufacturer, is not guaranteed or endorsed by the publisher.
References
1. Liu X, Faes L, Kale AU, Wagner SK, Fu DJ, Bruynseels A, et al. A comparison of deep learning performance against health-care professionals in detecting diseases from medical imaging: a systematic review and meta-analysis. Lancet Digit Health. (2019). 1:e271–97. doi: 10.1016/S2589-7500(19)30123-2
2. van Smeden M. Machine Learning Versus Traditional Statistical Modeling Medical Doctors. (2019). Available online at: https://de.slideshare.net/MaartenvanSmeden/machine-learning-versus-traditional-statistical-modeling-and-medical-doctors (accessed February 11, 2020).
3. Oberski D,. Incidental Data for Serious Social Research. (2018). Available online at: https://www.db-thueringen.de/receive/dbt_mods_00035117 (accessed December 21, 2021).
4. Tibshirani R. Modern Applied Statistics: Elements of Statistical Learning – Course. (2018). Available online at: http://statwebstanfordedu/~tibs/stat315a/glossary.pdf. (accessed February 11, 2020).
5. Bossuyt PM, Reitsma JB, Bruns DE, Gatsonis CA, Glasziou PP, Irwig L, et al. STARD 2015: an updated list of essential items for reporting diagnostic accuracy studies. BMJ. (2015) 351:h5527. doi: 10.1136/bmj.h5527
6. Collins GS, Reitsma JB, Altman DG, Moons KG. Transparent reporting of a multivariable prediction model for individual prognosis or diagnosis (TRIPOD): the TRIPOD statement. BMJ. (2015) 350:g7594. doi: 10.1136/bmj.g7594
7. Wasserman L. Normal Deviate - Thoughts on Statistics Machine Learning. (2013). Available online at: https://normaldeviate.wordpress.com/2013/02/16/rise-of-the-machines/ (accessed December 23, 2021).
8. Friedrich S, Antes G, Behr S, Binder H, Brannath W, Dumpert F, et al. Is there a role for statistics in artificial intelligence? Adv Data Anal Classif. (2021). doi: 10.1007/s11634-021-00455-6
9. Christodoulou E, Ma J, Collins GS, Steyerberg EW, Verbakel JY, Van Calster B, et al. systematic review shows no performance benefit of machine learning over logistic regression for clinical prediction models. J Clin Epidemiol. (2019) 110:12–22. doi: 10.1016/j.jclinepi.2019.02.004
10. Ebner J. What's the Difference Between Machine Learning, Statistics Data Mining? (2016). Available online at: https://wwwsharpsightlabscom/blog/difference-machine-learning-statistics-data-mining/ (accessed December 23, 2021).
11. Moons KG, Hooft L, Williams K, Hayden JA, Damen JA, Riley RD. Implementing systematic reviews of prognosis studies in Cochrane. Cochrane Database Syst Rev. (2018) 10:ED000129. doi: 10.1002/14651858.ED000129
12. Collins GS, Dhiman P, Andaur Navarro CL, Ma J, Hooft L, Reitsma JB, et al. Protocol for development of a reporting guideline (TRIPOD-AI) and risk of bias tool (PROBAST-AI) for diagnostic and prognostic prediction model studies based on artificial intelligence. BMJ Open. (2021) 11:e048008. doi: 10.1136/bmjopen-2020-048008
13. Liu X, Cruz Rivera S, Moher D, Calvert MJ, Denniston AK, Spirit AI, et al. Reporting guidelines for clinical trial reports for interventions involving artificial intelligence: the CONSORT-AI extension. Lancet Digit Health. (2020) 2:e537–48. doi: 10.1136/bmj.m3164
14. Sounderajah V, Ashrafian H, Aggarwal R, De Fauw J, Denniston AK, Greaves F, et al. Developing specific reporting guidelines for diagnostic accuracy studies assessing AI interventions: the STARD-AI Steering Group. Nat Med. (2020) 26:807–8. doi: 10.1038/s41591-020-0941-1
15. Kelly CJ, Karthikesalingam A, Suleyman M, Corrado G, King D. Key challenges for delivering clinical impact with artificial intelligence. BMC Med. (2019) 17:195. doi: 10.1186/s12916-019-1426-2
16. Oala L, Murchison AG, Balachandran P, Choudhary S, Fehr J, Leite AW, et al. Machine learning for health: algorithm auditing and quality control. J Med Syst. (2021) 45:105. doi: 10.1007/s10916-021-01783-y
17. Shelmerdine SC, Arthurs OJ, Denniston A, Sebire NJ. Review of study reporting guidelines for clinical studies using artificial intelligence in healthcare. BMJ Health Care Inform. (2021) 28:e100385. doi: 10.1136/bmjhci-2021-100385
18. Panch T, Mattie H, Celi LA. The “inconvenient truth” about AI in healthcare. NPJ Digit Med. (2019) 2:77. doi: 10.1038/s41746-019-0155-4
19. Korot E, Wood E, Weiner A, Sim DA, Trese M A. renaissance of teleophthalmology through artificial intelligence. Eye (Lond). (2019) 33:861–3. doi: 10.1038/s41433-018-0324-8
20. Sim DA, Mitry D, Alexander P, Mapani A, Goverdhan S, Aslam T, et al. The evolution of teleophthalmology programs in the United Kingdom: beyond diabetic retinopathy screening. J Diabetes Sci Technol. (2016) 10:308–17. doi: 10.1177/1932296816629983
21. Bullock GS, Hughes T, Sergeant JC, Callaghan MJ, Riley R, Collins G. Methods matter: clinical prediction models will benefit sports medicine practice, but only if they are properly developed and validated. Br J Sports Med. (2021) 55:1319–21. doi: 10.1136/bjsports-2021-104329
22. Dhiman P, Ma J, Navarro CA, Speich B, Bullock G, Damen JA, et al. Reporting of prognostic clinical prediction models based on machine learning methods in oncology needs to be improved. J Clin Epidemiol. (2021) 138:60–72. doi: 10.1016/j.jclinepi.2021.06.024
23. Krizhevsky A, Sutskever I, Hinton GE. ImageNet Classification with Deep Convolutional Neural Networks. (2012). Available online at: https://papersnipscc/paper/4824-imagenet-classification-with-deep-convolutional-neural-networks (accessed February 11, 2020).
24. Hinton G, Deng L, Yu D, Dahl G, Mohamed A-R, Jaitly N, et al. Deep Neural Networks for Acoustic Modeling in Speech Recognition. (2012). Available online at: https://staticgoogleusercontentcom/media/researchgooglecom/de//pubs/archive/38131pdf (accessed February 11, 2020).
25. Hadsell R, Erkan A, Sermanet P, Scoffier M, Muller U, LeCun Y. Deep belief net learning in a long-range vision system for autonomous off-road driving. In: 2008 IEEE/RSJ International Conference on Robots and Intelligent Systems, Vols. 1–3, Conference Proceedings. (2008) 2008:628–33. doi: 10.1109/IROS.2008.4651217
26. Hadsell R, Sermanet P, Ben J, Erkan A, Scoffier M, Kavukcuoglu K, et al. Learning long-range vision for autonomous off-road driving. J Field Robot. (2009) 26:120–44. doi: 10.1002/rob.20276
27. Oriol Vinyals, Alexander Toshev, Samy Bengio, Erhan D. Show and tell: a neural image caption generator. arXiv. (2015) https://arxiv.org/abs/1411.4555
28. Collobert R, Weston J, Bottou L, Karlen M, Kavukcuoglu K, Kuksa P. Natural language processing (almost) from scratch. J Mach Learn Res. (2011). 12:2493–537. doi: 10.5555/1953048.2078186
29. Bachmann LM, Puhan MA. ter Riet G, Bossuyt PM. Sample sizes of studies on diagnostic accuracy: literature survey. BMJ. (2006) 332:1127–9. doi: 10.1136/bmj.38793.637789.2F
30. Panch T, Pearson-Stuttard J, Greaves F, Atun R. Artificial intelligence: opportunities and risks for public health. Lancet Digit Health. (2019) 1:E13–4. doi: 10.1016/S2589-7500(19)30002-0
31. Wilson ML, Atun R, DeStigter K, Flanigan J, Fleming KA, Horton S, et al. The Lancet Commission on diagnostics: advancing equitable access to diagnostics. Lancet. (2019) 393:2018–20. doi: 10.1016/S0140-6736(19)31052-9
32. Van Calster B, Wynants L. Machine learning in medicine. N Engl J Med. (2019) 380:2588. doi: 10.1056/NEJMc1906060
33. Rajkomar A, Dean J, Kohane I. Machine learning in medicine. N Engl J Med. (2019) 380:1347–58. doi: 10.1056/NEJMra1814259
34. Beam AL, Kohane IS. Big data and machine learning in health care. JAMA. (2018) 319:1317–8. doi: 10.1001/jama.2017.18391
35. van Smeden M, Lash TL, Groenwold RHH. Reflection on modern methods: five myths about measurement error in epidemiological research. Int J Epidemiol. (2020) 49:338–47. doi: 10.1093/ije/dyz251
36. Miettinen OS, Bachmann LM, Steurer J. Towards scientific medicine: an information-age outlook. J Eval Clin Pract. (2008) 14:771–4. doi: 10.1111/j.1365-2753.2008.01078.x
37. Fisher LD. Advances in clinical trials in the twentieth century. Annu Rev Public Health. (1999) 20:109–24. doi: 10.1146/annurev.publhealth.20.1.109
38. Foote KD. A Brief History of Machine Learning. (2021). Available online at: https://wwwdataversitynet/a-brief-history-of-machine-learning/ (accessed December 23, 2021).
Keywords: artificial intelligence (AI), machine learning (ML), statistics, methodology, reporting guideline
Citation: Faes L, Sim DA, van Smeden M, Held U, Bossuyt PM and Bachmann LM (2022) Artificial Intelligence and Statistics: Just the Old Wine in New Wineskins? Front. Digit. Health 4:833912. doi: 10.3389/fdgth.2022.833912
Received: 14 December 2021; Accepted: 03 January 2022;
Published: 26 January 2022.
Edited by:
Florian B. Pokorny, Medical University of Graz, AustriaReviewed by:
Karthik Seetharam, West Virginia State University, United StatesCopyright © 2022 Faes, Sim, van Smeden, Held, Bossuyt and Bachmann. This is an open-access article distributed under the terms of the Creative Commons Attribution License (CC BY). The use, distribution or reproduction in other forums is permitted, provided the original author(s) and the copyright owner(s) are credited and that the original publication in this journal is cited, in accordance with accepted academic practice. No use, distribution or reproduction is permitted which does not comply with these terms.
*Correspondence: Livia Faes, bC5mYWVzQG5ocy5uZXQ=