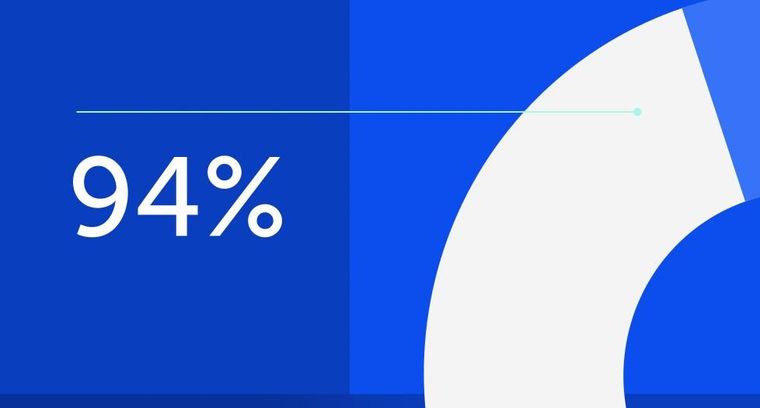
94% of researchers rate our articles as excellent or good
Learn more about the work of our research integrity team to safeguard the quality of each article we publish.
Find out more
BRIEF RESEARCH REPORT article
Front. Digit. Health, 09 July 2021
Sec. Health Informatics
Volume 3 - 2021 | https://doi.org/10.3389/fdgth.2021.704411
The spread of the current Sars-Cov-2 pandemics leads to the development of mutations that are constantly monitored because they could affect the efficacy of vaccines. Three recently identified mutated strains, known as variants of concern, are rapidly spreading worldwide. Here, we study possible effects of these mutations on the immune response to Sars-Cov-2 infection using NetTepi a computational method based on artificial neural networks that considers binding and stability of peptides obtained by proteasome degradation for widely represented HLA class I alleles present in human populations as well as the T-cell propensity of viral peptides that measures their immune response. Our results show variations in the number of potential highly ranked peptides ranging between 0 and 20% depending on the specific HLA allele. The results can be useful to design more specific vaccines.
The current COVID-19 pandemic is caused by the coronavirus SARS-CoV-2, one out of seven coronaviruses known to infect humans. Not all coronaviruses cause diseases of the same severity: SARS-CoV, MERS-CoV, and SARS-CoV-2 cause serious symptoms while HCoV-HKU1, HCoV-NL63, HCoV-OC43, and HCoV-229E only produce mild symptoms (1). In order to successfully infect the host, coronaviruses must overcome the innate and the adaptive immune system (2). The individual genetic susceptibility to viral infection is known to be affected by the Human Leukocyte Antigen (HLA) system or the Major Histocompatibility Complex (MHC), a very polymorphic region of the human genome (3). For example, H1N1 flu infection was shown to be correlated with several HLAs (4, 5) and HIV infection was more pronounced in individuals with HLA-A*29, HLA-B*35, and HLA-B*57 (6–11). Most importantly, an association between disease severity and HLA was also revealed for patients infected by SARS-CoV (12–16).
Because experimental characterization of neoantigens is costly and time-consuming, a growing effort has been devoted to developing computational methods that could estimate the binding of individual peptides to the MHC and predict the subsequent immune response. The class I regions are located on the most telomeric part of the human MHC and include 3 highly polymorphic HLA genes, known as classical (Class Ia: HLA-A, HLA-B, and HLA-C) and 3 lowly polymorphic HLA genes, known as non-classical (class Ib: HLA-E, HLA-F, and HLA-G) (17). After viral infection, viral peptides are produced in the cytosol from proteasome activity, bind to the HLA class I molecules and are then exposed to the cellular membrane. The immune response is triggered when CD8+ T cells recognize these peptide-HLA pairs (18, 19). In a recent paper (20), we identified a set of haplotypes that bind weakly and strongly to SARS-CoV-2 peptides and assessed their prevalence in specific human subpopulations (20).
The dissemination of the SARS-CoV-2 virus in the past few months, lead to the development of many genomic variants. The two major classifications have been produced by GISAID (https://www.gisaid.org/references/statements-clarifications/clade-and-lineage-nomenclature-aids-in-genomic-epidemiology-of-active-hcov-19-viruses/) and Nextstrain (https://nextstrain.org/ncov). Nextstrain, in particular, assigns nomenclature through the designation of SARS-CoV-2 clades to label well-defined clades that reached geographic spread with significant frequency (21). According to the GISAID classification, the virus that was first detected in Wuhan in December 2019 belongs to the L clade. The next important clade is the so-called S clade appearing at the beginning of 2020. From mid-January 2020 two new variants, known as the V and G variants, appeared and rapidly became prevalent across the world.
From early December 2020 a new viral lineage, known as B.1.1.7, appeared in the UK and spread extremely rapidly, due to its increased transmissibility and longer lasting infections (22). At about the same time, the second variant of SARS-CoV-2 known as 501Y.V2 (B.1.351 lineage) appeared in South Africa. The B.1.351 variant was reported by the WHO to possess increased transmission ability and higher viral load, although it is not clear if it is associated with more severe disease (https://www.who.int/csr/don/31-december-2020-sars-cov2-variants/en/). A third variant that is spreading across the world is the lineage P.1, also known as 20J/501Y.V3, Variant of Concern 202101/02 (VOC-202101/02) or colloquially known as the Brazilian variant. The P.1 variant has 17 unique amino acid changes, ten of which are located in the spike protein. Collectively, these three variants (B.1.1.7, B.1.351, and P.1) are known as variants of concern.
Here, we use supervised neural network machine learning approaches (23) to compute binding affinities, stability and T cell propensity for peptides derived by proteosome degradation (24) from the three variants of concern of SARS-CoV-2 and 13 common HLA alleles. Similar calculations are commonly performed to identify peptides for vaccine development (25). Our results allow studying the variations in potential T-cell epitopes due to the variants of concern.
The source code used to obtain the results in this paper are available at https://github.com/ComplexityBiosystems/hla-covid.
We downloaded the fasta sequence for SARS-CoV-2 (GenBank: MN908947.3). We obtained the mutated sequences by modifying the reference sequence according to the three variants of interest B.1.1.7, B.1.351, and P1. We restrict our analysis to the most abundant structural proteins (26): S,N,E,M. The resulting fasta sequences are reported as Supplementary Data.
To identify potential T cell epitopes, we use NetTepi 1.0 server (https://services.healthtech.dtu.dk/service.php?NetTepi-1.0) which combines estimates for peptide-MHC binding affinity, peptide-MHC stability, and T cell propensity (23). Peptides are then ranked against a set of 200,000 natural peptides to obtain a global rank score. Here we scan all SARS-Cov-2 peptides with lengths 8–11 from the 4 structural viral proteins and retain the peptides with rank scores lower than 2%. We perform the calculations for all the available class I MHC alleles using the default values for the relative weight on stability prediction and the relative weight on T cell propensity prediction. We only consider peptides that are likely to be produced by proteasome degradation. To this end, we employ NetChop 3.1 (24) a neural network based algorithm that scans proteins for probable cleavage sites of the human proteasome.
To investigate the variations in the T cell response to the SARS-CoV-2 variants of concern as compared with the reference virus, we use NetTepi (23), a neural network based software combining information of peptide-HLA binding, peptide-HLA stability and peptide T cell propensity. We consider the 13 HLA type I alleles available for this method, which are widely represented in human populations. In particular, the 6 HLA-A alleles are present in around 60% of the population, while the 7 HLA-B are present in around 30% of the population (20). As discussed in the Methods section, we only consider peptides that are most likely to result from proteasome degradation.
For each virus variant, we obtain a list of highly ranked peptides that are most likely to be potential epitopes recognized by T cells. We then compare these lists with the list obtained from the reference virus and count how many potential were already present in the reference virus (Figure 1A). Figure 1B shows that the total number of potential epitopes varies only slightly for different virus variants and slightly more when comparing different HLA alleles. As illustrated in Figure 1C, the percentage of new peptides not present in the reference virus varies in the range of 0–20% depending on the HLA allele. The lowest rate of variations is found for HLA-A26 for which all the potential epitopes were already present in the reference virus, while the highest variation rate is found for HLA-B39, with more than 20% of new epitopes.
Figure 1. Variation of the number of T cell epitopes in virus variants. (A) We distinguish potential epitopes in virus variants according to their presence in the reference Sars-Cov-2 virus genome. (B) The total number of highly ranked peptides for each allele is reported for each virus variant and each allele. (C) The fraction of highly ranked peptides that were not present in the reference genome is reported for each variant and each allele.
In Figure 2, we provide a more detailed picture of the variations in the score for the highly ranked peptides selected by NetTepi, considering binding affinity, peptide stability, and the combined score which also includes T-cell propensity. The results show that the main source of variations comes from the considered allele, while the range of values does not change significantly across the different mutations.
Figure 2. Variations of T cell epitope properties in virus variants. For the highly ranked peptides selected by NetTepi we report the boxplots for (A) binding affinity, (B) peptide stability, and (C) the combined score also including T cell propensity. Data are reported for different virus variants and alleles.
In Figure 3 we report the protein localization of highly ranked peptides. Notice that most highly ranked peptides are located in the spike protein for all virus variants. We have also checked the localization of the new epitopes, not present in the reference virus. We found that virtually all the new epitopes are located in the spike protein, with a single exception of the P1 variant where one peptide stems from the mutated envelope protein.
Figure 3. Distribution of highly ranked peptides across viral proteins. (A) Reference virus, (B) B1.1.7 (C) P1, and (D) B1.351.
Coronaviruses represent a broad class of viruses infecting humans through the upper respiratory tract and causing diseases with varying severity from common cold to flu-like diseases. SARS-CoV-2 has rapidly spread worldwide and has lead to thousands of mutations in a relatively short time, despite its low mutation rate. While most of these mutations do not carry any practical effect on the infection capability of the virus, some mutation can acquire higher transmissibility, the ability to better evade the immune system and stronger drug resistance (27–29). Three of these mutated strains, known as variants of concern (B.1.1.7, B.1.351, and P.1), have emerged and spread worldwide. Understanding the impact of mutations on viral infectivity and antigenicity is thus becoming a very pressing question (30). A recent paper showed that these mutations have only a small effect on SARS-CoV-2-specific CD4+ and CD8+ T cell responses in patients infected with the three virus variants (31).
In a recent paper (20), we have investigated the possible role of HLA type I polymorphism in SARS-CoV-2 susceptibility and we identified a set of peptides that were able to bind with high affinity a specific set of HLA type I alleles. We then studied the distribution of the relevant HLA type I alleles across human populations (20). Our conclusion was that the immune response may depend on the specific HLA class I haplotype of the infected subject. Therefore it is important to study the immune response to SARS-CoV-2 variants in an HLA-type I-dependent fashion.
In the present paper, we perform a computational analysis of the immune response to SARS-CoV-2 variants as compared with the original reference virus. Our results show that the number of potential peptides presented by HLA to T-cells varies depending on the HLA type I allele. While for some HLA class I alleles there is no change in the variant peptides with respect to the peptides in the reference virus, for some other HLA class I alleles the variation can be relatively large reaching more than 20% of the total. Our strategy can help screen for vaccine candidates that are robust against mutation. To design an effective vaccine, it is necessary to select peptides that can be presented to T cells by a range of HLAs that are broadly distributed in human populations. With our strategy one could also assess in silico if the peptides are still able to bind to HLAs when mutated.
The original contributions presented in the study are included in the article/Supplementary Material, further inquiries can be directed to the corresponding author/s.
CL and SZ designed and performed research and wrote the paper.
The authors declare that the research was conducted in the absence of any commercial or financial relationships that could be construed as a potential conflict of interest.
The Supplementary Material for this article can be found online at: https://www.frontiersin.org/articles/10.3389/fdgth.2021.704411/full#supplementary-material
1. Corman VM, Muth D, Niemeyer D, Drosten C. Hosts and Sources of Endemic Human Coronaviruses. Adv Virus Res. (2018) 100:163–88. doi: 10.1016/bs.aivir.2018.01.001
2. Mandl JN, Ahmed R, Barreiro LB, Daszak P, Epstein JH, Virgin HW, et al. Reservoir host immune responses to emerging zoonotic viruses. Cell. (2015) 160:20–35. doi: 10.1016/j.cell.2014.12.003
3. Dendrou CA, Petersen J, Rossjohn J, Fugger L. HLA variation and disease. Nat Rev Immunol. (2018) 18:325. doi: 10.1038/nri.2017.143
4. Falfán-Valencia R, Narayanankutty A, Reséndiz-Hernández JM, Pérez-Rubio G, Ramírez-Venegas A, Nava-Quiroz KJ, et al. An increased frequency in HLA Class I alleles and haplotypes suggests genetic susceptibility to influenza A (H1N1) 2009 pandemic: a case-control study. J Immunol Res. (2018) 2018:3174868. doi: 10.1155/2018/3174868
5. Luckey D, Weaver EA, Osborne DG, Billadeau DD, Taneja V. Immunity to Influenza is dependent on MHC II polymorphism: study with 2 HLA transgenic strains. Sci Rep. (2019) 9:1–10. doi: 10.1038/s41598-019-55503-1
6. Hill AV. The immunogenetics of human infectious diseases. Annu Rev. Immunol. (1998) 16:593–617. doi: 10.1146/annurev.immunol.16.1.593
7. Mallal S, Nolan D, Witt C, Masel G, Martin A, Moore C, et al. Association between presence of HLA-B* 5701, HLA-DR7, and HLA-DQ3 and hypersensitivity to HIV-1 reverse-transcriptase inhibitor abacavir. Lancet. (2002) 359:727–32. doi: 10.1016/S0140-6736(02)07873-X
8. Carrington M, Nelson GW, Martin MP, Kissner T, Vlahov D, Goedert JJ, et al. HLA and HIV-1: heterozygote advantage and B* 35-Cw* 04 disadvantage. Science. (1999) 283:1748–52. doi: 10.1126/science.283.5408.1748
9. Goulder PJ, Watkins DI. Impact of MHC class I diversity on immune control of immunodeficiency virus replication. Nat Rev Immunol. (2008) 8:619–30. doi: 10.1038/nri2357
10. Mekue LM, Nkenfou CN, Ndukong E, Yatchou L, Dambaya B, Ngoufack MN, et al. HLA A* 32 is associated to HIV acquisition while B* 44 and B* 53 are associated with protection against HIV acquisition in perinatally exposed infants. BMC Pediatr. (2019) 19:249. doi: 10.1186/s12887-019-1620-6
11. Valenzuela-Ponce H, Alva-Hernández S, Garrido-Rodríguez D, Soto-Nava M, García-Téllez T, Escamilla-Gómez T, et al. Novel HLA class I associations with HIV-1 control in a unique genetically admixed population. Sci Rep. (2018) 8:1–17. doi: 10.1038/s41598-018-23849-7
12. Lin M, Tseng HK, Trejaut JA, Lee HL, Loo JH, Chu CC, et al. Association of HLA class I with severe acute respiratory syndrome coronavirus infection. BMC Med Genet. (2003) 4:9. doi: 10.1186/1471-2350-4-9
13. Ng MH, Lau KM, Li L, Cheng SH, Chan WY, Hui PK, et al. Association of human-leukocyte-antigen class I (B* 0703) and class II (DRB1* 0301) genotypes with susceptibility and resistance to the development of severe acute respiratory syndrome. J Infect Dis. (2004) 190:515–8. doi: 10.1086/421523
14. Chen YMA, Liang SY, Shih YP, Chen CY, Lee YM, Chang L, et al. Epidemiological and genetic correlates of severe acute respiratory syndrome coronavirus infection in the hospital with the highest nosocomial infection rate in Taiwan in 2003. J Clin Microbiol. (2006) 44:359–65. doi: 10.1128/JCM.44.2.359-365.2006
15. Keicho N, Itoyama S, Kashiwase K, Phi NC, Long HT, Ha LD, et al. Association of human leukocyte antigen class II alleles with severe acute respiratory syndrome in the Vietnamese population. Hum Immunol. (2009) 70:527–31. doi: 10.1016/j.humimm.2009.05.006
16. Spínola H. HLA loci and respiratory infectious diseases. J Respir Res. (2016) 2:56–66. doi: 10.17554/j.issn.2412-2424.2016.02.15
17. Shiina T, Hosomichi K, Inoko H, Kulski JK. The HLA genomic loci map: expression, interaction, diversity and disease. J Hum Genet. (2009) 54:15–39. doi: 10.1038/jhg.2008.5
18. Maffei A, Papadopoulos K, Harris PE. MHC class I antigen processing pathways. Hum Immunol. (1997) 54:91–103. doi: 10.1016/S0198-8859(97)00084-0
19. Goldberg AC, Rizzo LV. MHC structure and function - antigen presentation. Part 2. Einstein. (2015) 13:157–62. doi: 10.1590/S1679-45082015RB3123
20. La Porta CAM, Zapperi S. Estimating the binding of Sars-CoV-2 peptides to HLA class I in human subpopulations using artificial neural networks. Cell Syst. (2020) 11:412–7.e2. doi: 10.1016/j.cels.2020.08.011
21. Hadfield J, Megill C, Bell SM, Huddleston J, Potter B, Callender C, et al. Nextstrain: real-time tracking of pathogen evolution. Bioinformatics. (2018) 34:4121–3. doi: 10.1093/bioinformatics/bty407
22. Kissler SM, Fauver JR, Mack C, Tai C, Breban M, Watkins AE, et al. Densely sampled viral trajectories suggest longer duration of acute infection with B. 1.1. 7 variant relative to non-B. 1.1. 7 SARS-CoV-2. medRxiv [preprint]. (2021). doi: 10.1101/2021.02.16.21251535
23. Trolle T, Nielsen M. NetTepi: an integrated method for the prediction of T cell epitopes. Immunogenetics. (2014) 66:449–56. doi: 10.1007/s00251-014-0779-0
24. Nielsen M, Lundegaard C, Lund O, Keşmir C. The role of the proteasome in generating cytotoxic T-cell epitopes: insights obtained from improved predictions of proteasomal cleavage. Immunogenetics. (2005) 57:33–41. doi: 10.1007/s00251-005-0781-7
25. Campbell KM, Steiner G, Wells DK, Ribas A, Kalbasi A. Prediction of SARS-CoV-2 epitopes across 9360 HLA class I alleles. bioRxiv [preprint]. (2020). doi: 10.1158/1557-3265.COVID-19-S03-01
26. Bar-On YM, Flamholz A, Phillips R, Milo R. SARS-CoV-2 (COVID-19) by the numbers. Elife. (2020) 9:e57309. doi: 10.7554/eLife.57309
27. Callaway E. Making sense of coronavirus mutations. Nature. (2020) 585:174–7. doi: 10.1038/d41586-020-02544-6
28. Padhi AK, Tripathi T. Can SARS-CoV-2 accumulate mutations in the S-protein to increase pathogenicity? ACS Pharmacol Transl Sci. (2020) 3:1023–6. doi: 10.1021/acsptsci.0c00113
29. Padhi AK, Kalita P, Zhang KY, Tripathi T. High throughput designing and mutational mapping of RBD-ACE2 interface guide non-conventional therapeutic strategies for COVID-19. BioRxiv [preprint]. (2020). doi: 10.1101/2020.05.19.104042
30. Li Q, Wu J, Nie J, Zhang L, Hao H, Liu S, et al. The Impact of Mutations in SARS-CoV-2 Spike on Viral Infectivity and Antigenicity. Cell. (2020) 182:1284–94.e9. doi: 10.1016/j.cell.2020.07.012
Keywords: SARS-CoV-2, T cells, MHC, polymorphism, virus mutation
Citation: La Porta CAM and Zapperi S (2021) Immune Profile of SARS-CoV-2 Variants of Concern. Front. Digit. Health 3:704411. doi: 10.3389/fdgth.2021.704411
Received: 02 May 2021; Accepted: 17 June 2021;
Published: 09 July 2021.
Edited by:
Daihai He, Hong Kong Polytechnic University, Hong KongReviewed by:
Hao Wang, Shenzhen University General Hospital, ChinaCopyright © 2021 La Porta and Zapperi. This is an open-access article distributed under the terms of the Creative Commons Attribution License (CC BY). The use, distribution or reproduction in other forums is permitted, provided the original author(s) and the copyright owner(s) are credited and that the original publication in this journal is cited, in accordance with accepted academic practice. No use, distribution or reproduction is permitted which does not comply with these terms.
*Correspondence: Caterina A. M. La Porta, Y2F0ZXJpbmEubGFwb3J0YUB1bmltaS5pdA==
Disclaimer: All claims expressed in this article are solely those of the authors and do not necessarily represent those of their affiliated organizations, or those of the publisher, the editors and the reviewers. Any product that may be evaluated in this article or claim that may be made by its manufacturer is not guaranteed or endorsed by the publisher.
Research integrity at Frontiers
Learn more about the work of our research integrity team to safeguard the quality of each article we publish.