- 1The Boston Consulting Group, Boston, MA, United States
- 2International Consortium for Health Outcome Measurement, London, United Kingdom
- 3Nictiz, The Hague, Netherlands
- 4The Boston Consulting Group, Stockholm, Sweden
- 5University of Utah Department of Biomedical Informatics, Intermountain Health Care, Salt Lake City, UT, United States
- 6Department of Clinical Neuroscience, Karolinska Institutet, Solna, Sweden
- 7Department of Clinical Neuroradiology, Karolinska University Hospital, Solna, Sweden
Objectives: To update the sets of patient-centric outcomes measures (“standard-sets”) developed by the not-for-profit organization ICHOM to become more readily applicable in patients with multimorbidity and to facilitate their implementation in health information systems. To that end we set out to (i) harmonize measures previously defined separately for different conditions, (ii) create clinical information models from the measures, and (iii) restructure the annotation to make the sets machine-readable.
Materials and Methods: First, we harmonized the semantic meaning of individual measures across all the 28 standard-sets published to date, in a harmonized measure repository. Second, measures corresponding to four conditions (Breast cancer, Cataracts, Inflammatory bowel disease and Heart failure) were expressed as logical models and mapped to reference terminologies in a pilot study.
Results: The harmonization of semantic meaning resulted in a consolidation of measures used across the standard-sets by 15%, from 3,178 to 2,712. These were all converted into a machine-readable format. 61% of the measures in the 4 pilot sets were bound to existing concepts in either SNOMED CT or LOINC.
Discussion: The harmonization of ICHOM measures across conditions is expected to increase the applicability of ICHOM standard-sets to multi-morbid patients, as well as facilitate their implementation in health information systems.
Conclusion: Harmonizing the ICHOM measures and making them machine-readable is expected to expedite the global adoption of systematic and interoperable outcomes measurement. In turn, we hope that the improved transparency on health outcomes that follows will let health systems across the globe learn from each other to the ultimate benefit of patients.
Background
Value-based healthcare aims to transform care by aligning different stakeholders to focus on patient centric outcomes. Instead of promoting volumes of care services, value-based health care incentivizes value (1–4), with value defined as health outcomes per unit cost for defined conditions over the full cycle of care (1). As the definition of outcomes will impact behavior in value-based contracting, it is paramount that outcomes capture the circumstances most relevant to patients (1). Additionally, the validity of outcomes measurement requires consistent and objective measurement across sites (1, 5–7), which emphasizes the need for high-quality data.
Efforts to systematically collect outcomes measures along the continuum of care are laborious and in some cases constitute questionable use of clinical resources (8–11). Therefore, leveraging routinely collected data for secondary use in quality measurement is growing in popularity (12, 13). Data quality issues (6, 14, 15) and challenges in repurposing data have caused concerns on validity (6, 14) of the wealth of data available in clinical information systems (16). The latter is frequently discussed in relation to electronic health records (EHRs), which many argue are tailored to support billing processes, rather than quality improvement efforts. Another challenge to secondary use of data is fragmentation across organizations and applications with low interoperability (8, 14, 17–19). This could partly be explained by the influence of individual organizations on health data infrastructure decisions, and frequently results in an organization-centric data architecture, aggravated by business models that make it challenging for healthcare organizations to change provider of health information systems (vendor lock-in) (19). The resulting challenges in extracting, sharing and validly analyzing data remain a barrier to standardized and cost-effective quality measurement (7). Such lack of interoperability may also explain why the impact of digital technology on healthcare systems remains equivocal (17, 19–21), despite having been projected to partly “replace the intellectual functions of the physician” 50 years ago (22).
Also in case of sufficient technical- and legal interoperability to allow sharing of data across systems, profound variability in local capture patterns, standards usage and documentation processes render data incomparable due to different structure and meaning (lack of structural- and semantic interoperability) (7, 8, 23). As interoperability standards allow the normalization of data originating from different sources to a common structure and meaning (6, 23, 24), standards have been proposed as a potential solution to the interoperability challenge. However, additional complexity arises as the successful use of interoperability standards requires valid mapping to source data (7), emphasizing the need for interoperability standards with well-defined elements that are easy to implement.
While condition-centric quality registries have promoted quality and procedure development in healthcare to date (25), a mechanism for timely feedback to healthcare processes is required to fully enable a continuously learning healthcare system (26). In contrast to condition-focused quality measures that have been widely implemented to date, measuring patient centric value in healthcare requires the ability to assess patients suffering from multiple conditions (27).
Setting out to achieve the goal of a global, patient-centric health outcomes standard, two of the coauthors (SL, MI) together with Professor Michael Porter from Harvard Business School founded the non-profit international consortium for health outcomes measurement (ICHOM) in 2012 (28). Since its foundation, ICHOM has convened groups of clinical experts and patients from across the globe to define collections of standard outcome measures and risk factors (“standard-sets”) for conditions covering almost 50% of the global disease burden, using a structured consensus process (29). In addition to measures, which are designed to be patient-centric and focus on aspects of health that matter most to the patient, ICHOM standard-sets provide guidance for measurement (e.g., time points, inclusion criteria etc.).
The patient-centeredness and global footprint of the ICHOM standard-sets contrast several other efforts to define quality metrics in healthcare. Committed to ensure sufficient depth of analysis, ICHOM deliberately deploys a condition-centric approach to developing the standard-sets. However, the high level of independence of individual expert groups resulted in insufficient harmonization of measures, and several examples exist where identical measures occur in different standard-sets but are labeled with different unique identifiers, as well as examples of identical unique identifiers mapping to measures of differing meaning. One example of this issue is illustrate by the measure “HORMONTX,” which originally referred to hormonal therapy other than androgen deprivation therapy in the advanced prostate cancer standard-set, but to the intent of hormonal therapy in the breast cancer set. Such ambiguities magnify implementation challenges in health information systems (7) and add to the registration-burden for patients with multimorbidity, who frequently are subject to measurement following multiple standard-sets.
Objective
Aiming to reduce the burden of implementing ICHOM standard-sets (30), reduce the patient registration-burden and facilitate future alignment with emerging health data standards, the authors set out to harmonize the ICHOM measures and create a repository of measures with well-defined meaning and little redundancy.
Methods
The harmonization work was executed in two phases, the first of which focused on harmonizing the semantic meaning of measures across all the 28 standard-sets published to date. In the second phase, measures corresponding to four pilot conditions [Breast cancer (31), Cataracts (32), Inflammatory bowel disease (33), and Heart failure (34)] were expressed as logical models and mapped to reference terminologies in a pilot study, which is expected to further facilitate data collection and analysis (35).
Approaching Harmonization
The overall process for harmonizing the ICHOM measures is depicted in Figure 1, and draws on learnings from the field of clinical information modeling (36).
In addition to harmonizing the ICHOM measures across different standard-sets, particular care was taken to specify measure definitions unambiguously, while removing any contextual information specific to individual standard-sets (e.g., regarding timing of measurement, inclusion criteria etc.). Such information is instead expressed in separate execution-logic files (ongoing). This approach of separating the ICHOM information model into two layers aims to increase the recyclability of measures across different conditions and thus facilitate implementation as well as the creation of new standard-sets. Note that measure definitions were iterated as the work progressed, with final definitions agreed on at the end of phase 1 occurring at a point in time after phase 2 was concluded.
Phase 1–Harmonizing ICHOM Measures Across the 28 Published Sets
The harmonization of ICHOM measures followed the numeric sequence depicted below.
1. Requirement specification. Standard-sets of measures covering 28 different conditions were already defined and published at www.ichom.org. These measures have defining attributes depicted below, which are further detailed in the published ICHOM implementation guides (37).
• Variable ID. Human-readable unique identifier of the measure.
• Item. Human-readable short description of the measure.
• Definition. Detailed definition of the measure.
• Supporting definition. Further detailed definition of the measure.
• Inclusion criteria. Patient population subject to measurement.
• Timing. Timing of the measurement.
• Type. The required measure data type.
• Response options. Response options that are allowed in response to the measure.
• Reporting source. The primary source of the information to populate the measure.
2. Analyzing condition-specific information. Published ICHOM implementation guides and data dictionaries (all available on www.ichom.org, comprehensive list of conditions available in the Appendix) were studied at the outset of the pilot. Extended search for information was employed to provide additional context where needed.
3. Indexing the ICHOM measures. All measures from the 28 published standard-sets were read into a common data structure in the programming language Python (version 3.7.5). After basic formatting, the resulting data dictionary was indexed at the “concept-usage” level (i.e., at the level of the single prescribed ICHOM measure). This was done as to circumvent the indexing issues related to the ambiguous unique identifiers (attribute “Variable ID”) and semantic meanings that were described in the background section. Each row in the resulting dictionary consisted of a unique combination of the attributes “Variable ID,” “Item,” “Definition,” “Supporting definition,” “Response options,” and a unique identifier of each standard-set.
4. Identifying measures to harmonize. Differences in semantic meaning of measures across standard-sets were manually assessed by one of the authors (MB). Concept-usages that were judged to be similar enough (different Variable ID with overlapping defining attributes), or different enough (identical Variable ID with differing defining attributes) to be candidates for harmonization were highlighted with a flag and validated by a committee (consensus) consisting of three authors (MB, MK, MI).
5. Clustering related concept-usages into groups. Concept-usages assessed as sharing sufficient semantic meaning to warrant consolidation onto a common updated measure (following expert-based method outlined in step 4), were clustered into groups with a human-readable name. All concept-usages from across the 28 conditions (N = 3,421) were considered simultaneously, with the concept-usages touching the four pilot conditions subject to an additional step (phase 2 below).
6. Consolidating related concept-usages onto updated measures. The concept-usages in each of the related groups generated in step 5 were outputted to spreadsheets and manually consolidated onto single measures defined to cover all concept-usages in a group. In a following step, updated measures were specified using the same defining attributes as described under step 3.
7. Defining ICHOM taxonomy of measures. A taxonomy was developed for the ICHOM measures, to provide a hierarchical structure and facilitate the identification and retrieval of relevant measures from the ICHOM measure-repository. The final taxonomy was organized in two levels, with the first being based on the PICO-model for evidence-based decisions (38) (Figure 2).
8. Converting the ICHOM measure-repository to a machine-readable representation. The measure-repository was converted to JSON format in order to enable reliable versioning. Indexing of response options mapping to each updated measure was also harmonized across sets.
9. Defining standard-set execution-logic. A specification of which measures to include for each condition, along with the prescribed timing for measurement, inclusion criteria etc., was defined in a separate resource for each standard-set (ongoing), by transcribing the qualitative information in the implementation guides available at www.ichom.org into a machine readable file following an IF-THEN logic. The specification follows as closely as possible the original recommendations depicted in the implementation guides.
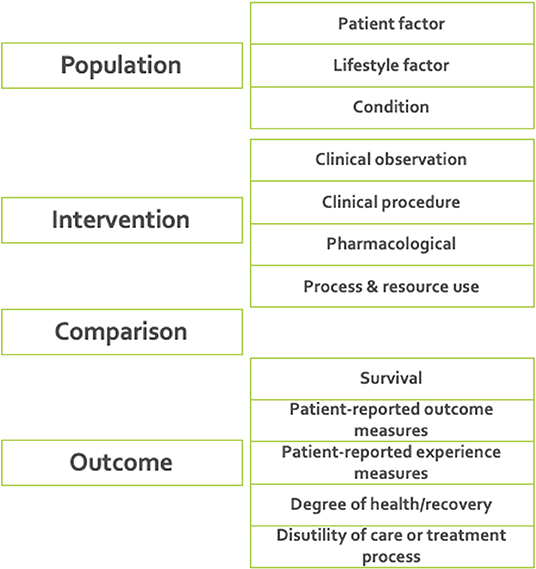
Figure 2. The final taxonomy was organized in two levels, with the first being based on the PICO-model for evidence-based decisions (38). The taxonomy was implemented as a tool for support of further developments including standard-sets for new diagnoses.
Phase 2–Defining Logical Models and Terminology
Enhancing Related Groups Using Advanced Analytics
To supplement the manual clustering of related concept-usages into groups, phase 2 leveraged advanced analytics to identify additional concept-usages related to those included in the four pilot conditions. A total of 361 concept-usages from the four pilot conditions had been manually clustered into related groups in phase 1 step 4-5. A supervised deep-learning model was then developed to assess concept-usages from across all 28 standard-sets for relatedness to the related groups “touching” the four pilot conditions. The approach included creating semantic embeddings from manually labeled concept-usages (attributes “item,” “definition,” “supporting definition,” and “response options”) that were part of the related groups touching the four pilot conditions, using the FastText algorithm (39). The FastText model is based on the skipgram model, which represents each word as a bag of character n-grams. Words are represented as the sum of these representations, which makes the method fast and allows for computing representations of words not appearing in the training set. In contrast to the traditional skipgram model, FastText deploys a scoring function which takes into account information on the internal structure of words. Context is represented as a bag of the words contained in a fixed size window around the target word. The model deployed in our case was pre-trained on the English Wikipedia using continuous bag of words (CBOW) with position-weights, dimension 300 and 5-length character n-grams. The model was freely available from the FastText GitHub repository (40). Semantic embeddings and their corresponding group labels were fed as inputs to train a supervised Neural Network (MLP Classifier) in Python scikit-learn 0.20.3. A hyperparameter search (three-fold cross-validation, overall accuracy as optimization objective) was conducted before training, to fine-tune model performance. The final model was trained on a random 67% of the observations and evaluated on the remainder 33%. After training, it was subsequently applied to semantic embeddings of all concept usages from across the standard-sets (N = 3,421) and used to assign each concept usage a group label from either of the groups touching the pilot conditions. These automatically assigned labels were manually validated by one of the authors (MB). In total, 391 additional concept usages from across all the 28 standard-sets were included in the related groups touching the four pilot conditions, resulting in a total of 752 concept-usages being clustered into these groups. The related groups were converted to XML-format and uploaded to a clinical information modeling platform (41).
Clinical Information Modeling
Logical models were defined to cover each group included in the pilot conditions (analogous to phase 1 step 6) and bound to reference terminologies (below). Measures were defined in agreement between three of the authors (MB, MK, MI) and the internal ICHOM standard-set development team, with input from informatics experts at one of the contributing organizations (NICTIZ). The taxonomy-category assigned to a measure guided the modeling approach and the “Response option” attribute guided the specification of value sets to describe which values are allowed in response to each measure (6).
Terminology Binding
The measures (including value sets) touching the four pilot conditions were bound to terminology by expert terminologists. Terminologies were deliberately constrained to the international coding systems SNOMED CT (42) and LOINC (43). Measures were defined in a technology-agnostic and human-readable format, and mapped to terminologies only when good matches were available. This design-choice is expected to facilitate re-mappings that may become necessary as technical formats and terminologies evolve (35) as well as promote backwards compatibility with the original versions of the standard-sets.
Technical Expert Peer-Review
Upon completion, the draft logical models, value sets and terminology bindings were distributed to individual peer-reviewers in the ICHOM network for review (please see section Acknowledgments). Guidance concerning harmonizing the ICHOM logical models with already available external standards (e.g., FHIR implementation guides), was not always followed, due to a perceived threat to backwards compatibility posed by making ICHOM dependent on independently evolving external standards.
Endorsement of Updated Measures by Clinical Expert Groups
After obtaining input from technical peer-reviewers and the chairpersons of the clinical expert groups that developed the original versions of the pilot standard-sets, proposed updates to each of the four sets were circulated to each clinical expert group for review and endorsement. This step is still ongoing for the remaining 24 conditions.
The ICHOM Measure-Repository
By expressing each measure in a defined syntax, the team aimed to facilitate future creation of computable model serializations (35) (instantiation artifacts) from both measures and execution-logic. While the exact format for these artifacts is not yet agreed, FHIR is a viable candidate (44).
In addition to the attributes defining ICHOM measures prior to harmonization, each measure in the ICHOM measure-repository was enhanced by a set of additional attributes (below) to facilitate versioning and linking to the standard-set specific execution logic files:
• TERM_ID. Numeric unique identifier of the measure.
• Value domains. Standard data type corresponding to the measure.
• Concept external code. Unique code in a reference terminology, corresponding to the measure.
• Concept external name. Unique name in a reference terminology, corresponding to the measure.
• Concept reference terminology. Reference terminology referred to by attributes “concept external code” and “concept external name”.
• Displayed value. Patient-friendly definition of the measure.
• Taxonomy category. Category in the ICHOM taxonomy that the measure belongs to.
• Timestamp. Timepoint of data recording.
The defining attributes of measures in the measure repository intentionally do not cover information related to the execution of measurement, which is expressed in execution-logic separately defined for each standard-set. The annotation was complemented to improve this. Note that measure definitions may be further modified, as ICHOM expert groups are engaged for feedback.
Results
The harmonization of the ICHOM measures for the 28 standard-sets resulted in 3,421 original concept-usages (3,178 unique measures) being consolidated onto harmonized measures. In total, 1,081 concept-usages were consolidated into updated measures that replaced two or more of the original concept-usages. The final repository of unique measures contained 2,712 measures, of which 11 were introduced as part of the harmonization process. Of the harmonized measures, 333 touched the four pilot conditions of the second phase. Thus, the creation of the harmonized global ICHOM repository and mappings resulted in a consolidation of the total number of measures by 15%, from 3,178 to 2,712 and all converted to a machine-readable state.
The deep-learning exercise (step 1, phase 2) aiming to improve grouping of the concept usages related to the pilot conditions, resulted in an 84.9% overall accuracy in predicting the correct cluster for a concept-usage, on the test-set.
The clinical information modeling (point 2, phase 2) resulted in 614 unique entities (each analogous to one response option) from across all value sets being linked to the 333 harmonized measures touching the pilot conditions.
Terminology binding (point 3, phase 2) resulted in 399 of the 614 value set items and 182 of the 333 harmonized measures touching the pilot conditions being readily mappable to SNOMED CT or LOINC. This yielded an overall mappable ratio of (399+182)/(614+333) = 61.4%.
Relevant feedback from the expert groups (point 5) was incorporated in the updated measures, again adhering to the ICHOM consensus process, and a total of 9 new measures were introduced by request from the expert groups, across the pilot conditions.
Discussion
By harmonizing the ICHOM measures, giving them unambiguous definitions and expressing them in a versioned, machine-readable repository we have laid the foundation to expedited integration and implementation of ICHOM health outcomes measurement in clinical information systems. The effects of the harmonization will be particularly notable to patients with multimorbidity and to partners aiming at implementing multiple sets, as harmonizing measures across diagnoses decreases redundancy in measures to be recorded. Harmonization also reduces the risk of conflicting meaning and format of measures, as well as offering new opportunities in risk-adjusting quality measures across diagnoses.
Packaging the proceedings in a versioned, global measure repository also makes it easier to maintain backwards compatibility of measures in the face of the periodic updates that are necessary to keep the standard up to date. Given its associated metadata, human-readable definitions and terminology layer, the measure repository is designed to support the extraction, transform and load (ETL) processes required to enable secondary use of routinely collected data across multiple conditions and applications. Although several initiatives aiming at achieving standardization of meaning in healthcare data exist, we argue that ICHOM is unique in its global and patient-centric approach to defining health outcomes, as well as in prescribing a sequence of measurement required to create transparency on health outcomes.
Although the work reported in this paper aims to make standardized health outcomes measurement easier to implement, additional steps are required to enable a learning healthcare system that would achieve the systematic feedback-loops that start to emerge in the context of other industries, e.g., industry 4.0 (45). One such step is the comparison of performance across providers with subsequent sharing of identified best practices (5), collectively referred to as benchmarking. In turn, the benchmarking process has potential to fuel a virtuous cycle in which organizational learning precipitates repeated performance improvements and innovations. For this to work, the benchmarking process must be connected to an ability to apply learnings in the organization (26, 46). The implementation of benchmarking in healthcare typically requires careful consideration of processes for data collection, as well as the importance of selecting appropriate reference points (benchmarks) (46–49).
Benchmarking has traditionally been considered a management tool, but the importance of engaging clinical staff is increasingly recognized as key to understand practice (46), sources of variation and to create change (26, 27).
It is primarily the desire to involve clinicians that made us opt to maintain definitions sparse and human readable, despite that decomposition of complex ICHOM measures into simpler constituents could result in higher mapping-rates to reference terminologies. The project Steering Committee also decided to limit such post-coordination (23) to prevent overly relying on external terminologies that would make the standard susceptible to semantic drift as terminologies evolve (7). This rationale is also a leading cause of why we have not opted to construct exhaustive clinical information models to cover informational needs outside what was already specified in the original standard-sets.
While the reported work aims to improve the quality of the data collection process, it does not fully address standardized data analysis and benchmarking at scale. In response to this need, work is underway to develop a common data model (CDM) to support distributed analytics, which will be reported separately.
Conclusion
Standardized health outcomes measurement and the interoperability it brings are key enablers of a patient-centric, learning healthcare system that operates across organizational- and specialty boundaries. By harmonizing the ICHOM measures across conditions and making them machine-readable, we aim to create part of the foundation necessary to enable large-scale collection of high-quality data suitable for quality benchmarking, that can bring about this change.
Data Availability Statement
The datasets presented in this study can be found in online repositories. The names of the repository/repositories and accession number(s) can be found below: www.ichom.org.
Author Contributions
MB, SL, SH, MI: concept. MB, BV-L, HH, MI: execution. MB, MI: initial writing. All authors: have contributed to the design and assessment of results of the study as well as read and approved the manuscript.
Funding
The authors declare that this study received in-kind funding for the time allotted to the project by the co-authors MB and SL from Boston Consulting Group. The funder was not involved in the study design, collection, analysis, interpretation of data, the writing of this article or the decision to submit it for publication. MI received partial support from the Swedish Government agency Vinnova for part of this work.
Conflict of Interest
MI and SL are co-founders and members of the board of ICHOM and MK was an employee of ICHOM during the completion of this work.
The remaining authors declare that the research was conducted in the absence of any commercial or financial relationships that could be construed as a potential conflict of interest.
Acknowledgments
The authors wish to thank the following organizations (alphabetical order) for contributions to the study: NICTIZ, The Boston Consulting Group, The Dutch Ministry of Health (MINVWS). We also want to thank the following individuals for contributing to the work through representation in the pilot project steering committee (alphabetical order): GCC Molenaar, Jan-Eric Slot, Ron Roozendaal. At last, we wish to thank the following individuals for their contributions to the technical peer-review of the work: Harold Solbrig, Sarah Riepenhausen, Swapna Abhyankar and Yan Heras.
References
1. Porter ME. What is value in health care. N Engl J Med. (2010) 363:2477–81. doi: 10.1056/NEJMp1011024
2. Lee TH. Putting the value framework to work. N Engl J Med. (2010) 363:2481–3. doi: 10.1056/NEJMp1013111
3. Feeley TW, and Mohta NS. New Marketplace Survey: Transitioning Payment Models: Fee-for-Service to Value-Based Care. Boston, MA: NEJM Catalyst. (2018) 4.
5. Porter ME, Larsson S, and Lee TH. Standardizing patient outcomes measurement. N Engl J Med. (2016) 374:504–6. doi: 10.1056/NEJMp1511701
6. D'Amore JD, Li C, McCrary L, Niloff JM, Sittig DF, McCoy AB, et al. Using clinical data standards to measure quality: a new approach. Appl Clin Inform. (2018) 9:422–31. doi: 10.1055/s-0038-1656548
7. McClure RC, Macumber CL, Skapik JL, and Smith AM. Igniting harmonized digital clinical quality measurement through terminology, CQL, and FHIR. Appl Clin Inform. (2020) 11:023–33. doi: 10.1055/s-0039-3402755
8. Rajkomar A, Dean J, and Kohane I. Machine learning in medicine. N Engl J Med. (2019) 380:1347–58. doi: 10.1056/NEJMra1814259
9. Wilensky G. The need to simplify measuring quality in health care. JAMA. (2018) 319:2369–70. doi: 10.1001/jama.2018.6858
10. Dunlap NE, Ballard DJ, Cherry RA, Dunagan WC, Ferniany W, Hamilton AC, et al. Observations from the field: reporting quality metrics in health care. In: NAM Perspectives. Discussion Paper, National Academy of Medicine. Washington, DC (2016). doi: 10.31478/201607e
11. Erb N, Zhang S, and Sikka R. Leading Health Care Transformation: A Primer for Clinical Leaders. New York, NY: CRC Press. (2015)
12. Safavi KC, Bates DW, and Chaguturu SK. Harnessing emerging information technology for bundled payment care using a value-driven framework. NEJM Catalyst. (2019) 5. doi: 10.1056/CAT.19.0701
13. Jha AK, and Classen DC. Getting moving on patient safety—harnessing electronic data for safer care. N Engl J Med. (2011) 365:1756–8. doi: 10.1056/NEJMp1109398
14. Hersh WR, Weiner MG, Embi PJ, Logan JR, Payne PR, Bernstam EV, et al. Caveats for the use of operational electronic health record data in comparative effectiveness research. Med Care. (2013) 51:S30. doi: 10.1097/MLR.0b013e31829b1dbd
15. Johnson SG, Speedie S, Simon G, Kumar V, and Westra BL. Quantifying the effect of data quality on the validity of an eMeasure. Appl Clin Inform. (2017) 8:1012–21. doi: 10.4338/ACI-2017-03-RA-0042
17. Panch T, Mattie H, and Celi LA. The “inconvenient truth” about AI in healthcare. NPJ Dig Med. (2019) 2:1–3. doi: 10.1038/s41746-019-0155-4
18. Stupple A, Singerman D, and Celi LA. The reproducibility crisis in the age of digital medicine. NPJ Dig Med. (2019) 2:2. doi: 10.1038/s41746-019-0079-z
19. Panch T, Szolovits P, and Atun R. Artificial intelligence, machine learning and health systems. J Glob Health. (2018) 8:020303. doi: 10.7189/jogh.08.020303
20. Jones SS, Heaton PS, Rudin RS, and Schneider EC. Unraveling the IT productivity paradox— lessons for health care. N Engl J Med. (2012) 366:2243–5. doi: 10.1056/NEJMp1204980
21. Compton-Phillips A, and Catalyst NE. Care Redesign Survey: What Data Can Really do for Health Care. Boston, MA: NEJM Catalyst. (2017) 3.
22. Schwartz WB. Medicine and the computer: the promise and problems of change. N Engl J Med. (1970) 283:1257–64. doi: 10.1056/NEJM197012032832305
23. Benson T. Principles of health interoperability HL7 and SNOMED. In: Science & Business Media. London: Springer-Verlag. (2012) 19–36. doi: 10.1007/978-1-4471-2801-4_15
24. Haug PJ, Narus SP, Bledsoe J, and Huff S. Promoting national and international standards to build interoperable clinical applications. AMIA Annu Symp Proc. (2018) 2018:555–63.
25. Lindblad S, Ernestam S, Van Citters AD, Lind C, Morgan TS, Nelson EC, et al. Creating a culture of health: evolving healthcare systems and patient engagement. QJM. (2017) 110:125–9. doi: 10.1093/qjmed/hcw188
26. Nelson EC, Dixon-Woods M, Batalden PB, Homa K, Van Citters AD, Morgan TS, et al., Patient focused registries can improve health, care, and science. BMJ. (2016). 354:i3319. doi: 10.1136/bmj.i3319
27. Berwick DM, and Hackbarth AD. Eliminating waste in US health care. JAMA. (2012) 307:1513–6. doi: 10.1001/jama.2012.362
28. Kelley TA. International consortium for health outcomes measurement (ICHOM). Trials. (2015) 16.3:O4. doi: 10.1186/1745-6215-16-S3-O4
29. McNamara RL, Spatz ES, Kelley TA, Stowell CJ, Beltrame J, Heidenreich P, et al. Standardized outcome measurement for patients with coronary artery disease: consensus from the International Consortium for Health Outcomes Measurement (ICHOM). J Am Heart Assoc. (2015) 4:e001767. doi: 10.1161/JAHA.115.001767
30. Ackerman IN, Cavka B, Lippa J, and Bucknill A. The feasibility of implementing the ICHOM standard set for hip and knee osteoarthritis: a mixed-methods evaluation in public and private hospital settings. J patient Rep Outcomes. (2018) 2:32. doi: 10.1186/s41687-018-0062-5
31. Ong WL, Schouwenburg MG, van Bommel ACM, Stowell C, Allison KH, Benn KE, et al. A standard set of value-based patient-centered outcomes for breast cancer: the International Consortium for Health Outcomes Measurement (ICHOM) initiative. JAMA Oncol. (2017) 3.5:677–85. doi: 10.1001/jamaoncol.2016.4851
32. Mahmud I, Kelley T, Stowell C, Haripriya A, Boman A, Kossler I, et al. A proposed minimum standard set of outcome measures for cataract surgery. JAMA Ophthalmol. (2015) 133:1247–52. doi: 10.1001/jamaophthalmol.2015.2810
33. Kim AH, Roberts C, Feagan BG, Banerjee R, Bemelman W, Bodger K, et al. Developing a standard set of patient-centred outcomes for inflammatory bowel disease—an international, cross-disciplinary consensus. J Crohns Colitis. (2017) 12.4:408–18. doi: 10.1093/ecco-jcc/jjx161
34. Burns DJP, Arora J, Okunade O, Beltrame JF, Bernardez-Pereira S, Crespo-Leiro MG, et al. International Consortium for Health Outcomes Measurement (ICHOM): standardized patient centered outcomes measurement set for heart failure patients. JACC Heart Failure. (2020) 8:212–22. doi: 10.1016/j.jchf.2019.09.007
35. Matney SA, Heale B, Hasley S, Decker E, Frederiksen B, Davis N, et al. Lessons learned in creating interoperable fast healthcare interoperability resources profiles for large-scale public health programs. Appl Clin Inform. (2019) 10:87–95. doi: 10.1055/s-0038-1677527
36. Moreno-Conde A, Moner D, Cruz WD, Santos MR, Maldonado JA, Robles M, et al. Clinical information modeling processes for semantic interoperability of electronic health records: systematic review and inductive analysis. J Am Med Inform Assoc. (2015) 22:925–34. doi: 10.1093/jamia/ocv008
37. ICHOM. Our Work. (2020). Available online at: www.ichom.org/standardsets/ (accessed January 16, 2020).
38. Richardson WS, Wilson MC, Nishikawa J, and Hayward RS. The well-built clinical question: a key to evidence-based decisions. ACP J Club. (1995) 123:A12–3.
39. Bojanowski P, Grave E, Joulin A, and Mikolov T. Enriching word vectors with subword information. Trans Assoc Comput Linguis. (2017) 5:135–46. doi: 10.1162/tacl_a_00051
40. Facebook Research. FastText GitHub Repository. Available online at: https://github.com/facebookresearch/fastText (accessed October 30, 2020).
41. ART-DECOR. Main Page. (2020). Available online at: https://art-decor.org (accassd January 16, 2020).
42. SNOMED International. (2020). Available online at: https://www.snomed.org (accessed January 16, 2020).
43. The Regenstrief Institute. LOINC. (2020). Available online at: https://loinc.org (accessed January 16, 2020).
44. HL7. FHIR overview. (2017). Available online at: http://hl7.org/fhir/over-view.html (accessed January 16, 2020).
45. Zhong RY, Klotz E, and Newman ST. Intelligent manufacturing in the context of industry 4.0: a review. “Engineering. (2017) 3:616–30. doi: 10.1016/J.ENG.2017.05.015
46. Ettorchi-Tardy A, Levif M, and Michel P. Benchmarking: a method for continuous quality improvement in health. Healthc Policy. (2012) 7:e101. doi: 10.12927/hcpol.2012.22872
47. Ellis J. All Inclusive Benchmarking. J Nurs Manag. (2006)14:377–83. doi: 10.1111/j.1365-2934.2006.00596.x
48. Pitarelli E, and Monnier E. Benchmarking: the missing link between evaluation and management. In: 4th ESS Conference October. Mannheim (2000).
49. Woodhouse D, Berg M, van der Putten J, and Houtepen J. Will Benchmarking ICUs Improve Outcome? Curre Opin Crit Care. (2009) 15:450–55. doi: 10.1097/MCC.0b013e32833079fb
Appendix
ICHOM original standard sets subject to harmonization (all available at www.ichom.org)
• Atrial Fibrillation
• Diabetes
• Paediatric Facial Palsy
• Congenital Upper Limb Anomalies
• Inflammatory Arthritis
• Hypertension LMIC
• Chronic Kidney Disease
• Pregnancy Childbirth
• Overactive Bladder
• Colorectal Cancer
• Older Person
• Cranofacial Microsomia
• Dementia
• Coronary Artery Disease
• Localized Prostate Cancer
• Lower Back Pain
• Parkinson Disease
• Depression Anxiety
• Advanced Prostate Cancer
• Cleft Lip Palate
• Lung Cancer
• Hip Knee Osteoarthritis
• Stroke
• Macular Degeneration
• Cataracts
• HeartFailure
• BreastCancer
• Inflammatory Bowel Disease
Keywords: health data interoperability, outcomes measurement, standardization, machine learning, patient centered
Citation: Blom MC, Khalid M, Van-Lettow B, Hutink H, Larsson S, Huff S and Ingvar M (2020) Harmonization of the ICHOM Quality Measures to Enable Health Outcomes Measurement in Multimorbid Patients. Front. Digit. Health 2:606246. doi: 10.3389/fdgth.2020.606246
Received: 14 September 2020; Accepted: 13 November 2020;
Published: 15 December 2020.
Edited by:
Daniel Capurro, The University of Melbourne, AustraliaReviewed by:
Xia Jing, Clemson University, United StatesKarmen S. Williams, City College of New York (CUNY), United States
Copyright © 2020 Blom, Khalid, Van-Lettow, Hutink, Larsson, Huff and Ingvar. This is an open-access article distributed under the terms of the Creative Commons Attribution License (CC BY). The use, distribution or reproduction in other forums is permitted, provided the original author(s) and the copyright owner(s) are credited and that the original publication in this journal is cited, in accordance with accepted academic practice. No use, distribution or reproduction is permitted which does not comply with these terms.
*Correspondence: Martin Ingvar, bWFydGluLmluZ3ZhckBraS5zZQ==