- Molecular Architects, Drug Discovery Chemistry, Evotec (UK) Ltd., Abingdon, United Kingdom
Over 90% of marketed drugs are small molecules, low molecular weight organic compounds that have been discovered, designed, and developed to prompt a specific biological process in the body. Examples include antibiotics (penicillin), analgesics (paracetamol) and synthetic hormones (corticosteroids). On average, it takes 10–15 years to develop a new medicine from initial discovery through to regulatory approval and the total cost is often in the billions. For every drug that makes it to the market, there are many more that do not, and it is the outlay associated with abortive efforts that accounts for most of this expense. The discovery of new drugs remains a significant challenge, involving teams of researchers from chemistry, biology, drug development, computer science and informatics. In this article we will discuss the key concepts and issues encountered in small molecule preclinical drug discovery and introduce some of the emerging technologies being developed to overcome current obstacles.
1 Introduction
Small molecule drugs are synthetic medicinal chemicals designed to mimic, enhance, or diminish the behaviour of natural substances or products within the body. They have relatively simple structures, customizable to meet specific therapeutic goals. They are generally stable and rarely need specialized storage conditions. Their behaviour in the body, or in vivo, is usually predictable, leading to straightforward, often oral, dosing protocols that patients find easy to manage. They can treat a wide variety of diseases because they can move through the body easily, transferring from the gut via the blood stream to the site of action, permeating through cell membranes to reach intracellular targets. They can be administered as pills, inhalers, suppositories or injectables, making them very flexible.
The chemical structure of small molecules can be designed to interact selectively with specific biological targets. By altering the atomic composition of small molecules, their overall properties can be fine-tuned to a particular purpose, eliciting only the desired response. The flexibility afforded by being able to explore all “chemical space” in this way, offers small molecule approaches a marked advantage over other modalities. The process of inventing a small molecule drug and ensuring that it performs precisely as it should, minimizing unwanted side effects, involves meticulous design and synthetic mastery from researchers, often over several years.
Compared to therapeutic proteins, or biologics, they are also easier to develop (Makurvet, 2021). Once optimized, small molecule drugs can be manufactured very reproducibly, an advantage for researchers seeking a return on their investment. Once patent life expires, non-branded generic forms of the medicine will increase availability to patients.
In this mini-review we will describe the key concepts and considerations involved in the discovery of small molecule drugs, covering traditional approaches, and discuss how recent advances such as the rise of artificial intelligence and innovative new modalities have reinvigorated the field.
1.1 How do small molecules interact with biological targets?
Proteins are the most common therapeutic targets; they are large complex molecules that play important roles in the body. Proteins are comprised of small building blocks known as amino acids. The sequence in which these amino acids are arranged determines the precise shape and function of the protein.
There are many ways in which small molecules work to elicit a therapeutic response in the human body (Silverman, 1992; Patrick, 2001; Young, 2009). Three of the most common are listed below:
1. Enzyme inhibitors—enzymes are proteins that catalyze biochemical reactions. By blocking the activity of these proteins, small molecules can interfere with disease processes to provide therapeutic benefits. Statins are a class of enzyme inhibitor drugs; they work by inhibiting the activity of an enzyme involved in the production of cholesterol in the liver. By reducing overall cholesterol levels in the body, they reduce the risk of heart disease and stroke.
2. Receptor agonist/antagonists—small molecules that can interact with proteins that exist on the surface of cells, usually in one of two ways: agonists which activate the receptor, mimicking the natural signaling molecule or antagonists which block the receptor, inhibiting the binding of the natural signaling molecule and reducing activation. Albuterol is a receptor agonist prescribed for the treatment of asthma which activates the receptor responsible for opening the airways in the lung.
3. Ion channel modulators—ion channels are proteins embedded in cell membranes which are responsible for regulating the flow of ions into and out of those cells. They play a key role in a wide variety of physiological processes including regulation of heartbeat and neurotransmission. Small molecule drugs can modulate the opening and closing of these channels to treat diseases such as epilepsy.
Many of the mechanisms of action described above involve a well-defined region on the protein into which a small molecule can fit and bind. These regions are known as active sites, and the geometric arrangement of their amino acids is such that they only have affinity for a few naturally occurring molecules, or substrates, within the body. This mechanism is often referred to as the “lock and key” theory. By understanding the requirements of the lock, researchers can create the best small molecule “key” to fit it and thereby generate the desired response. The stronger and the more specific the compound interaction with the amino acids of the targeted active site is, the less likely that compound will be to bind to different proteins. In turn, the fewer side effects it will have. Thus, the design of small molecule drugs is highly specialized.
As a result of better disease understanding and the development of innovative technologies, more diverse approaches for disease modulation by small molecules have evolved that exploit different mechanisms of action. Examples include the modulation of protein-protein interactions (PPI) (Wells and McClendon, 2007) (Trisciuzzi, et al., 2023), bifunctional protein degraders (Sun, et al., 2019) and stabilizers (Dong, et al., 2021; Henning, et al., 2022; Mullard, 2023). Some of these other modalities are explored later in the article. Table 1 shows some of the small molecule drugs that have reached the clinic over the last century, highlighting the evolution of their structural complexity as well as their mechanisms of action.
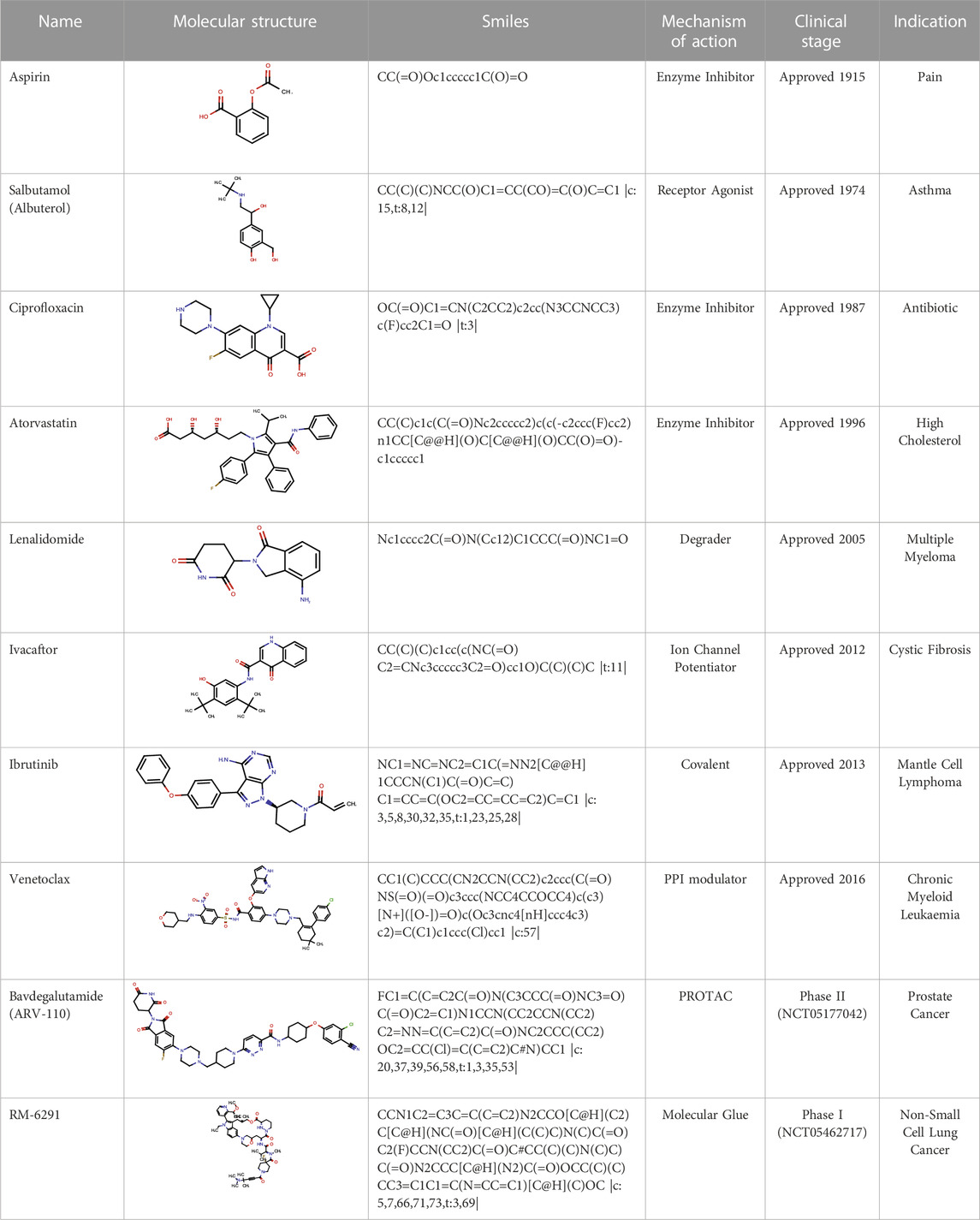
TABLE 1. Examples of small molecule drugs that have reached the clinic and the mechanisms of action associated with them.
2 The preclinical drug discovery process
Paths towards the identification of a preclinical drug candidate that successfully reaches the market are complicated, and depend upon a variety of factors including the complexity of the disease and the rigorous validation and testing required to meet regulatory approval requirements. The general process is outlined in Figure 1. In the following sections we will discuss each stage of the process in more detail.
2.1 Target discovery
Before drug discovery programs are prosecuted, the chosen biological target must be validated as relevant. This can be a time-consuming process as researchers try to demonstrate the role of the target in a particular biological pathway, process, or disease of interest (Schenone, et al., 2013). Upon validation, the assumption is made that modulation of that target will elicit the desired effect. Proteins remain the most represented class of therapeutic targets (Santos, et al., 2017), but other types of biological molecules can also be targeted by drugs, such as nucleic acids (DNA and RNA) (Kulkarni, et al., 2021). Therapeutic targets are chosen based on the disease being treated and the potential to interfere with the mechanisms of disease progression. For example, many anti-cancer drugs target proteins responsible for abnormal cell growth and division whilst for Alzheimer’s disease, a common target is amyloid beta, a protein that forms plaques (Ramanan and Day, 2023). Drugs can be developed to prevent plaque formation or degrade those that have already formed.
2.2 Screening and lead identification
Once the research team understands the physiological role played by the target, they can assess how its modulation will affect the disease state and begin their search for a chemical agent to achieve the desired outcome.
If researchers know little or nothing about a target at the outset of their work (maybe the utility of the target has only just been discovered, and the team is seeking to be first-in-class) then the simplest approach is a random high throughput screen (HTS). In this approach a large library of structurally diverse compounds (usually over 100 K) will be tested against the target, in the hope that some will prove active. These can be compounds that the researchers have purchased or made before, or purified natural products. Hit rates are typically low (∼1%, or sometimes lower!) but the goal is to find something, and build the project up from the actives, or seeds, identified.
Medicinal chemists continually wrestle to ensure that the compound collections they use for screening are fit for purpose. It is estimated that the total number of compounds that make up oral druglike chemical space is 1060 (Reymond, et al. 2010). Even a screening set containing a million compounds—which is rare—barely scratches the surface of available druglike space. In cases where more is known about a target (perhaps the binding site of the agents has already been ascertained), the screening set can be more focused. To create that focus, virtual screening can be undertaken. Docking experiments, in which compounds are fitted into the known binding site using a software package, can be carried out ahead of the physical testing. In this way researchers can eliminate compounds that have no hope of binding and promote those that have a better chance. This process is known as structure-based virtual screening because it is enabled by knowledge of the protein structure. This type of screening is designed to evaluate specific compound binding hypotheses, unlike the random HTS experiments described earlier.
Where the binding site is not known, it may still be possible to create a focused screening set if agents active against the target have been identified in previous efforts. In what is known as a ligand-based virtual screen, compounds with similar structures to the known actives are selected, in the hope that these will provide a best-in-class solution. These might contain motifs structurally similar to those in the comparator actives, or different while still bearing similar protein interaction properties. These scaffold hopping searches can be conducted in two- or three-dimensions, and success often opens new intellectual property space.
The concept of the fast follower takes the focus concept even further. Here a synthetic effort will start from a validated active discovered during a previous effort, to address residual target selectivity, drug stability or toxicological concerns as rapidly as possible. Often, this can result in the discovery of one or more new chemical series.
Having identified a suitable set of compounds for test, whether that be a random HTS with many compounds, or a smaller focused set informed by prior knowledge, the search is then performed. Lots of preparation goes into this: an assay must be created, expressing the target protein in relevant cell systems, validated using appropriate control compounds, and the correct concentrations at which to test compounds determined through pilot work.
2.3 Lead expansion
Hopefully, the researchers will get some hits. These may all come from the same structural class of compounds, or chemotype, or from several different ones. Either way, attention will now focus strongly on the active chemotypes, and the elucidation of the structure-activity relationship (SAR).
In a manner akin to the ligand-based virtual screening described above, researchers will seek to augment their knowledge around hit chemotypes by searching compound databases for additional, structurally related test materials. Such databases could be corporate, in-house compound collections, or the catalogues of commercial suppliers. If key compounds prove unavailable, the team will synthesize them.
Armed with the additional information this augmentation provides, researchers will first verify that there really is an SAR. A group of structurally related compounds which are all active is a potential red flag: genuinely bioactive compounds interact with the target protein and cases should exist where those interactions are suboptimal, and activity is reduced. If a chemotype is active across all its members (a “flat” SAR) this may indicate false positivity, arising from an artefactual phenomenon in the assay and not the desired interaction. Such chemotypes would drop out of contention.
Having removed these duds, the surviving chemotypes are compared to see which ones are most likely to generate a marketable clinical candidate down the line. Several factors inform the decision. For instance, can new members of the chemotype be synthesized quickly so that its optimization will be cost effective? Are the actives already known in the literature, and/or the intellectual property of competitors? Can the probable side effects of the compounds or their stability in vivo be anticipated, through modelling and testing, and plans created to discharge such risks?
Researchers will execute a design-make-test-analyse (DMTA) cycle to explore a chemotype through synthesis and assay. Computational scientists help to design the compounds best able to complete the study in the fewest number of cycles, deploying models to assess risks and predict activity, refining their forecasts and guiding the research ever more accurately over time as further data are acquired. In this way, the team builds a data package for each chemotype, allowing them to make an informed choice between them and create an issue-focused plan as they move forward into the optimization phase.
2.4 Lead optimization to preclinical development
At this stage of the process the objective is to gain as much knowledge as possible about the lead compounds’ efficacy and safety before they are tested in humans during clinical trials. This usually involves a multitude of laboratory tests, or assays, to assess both the drug’s action on the body and the body’s action on the drug.
A successful drug needs to be able to reach its target and exert a medicinal effect for the required length of time. Depending on the indication, this timeframe can vary markedly. A sleeping pill must reach its site of action quickly and be eliminated from the body by morning, whereas a drug designed to alleviate more chronic indications, such as cancer or dementia, will ideally last much longer. While target interaction, or engagement, is essential for a small molecule to have the desired pharmacological effect, it is equally vital that drugs be able to reach the location of the target efficiently and in sufficient concentration to effect that target engagement. It must also do so as selectively as possible to minimize potential toxicity and undesired side effects.
The engagement of a compound with the target of interest is investigated using structural biology techniques, through the computational creation and exploration of 3D models of the target. These structural models are central to the further design and optimization of compounds during the lead optimization phase, as researchers endeavour to improve the “fit” of the compound to the active site.
DMPK (drug metabolism and pharmacokinetics) studies allow researchers to understand how their compounds are absorbed into, distributed around, and eliminated from the body whilst pharmacodynamics (PD) studies the interactions of the drug with the body and tells the team about the potency and effectiveness of the compound. These assessments drive decisions during the discovery process, combining lab-based experimentation with the use of computational modelling and machine learning methods to make early predictions of various DMPK outcomes (Obrezanova, 2023). They help to determine the optimal dosage, side effect profile and toxicity risks. Toxicity can arise directly, through the action of the compound itself (insufficient selectivity for the required target) or indirectly, by interfering with the action of other drugs. Such drug-drug interactions (DDIs) are often problematic: if one drug inhibits critical enzymes then another might endure in the body to a much greater extent than is safe. This is a major concern in geriatric care for instance, where frail patients often take many medicines at once.
Thus, there are a vast number of consider researchers must think through at this stage to ensure their agents are safe and effective, and the DMTA cycle continues until this is achieved. Researchers will make modifications to the structures of their leading compounds to arrive at a better balance of DMPK properties. They will seek to bring about the desired clinical outcome from the smallest possible dose—another good strategy for the elimination of side effects. At the same time, they will seek to ensure that any structural changes they make will neither diminish potency against their target nor reduce selectivity. Drug discovery researchers often find themselves optimizing multiple, often competing parameters to find the “sweet spot” that will deliver the optimal properties for a given indication.
Prior to entering clinical trials, compounds are assessed both in vitro and in vivo. The latter involves the use of animal models, usually but not exclusively rodents, to represent the systems and functions of the human body. Such studies reveal important pharmacological and toxicological information, and until such time as computer modelling becomes so accurate that we can turn confidently to human volunteers when testing new drugs in vivo for the first time, their use will always have a place. At the same time, ethical concerns clearly have a huge role in driving the future of drug discovery, and pharmaceutical companies continue to drive animal testing down to the bare minimum needed, cognizant of the fact that in vivo responses in such testing do not always translate effectively to humans. There is continued heavy investment in more and better in vitro and ex vivo testing, and computer modelling, to meet this important challenge (Powell, 2018).
Many times, despite months of costly effort, it proves impossible to design a drug which is both safe and efficacious (DiMasi et al., 2003). At other times though, success is achieved. A drug candidate is developed with the activity, safety and DMPK profile needed to combat the targeted disease with a dose regimen that best suits the lifestyle of the patient. These compounds move forward to clinical trials.
3 Emerging technologies in small molecule drug discovery
Despite the large amount of money invested in drug discovery, there are still only around 500 treatments but over 7,000 human diseases (Austin, 2021). Drug discovery is expensive and time-consuming, with high rates of late-stage attrition due to lack of efficacy or compound related safety issues. The high failure rate underlines the complexity of drug discovery; learning from past mistakes and exploring new technologies is crucial if the industry is to improve its success rate. The implementation of innovative methodologies such as artificial intelligence (AI) and the development of new modalities to target “undruggable” targets are two ways the industry is evolving to improve the chances of producing new therapies and better patient outcomes.
3.1 Artificial intelligence (AI)
AI techniques offer the potential to improve the speed, efficiency and therefore cost of the drug discovery process on the basis that computers are more efficient at analyzing and processing large amounts of data to help researchers make less biased, more informed decisions (Brown, et al., 2020). A current example of AI application is in compound screening (Sadybekov and Katritch, 2023). Traditional high throughput screening is limited to hundreds of thousands of compounds at best, but AI can be used to screen millions of compounds virtually, solving the chemical space conundrum in a much more cost-effective way to identify potential novel drug candidates. Some commercial vendors have enormous compound libraries of synthetically tractable compounds which can be made to order if a virtual screen suggests they will be active. The ability of AI to explore broader chemical space improves the chances of success in finding potential leads and is especially pertinent given the rise of clinically approved compounds that reside outside traditional “oral druglike chemical space” (Doak, 2014). In other areas, advanced machine learning models are trained utilizing neural networks, imitating the biological network of the human brain, and used to predict the efficacy of compounds and safety endpoints (Cavasatto and Scardino, 2022). In turn, better informed decisions are made at an earlier stage in the process, mitigating the risk of more costly late-stage failure (Vamathevan, et al., 2019). Natural language processing (Gruetzemacher, 2022) can mine and process millions of scientific research articles to reveal insights into disease mechanisms and biology. AI tools now commonly used in drug discovery can design molecules from first principles (Vanhaelen et al., 2020), repurpose (Prasad and Kumar 2021; Roessler, et al., 2021) known drugs for the treatment of other diseases and even help plan synthetic routes (Thakkar, et al., 2021) based on prior knowledge.
This all highlights one of the key challenges with AI in drug discovery (Bender and Cortés-Ciriano, 2021). To be well enough informed to make accurate decisions, AI tools require large volumes of data from which they are “trained”. In the absence of sufficient quality and volume of data, these tools can be unreliable and inaccurate.
One of the most cited examples of how transformative AI can be in the field of life sciences is the release of DeepMind’s AlphaFold, which uses deep learning technology to predict three-dimensional protein structures from amino acid sequences (Jumper, et al., 2021). The neural network system used was trained on a vast dataset of protein structures and sequences, learning the intricate relationship between amino acid sequences and their preferred spatial arrangements with high accuracy. Whilst this has revolutionized the field of protein structure prediction, its full impact on the field of drug discovery is only just starting to be realized (Arnold, 2023).
AI holds much promise and has achieved some notable successes so far but human researchers are clearly far from being replaced; their experience is key to the validation of AI tools and the interpretation of their predictions (Jiménez-Luna et al., 2020). The synergistic integration of AI technologies, traditional screening approaches and human wisdom remains essential for modern drug discovery programs to successfully deliver new treatments (Griffen et al., 2020).
3.2 New modes of action for small molecules
The occupancy-based mode of action for many small molecule drugs has historically precluded a significant portion of the genome. For example, non-enzymatic and/or intrinsically disordered proteins do not possess well-defined active sites to which small molecules can bind. Such targets have been considered “undruggable”, but innovative strategies to chemically modulate these proteins are emerging and have attracted huge interest in drug research and development, offering the potential to treat many more diseases and address at least some of the gaps in unmet patient need (Blanco and Gardinier, 2020).
For instance, certain amino acids on the surface of a target protein, even in otherwise featureless active sites, are capable of bonding chemically to covalent drugs. Since such compounds directly attach to target proteins, rather than interact transiently, they offer benefits such as increased efficacy and specificity as well as extended duration of action. An example of a marketed covalent drug is ibrutinib, approved by the FDA in 2013 for the treatment of various blood cancers, which works by blocking the activity of a protein involved in the growth and survival of cancer cells (Shaywitz, 2013).
Another new modality for small molecules is their use in the disruption/modulation of the interactions between two or more target proteins. Biological processes are frequently regulated through such protein-protein interactions (PPIs), so directly targeting them can bring about therapeutic benefits. Venetoclax is an approved PPI drug prescribed for the treatment of acute myeloid leukemia (AML) and chronic lymphocytic leukemia (CLL). Its mode of action involves disrupting the interaction of two proteins that would otherwise work together to promote cancer cell survival (Roberts and Huang, 2017).
Protein degrader therapies take advantage of the body’s natural processes to schedule disease-causing proteins for degradation. The degrader recruits undesirable proteins to the cellular machinery responsible for protein breakdown, leading to their elimination. Lenalidomide (Armoiry et al., 2008) is a degrader that promotes the removal of a protein promoting cancer cell growth, FDA-approved for the treatment of multiple myeloma in 2005. This approach has been developed further in recent years, giving rise to a new class of drugs called PROTACs (proteolysis-targeting chimeras). Bavdegalutamide is an example of a PROTAC drug currently in Ph II trials for the treatment of prostate cancer (Gao, et al., 2022).
4 Conclusion
The field of small molecule drug discovery continues to evolve and remains a highly promising path in the pursuit of novel therapeutics (Härter, et al., 2022; Howes, 2023). Through meticulous design and rigorous testing in both preclinical and clinical settings, researchers in both the pharmaceutical industry and academia are advancing our understanding of disease biology. Compounds entering the clinic in recent years have successfully challenged previous dogma regarding the molecular properties required for an oral drug and the methodology by which disease progression can be arrested. Ongoing investment and advances in biology, computational technologies and innovative synthetic chemistry are providing researchers with increasingly efficient and precise tools for small molecule discovery and design. The synergistic partnership between scientific expertise and technological progress still holds great promise for the discovery and development of small molecule medications and treatments in the future.
Author contributions
MS: Writing–original draft, Writing–review and editing. MB: Writing–original draft, Writing–review and editing.
Funding
The author(s) declare that no financial support was received for the research, authorship, and/or publication of this article.
Conflict of interest
Authors MS and MB were employed by the company Evotec (UK) Ltd.
Publisher’s note
All claims expressed in this article are solely those of the authors and do not necessarily represent those of their affiliated organizations, or those of the publisher, the editors and the reviewers. Any product that may be evaluated in this article, or claim that may be made by its manufacturer, is not guaranteed or endorsed by the publisher.
References
Adams, D., Gonzalez-Duarte, A., O'Riordan, D., Yang, C.-C., Ueda, M., Kristen, V., et al. (2018). Patisiran, an RNAi therapeutic, for hereditary transthyretin amyloidosis. N. Engl. J. Med. 379, 11–21. doi:10.1056/NEJMoa1716153
Armoiry, X., Aulagner, G., and Facon, T. (2008). Lenalidomide in the treatment of multiple myeloma:a review. J. Clin. Pharm. Ther. 33, 219–226. doi:10.1111/j.1365-2710.2008.00920.x
Arnold, C. (2023). AlphaFold touted as next big things for drug discovery - but is it? Nature 622, 15–17. doi:10.1038/d41586-023-02984-w
Austin, C. P. (2021). National Center for advancing translational sciences. 28 january. Available at: https://ncats.nih.gov/director/january-2021 (Accessed October 30, 2023).
Bender, A., and Cortés-Ciriano, I. (2021). Artificial intelligence in drug discovery: what is realistic, what are illusions? Part 1: ways to make an impact, and why we are not there yet. Drug Discov. Today 26, 511–524. doi:10.1016/j.drudis.2020.12.009
Blanco, M.-J., and Gardinier, K. M. (2020). New chemical modalities and strategic thinking in early drug discovery. ACS Med. Chem. Lett. 228–231. doi:10.1021/acsmedchemlett.9b00582
Brown, N., Ertl, P., Lewis, R., Luksch, T., Reker, D., and Schneider, N. (2020). Artificial intelligence in chemistry and drug design. J. Comput. Aided Mol. Des. 34, 709–715. doi:10.1007/s10822-020-00317-x
Cavasatto, C. N., and Scardino, V. (2022). Machine learning toxicity prediction: latest advances by toxicity end point. ACS Omega 7, 47536–47546. doi:10.1021/acsomega.2c05693
DiMasi, J. A., Hansen, R. W., and Grabowski, H. G. (2003). The price of innovation: new estimates of drug development costs. J. Health Econ. 22, 151–185. doi:10.1016/S0167-6296(02)00126-1
Doak, B. C., Over, B., Giordanetto, F., and Kihlberg, J. (2014). Oral druggable space beyond the rule of 5: insights from drugs and clinical candidates. Chem. Biol. 21, 1115–1142. doi:10.1016/j.chembiol.2014.08.013
Dong, G., Ding, Yu, He, S., and Sheng, C. (2021). Molecular glues for targeted protein degradation: from serendipity to rational discovery. J. Med. Chem. 64, 10606–10620. doi:10.1021/acs.jmedchem.1c00895
Gao, X., Howard, A., Vuky, J., Dreicer, R., Oliver Sartor, A., Sternberg, C. N., et al. (2022). Phase 1/2 study of ARV-110, an androgen receptor (AR) PROTAC degrader, in metastatic castration-resistant prostate cancer (mCRPC). J. Clin. Oncol. 17–17. doi:10.1200/JCO.2020.38.15_suppl.3500
Griffen, E. J., Dossetter, A. G., and Leach, A. G. (2020). Chemists: AI is here; unite to get the benefits. J. Med. Chem. 63, 8695–8704. doi:10.1021/acs.jmedchem.0c00163
Gruetzemacher, R. (2022). The power of natural language processing. Available at: https://hbr.org/2022/04/the-power-of-natural-language-processing.
Härter, M., Beck, H., Haß, B., Schmeck, C., and Baerfacker, L. (2022). Small molecules and their impact in drug discovery: a perspective on the occasion of the 125th anniversary of the Bayer Chemical Research Laboratory. Drug Discov. Today 27, 1560–1574. doi:10.1016/j.drudis.2022.02.015
Henning, N. J., Boike, L., Spradlin, J. N., Ward, C. C., Liu, G., Zhang, E., et al. (2022). Deubiquitinase-targeting chimeras for targeted protein stabilization. Nat. Chem. Biol. 18, 412–421. doi:10.1038/s41589-022-00971-2
Howes, L. (2023). Chemical and engineering news. Available at: https://cen.acs.org/pharmaceuticals/drug-discovery/small-molecule-drug-discovery-having/101/i36 (Accessed November 3, 2023).
Jiménez-Luna, J., Grisoni, F., and Schneider, G. (2020). Drug discovery with explainable artificial intelligence. Nat. Mach. Intell. 2, 573–584. doi:10.1038/s42256-020-00236-4
Jumper, J., Evans, R., Alexander, P., Green, T., Figurnov, M., Ronneberger, O., et al. (2021). Highly accurate protein structure prediction with AlphaFold. Nature 596, 583–589. doi:10.1038/s41586-021-03819-2
Kulkarni, J. A., Witzigmann, D., Thomson, S. B., Chen, S., Leavitt, B. R., Cullis, R., et al. (2021). The current landscape of nucleic acid therapeutics. Nat. Nanaotechnology 16, 630–643. doi:10.1038/s41565-021-00898-0
Makurvet, F. D. (2021). Medicine in drug discovery 1-8. Available at: https://pharmanewsintel.com/news/key-differences-in-small-molecule-biologics-drug-development (Accessed October 27, 2023).Biologics vs. small molecules: drug costs and patient access
Mullard, A. (2023). Glue-based KRAS inhibitors make their debut cancer trial mark. Nat. Rev. Drug Discov. doi:10.1038/d41573-023-00169-8
Obrezanova, O. (2023). Artificial intelligence for compound pharmacokinetics prediction. Curr. Opin. Struct. Biol. 79, 102546–102547. doi:10.1016/j.sbi.2023.102546
Powell, K. (2018). Replacing the replacements: animal model alternatives. Science 12 (October), 243–245. doi:10.1126/science.362.6411.246-b
Prasad, K., and Kumar, V. (2021). Artificial intelligence-driven drug repurposing and structural biology for. Curr. Res. Pharmacol. Drug Discov., 2590–2571. doi:10.1016/j.crphar.2021.100042
Ramanan, V. K., and Day, G. S. (2023). Anti-amyloid therapies for Alzheimer disease: finally, good news for patients. Mol. Neurodegener. 18, 42–43. doi:10.1186/s13024-023-00637-0
Reymond, J.-L., Van Deursen, R., Blum, L. C., and Ruddigkeit, L. (2010). Chemical space as a source for new drugs. Med. Chem. Comm. 30-38. doi:10.1002/wcms.1104
Roberts, A. W., and Huang, D. C. S. (2017). Targeting BCL2 with BH3 mimetics: basic science and clinical application of venetoclax in chronic lymphocytic leukemia and related B cell malignancies. Clin. Pharmacol. Ther. 89–98. doi:10.1002/cpt.553
Roessler, H. I., Knoers, N. V. A. M., M van Haelst, M., and Gijs van Haaften, (2021). Drug repurposing for rare diseases. Trends Pharmacol. Sci. 42, 255–267. doi:10.1016/j.tips.2021.01.003
Sadybekov, A. V., and Katritch, V. (2023). Computational approaches streamlining drug discovery. Nature 616, 673–685. doi:10.1038/s41586-023-05905-z
Santos, R., Ursu, O., Gaulton, A., Bento, A. P., Donadi, S., Bologa, C. G., et al. (2017). A comprehensive map of molecular drug targets. Nat. Rev. Drug Discov. 19–34. doi:10.1038/nrd.2016.230
Schenone, M., Dančík, V., Wagner, K., and Clemons, P. A. (2013). Target Identification and mechanism of action in chemical biology and drug discovery. Nat. Chem. Biol. 9, 232–240. doi:10.1038/nchembio.1199
Shaywitz, D. (2013). Forbes. 5 april. Available at: https://www.forbes.com/sites/davidshaywitz/2013/04/05/the-wild-story-behind-a-promising-experimental-cancer-drug/ (Accessed November 3, 2023).
Silverman, R. B. (1992). The organic chemistry of drug design and drug action. California: Academic Press.
Sun, X., Gao, H., Yang, Y., He, M., Yue, Yu, Song, Y., et al. (2019). PROTACs: great opportunities for academia and industry.” Signal Transduction and Targeted Therapy.
Thakkar, A., Chadimova, V., Engkvist, O., Reymond, J.-L., and Reymond, J. L. (2021). Retrosynthetic accessibility score (RAscore) – rapid machine learned synthesizability classification from AI driven retrosynthetic planning. Chem. Sci. 12, 3339–3349. doi:10.1039/d0sc05401a
Vamathevan, J., Clark, D., Paul, C., Ian Dunahm, H., Ferran, E., Lee, G., et al. (2019). Applications of machine learning in drug discovery and development. Nat. Rev. Drug Discov. 463–477. doi:10.1038/s41573-019-0024-5
Vanhaelen, Q., Lin, Y.-C., and Zhavoronkov, A. (2020). The advent of generative chemistry. ACS Med. Chem. Lett. 11, 1496–1505. doi:10.1021/acsmedchemlett.0c00088
Wells, J. A., and McClendon, C. L. (2007). Reaching for high-hanging fruit in drug discovery at protein-protein interfaces. Nature 450, 1001–1009. doi:10.1038/nature06526
Keywords: drug discovery, small molecules, compound screening, artificial intelligence, multimodalities
Citation: Southey MWY and Brunavs M (2023) Introduction to small molecule drug discovery and preclinical development. Front. Drug Discov. 3:1314077. doi: 10.3389/fddsv.2023.1314077
Received: 10 October 2023; Accepted: 14 November 2023;
Published: 30 November 2023.
Edited by:
Bruno Villoutreix, Institut National de la Santé et de la Recherche Médicale (INSERM), FranceReviewed by:
Taisiia Feoktistova, Merck, United StatesDaniela Trisciuzzi, University of Bari Aldo Moro, Italy
Copyright © 2023 Southey and Brunavs. This is an open-access article distributed under the terms of the Creative Commons Attribution License (CC BY). The use, distribution or reproduction in other forums is permitted, provided the original author(s) and the copyright owner(s) are credited and that the original publication in this journal is cited, in accordance with accepted academic practice. No use, distribution or reproduction is permitted which does not comply with these terms.
*Correspondence: Michelle W. Y. Southey, bWljaGVsbGUuc291dGhleUBldm90ZWMuY29t