- 1Department of Medical Parasitology and Infection Biology, Parasite Chemotherapy Unit, Swiss Tropical and Public Health Institute, Allschwil, Switzerland
- 2Faculty of Science, University of Basel, Basel, Switzerland
Introduction: Suramin is one of the pharmacopeia’s most promiscuous drugs. Originally developed for African trypanosomiasis, suramin was also used for onchocerciasis and it has been proposed as an anticancer agent, antiviral drug, therapy for arthritis, autism, and antidote for snake bites. Target proteins of suramin have been described from different species. Here we identify the common motifs among these various targets, aiming to explain the promiscuous nature of suramin.
Methods: We have searched for suramin target proteins in the literature and in chemical databases. Applying rigorous inclusion criteria, a list of 44 diverse proteins was assembled with experimental evidence for direct interaction with, and inhibition by, suramin. Hidden Markov model-based target profiling was performed by running the full set of Pfam protein family domains against these proteins.
Results: Common denominators were identified by mapping the identified Pfam domains to molecular function gene ontology terms. This in silico pipeline identified nucleotide binding, nucleic acid binding, and binding to divalent cations as the most common denominators of the suramin targets.
Discussion: Our results suggest that the extraordinary polypharmacology of suramin may be caused by its ability to inhibit the interaction of proteins with nucleotides or nucleic acids and with divalent cations (Mg2+, Ca2+, Zn2+). Suramin is well known to inhibit nucleotide receptors and nucleic acid-binding enzymes. The association with divalent cations is new and might be key towards the design of better, more selective inhibitors.
1 Introduction
Suramin is one of the oldest drugs in use today. It was developed by Bayer in 1916 for African trypanosomiasis, has been on the WHO Model List of Essential Medicines since its onset in 1977, and is still the drug of choice for treating the first-stage of human African trypanosomiasis caused by Trypanosoma brucei rhodesiense (Lejon et al., 2013). Suramin is a colorless derivative of the azo-dye trypan blue (Wainwright, 2010). It is a large molecule (the hexasodium salt has a molecular weight of 1429 g/mol), carries six negative charges at physiological pH, is not orally bioavailable, strongly binds to albumin and other serum proteins, and lacks drug-like properties concerning the numbers of hydrogen bond donors or acceptors (Wiedemar et al., 2020). Furthermore, suramin causes various adverse effects, in particular hypersensitivity reactions and nephrotoxicity (WHO, 2013). Yet in spite of all these shortcomings, suramin has found numerous potential areas of application in the course of its hundred years of history.
Besides human African trypanosomiasis, suramin is also being used for Surra (also known as mal de caderas), a livestock disease that is caused by Trypanosoma evansi (Giordani et al., 2016). Suramin had been in clinical use against river blindness (caused by the nematode Onchocerca volvulus) (Hawking, 1978), until it got replaced by ivermectin in the early 1990s. Suramin was in the clinical phases of development against various forms of cancer (Larsen, 1993) and also against human immunodeficiency virus (De Clercq, 1987). It inhibits host cell entry by several viruses, including SARS-CoV-2 virus (Salgado-Benvindo et al., 2020). Other potential uses include arthritis and autism (Sahu et al., 2012; Naviaux et al., 2017). Furthermore, suramin was proposed as a protective agent against liver or kidney damage (Liu & Zhuang, 2011), and even as an antidote for snakebite due to its ability to inhibit the thrombin-like proteases of snake venom (Murakami et al., 2005). In accordance with such a multifaceted use, a large variety of different proteins have been proposed as targets of suramin. These include enzymes of core metabolism, enzymes involved in nucleic acid replication and epigenetics, proteases, kinases, and also several membrane receptor channels [summarized in (Wiedemar et al., 2020)]. To our knowledge, no other drug has as many different targets as suramin.
Here we perform a bioinformatic target profiling of suramin based on the hypothesis that the many targets of suramin, although of highly diverse biological nature, possess common motifs that suramin is binding to. To identify such common motifs we are using HMMer, which implements profile hidden Markov models (HMMs) built from multiple sequence alignments, as probabilistic models to score sequence homology in a position-dependent way (Eddy, 1998, 2011). Combining HMMer searches with GO term classification (Ashburner et al., 2000; Alborzi et al., 2018), we aim to identify common denominators, i.e., protein domains that are overrepresented, among the suramin targets. The overall in silico approach is outlined in Figure 1. It is sequence-based and complementary to the structure-based approach taken by Dey and co-workers (Dey et al., 2021). Both have the same aim: to understand the nature of suramin’s promiscuous mode of action and, based on this knowledge, to design more specific inhibitors with fewer side effects.
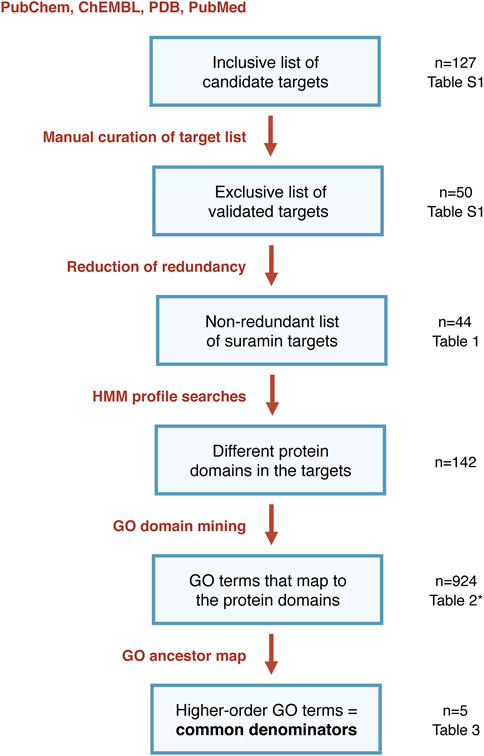
FIGURE 1. Flowchart of the bioinformatic pipeline from published proteins to common denominators of suramin targets (*note that Table 2 does not show all the 924 identified GO terms but only those that were associated to at least five different suramin target proteins).
2 Materials and methods
2.1 Database searches
The chemical databases that were accessed are ChEMBL (www.ebi.ac.uk/chembl/) (Davies et al., 2015; Mendez et al., 2019) (RRID:SCR_014042) and PubChem (pubchem.ncbi.nlm.nih.gov/) (Kim et al., 2021) (RRID:SCR_004284). The databases were searched with the term “suramin”, and also with the identification numbers (ID) of suramin and its various salts and further derivatives. For PubChem, the IDs used were 8514, 5361, 135538647, 16760668, 11979655, 11979631, 3943541, 11979654, 49772374, 49771850, 54600747, and 11979493. PubMed (pubmed.ncbi.nlm.nih.gov/) (RRID:SCR_004846) was used as a literature database, searching e.g., with “suramin AND target” and manually sorting out the relevant publications from the retrieved results.
2.2 Protein sequences
All protein sequences were obtained from UniProt (www.uniprot.org) (UniProt-Consortium, 2021) (RRID:SCR_004426) except those of viruses, which were obtained from PDB (https://www.rcsb.org) (RRID:SCR_012820) (PDB-Consortium, 2019). PDB was resorted to in order to make sure that the processed, functional polypeptides were retrieved rather than the whole viral polyproteins. Reviewed entries were used preferably. For posttranslationally cleaved proteins (e.g., thrombin), the sequence of the precursor was used (e.g., prothrombin). For proteins with several isoforms, only the isoform stated by the reference was included; if no such information was provided, the longest isoform was selected.
2.3 Perl scripting
All procedures were automated with self-made Perl (RRID:SCR_018313) scripts on a BioLinux platform (Field et al., 2006) (RRID:SCR_005399). The scripts served to run the described programs for profile and motif searching, and to parse the programs’ output into tabular format for further analysis. All scripts were tested for accuracy by monitoring the overall numbers of sequences processed and by manual re-testing of individual samples. The scripts are available on request.
2.4 Redundancy reduction
Needleman-Wunsch global alignments (Needleman & Wunsch, 1970) were performed with “needle” of the EMBOSS 6.6.0 suite (Rice et al., 2000) (RRID:SCR_008493). Protein distance, defined as d = 1—(No. Similar residues/alignment length), was calculated for all pairs of proteins. The frequency distribution of the distances was visualized with RStudio (version 1.2.1335) (RRID:SCR_000432) using R (version 3.6.0) (RRID:SCR_001905).
2.5 Calculation of isoelectric points
Isoelectric points of amino acid sequences were determined with the command “iep” of EMBOSS 6.6.0 (Rice et al., 2000) (RRID:SCR_008493). It calculates the isoelectric point of an amino acid sequence by estimating the overall charge at different pH values. This was performed for the suramin targets as well as for the human proteome, downloaded from UniProt (www.uniprot.org; accession UP000005640; date: 06.01.20). Statistical tests were done in RStudio (version 1.2.1335) (RRID:SCR_000432) using R (version 3.6.0) (RRID:SCR_001905).
2.6 Motif searches and GO terms
Motifs were identified using “hmmscan” with tabular output of the HMMer 3.2.1 package (hmmer.org/) (Eddy, 2009, 2011) (RRID:SCR_005305) against Pfam version 32.0 (El-Gebali et al., 2019) (RRID:SCR_004726). The expectancy (E) value cut-off was set to 0.01. Pfam accessions were linked to ‘molecular function’ GO terms by using a text file produced by GODomainMiner (Alborzi et al., 2018) providing associations between GO term id and Pfam accession numbers (godm.loria.fr/). The GO names were retrieved from QuickGO (www.ebi.ac.uk/QuickGO/) (Binns et al., 2009) (RRID:SCR_004608). For quality control the targets associated with these GO terms were compared to the Denylist of the respective GO term on QuickGO (where the Denylist is called Blacklist) (Binns et al., 2009). QuickGO was further used to link GO terms via “is a” relationship to higher-order terms using the ancestor chart.
3 Results
3.1 Collection of the published suramin targets
A comprehensive list of suramin targets was required as the starting point for target profiling. We aimed to assemble all the proteins that had been published as putative targets of suramin in the scientific literature by performing compound searches in the chemical databases ChEMBL and PubChem. The reported proteins were supplemented with those obtained from papers on suramin targets found in PubMed, and from the references therein. Finally, the solved co-crystal structures of suramin deposited in PDB (Wiedemar et al., 2020) were added. This resulted in an initial, maximally inclusive list of 127 candidate suramin target proteins from 36 different species encompassing mammals (n = 131 sequences) and other vertebrates (n = 6), fungi (n = 1), protozoa (n = 18), plants (n = 1), bacteria (n = 10), and viruses (n = 13) (Supplementary Table S1). Additional information that was collected alongside the targets included the type of assay that was used, the potency of suramin in that assay, and the nature of the evidence for interaction of suramin with its proposed target.
3.2 Curation of the suramin target list
Special care was taken to use only proteins that physically interact with suramin. Thus the priority for curation of the suramin target list was to minimize the number of false positives; this meant accepting a few false negatives—i.e., proteins that had been wrongly excluded from the list—rather than including proteins that did not actually bind suramin. The following were used as inclusion criteria: inhibition of activity by at least 50% by a suramin concentration of no more than 50 μM, determined in an enzyme-based assay (as opposed to whole-cell assay), except for cell-based assays with viral proteins. Regarding protein complexes of multiple subunits, only the subunit interacting with suramin was included. A subunit was considered to be interacting with suramin if either only one subunit had been included in the assay, or if binding to a specific subunit had been validated experimentally. Otherwise, or if no such information was provided, the whole complex was excluded. Cases where suramin inhibited protein-protein interaction (rather than protein function) were excluded as well. The resulting list of targets consisted of 50 proteins, experimentally validated to be inhibited by suramin (column E of Supplementary Table S1; Figure 1).
3.3 Redundancy reduction of the suramin targets
To avoid a possible bias from overrepresentation of certain proteins among the suramin targets, e.g., due to the presence of closely related orthologues from different species, redundancy reduction of the sequence set was carried out as follows. All pairwise global alignments of the 50 amino acid sequences were performed and the distance d between each sequence pair was calculated. Based on the frequency distribution of d, a cut-off of 0.6 was chosen (Figure 2). Sequence pairs with a distance below that cut-off were regarded as highly similar, and of a group of highly similar sequences only the longest sequence was kept. After this final step of curation, 44 diverse proteins from 14 different species remained (Table 1; column E of Supplementary Table S1). This renders suramin the most promiscuous drug, surpassing other polypharmacological agents with respect to the number of reported targets (Haupt et al., 2013).
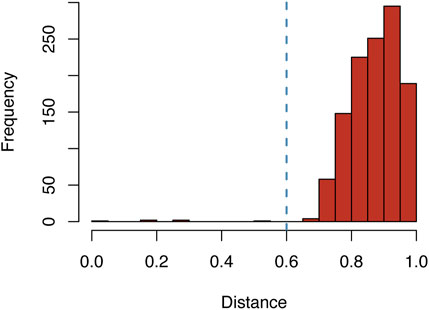
FIGURE 2. Frequency distribution of the distances between all pairs of validated suramin targets. The blue line indicates the cut-off value of 0.6 for the distance d between two sequences, which was chosen for redundancy reduction (where d = 1—No. Similar residues/length of global alignment).
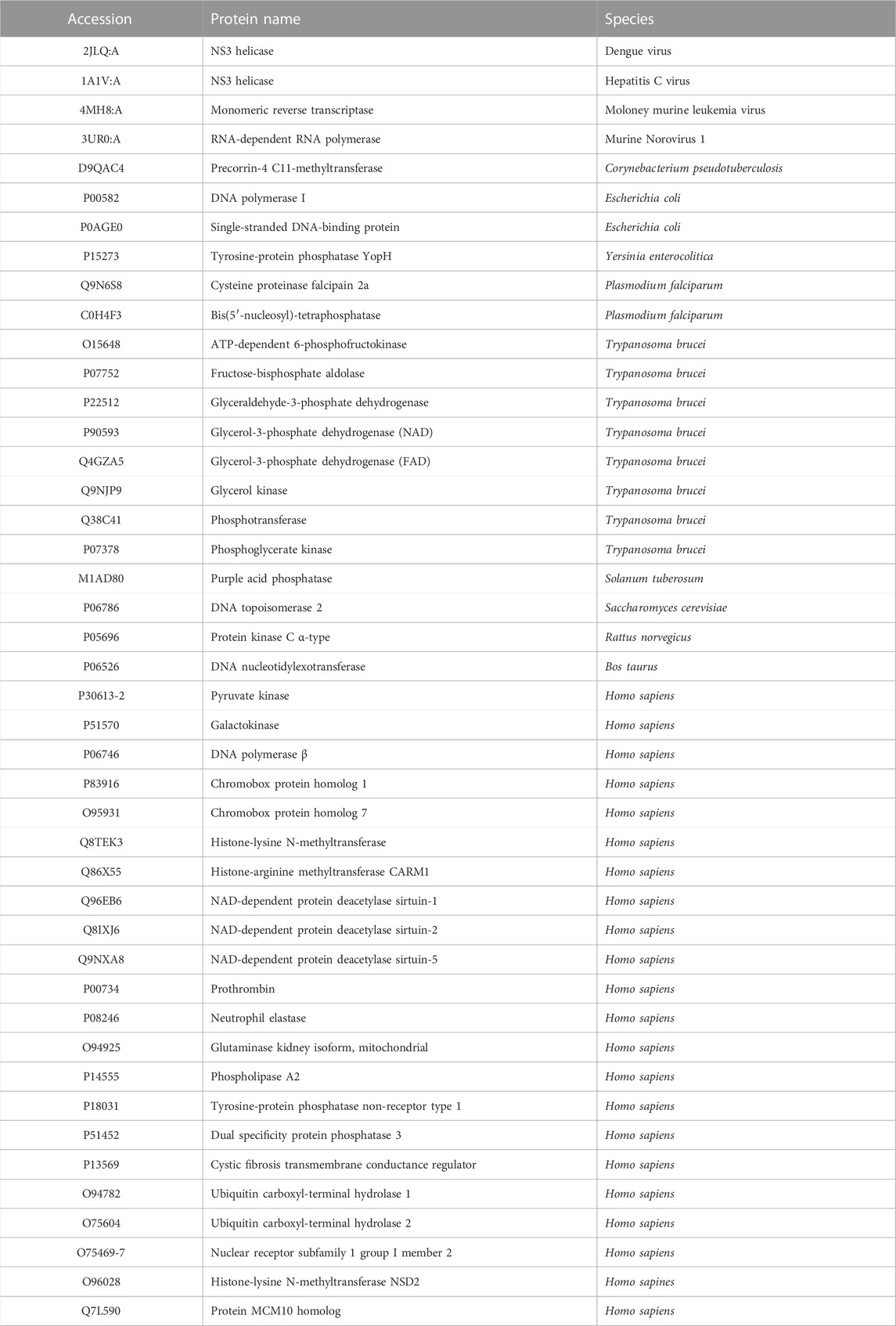
TABLE 1. List of the 44 experimentally validated, redundancy-reduced suramin targets. See Supplementary Table S1 for the references and for information on the selection criteria for inclusion of targets.
3.4 Isoelectric points of the suramin targets
Suramin is much more active against the glycolytic enzymes of T. brucei than against their mammalian orthologues (Willson et al., 1993). At the same time, the glycolytic enzymes of T. brucei have clearly higher isoelectric points (pI between 9 and 11) than their mammalian counterparts (Misset & Opperdoes, 1987). This observation has raised the hypothesis that the negatively charged suramin preferably binds the trypanosomal enzymes because it interacts with clusters of positively charged amino acids that are absent from the mammalian enzymes (Willson et al., 1993). We therefore tested whether the suramin target set has an overrepresentation of positive charges in general. However, the mean isoelectric point of the 44 suramin targets (Table 1) was only slightly higher (pI 7.67) than that of the predicted human proteome (pI 7.40). This difference was not statistically significant (p = .27, Welch two sample t-test).
3.5 HMM profiling of the suramin targets
To identify all the functional motifs in the suramin target sequences, the set of 44 proteins (Table 1) was run against the complete Pfam collection of protein domain families (El-Gebali et al., 2019) with the program hmmscan of the HMMer3 suite (Eddy, 2009). The Pfam database contained 18,000 position-dependent scoring matrices for hidden Markov model-based profile searches (El-Gebali et al., 2019). Using an expectancy (E-value) cut-off of 0.01, this search returned on average eight hits per protein. The total number of different Pfam domains that was detected in the suramin targets was 142. Only 16 of these were associated with more than one protein, and none was associated with more than three, underscoring the heterogeneity of the presumed suramin targets.
3.6 Common denominators of the HMM profiles
Given the diversity not only of the suramin targets but also of the associated Pfam domains, we had to move up yet another level of abstraction to identify potential common denominators. This was done by linking the identified Pfam domains to GO (gene ontology) terms (Ashburner et al., 2000) based on the annotations provided by GODomainMiner (Alborzi et al., 2018). Thus GO terms for molecular function were assigned to the suramin targets via their Pfam domains. The resulting annotations were examined against the Denylist provided by QuickGO (Binns et al., 2009) and annotations that were likely to be incorrect were removed. After this purification step, there remained thirteen GO terms that matched five or more targets (Table 2). Two common themes emerged from this analysis: binding to nucleotides or nucleic acids, and binding to divalent cations such as Mg2+, Ca2+, or Zn2+. This was confirmed by a QuickGO ancestor chart (Binns et al., 2009) to determine the higher-order GO terms, which identified “cation binding” and “nucleotide binding” as the two most frequent entries (Table 3).
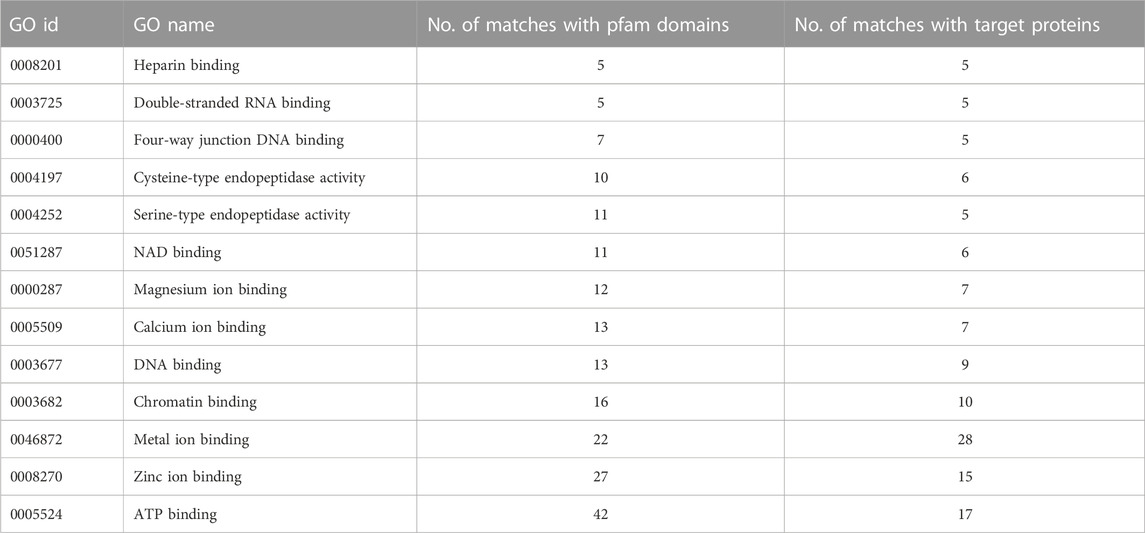
TABLE 2. GO terms associated with suramin targets, their number of associations with the Pfam domains identified in the targets, and the resulting number of associations with the targets themselves. Only terms that matched at least five different targets are included.
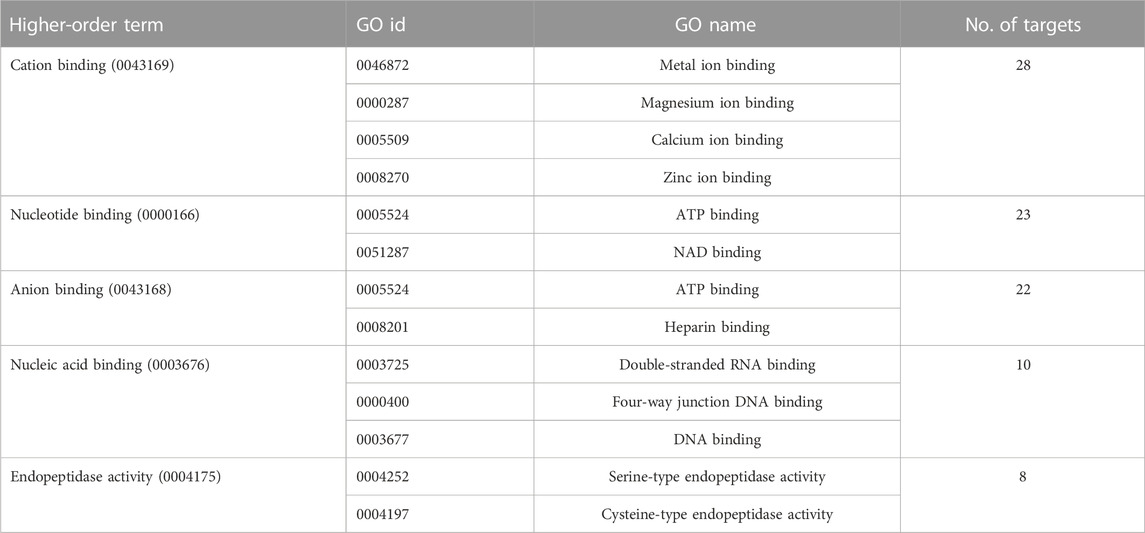
TABLE 3. The higher-order GO terms of the GO id’s of Table 2 and the numbers of suramin targets these higher-order GO terms are associated with.
4 Discussion
Suramin stands out as an atypical molecule for a drug due to 1) its high molecular weight, 2) its comparably high degree of flexibility (Haupt et al., 2013), and 3) the fact that it carries six negative charges at physiological pH. These properties likely account for suramin’s polypharmacology, allowing it to bind to diverse kinds of target proteins. However, while suramin is a promiscuous drug, it is not indiscriminate. It binds its many targets in a selective way, which accounts for the fact that suramin is actually used as a therapeutic agent (and this for over a century). Suramin experiences no metabolism in the human body, and it has a an extremely long half-life of elimination of over 50 days (Burri et al., 2014). Understanding why suramin binds to so many different target proteins is the first step towards better, more specific inhibitors.
The prerequisite for this is a scrutinized list of suramin targets. To our knowledge, Supplementary Table S1 and Table 1 provide the first comprehensive list of proteins that are directly inhibited by suramin as based on experimental evidence. After an extensive search of the literature resulting in a maximally inclusive list of 127 putative suramin targets (Supplementary Table S1), the focus for the subsequent bioinformatic pipeline (Figure 1) was on specificity rather than sensitivity. Stringent criteria were applied to ensure that only proteins were included in Table 1 that physically interact with suramin. While these inclusion criteria were somewhat subjective, the subsequent analyses proceeded in an unbiased way. The cut-off for redundancy reduction of d = .6 was obvious from the frequency distribution of the pairwise distances (Figure 2).
The direct mapping of the identified suramin targets to GO terms was not possible in an unbiased way because the targets stemmed from different species (Table 1), not all of which had the same high level of annotation as e.g., H. sapiens. This is why the targets were first linked to the complete set of HMM profiles from PFAM, and then the PFAM profiles were linked to GO terms in an unbiased way. Finally, the QuickGO denylist of frequent matchers allowed to remove likely wrong associations. Thus we are confident that the identified common denominators shown in Table 2 are unbiased and indeed reflect the binding properties of suramin.
The predominant GO terms associated with the identified Pfam motifs of the suramin targets were “nucleotide-binding”, “anion binding”, and “cation-binding”. The terms “nucleic acid binding” and “endopeptidase activity” were less frequent. Nucleotide binding as well as nucleic acid binding were to be expected given that suramin is well known to inhibit not only polymerases and other enzymes in nucleic acid metabolism but also ATP receptors (Wiedemar et al., 2020). Seventeen of the 22 targets linked to the GO term “anion binding” were linked also to the more specific term “ATP binding”, which is in turn is associated with the broader term “nucleotide binding”. In addition, anion binding can be explained by the frequent binding of the anionic suramin to positively charged amino acids, which can bind other anions as well—in particular heparin (Table 3; Dey et al., 2021). Although associated only with eight targets, the GO term “endopeptidase activity” is in agreement with previous findings (Morty et al., 1998).
Cation-binding was more surprising—at least to us—but actually had emerged on top of the list of common denominators (Table 3). This indicates that suramin might interfere with the binding of proteins to divalent cations (Mg2+, Ca2+, or Zn2+). The negative charge of suramin suggests that it disturbs ion binding by interacting with the cations themselves; an interaction with Mg2+ might even explain some of suramin’s effects on DNA- and RNA-binding enzymes. However, suramin’s action was not dependent on the concentration of divalent cations (Fong & Good, 1972), which would argue against a direct interaction between suramin and the cations. Direct interaction between suramin and cation binding sites on the target proteins is an alternative possibility. Suramin was shown to bind to the same amino acids on the P2X1 receptor that are involved in the binding of divalent cations (Igawa et al., 2015). Co-crystal structures with suramin have been solved mainly for viral proteins and snake venom proteases (Wiedemar et al., 2020). In the co-crystal structure with myotoxin I of Bothrops moojeni (Salvador et al., 2018) as well as myotoxin II of Bothrops asper (Murakami et al., 2005), suramin attaches to the so-called calcium binding loop. However, these phospholipases are catalytically inactive and their calcium binding loops harbor mutations that prevent Ca2+ from binding. Therefore, it remains to be resolved whether suramin binding is a consequence of these mutations, or whether suramin would bind also to functional calcium binding loops. Therefore, co-crystal structures of suramin with proteins that contain functional binding sites for divalent cations will be necessary to understand the polypharmacology of suramin. Elucidation of the role of divalent cations in the mode of action of suramin may be key towards designing new and more selective inhibitors.
Data availability statement
The original contributions presented in the study are included in the article/Supplementary Material, further inquiries can be directed to the corresponding author.
Author contributions
Planning of the study and experimental design, DH and PM; experimental performance, DH; data analysis and representation, DH; writing of the manuscript, DH and PM; acquisition of funding, PM.
Funding
This research was funded by the Swiss National Science Foundation (grant 310030_156264).
Conflict of interest
The authors declare that the research was conducted in the absence of any commercial or financial relationships that could be construed as a potential conflict of interest.
Publisher’s note
All claims expressed in this article are solely those of the authors and do not necessarily represent those of their affiliated organizations, or those of the publisher, the editors and the reviewers. Any product that may be evaluated in this article, or claim that may be made by its manufacturer, is not guaranteed or endorsed by the publisher.
Supplementary material
The Supplementary Material for this article can be found online at: https://www.frontiersin.org/articles/10.3389/fddsv.2023.1112992/full#supplementary-material
References
Alborzi, S. Z., Ritchie, D. W., and Devignes, M. D. (2018). Computational discovery of direct associations between GO terms and protein domains. BMC Bioinforma. 19 (14), 413. doi:10.1186/s12859-018-2380-2
Ashburner, M., Ball, C. A., Blake, J. A., Botstein, D., Butler, H., Cherry, J. M., et al. (2000). Gene ontology: Tool for the unification of biology. The gene ontology consortium. Nat. Genet. 25 (1), 25–29. doi:10.1038/75556
Binns, D., Dimmer, E., Huntley, R., Barrell, D., O'Donovan, C., and Apweiler, R. (2009). QuickGO: A web-based tool for gene ontology searching. Bioinformatics 25 (22), 3045–3046. doi:10.1093/bioinformatics/btp536
Burri, C., Chappuis, F., and Brun, R. (2014). “Human african trypanosomiasis,”. Manson's tropical diseases. Editors J. Farrar, P. J. Hotez, T. Junghanss, G. Kang, D. Lalloo, and N. J. White (editionElsevier Saunders), 23, 606–621.
Davies, M., Nowotka, M., Papadatos, G., Dedman, N., Gaulton, A., Atkinson, F., et al. (2015). ChEMBL web services: Streamlining access to drug discovery data and utilities. Nucleic Acids Res. 43 (W1), W612–W620. doi:10.1093/nar/gkv352
De Clercq, E. (1987). Suramin in the treatment of AIDS: Mechanism of action. Antivir. Res. 7 (1), 1–10. doi:10.1016/0166-3542(87)90034-9
Dey, D., Ramakumar, S., and Conn, G. L. (2021). Targeted redesign of suramin analogs for novel antimicrobial lead development. J. Chem. Inf. Model 61 (9), 4442–4454. doi:10.1021/acs.jcim.1c00578
Eddy, S. R. (2009). A new generation of homology search tools based on probabilistic inference. Genome Inf. 23 (1), 205–211.
Eddy, S. R. (2011). Accelerated profile HMM searches. PLoS Comput. Biol. 7 (10), e1002195. doi:10.1371/journal.pcbi.1002195
Eddy, S. R. (1998). Profile hidden Markov models. Bioinformatics 14 (9), 755–763. doi:10.1093/bioinformatics/14.9.755
El-Gebali, S., Mistry, J., Bateman, A., Eddy, S. R., Luciani, A., Potter, S. C., et al. (2019). The Pfam protein families database in 2019. Nucleic Acids Res. 47 (D1), D427–D432. doi:10.1093/nar/gky995
Field, D., Tiwari, B., Booth, T., Houten, S., Swan, D., Bertrand, N., et al. (2006). Open software for biologists: From famine to feast. Nat. Biotechnol. 24 (7), 801–803. doi:10.1038/nbt0706-801
Fong, J. S., and Good, R. A. (1972). Suramin--a potent reversible and competitive inhibitor of complement systems. Clin. Exp. Immunol. 10 (1), 127–138.
Giordani, F., Morrison, L. J., Rowan, T. G., Hp, D. E. K., and Barrett, M. P. (2016). The animal trypanosomiases and their chemotherapy: A review. Parasitology 143 (14), 1862–1889. doi:10.1017/S0031182016001268
Haupt, V. J., Daminelli, S., and Schroeder, M. (2013). Drug promiscuity in PDB: Protein binding site similarity is key. PLoS One 8 (6), e65894. doi:10.1371/journal.pone.0065894
Hawking, F. (1978). Suramin: With special reference to onchocerciasis. Adv. Pharmacol. Chemother. 15, 289–322. doi:10.1016/s1054-3589(08)60486-x
Igawa, T., Abe, Y., Tsuda, M., Inoue, K., and Ueda, T. (2015). Solution structure of the rat P2X4 receptor head domain involved in inhibitory metal binding. FEBS Lett. 589 (6), 680–686. doi:10.1016/j.febslet.2015.01.034
Kim, S., Chen, J., Cheng, T., Gindulyte, A., He, J., He, S., et al. (2021). PubChem in 2021: New data content and improved web interfaces. Nucleic Acids Res. 49 (D1), D1388–D1395. doi:10.1093/nar/gkaa971
Larsen, A. K. (1993). Suramin: An anticancer drug with unique biological effects. Cancer Chemother. Pharmacol. 32 (2), 96–98. doi:10.1007/BF00685609
Lejon, V., Bentivoglio, M., and Franco, J. R. (2013). Human african trypanosomiasis. Handb. Clin. Neurol. 114, 169–181. doi:10.1016/B978-0-444-53490-3.00011-X
Liu, N., and Zhuang, S. (2011). Tissue protective and anti-fibrotic actions of suramin: New uses of an old drug. Curr. Clin. Pharmacol. 6 (2), 137–142. doi:10.2174/157488411796151174
Mendez, D., Gaulton, A., Bento, A. P., Chambers, J., De Veij, M., Felix, E., et al. (2019). ChEMBL: Towards direct deposition of bioassay data. Nucleic Acids Res. 47 (D1), D930–D940. doi:10.1093/nar/gky1075
Misset, O., and Opperdoes, F. R. (1987). The phosphoglycerate kinases from Trypanosoma brucei. Eur. J. Biochem. 162, 493–500. doi:10.1111/j.1432-1033.1987.tb10667.x
Morty, R. E., Troeberg, L., Pike, R. N., Jones, R., Nickel, P., Lonsdale-Eccles, J. D., et al. (1998). A trypanosome oligopeptidase as a target for the trypanocidal agents pentamidine, diminazene and suramin. FEBS Lett. 433 (3), 251–256. doi:10.1016/s0014-5793(98)00914-4
Murakami, M. T., Arruda, E. Z., Melo, P. A., Martinez, A. B., Calil-Elias, S., Tomaz, M. A., et al. (2005). Inhibition of myotoxic activity of Bothrops asper myotoxin II by the anti-trypanosomal drug suramin. J. Mol. Biol. 350 (3), 416–426. doi:10.1016/j.jmb.2005.04.072
Naviaux, R. K., Curtis, B., Li, K., Naviaux, J. C., Bright, A. T., Reiner, G. E., et al. (2017). Low-dose suramin in autism spectrum disorder: A small, phase I/II, randomized clinical trial. Ann. Clin. Transl. Neurol. 4 (7), 491–505. doi:10.1002/acn3.424
Needleman, S. B., and Wunsch, C. D. (1970). A general method applicable to the search for similarities in the amino acid sequence of two proteins. J. Mol. Biol. 48 (3), 443–453. doi:10.1016/0022-2836(70)90057-4
Pdb-Consortium, (2019). Protein data bank: The single global archive for 3D macromolecular structure data. Nucleic Acids Res. 47 (D1), D520–D528. doi:10.1093/nar/gky949
Rice, P., Longden, I., and Bleasby, A. (2000). Emboss: The European molecular biology open software suite. Trends Genet. 16 (6), 276–277. doi:10.1016/s0168-9525(00)02024-2
Sahu, D., Saroha, A., Roy, S., Das, S., Srivastava, P. S., and Das, H. R. (2012). Suramin ameliorates collagen induced arthritis. Int. Immunopharmacol. 12 (1), 288–293. doi:10.1016/j.intimp.2011.12.003
Salgado-Benvindo, C., Thaler, M., Tas, A., Ogando, N. S., Bredenbeek, P. J., Ninaber, D. K., et al. (2020). Suramin inhibits SARS-CoV-2 infection in cell culture by interfering with early steps of the replication cycle. Antimicrob. Agents Chemother. 64 (8), e00900-e00920. doi:10.1128/AAC.00900-20
Salvador, G. H. M., Dreyer, T. R., Gomes, A. A. S., Cavalcante, W. L. G., Dos Santos, J. I., Gandin, C. A., et al. (2018). Structural and functional characterization of suramin-bound MjTX-I from Bothrops moojeni suggests a particular myotoxic mechanism. Sci. Rep. 8 (1), 10317. doi:10.1038/s41598-018-28584-7
UniProt-Consortium (2021). UniProt: The universal protein knowledgebase in 2021. Nucleic Acids Res. 49 (D1), D480–D489. doi:10.1093/nar/gkaa1100
Wainwright, M. (2010). Dyes, trypanosomiasis and DNA: A historical and critical review. Biotech. Histochem 85 (6), 341–354. doi:10.3109/10520290903297528
Who, (2013). Control and surveillance of human african trypanosomiasis. WHO technical report series, 984.
Wiedemar, N., Hauser, D. A., and Mäser, P. (2020). 100 Years of suramin. Antimicrob. Agents Chemother. 64 (3), 011688–e1219. doi:10.1128/AAC.01168-19
Keywords: suramin, drug target, hidden Markov model, motif search, nucleotide binding, ion binding, African trypanosomiasis
Citation: Hauser DA and Mäser P (2023) HMM-based profiling identifies the binding to divalent cations and nucleotides as common denominators of suramin targets. Front. Drug Discov. 3:1112992. doi: 10.3389/fddsv.2023.1112992
Received: 30 November 2022; Accepted: 30 January 2023;
Published: 10 February 2023.
Edited by:
Caio Haddad Franco, University of Coimbra, PortugalReviewed by:
Ramendra Pati Pandey, SRM University (Delhi-NCR), IndiaLucianna Helene Santos, Pasteur Institute of Montevideo, Uruguay
Copyright © 2023 Hauser and Mäser. This is an open-access article distributed under the terms of the Creative Commons Attribution License (CC BY). The use, distribution or reproduction in other forums is permitted, provided the original author(s) and the copyright owner(s) are credited and that the original publication in this journal is cited, in accordance with accepted academic practice. No use, distribution or reproduction is permitted which does not comply with these terms.
*Correspondence: Pascal Mäser, pascal.maeser@swisstph.ch