- EpiVax, Inc., Providence, RI, United States
The in silico prediction of T cell epitopes within any peptide or biologic drug candidate serves as an important first step for assessing immunogenicity. T cell epitopes bind human leukocyte antigen (HLA) by a well-characterized interaction of amino acid side chains and pockets in the HLA molecule binding groove. Immunoinformatics tools, such as the EpiMatrix algorithm, have been developed to screen natural amino acid sequences for peptides that will bind HLA. In addition to commonly occurring in synthetic peptide impurities, unnatural amino acids (UAA) are also often incorporated into novel peptide therapeutics to improve properties of the drug product. To date, the HLA binding properties of peptides containing UAA are not accurately estimated by most algorithms. Both scenarios warrant the need for enhanced predictive tools. The authors developed an in silico method for modeling the impact of a given UAA on a peptide’s likelihood of binding to HLA and, by extension, its immunogenic potential. In silico assessment of immunogenic potential allows for risk-based selection of best candidate peptides in further confirmatory in vitro, ex vivo, and in vivo assays, thereby reducing the overall cost of immunogenicity evaluation. Examples demonstrating in silico immunogenicity prediction for product impurities that are commonly found in formulations of the generic peptides teriparatide and semaglutide are provided. Next, this article discusses how HLA binding studies can be used to estimate the binding potentials of commonly encountered UAA and “correct” in silico estimates of binding based on their naturally occurring counterparts. As demonstrated here, these in vitro binding studies are usually performed with known ligands which have been modified to contain UAA in HLA anchor positions. An example using D-amino acids in relative binding position 1 (P1) of the PADRE peptide is presented. As more HLA binding data become available, new predictive models allowing for the direct estimation of HLA binding for peptides containing UAA can be established.
1 Introduction
1.1 Peptide drug products and T cell dependent immunogenicity
Ensuring drug safety and efficacy is of utmost importance for bringing novel and generic peptide drug products to market. Assessing the immunogenic potential of a given peptide drug product is a key element to safety and efficacy evaluations. Many factors can contribute to immunogenicity including the following: product origin (human vs. foreign); product-specific attributes (sequence, propensity for aggregation, purity, stability, mechanism of action, etc.); patient-specific factors (genetics, disease state, co-administered medications); administration factors (route of delivery, dose, and frequency); and immunomodulatory properties of the product (Singh, 2010; Ratanji et al., 2014). The focus of this paper is on the peptide sequence as it may determine immunogenic potential. A number of promising drug products have failed in clinical trials due to immunogenicity. One telling example is taspoglutide, a GLP-1 receptor agonist that was being developed for the treatment of diabetes. In 2010, the development of taspoglutide was halted during its phase three trial due to injection site and systemic allergic reactions as well as unacceptable levels of nausea and vomiting. Anti-taspoglutide antibodies were detected in 49% of patients in the study (Rosenstock et al., 2013). In general, T cell dependent immune responses can be attributed to T cell epitopes found within either the active pharmaceutical ingredient (API) or related impurities present in the final drug product. The latter is cause of the immunogenicity observed in the taspoglutide study. As outlined in the FDA’s abbreviated new drug application (ANDA) guidance to industry, peptide APIs and any peptide-related impurity sequences with relative abundance above 0.1% of the drug substance should be assessed for their potential to elicit an immune response. New impurities present at greater than 0.5% of the drug substance preclude submission of the product as an ANDA as it may require clinical studies for further evaluation (FDA - CDER, 2021). For those impurities that are present at acceptable levels, an in silico risk assessment can identify immunogenic risk at an early stage in the development process, enabling the removal of higher risk impurities while also indicating additional in vitro assays (HLA binding and T cell assay) that would be most useful in further evaluating immunogenicity.
Upon administration and circulation, biologic products and their impurities are taken up by antigen presenting cells, such as dendritic cells. As illustrated in Figure 1, proteolytic cleavage occurs upon processing inside the cell. Peptide fragments can then interact with the binding groove of human leukocyte antigens (HLA). Peptide-HLA complexes can then be trafficked to and presented on the cell surface. Once bound to HLA and presented on the surface, peptide epitopes are available for interaction with T cells. Naïve and memory T cells recognizing peptide-HLA complexes become activated and collaborate with antibody producing B cells thereby generating anti-drug antibodies potentially leading to safety and efficacy issues.
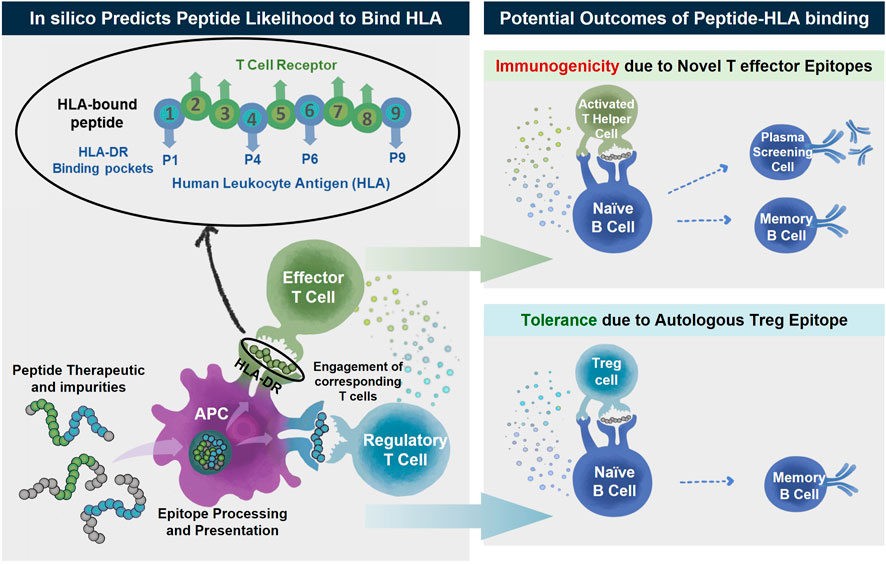
FIGURE 1. Peptide therapeutics and their impurities may contain T effector (green) or Treg (blue) epitopes. The peptides are taken up by antigen presenting cells where they are processed and any epitopes within the sequences are presented on the surface by human leukocyte antigen (HLA). The peptide-HLA complex can then engage either T effector or T regulatory cells. The activation of T effector cells (green) after engagement and recognition of T effector epitopes assists B cell maturation ultimately resulting in unwanted anti-drug antibody (ADA) development along with other immune reactions to the peptide drug product. In the absence of T effector epitopes or in the predominant engagement of T regulatory cells (blue) there is unlikely to be immune reactivity and generation of ADA. In silico immunogenicity prediction algorithms predict the likelihood for a peptide sequence to bind HLA, a first-step in immunological response.
HLA is one of the most polymorphic genes in the human genome. Each HLA has a unique structure and each one can accommodate a particular set of peptide epitopes. Class II HLA is associated with CD4+ T cell responses and drives anti-drug-antibody formation. The activation of CD4+ “helper” T cells is necessary for the initiation of significant and robust anti-therapeutic immune responses, including CD8+ T cell-mediated cytotoxic responses and B cell-mediated antibody responses. The binding grooves of Class II HLA are open-ended allowing for the presentation of longer peptide epitopes (15–25 amino acids in length), but the core binding region is just nine amino acids in length. The amino acids on either end of the binding 9-mer serve as flanking residues stabilizing the peptide in place. As illustrated in Figure 1, the side chains on amino acids in positions 1, 4, 6, and 9 are assumed to face downward where they can contact binding pockets located in the floor of the binding groove, locking the peptide into the HLA molecule (Stern et al., 1994). Amino acids in positions 2, 3, 5, 7, and 8 face outward, contacting the TCR of compatible T cells (Rudolph et al., 2006).
Class II HLA alleles can be subdivided into HLA-DR, HLA-DP, and HLA-DQ alleles. Most in silico algorithms focus on HLA-DR for the following reasons: these are the most prevalent Class II MHC molecules on the surface of antigen presenting cells; they contain polymorphisms only in the beta chain, leaving the alpha chain invariable; and they have been most strongly associated with ADA responses to therapeutic proteins (Hyun et al., 2021). In contrast, in both HLA-DP and HLA-DQ alleles, the alpha and beta chains of the heterodimer molecules are variable, making these alleles particularly challenging to model (Amatruda et al., 1987; Lecchi et al., 1989). Therefore, the immunogenic potential of a given therapeutic protein can be estimated based on the number and quality of the HLA Class II DR-restricted T cell epitopes it contains. Although there are over 4,000 known HLA-DR alleles (Robinson et al., 2020), several HLA-DR types that are common in humans share binding pocket preferences and thus can be grouped into a relatively small number of allele “supertypes.” A working set of nine Class II supertype alleles allows for the prediction of HLA binding covering the genetic backgrounds of over 95% of the human population worldwide (Southwood et al., 1998; Lund et al., 2004). To date, over 1.4 million peptidic epitopes have been catalogued by the Immune Epitope Database, a public resource of curated publications relating to T and B cell epitopes (Vita et al., 2018). These training data along with knowledge of the structure of HLA binding pockets allows for the creation of algorithms, such as EpiMatrix, to predict peptide binding to HLA.
1.2 In Silico tools for identifying putative T cell epitopes
In silico immunogenicity prediction is an important step in the development of novel or generic peptide drugs. There are many commercially or publicly available tools that are commonly utilized in the biopharma industry to assess the likelihood of an amino acid sequence to induce a T cell dependent immune response, such as EpiMatrix, NetMHC, Tepitope, SYFPEITHI, and others. These in silico tools use computer algorithms to assess the potential for an amino acid sequence to bind to HLA, a prerequisite for immunogenicity. As shown in Figure 2, the EpiMatrix algorithm is developed based on a careful curation of public data including ligand elution, HLA binding and T cell assay data. From these data, HLA and position specific coefficients are deduced for each of the 20 naturally occurring amino acids. Candidate peptides can be mapped against these coefficients to produce an immunogenicity score. High scoring peptides are more likely to bind HLA and activate T cells resulting in the induction of anti-drug immune responses.
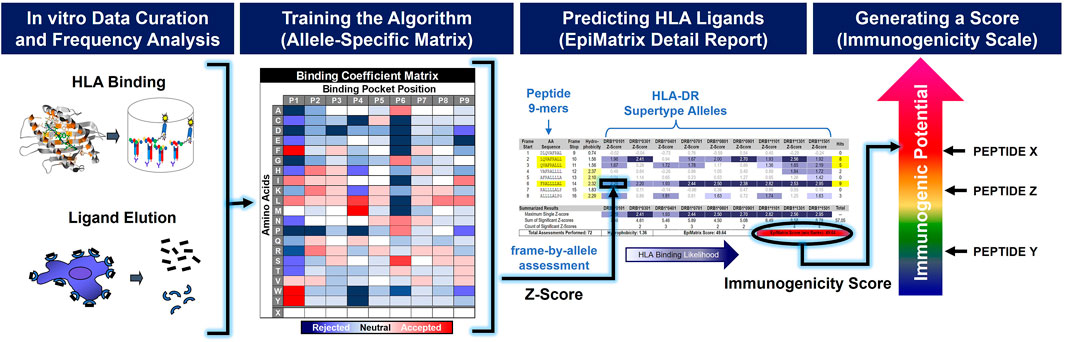
FIGURE 2. In silico immunogenicity scores are generated by prediction algorithms trained on in vitro data. Upon careful curation of in vitro data, a frequency analysis is applied in order to train the in silico algorithm. A 20 × 9 binding coefficient matrix is generated for each HLA-allele. These coefficients are used to generate a normalized 9-mer-by-allele binding assessment, or Z-score. The aggregation of significant Z-scores for a given peptide sequence provides a measure of immunogenic potential.
The authors have developed a predictive algorithm and associated coefficient set called EpiMatrix. EpiMatrix can be used to assess HLA binding likelihood to individual HLA-DR supertype alleles and therefore to generate predictions broadly applicable to a global population. For a global population analysis, EpiMatrix focuses the in silico Class II HLA binding predictions on nine HLA-DR representatives, one from each of the supertypes (Terry et al., 2015).
1.3 Immunogenic risk assessment for unnatural amino acid-containing peptides
Due to the availability of in vitro data supporting the modeling and training of in silico algorithms, EpiMatrix and other in silico immunogenicity prediction tools are limited to amino acid sequences composed of the 20 naturally occurring amino acids. In general, peptides composed purely of unmodified natural amino acids do not have optimal drug properties primarily due to their high rate of proteolytic cleavage and consequently short half-life (Di, 2015; Fosgerau and Hoffmann, 2015; Lee et al., 2019). Peptide drug developers often make use of unnatural amino acids (UAA) to achieve more optimal drug-like properties, such as increased half-life due to proteolytic resistance by incorporating D-amino acids (Di, 2015). For instance, when researchers discovered the benefits of GLP-1 in diabetes and developed a therapeutic analog, they found that the native human GLP-1 (7–37) half-life was too short to have the desired therapeutic effect (Deacon et al., 1995). Lipidation of the peptide provided an extended half-life and thus brought liraglutide to clinical utility (Knudsen et al., 2000; Tan et al., 2021; Victoza, 2022). Modification of the naturally occurring alanine in position 8 of hGLP-1 (7–37) to the UAA aminoisobutyric acid (Aib) prevented degradation by dipeptidyl peptidase IV (DPP-IV) and further increased the half-life (Deacon et al., 1995), bringing semaglutide to the market (Ozempic, 2022; Rybelsus, 2022).
Historically, in order to assess the immunogenic risk of sequences containing UAA, costly in vitro assays such as HLA binding assays or T cell activation assays have been required. In the future, as more HLA binding and ligand elution data become available for sequences containing UAA, in silico immunogenicity prediction tools will be able to handle sequences containing the most common UAA. However, peptide drug developers have an immediate need for assessing immunogenic risk of a given peptide drug substance and related impurities that may contain UAA. In this article, we present a new method using currently available in silico tools to estimate the immunogenic potential of peptides containing UAA. This allows for a rapid and inexpensive process to assess immunogenic risk as a first step and potentially eliminate the need for additional, more costly and time-consuming in vitro assays.
As will be discussed in detail below, the authors have established a three-step process for evaluating UAA using existing allele- and position-specific matrices plus two special coefficients, one describing a neutral binding profile and a second describing an unfavorable binding profile. In the first step, the UAA is replaced with a neutral placeholder. Here, the goal is to establish the binding potential of the other amino acids present in the candidate 9-mer. Next, the UAA is iteratively replaced with each of the 20 naturally occurring amino acids. Here, the goal is to characterize the sensitivity of the candidate peptide to changes in the position occupied by the UAA. Finally, the physical properties of the UAA is compared to each of the 20 naturally occurring amino acids in order to select a “best proxy.” While this method allows for the rapid in silico analysis of UAA-bearing peptides it is limited to cases where a reasonable best proxy can be identified.
The next step will involve compiling a set of “correction factors” that can be applied to in silico predictions for common UAA. Correction factors will evolve over the course of three steps including expert review, validation in vitro, and validation ex vivo. The first involves a review of the UAA side chain structure compared to the closest matching natural amino acid and the application of deductions (i.e., minimal, moderate, significant impact). Next, HLA binding confirmation studies are performed in support of the development of “correction factors” which can be applied to the in silico risk prediction of sequences containing the most common unnatural amino acids, such as D-amino acids. The “correction factors” are then further refined by data from ex vivo T cell assays evaluating the impact of the selected UAA on T cell recognition and immune response.
With the generation of more in vitro and ex vivo “training” data, predictive coefficients for common UAA will be directly incorporated into the structure of immunogenicity risk assessment tools, such as EpiMatrix (Figure 3).
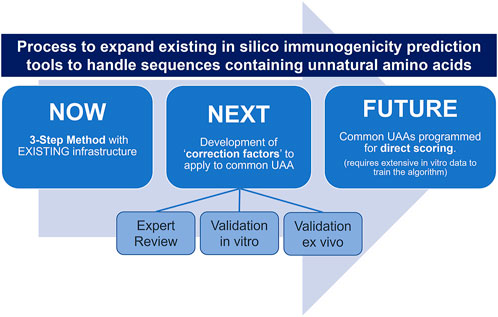
FIGURE 3. A tiered approach for expanding in silico immunogenicity prediction tools (such as EpiMatrix) to handle sequences containing UAA.
2 Methods and materials
The 3-step method to assess immunogenic potential in sequences containing UAA presented in this article uses EpiMatrix, a tool initially developed by Bill Jesdale and improved and further developed by Bill Martin (De Groot et al., 2003), but it is important to note that it can be applied to other publicly available in silico immunogenicity prediction tools as well.
2.1 EpiMatrix
EpiMatrix is a proprietary matrix-based prediction algorithm in which a given amino acid input sequence is assigned an immunogenicity score based on its putative T cell epitope content. As shown in Figure 2, the EpiMatrix algorithm is developed based on a careful curation of public data including ligand elution, HLA binding and T cell assay data. After review and qualification, observed ligands are separated by allele and their sequences are aligned. Position-specific frequency distributions are then compared to statistical expectations and coefficients of binding affinity are established for each of the 20 naturally occurring amino acids across each of the nine positions in the HLA binding groove. The resulting allele-specific 20 × 9 coefficient matrix can be used to estimate the HLA binding potential of any 9-mer peptide. The matrix coefficients have been updated periodically since 1998 (De Groot et al., 2003).
In order to estimate the immunogenic potential of a candidate therapeutic peptide or protein, EpiMatrix will parse the input sequence into overlapping 9-mer frames and assess each frame for binding potential with respect to nine common HLA alleles including: DRB1*0101, DRB1*0301, DRB1*0401, DRB1*0701, DRB1*0801, DRB1*0901, DRB1*1101, DRB1*1301, and DRB1*1501. Taken collectively, these alleles offer coverage of approximately 95% of the global population (Southwood et al., 1998). Individual scores are then aggregated and normalized to produce a standardized score. Scores above zero indicate that the input protein contains more predicted T cell epitopes than expected for a peptide/protein of its length and demonstrate an increased potential for immunogenicity. Scores below zero indicate that the input protein contains fewer predicted T cell epitopes than expected for a peptide/protein of its length and demonstrate a decreased potential for immunogenicity. EpiMatrix immunogenicity scores are correlated with clinical immunogenicity (De Groot and Martin, 2009). An example of an EpiMatrix Detail report is shown in Figure 4.
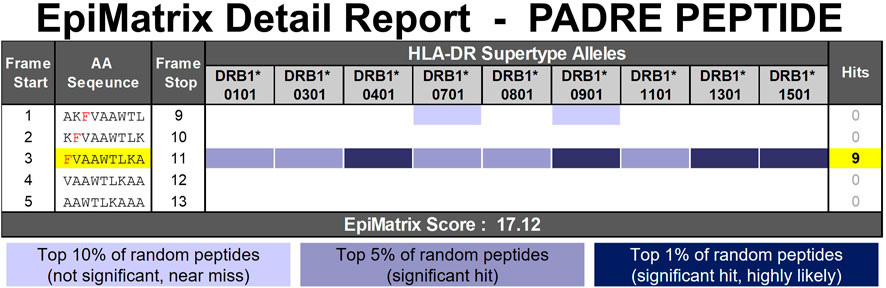
FIGURE 4. EpiMatrix Detail Report for a known promiscuous HLA-DR binding peptide, PAN-HLA-DR-epitope, or PADRE. The potential of a 9-mer frame to bind to a given HLA allele is indicated by a Z-score (scores omitted for simplicity); the strength of the score is indicated by the blue shading. All scores in the top 5% (Z-Score ≥ 1.64) are considered “Hits” (medium and dark blue shading). Scores in the top 10% are considered elevated, but not significant (light blue shading). Frames containing four or more alleles scoring above 1.64 are referred to as EpiBars and are highlighted in yellow. These frames have an increased likelihood of binding to a range of HLA alleles.
2.2 NOW—3-step method
The EpiMatrix algorithm can be used to score any amino acid sequence composed of naturally occurring amino acids. The binding potential of UAA cannot be directly estimated by the EpiMatrix system. The method outlined here describes a 3-step process used to assess the impact UAA can have on the HLA binding potential of a peptide and to ultimately select naturally occurring proxies for commonly encountered UAA. This method is applicable to amino acid sequences containing no more than one UAA within a 9-mer span. The method described here uses the well-known promiscuous HLA-DR binding peptide, known as PAN-HLA-DR-epitope or PADRE (AKFVAAWTLKAAA) (Alexander et al., 1994), modified with a 1-napthylalanine residue in position 3 as an example (AK1NalVAAWTLKAAA). Figure 4 provides the EpiMatrix Detail report of the PADRE peptide. PADRE is characterized by high affinity binding across HLA-DR alleles due to the presence of key amino acids in a single frame of the peptide. Examples demonstrating this 3-Step in silico method for immunogenicity prediction of product impurities commonly found within formulations of the generic peptides teriparatide and semaglutide are provided in the results section.
In the first step, the UAA is replaced with a neutral placeholder, the letter X, and uploaded into the EpiMatrix system. The neutral placeholder X has a binding coefficient of 0 and therefore neither promotes nor detracts from predicted HLA binding. With the neutral placeholder X substituted for the UAA, one can see the impact the other amino acids in each isolated 9-mer can have on predicted HLA binding. Figure 5: Step 1 shows how 1-napthylalanine is replaced with the neutral placeholder X.
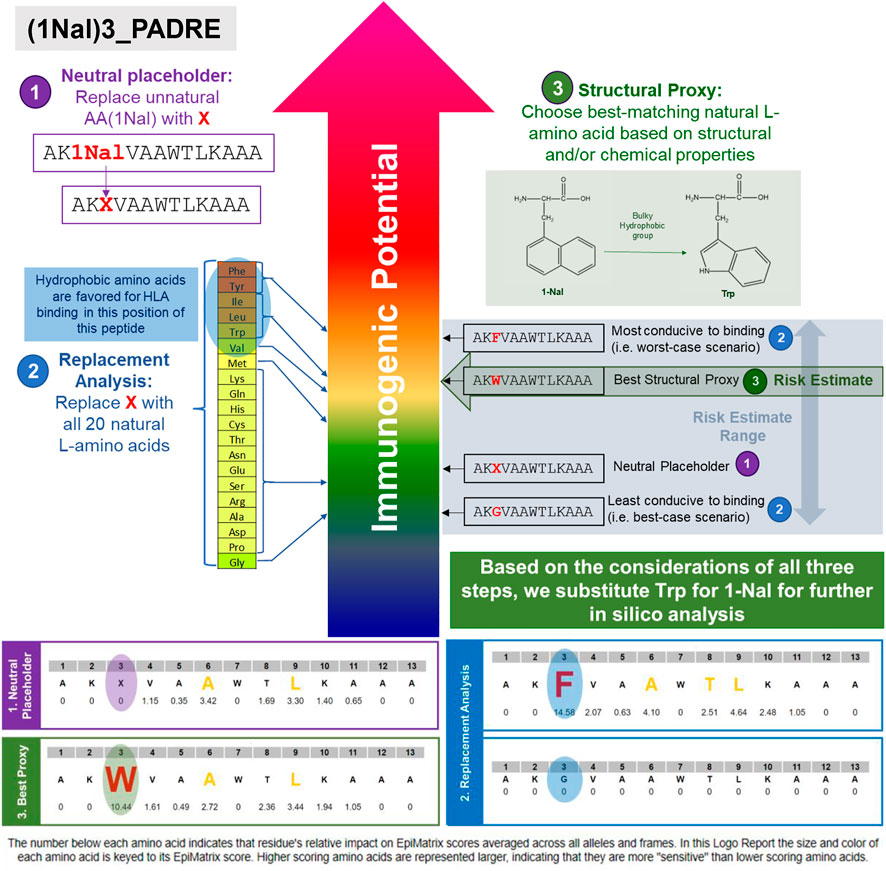
FIGURE 5. 3-Step in silico immunogenicity risk assessment method for sequences containing UAA is illustrated in this example using PADRE-3(1Nal). In step 1, the UAA (1Nal) is replaced with the neutral placeholder “X.” In step 2, a replacement analysis is performed by substituting X with all 20 naturally occurring L-amino acids to determine a range of possible binding affinities. In step 3, the structural and chemical properties of the UAA residue is evaluated and replaced with the closest matching natural L-amino acid, if available. In this case, tryptophan is selected as the best available substitute for 1-Nal.
In the second step, the potential impact the UAA can have on HLA binding is studied by performing a replacement analysis. In this step, the X is iteratively replaced with all 20 of the naturally occurring amino acids for which prediction coefficients are established. The range of scores generated is indicative of the potential impact any side chain in this particular position can have on HLA binding. A wide range of scores indicates that the UAA-containing position can have a significant impact on the HLA binding properties of the peptide. A narrow or negligible range of scores indicates that the UAA-containing position will have little to no impact on HLA binding properties. In the latter case, this may be evidence enough to eliminate the need for further immunogenicity studies. From this variation analysis, patterns regarding the properties of the substituted amino acids and the scores that are generated can be studied. For instance, in the example provided in Figure 5: Step 2, varying the amino acid substituted into position three reveals that hydrophobic amino acids substituted into this position of the baseline PADRE peptide generated higher scores relative to other amino acid substitutions, indicating that, in general, hydrophobic side chains promote HLA binding.
In the third step, a substitution is selected for further in silico analysis. In this step, the chemical and structural properties of the unnatural amino acid are assessed. If there is a well-matched naturally occurring amino acid in terms of overall properties, then that amino acid can be substituted for further in silico analysis (structural proxy). In many cases, there will not be a well-matched naturally occurring amino acid. In these instances, one can assign a placeholder based on the findings from step 1 and step 2. In EpiMatrix, aside from the 20 naturally occurring amino acids, there are two placeholders that can be utilized. The first is the neutral placeholder, which is defined in step one. The second is the low-affinity placeholder. This placeholder imputes a low binding coefficient and is reserved for instances where the neutral placeholder will likely represent an overestimate in HLA binding. The low-affinity placeholder may be used to represent very large sidechains that are likely to cause steric hindrance and disrupt potential HLA binding. Examples include PEGylated sidechains and the fatty acid group fused to the lysine of liraglutide. For this 1Nal3-PADRE example, the closest matching natural amino acid in terms of overall structural and chemical properties of the 1-napthylalanine side chain is tryptophan (Figure 5: Step 3). Both 1-Nal and Trp contain hydrophobic side chains with relatively bulky aromatic groups. In this example, 1-Nal can be replaced with Trp for further in silico analysis including epitope prediction with EpiMatrix.
2.3 Peptide synthesis
The semaglutide API and D-amino acid impurity peptides used in these studies were synthesized by Vivitide (Gardner, MA, United States). Molecular weight was verified by mass spectrometry and all peptides were determined to be >90% pure by HPLC. These peptides were manufactured with trifluoroacetic acid salt and net peptide concentration was confirmed with amino acid analysis. The modified PADRE peptides in the preliminary D-amino acid correction factor studies were synthesized by 21st Century Biochemicals (Marlborough, MA, United States). Molecular weight was verified by mass spectrometry and all peptides were determined to be >90% pure by HPLC. These peptides were manufactured with acetate salt.
2.4 In vitro human leukocyte antigen binding assay
Class II HLA binding assays are used to validate in silico binding predictions and measure the relative binding affinity of potentially immunogenic peptides. This assay is also being utilized to develop correction factors for common unnatural amino acids. The competition-based assay used in this study has been adapted from Steere et al. (2006). It yields an indirect measure of peptide-HLA affinity. Binding is measured against seven HLA DRB1 “supertype” alleles: DRB1*0101, DRB1*0301, DRB1*0401, DRB1*0701, DRB1*0901, DRB1*1101, and DRB1*1501. Based on peptide titration with seven concentrations, non-linear regression analysis is performed to produce a curve from which an IC50 value is calculated and used to assess binding strength. Briefly, unlabeled test peptides are incubated overnight to equilibrium with a soluble HLA DR molecule (Benaroya Research Institute, Seattle, Washington) and a biotinylated, allele-specific competitor peptide of known binding affinity. The binding reaction is then neutralized, and peptide-HLA complexes are transferred to a 96-well plate coated with the pan-HLA DR antibody, clone L243 (Biolegend) and incubated overnight. The following day, plates are resolved by the addition of Europium-labeled streptavidin (Perkin-Elmer Waltham, MA). An indirect measure of binding is determined by time-resolved fluorescence. Each peptide is evaluated in triplicate over a range of seven concentrations. The percent inhibition values for each experimental peptide across a range of concentrations is used to calculate an IC50, the concentration at which the test peptide inhibits 50% of the labeled competitor peptide. Peptides are categorized by the following HLA-DR binding affinity cutoffs: Non-Binder (No dose dependent inhibition), Negligible Affinity (100,000 nM < IC50 < 1,000,000 nM), Low Affinity (10,000 nM < IC50 < 100,000 nM), Moderate Affinity (1,000 nM < IC50 < 10,000 nM), High Affinity (100 nM < IC50 < 1,000 nM), Very High Affinity (IC50 < 100 nM).
3 Results
3.1 Illustrating the 3-step method with teriparatide and semaglutide
This section provides an illustration of the 3-step method for assessing immunogenic risk using two generic peptide APIs, teriparatide and semaglutide, and some of their commonly encountered impurities.
3.1.1 Example 1. Teriparatide oxidation impurities
The amino acid sequence of teriparatide is derived from the N-terminal 34 amino acids of human parathyroid hormone (hPTH). It is entirely composed of natural amino acids and contains two methionine residues in positions 8 and 18. Oxidation of the methionine residues within a peptide is a common occurrence and is frequently identified in drug product impurities analysis (Grassi and Cabrele, 2019; D'Hondt et al., 2014). Traditional in silico immunogenicity prediction algorithms cannot accommodate sequences containing methionine sulfoxide (or sulfone) residues. For the purposes of this analysis, methionine sulfoxide (MetO) residues are considered unnatural amino acids (UAA). The 3-step in silico method described above can be utilized to determine the potential impact this modification can have on the HLA binding properties of the peptide. As shown in the EpiMatrix Detail report in Figure 6, teriparatide contains a promiscuous HLA binding motif (EpiBar) in frame five and additional predicted HLA ligands in frames 4, 7, 8, 11, 21, and 23. The two methionine residues occur in predicted HLA ligands present in frames 4, 5, 7, 8, and 11. The methionine in position 8 occurs within the epitope dense N-terminal region of the peptide and modification to this position impacts the predicted HLA ligands present in frames 4, 5, 7, and 8. The methionine in position 18 occurs within a more epitope sparse region of the peptide and impacts the single predicted HLA ligand present in frame 11.
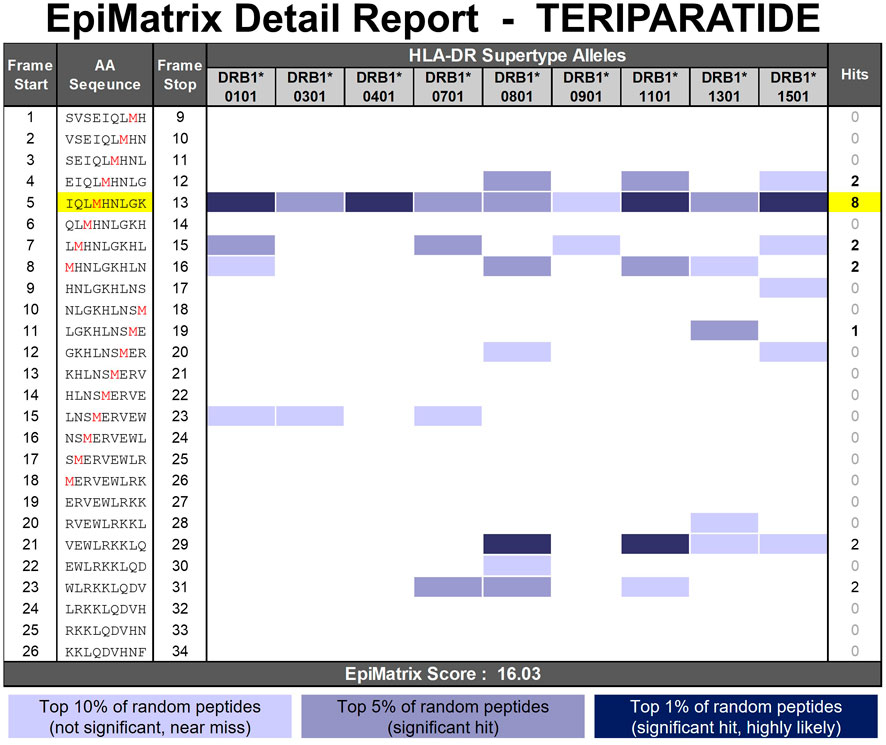
FIGURE 6. EpiMatrix Detail Report for teriparatide. The potential of a 9-mer frame to bind to a given HLA allele is indicated by a Z-score (scores omitted for simplicity); the strength of the score is indicated by the blue shading. All scores in the top 5% (Z-Score ≥ 1.64) are considered “Hits” (medium and dark blue shading). Scores in the top 10% are considered elevated, but not significant (light blue shading). Frames containing four or more alleles scoring above 1.64 are referred to as EpiBars and are highlighted in yellow. These frames have an increased likelihood of binding to a range of HLA alleles. The two methionine residues prone to oxidation are shown in red font.
As illustrated in Figure 7 (left side), in the first step, to enable upload into the EpiMatrix system, the UAA in position 8 is replaced with the neutral placeholder, X. By substituting X for M in position 8 one can see that frame 5 has significant binding potential even if the contribution of M8 is nullified. Next, in the replacement analysis, the X is substituted with each of the 20 naturally occurring amino acids. From this step, one can see that there is a significant range of predicted HLA ligands, or EpiMatrix hits, generated (from nine to 20), indicating that modification in this position can have a significant impact on predicted HLA binding. Notably, however, relative to both the baseline (M in position 8, 19 hits) and to the neutral placeholder (X in position 8, 19 hits), most substitutions yield fewer predicted HLA ligands. This indicates that modification in position 8 of the teriparatide peptide has the potential to disrupt HLA binding events. Only two natural amino acid substitutions marginally increase the overall score relative to the baseline, indicating that modification in position 8 of the teriparatide peptide only has a slight potential to introduce additional HLA binding events relative to the baseline.
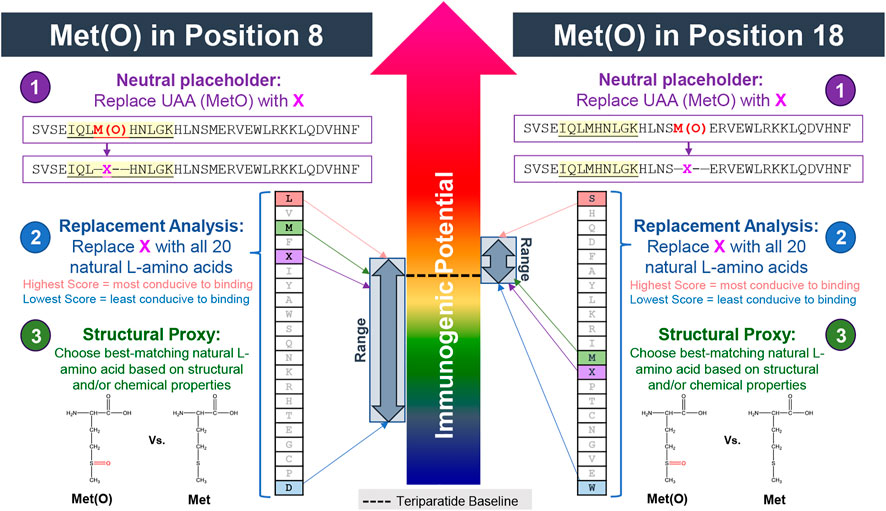
FIGURE 7. Assessing the impact of methionine oxidation on predicted HLA binding in positions 8 and 18 of teriparatide using the 3-step method. In step 1, the UAA (MetO) is replaced with the neutral placeholder “X.” In step 2, a replacement analysis is performed by substituting X with all 20 naturally occurring L-amino acids to determine a range of possible binding affinities. In step 3, the structural and chemical properties of the UAA residue is evaluated and replaced with the closest matching natural L-amino acid, if available. In this case, unmodified methionine is selected as the best available substitute for Met(O). Modification to the methionine in position 8 (left side) is predicted to have a significant (likely reducing) impact on HLA binding properties relative to the baseline. Modification to the methionine in position 18 (right side) is likely to have a minimal impact on HLA binding properties relative to the baseline but does carry risk of introducing new T cell epitope content.
When performing the same analysis for Met(O) in position 18 (Figure 7, right side), there is a much narrower range of predicted HLA ligands generated (from 19 to 22), indicating a more limited potential to impact the HLA binding properties of the peptide compared to modification in position 8. However, as evident by 11 of 20 natural amino acid substitutions generating a higher EpiMatrix Score than the baseline peptide or neutral placeholder peptide, modification to the methionine in position 18 of teriparatide has the potential to result in the creation of additional epitope content, relative to the baseline API peptide.
Step 3 of the UAA substitution analysis is the same regardless of the position of the UAA. In this step, when considering the overall structural and chemical properties of Met(O), there is not a well-matched natural amino acid for substitution. The closest-matching natural amino acid is the unmodified methionine residue. Given the limited impact observed when substituting X for Met and the limited structural and chemical differences between Met and Met(O), Met has been accepted as a reasonable substitute for Met(O). The authors hypothesize that HLA binding pockets have co-evolved with the naturally occurring amino acids and that most unnatural amino acids will be less well adapted to HLA binding than their naturally occurring proxies. Therefore, the substitution of Met for Met(O) may lead to a slight overestimate of HLA binding potentials. The predicted decrease in HLA binding relative to the baseline can be confirmed with in vitro HLA binding studies.
This analysis has established that the modification from Met to Met(O) may result in a limited reduction in HLA binding. However, the immunogenicity assessment must consider factors in addition to HLA binding. In most cases, peptide epitopes derived from human proteins are assumed to be tolerated by the human immune system. T cells capable of recognizing these human derived peptide/HLA complexes may be deleted or rendered anergic in the thymus before being released to the periphery. In some cases, these cognate T cells have a regulatory phenotype. The presence of UAA that change the TCR-facing contour of peptide/HLA complexes may alter T cell recognition patterns allowing effector T cells to engage UAA-containing peptide/HLA complexes that would normally be ignored by the human immune system. In this case the UAA in position 8 occurs in TCR-facing positions in frames 4 and 7 while the UAA in position 18 occurs in a TCR-facing position in frame 11. In both cases, new T cell responses could be induced. Therefore, the presence of UAA in positions 8 and 18 could result in increased immunogenicity despite the predicted decrease in HLA binding affinity. The immunological impact of the two Met(O) for Met substitutions present in this impurity could be studied in ex vivo T cell induction assays.
3.1.2 Example 2. Semaglutide active pharmaceutical ingredient
Semaglutide is a GLP-1 receptor agonist with 94% sequence homology to native hGLP-1. Semaglutide differs from hGLP-1 (7–37) by three distinct modifications. First, the lysine in position 26 has been modified with a C18 diacid connected to the lysine side chain via a mini PEG spacer and γ-glutamic acid (OEG-OEG-γGlu-C18 diacid). The fatty acid chain reversibly binds human serum albumin in vivo while the mini PEG spacer provides flexibility to allow for improved binding to the receptor (Tan et al., 2021). Additionally, a modification from lysine to arginine in position 34 was introduced to ensure direct fatty acid conjugation to the lysine in position 26 (Knudsen et al., 2000). Finally, the naturally occurring alanine in position 8 is modified to α-aminoisobutyric acid to reduce degradation by DPP-IV (Deacon et al., 1995). These modifications were designed into the semaglutide API to extend the half-life relative to native hGLP-1 (only 1–2 min) (Deacon et al., 1995) allowing for a once-weekly injectable administration (Ozempic®) (Ozempic, 2022) or a once-daily oral administration (Rybelsus®) (Rybelsus, 2022).
In order to assess the immunogenic potential of the semaglutide API peptide in silico, the 3-step method can be applied to select substitutions for both the Aib residue and the Lys (OEG-OEG-γGlu-C18diacid) residue. These two UAA positions occur in two different areas of the semaglutide peptide and the 9-mers impacted by each unnatural residue do not overlap. The 3-step method is applied to each UAA separately and is demonstrated in Figure 8.
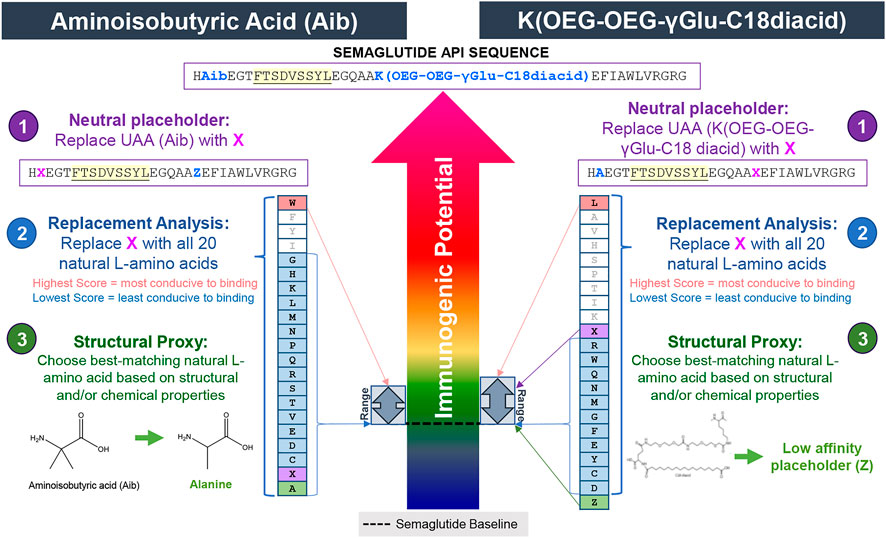
FIGURE 8. Selecting substitutes for the UAA in semaglutide API using the 3-step method. In step 1, the UAA Aib and the UAA K(OEG-OEG-γGlu-C18 diacid) are replaced with the neutral placeholder “X.” In step 2, a replacement analysis is performed by substituting X with all 20 naturally occurring L-amino acids to determine a range of possible binding affinities. In step 3, the structural and chemical properties of the UAA residue is evaluated and replaced with the closest matching natural L-amino acid, if available. In this case, alanine is selected as the best available substitute for Aib while the low affinity placeholder “Z” is selected for the K(OEG-OEG-γGlu-C18 diacid) residue. A narrow range of scores are generated in the replacement analysis for both residues indicating that both of these positions will have very little impact on the HLA binding potential of the semaglutide peptide in general.
First, the 3-step method is applied to the Aib in position 8. Using the neutral placeholder X at position 8, one can see that there are 10 EpiMatrix hits within the semaglutide sequence. None of the ligands predicted by EpiMatrix are found in a 9-mer frame that contains X suggesting that the Aib containing region of semaglutide does not contain significant HLA binding potential. Not surprisingly, the replacement analysis suggests that natural amino acid substitutions in position 8 have only a minimal impact on potential HLA binding. Variation in position 8 has only a small potential to create new putative T cell epitope content relative to the neutral placeholder, X. In addition to the neutral placeholder, X, substitution with 16 of the 20 natural amino acids also produces no predicted HLA ligands. The amino acid substitution most conducive to binding in this position is tryptophan, which only creates one new predicted HLA ligand relative to the neutral placeholder. In the third step, the overall structural and chemical properties of Aib are considered for the selection of a substitute. As shown in Figure 8, bottom left, Aib has two methyl groups making up its side chain. The closest structural proxy is Ala. Like X, the substitution of alanine for Aib creates no new predicted HLA ligands. Therefore, Ala has been accepted as a reasonable substitute for Aib.
Next, the 3-step method is applied to select a substitute for Lys (OEG-OEG-γGlu-C18 diacid) at position 26. Using the neutral placeholder X at position 26, one can see that there are 11 EpiMatrix hits within the semaglutide sequence. Only one of the HLA ligands predicted by EpiMatrix is found in a 9-mer frame that contains X. Again, the replacement analysis suggests that natural amino acids substitutions in position 26 have only a minimal impact on potential HLA binding. Variation in position 26 has only a small potential to create new putative T cell epitope content, relative to the neutral placeholder, X. There are 11 natural amino acid substitutions that produce no predicted HLA ligands. The amino acid substitution most conducive to binding is leucine, which creates one new predicted HLA ligand relative to the neutral placeholder. Comparing Lys (OEG-OEG-γGlu-C18 diacid) to the 20 naturally occurring amino acids, there is not a well-matched naturally occuring proxy for this large moiety. Assuming it remains intact during antigen processing, the C18 diacid-γGlu-OEG-OEG group is likely to cause steric hindrance that may reduce or disrupt HLA binding. Considering this, it is expected that replacing the Lys (OEG-OEG-γGlu-C18 diacid) moiety with the neutral placeholder X will yield an overestimate of HLA binding potential. In cases where the neutral placeholder results in an overestimate in HLA binding potential, it is preferable to use the low-affinity placeholder “Z.” In the EpiMatrix scoring system, for each allele and binding position, amino acid Z imputes the value of the lowest affinity natural amino acid. Z has been accepted as a reasonable substitute for Lys (OEG-OEG-γGlu-C18 diacid).
In summary, to enable further in silico analysis, the semaglutide API peptide sequence is modified to HAEGTFTSDVSSYLEGQAAZEFIAWLVRGRG. Based on the 3-step method to select the A and Z substitutions, modification in either position is expected to have little impact on the HLA binding properties of the semaglutide API peptide. This observation is consistent with the semaglutide API EpiMatrix Detail Report (Figure 9) which predicts promiscuous HLA binding in frame 6 as well as additional HLA ligands at the C-terminus. Both the Aib in position 8 and the large group in position 26 occur in regions of the peptide that are devoid of any significant predicted epitope content (see frame start 7–8 for Aib and frame start 18–26 for C18 group).
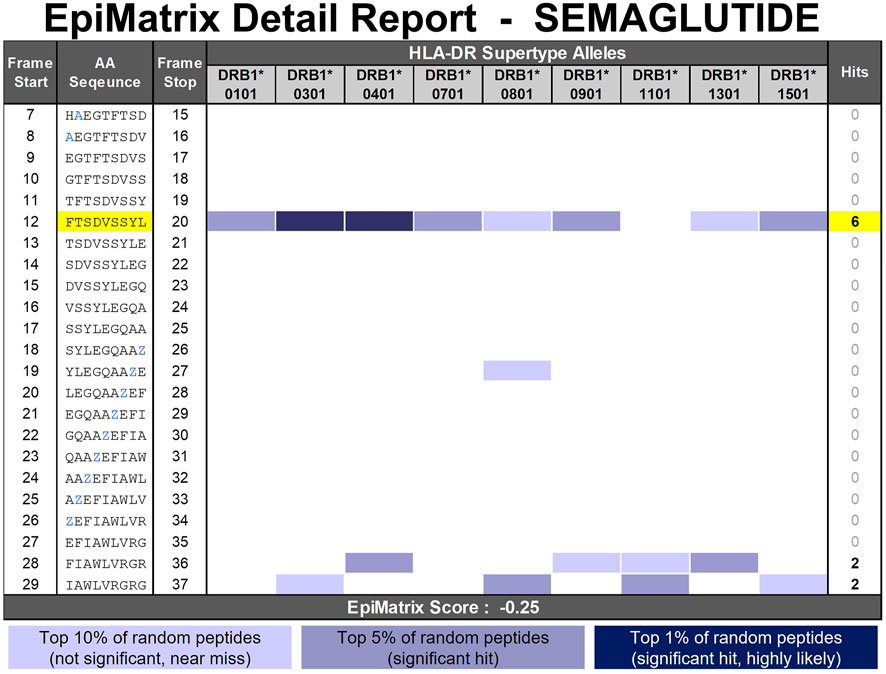
FIGURE 9. EpiMatrix Detail Report for Semaglutide. The potential of a 9-mer frame to bind to a given HLA allele is indicated by a Z-score (scores omitted for simplicity); the strength of the score is indicated by the blue shading. All scores in the top 5% (Z-Score ≥ 1.64) are considered “Hits” (medium and dark blue shading). Scores in the top 10% are considered elevated, but not significant (light blue shading). Frames containing four or more alleles scoring above 1.64 are referred to as EpiBars and are highlighted in yellow. These frames have an increased likelihood of binding to a range of HLA alleles. Selected placeholder substitutions to enable in silico analysis are shown in blue font. Z represents the low-affinity placeholder which imputes the lowest natural amino acid coefficient for each frame-by-allele assessment.
3.1.3 Example 3. Semaglutide D-amino acid impurities
Here, the 3-step method is applied to two D-amino acid impurities occurring in semaglutide–D-His7 and D-Phe12. The process is illustrated in Figure 10.
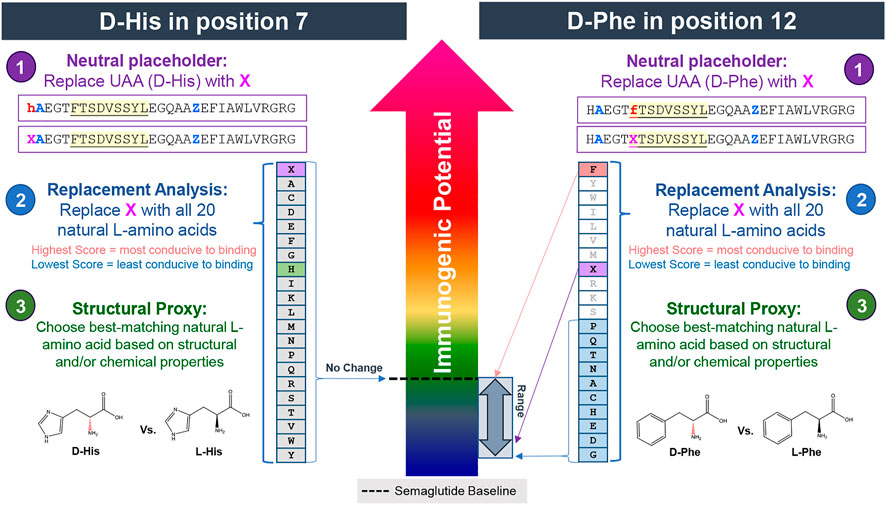
FIGURE 10. Assessing the impact of D-amino acids on predicted HLA binding in positions 7 and 12 of semaglutide using the 3-step method. In step 1, the UAA is replaced with the neutral placeholder “X.” In step 2, a replacement analysis is performed by substituting X with all 20 naturally occurring L-amino acids to determine a range of possible binding affinities. In step 3, the structural and chemical properties of the UAA residue is evaluated and replaced with the closest matching natural L-amino acid, if available. In this case, the natural L-isomers is selected as the best available substitute for D-His while the neutral placeholder X is selected as the substitute for D-Phe. Modification to the histidine in position 7 (left side) is predicted to have no impact on HLA binding properties relative to the baseline. Modification to the phenylalanine in position 12 (right side) is likely to have a significant (likely reducing) impact on HLA binding properties relative to the baseline.
In the first of the two D-amino acid impurity examples, the N-terminal histidine of the semaglutide peptide has been enantiomerized to its D-isomer. In the first step, the sequence is uploaded with the neutral placeholder X at the N-terminus (position 7 relative to hGLP-1). The X is then iteratively replaced with all 20 of the naturally occurring amino acids. This step reveals that neither the neutral placeholder X nor any of the 20 naturally occurring amino acids produces any predicted HLA ligands or has any effect on the resulting EpiMatrix Score relative to the baseline peptide (Figure 10, left side). This implies that modification at this position of the peptide is unlikely to impact the HLA binding properties of the molecule and therefore is predicted to have an insignificant impact on the risk profile of the baseline sequence. In this case, L-His was accepted as a reasonable substitute for D-His.
In the second D-amino acid impurity example, the phenylalanine in position 12 of the semaglutide peptide has been enantiomerized to its D-isomer. Contrary to the D-His7 example occurring in an area devoid of any putative T cell epitope content, the phenylalanine occurs in relative position one of a predicted promiscuous HLA binding motif (see frame start 12 in Figure 9). As evidenced by the wide range of scores generated in the replacement analysis (Figure 10, right side), modification to this position can have a significant impact on the HLA binding potential of the peptide. Natural amino acid substitutions in this position all result in a lower EpiMatrix Score and a reduction of predicted HLA ligands relative to the baseline sequence and therefore this analysis indicates that modification in this position of this peptide has a significant potential to disrupt HLA binding but does not have significant potential to create new HLA binding events relative to the baseline peptide. From the available literature (Azam et al., 2021) and extensive experience with evaluation of generic peptides, it is clear that the presence of D-amino acids in synthesized peptides significantly reduces binding affinity relative to their L-amino acid counterparts. In this case, the neutral placeholder X was selected to represent D-Phe12. This substitution reduces four predicted HLA ligands compared to the semaglutide baseline sequence (green boxes in Figure 11).
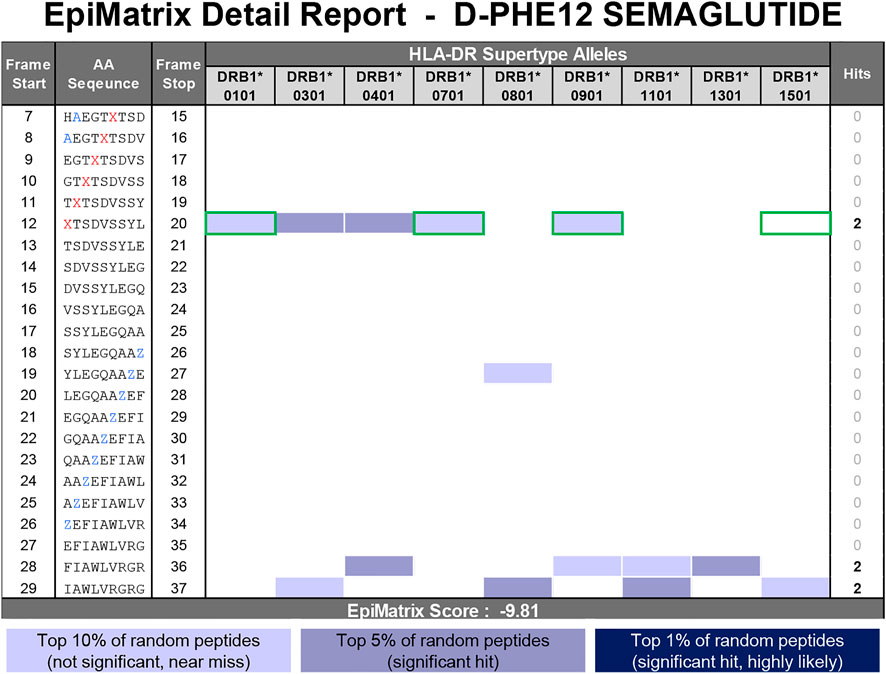
FIGURE 11. EpiMatrix Detail Report for D-Phe12 semaglutide impurity. The potential of a 9-mer frame to bind to a given HLA allele is indicated by a Z-score (scores omitted for simplicity); the strength of the score is indicated by the blue shading. All scores in the top 5% (Z-Score ≥ 1.64) are considered “Hits” (medium and dark blue shading). Scores in the top 10% are considered elevated, but not significant (light blue shading). Frames containing four or more alleles scoring above 1.64 are referred to as EpiBars and are highlighted in yellow. These frames have an increased likelihood of binding to a range of HLA alleles. Selected placeholder substitutions to enable in silico analysis are shown in blue font. Z represents the low-affinity placeholder which imputes the lowest natural amino acid coefficient for each frame-by-allele assessment. The D-Phe residue is replaced with the neutral placeholder X in red font. Green boxes indicate the loss of predicted HLA ligands found in the semaglutide API peptide.
The predicted impact of the two D-amino acid impurities on the HLA-binding properties of the semaglutide peptide is supported by in vitro HLA binding studies performed and shown below (Figure 12). In this study, the D-HIS7_SEMAGLUTIDE (7–23) impurity peptide had a similar binding profile compared to the baseline peptide SEMAGLUTIDE (7–23), whereas the D-PHE12_SEMAGLUTIDE (9–23) impurity peptide yielded a reduction in HLA binding relative to the SEMAGLUTIDE (9–23) baseline peptide.
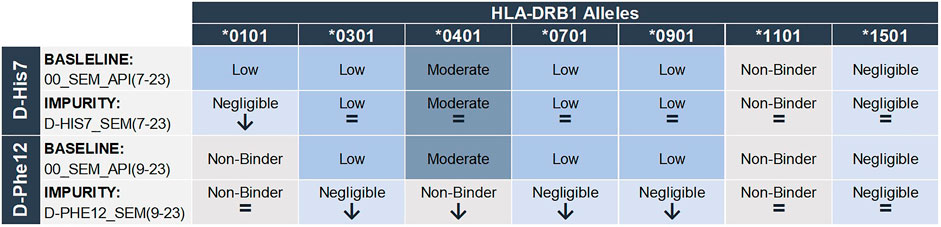
FIGURE 12. In vitro HLA binding results for D-His7 and D-Phe12 impurities compared to their corresponding semaglutide API baseline sequence. Peptides are categorized by the following HLA-DR binding affinity cutoffs: Non-Binder (No dose dependent inhibition), Negligible Affinity (100,000 nM < IC50 < 1,000,000 nM), Low Affinity (10,000 nM < IC50 < 100,000 nM), Moderate Affinity (1,000 nM < IC50 < 10,000 nM), High Affinity (100 nM < IC50 < 1,000 nM), Very High Affinity (IC50 < 100 nM).
4 Discussion
The assessment of HLA binding potentials is an important first step to understanding the immunogenic potential of any given peptide or protein therapeutic. In silico immunogenicity tools, such as EpiMatrix, predict whether or not a given amino acid sequence is likely to bind HLA and therefore likely to be presented on the surface of an antigen presenting cell where it can be recognized by T cells. These in silico algorithms are trained based on vast amounts of curated data including HLA ligand elution, HLA binding, and T cell assay data. In general, they show remarkable accuracy at estimating the potential of naturally occurring amino acid sequences to bind to specific HLA haplotypes.
Peptide drugs produced by synthetic means often include UAA-containing impurities. In addition, UAA are now more frequently incorporated into novel peptide and protein therapeutics to improve properties such as half-life and stability. There is an immediate and ever-growing need to assess the immunogenic potential of peptide sequences that contain UAA. However, there is only a very limited amount of training data available for sequences containing UAA. As a result, most, if not all, in silico immunogenicity prediction algorithms are limited to sequences composed entirely of natural amino acids.
4.1 Now–The 3-step in silico method for unnatural amino acid-containing sequences
This 3-step method provides a needed immediate solution, enabling in silico immunogenicity risk assessment for sequences containing UAA. The 3-step method leverages existing in silico capabilities, information pertaining to the physical and chemical properties of natural and unnatural amino acids and immunoinformatic expertise to establish proxies for commonly encountered UAA that can be used within existing in silico immunogenicity prediction tools.
The 3-step in silico risk assessment method for sequences containing UAA provides a fast and inexpensive way to understand the potential impact that UAA occurring at a specific position of a specific peptide can have on HLA binding properties. In some cases, this step could eliminate the need for further, more time-consuming and costly in vitro immunogenicity studies. In other cases, the in silico risk assessment can inform and direct the proper in vitro immunogenicity assay. For instance, the HLA binding assay is a non-cellular assay using HLA monomers. Without cellular processing of the peptides, it is important to design the test articles to ensure that predicted epitopes or regions of interest are properly centered and that the test article peptide length is within an optimal range for HLA binding. Without in silico prediction and therefore without guided test article design, the HLA binding assay may not yield results that are indicative of the peptide’s true in vivo HLA binding properties. In addition, in silico immunogenicity assessments indicate whether the modified amino acid occurs in an HLA-binding (1, 4, 6, and 9) or in a TCR-facing (2, 3, 5, 7, and 8) residue of a predicted HLA ligand. In immunogenicity risk assessments for peptide-related impurities this is a particularly important distinction. HLA-binding studies may be the in vitro assay of choice to assess the impact of the modified residue in an HLA-binding position of a predicted epitope occurring within the baseline API sequence. Importantly, an ex vivo T cell assay would be more appropriate to test the immunological impact of a modified residue occurring in a TCR-facing position of a predicted epitope within the baseline API sequence.
Although this 3-step method opens the door to enabling in silico immunogenicity analysis for sequences containing UAA with existing in silico prediction tools, there are some limitations. Particularly, this method relies on the predicted impact that the surrounding amino acids will have on HLA binding potential. In other words, this method is applicable to sequences that contain only one UAA within a 9-mer span. Peptide sequences containing more than one UAA within a 9-mer span are not eligible for confident in silico immunogenicity analyses using current in silico prediction algorithms. In addition, this method considers each amino acid within a sequence as a unique entity in isolation. In other words, it assumes a static backbone and evaluates the impact of modified side chains at a single position. In reality, some UAA may have an impact on the orientation of other amino acid side chains within the peptide. For example, a peptide with a modification in an HLA-binding position may cause steric changes that alter the amino acids that are ‘seen’ by the T cell receptor, or vice versa. This is a possible explanation for the reported decrease in HLA binding affinity for peptides with a D-amino acid incorporated into a TCR-facing position relative to the L-amino acid version (Azam et al., 2021).
4.2 Next–The development of correction factors to apply to common unnatural amino acids
Most common UAA are mutated versions of naturally occurring amino acids. In the next phase of the program, the authors plan to establish “correction factors” that can be used to account for the presence of UAA in candidate peptides and proteins. As discussed above, EpiMatrix relies on our in silico predictive algorithms rely on allele-specific matrices of binding affinity coefficients. For each HLA allele and naturally occurring amino acid, a vector of 9 binding coefficients (one for each possible binding position) has been established. By synthesizing and testing known HLA ligands and experimental counterparts containing a single UAA at one or more selected binding positions, the authors will establish the magnitude of impact that a given mutation can have on HLA binding. The resulting “correction factor” can then be used to establish a first generation of UAA-specific binding coefficients.
The development of correction factors for common unnatural amino acids will provide a longer-term solution to some of the limitations of the currently available in silico immunogenicity prediction tools and methods. The first iteration of correction factors for common UAA are based upon a comparison of the UAA side chain structure to the closest matching natural amino acid and the application of minimal, moderate, or significant correction factor deductions. These estimations will then be confirmed in vitro with carefully designed HLA binding studies based on known ligands which have been modified to contain UAA in HLA anchoring positions. The binding affinity data can then be used to “correct” binding affinity coefficients derived from the naturally-occurring counterparts of UAA. Finally, the estimations will be further confirmed ex vivo with T cell assays that assess the impact selected UAA will have on T cell recognition and immunogenic potentials.
The first phase of this effort focuses on generating preliminary data to ultimately develop correction factors for D-amino acids. D-amino acids are commonly encountered in synthetic peptide impurities (D'Hondt et al., 2014) or by design in novel peptide drug development to decrease the rate of proteolytic cleavage and therefore increase the half-life of the peptide drug candidate (Evans et al., 2020; Wang et al., 2022). Reported studies have demonstrated that D-amino acids incorporated into some positions in the core sequence of a known promiscuous HLA-DR binding peptide, Flu-HA (306–318), diminish HLA binding affinity compared to the L-amino acid counterpart (Azam et al., 2021). This suggests that substituting the L-isomer for in silico immunogenicity analysis of D-amino acid containing sequences likely yields an overestimate in HLA binding potential. In addition, steric changes due to D-amino acids have been shown to change the conformation of amino acid residues seen by the T cell receptor (TCR), lowering the T cell response (Azam et al., 2021). Thus, in addition to HLA binding, D-amino acids could modify recognition of the peptide by a CD4+ T cell. More position- and allele-specific precision is needed.
Here, a brief example of the ongoing in vitro confirmation studies used to generate correction factors for D-amino acids is provided. Starting with a known promiscuous HLA-DR binding peptide backbone, PADRE, modifications with a set of D-amino acid vs L-amino acids substitutions at HLA-binding positions 1, 4, 6, and 9 were designed, produced, and are being tested in HLA binding assays. Each HLA binding assay generates a direct estimate of the impact of L-to D-modification on a specific allele, binding pocket and backbone allowing us to model impacts of D-amino acids on HLA binding relative to L-isomers. Preliminary data are shared for binding pocket one in Figure 13. The HLA binding results of this preliminary study indicate that D-amino acids substituted in position one significantly disrupt HLA binding. Compared to L-amino acids, most D-amino acids in P1 are not compatible with HLA-DR binding. Eighty percent of the peptides with L-amino acids in position one bound, while only 18% of their D-amino acids counterparts bound (Figure 13).
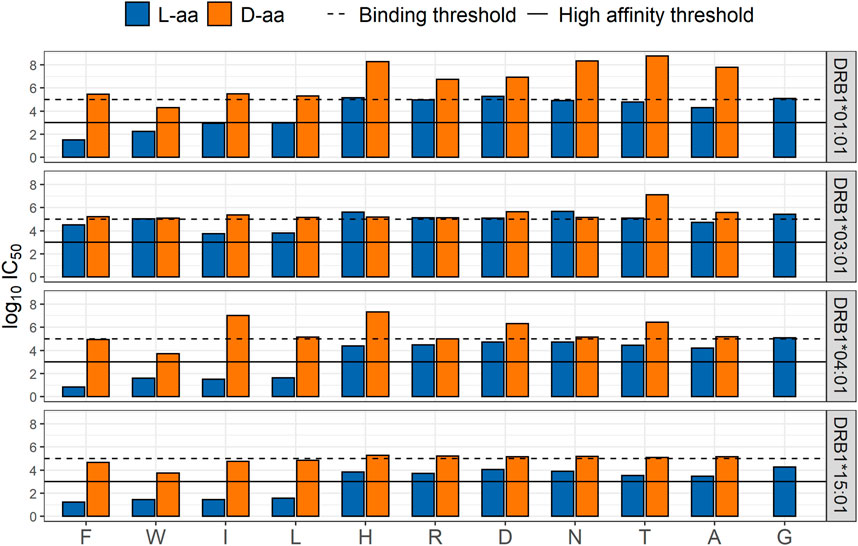
FIGURE 13. Comparison of HLA-DRB1 binding affinities in position one between L- and D-amino acids. Log10IC50 HLA binding affinity based on a competition-based assay for L- (blue) and D- (orange) amino acids in position one; substitutions are shown on the X-axis.
The impact of D-amino acids in the remaining HLA-binding positions is currently being evaluated and the impact of D-amino acids in TCR-facing positions will also be assessed in future studies. Because the peptide backbone may also influence HLA binding, the impact of D-amino acid modifications in different baseline peptides, including generic peptides, should also be evaluated. Finally, due to the potential that steric changes caused by substitution with a D-amino acid can impact the T cell response, future studies will also include the use of an in vitro Immunization Protocol (IVIP) T cell assay, to further assess the impact of D-amino acids on the T cell response. It is possible that D-amino acids in other positions may exhibit similar binding propensities as the L-version yet result in a decrease or increase in the T cell responsiveness.
Collectively, these studies will allow us to develop correction factors (e.g., a D-lysine substituted for L-lysine in pocket one reduces HLA binding by 50% for HLA-DRB1*0101). These data can then be used to adjust the predicted immunogenic risk of peptides (API or impurities), containing D-amino acids, that are found in peptide drug products. The correction factors will enable currently available in silico prediction tools (EpiMatrix and other public tools) to adjust for differences between the HLA binding potential of peptides with natural L-amino acids and those with D-amino acids. Once a set of correction factors has been established for D-amino acids, the same approach can be taken to develop correction factors for other common UAA.
4.3 Future–direct in silico immunogenicity prediction for common unnatural amino acids
In the future, more UAA-specific training data will become available. With these data in hand, improved predictive algorithms capable of directly estimating the HLA binding affinity of UAA can be developed, allowing for the direct in silico immunogenicity assessment of UAA-containing peptide and protein therapeutics. However, given the vast amount of data required to train the algorithms, this is likely only a realistic vision for the most common UAA. Novel peptide drug discovery involves the potential incorporation of hundreds to thousands of different UAA into investigational lead candidates. With common UAA directly incorporated into in silico immunogenicity prediction algorithms, peptide sequences containing the more obscure UAA can be assessed using the 3-step method and through the application of correction factors.
4.4 Other factors can influence immunogenic risk
Finally, the authors recognize that although the method and discussion in this paper focus solely on a peptide’s HLA binding properties, there are many other factors contributing to immunogenicity, including but not limited to whether the product is human-derived or foreign, the product’s propensity for aggregation, purity, stability, mechanism of action, the patient’s HLA and disease state, the route of delivery, dose and frequency, immunomodulatory properties of the product, etc.
Another important attribute to in silico risk assessment is the characterization of predicted epitope content as T effector or T regulatory based on its level of cross-conservation with epitopes in the human proteome. The immunological response to seemingly similar peptides in terms of HLA-binding properties can be vastly different depending on whether the epitope is likely to engage effector T cells or regulatory T cells. For instance, in addition to being derived from a human protein, the putative promiscuous T cell epitope found in teriparatide is highly cross-conserved with epitopes found in other prevalent human proteins, indicating that this promiscuous HLA binding region is likely to be tolerated by the human immune system, if not actively tolerogenic (Jawa et al., 2020). However, UAA-containing impurities may engage an entirely different cohort of T cells. It is especially important to consider the immunogenic impact of peptide impurities or modifications occurring within these highly human-like putative epitopes. Another factor to consider when assessing the immunogenic risk of a peptide containing UAA(s) is the impact the UAA is having on peptide processing. For instance, many times UAA are introduced to a natural peptide sequence to provide resistance to proteolytic cleavage. If the inclusion of UAA into the sequence changes the patterns in proteolytic cleavage then one can anticipate that the peptide’s ability to be processed and presented by the antigen presenting cells could be altered leading to a potential difference in the epitope presentation patterns relative to the natural sequence.
In addition, future studies should include exploration into the impacts of unnatural amino acids on peptide binding to HLA Class I molecules and potential for CD8+ T cell response.
In vivo studies would be helpful to prospectively validate the immunogenicity findings described here. However, preclinical models for immunogenicity research are limited by three factors 1) the native peptides that are the focus of drug development may have slightly different sequences that could impact immune responses to the API, 2) The MHC molecules that are engaged in the immune responses may have different MHC-binding motif preferences (different side chains bind to the binding pockets), and 3) cross-conservation of the peptide with other peptide epitopes in the genome of the in vivo model system being used may be different enough to affect the tolerogenicity profile of the drug. These types of issues have been encountered with peptide drugs that do not contain unnatural amino acids.
In conclusion, we offer a method to estimate immunogenic risk for peptides containing UAA residues using the existing infrastructure of in silico algorithms and provide our vision for a tiered approach to the eventual inclusion of common UAA into in silico immunogenicity prediction algorithms.
Data availability statement
The original contributions presented in the study are included in the article, further inquiries can be directed to the corresponding author.
Author contributions
AM wrote the first draft of the manuscript and created figures. WM is responsible for the original conception of both the 3-step in silico method and the correction factor studies. AM contributed to the conception and design of 3-Step in silico method. AG contributed to the design and performed the statistical analysis and created Figure 13 for the D-amino acid correction factor studies. FT provided valuable guidance. BR, AR, and AD provided immunology expertise. All authors made substantial edits, reviewed, and approved the manuscript for submission.
Acknowledgments
Authors are grateful to Mitchell McAllister for careful review, and to Genna De Groot for artwork in Figure 1. We are also grateful to the EpiVax laboratory team for contributing to the in vitro HLA binding assays.
Conflict of interest
AD and WM are senior officer and shareholders, and AM, AG, BR, FT, and AR are employees of EpiVax, Inc., a privately owned biotechnology company located in Providence, RI.
Publisher’s note
All claims expressed in this article are solely those of the authors and do not necessarily represent those of their affiliated organizations, or those of the publisher, the editors and the reviewers. Any product that may be evaluated in this article, or claim that may be made by its manufacturer, is not guaranteed or endorsed by the publisher.
Author Disclaimer
These authors acknowledge that there is a potential conflict of interest related to their relationship with EpiVax and attest that the work contained in this research report is free of any bias that might be associated with the commercial goals of the company.
References
Alexander, J., Sidney, J., Southwood, S., Ruppert, J., Oseroff, C., Maewal, A., et al. (1994). Development of high potency universal DR-restricted helper epitopes by modification of high affinity DR-blocking peptides. Immunity 1 (9), 751–761. doi:10.1016/s1074-7613(94)80017-0
Amatruda, T. T., Bohman, R., Ranyard, J., and Koeffler, H. P. (1987). Pattern of expression of HLA-DR and HLA-DQ antigens and mRNA in myeloid differentiation. Blood 69 (4), 1225–1236.
Azam, A., Mallart, S., Illiano, S., Duclos, O., Prades, C., and Maillère, B. (2021). Introduction of non-natural amino acids into T-cell epitopes to mitigate peptide-specific T-cell responses. Front. Immunol. 12, 637963. doi:10.3389/fimmu.2021.637963
D'Hondt, M., Bracke, N., Taevernier, L., Gevaert, B., Verbeke, F., Wynendaele, E., et al. (2014). Related impurities in peptide medicines. J. Pharm. Biomed. Anal. 101, 2–30. doi:10.1016/j.jpba.2014.06.012
De Groot, A. S., Jesdale, B., Martin, W., Saint Aubin, C., Sbai, H., Bosma, A., et al. (2003). Mapping cross-clade HIV-1 vaccine epitopes using a bioinformatics approach. Vaccine 21 (27-30), 4486–4504. doi:10.1016/s0264-410x(03)00390-6
De Groot, A. S., and Martin, W. (2009). Reducing risk, improving outcomes: Bioengineering less immunogenic protein therapeutics. Clin. Immunol. 131 (2), 189. doi:10.1016/j.clim.2009.01.009
Deacon, C. F., Nauck, M. A., Toft-Nielsen, M., Prida, L., Willms, B., and Holst, J. J. (1995). Both subcutaneously and intravenously administered glucagon-like peptide I are rapidly degraded from the NH2-terminus in type II diabetic patients and in healthy subjects. Diabetes 44, 1126–1131. doi:10.2337/diab.44.9.1126
Di, L. (2015). Strategic approaches to optimizing peptide ADME properties. AAPS J. 17 (1), 134–143. doi:10.1208/s12248-014-9687-3
Evans, B. J., King, A. T., Katsifis, A., Matesic, L., and Jamie, J. F. (2020). Methods to enhance the metabolic stability of peptide-based PET radiopharmaceuticals. Molecules 25 (10), 2314. doi:10.3390/molecules25102314
FDA - CDER (2021). Center for Biologics Evaluation Research C for DER. ANDAs for Certain Highly Purified Synthetic Peptide Drug Products That Refer to Listed Drugs of rDNA Origin. Silver Springs, MD: US Food and Drug Administration. https://www.fda.gov/regulatory-information/search-fda-guidance-documents/andas-certain-highly-purified-synthetic-peptide-drug-products-refer-listed-drugs-rdna-origin.
Fosgerau, K., and Hoffmann, T. (2015). Peptide therapeutics: Current status and future directions. Drug Discov. Today 20 (1), 122–128. doi:10.1016/j.drudis.2014.10.003
Grassi, L., and Cabrele, C. (2019). Susceptibility of protein therapeutics to spontaneous chemical modifications by oxidation, cyclization, and elimination reactions. Amino Acids 51, 1409–1431. doi:10.1007/s00726-019-02787-2
Hyun, Y. S., Jo, H. A., Lee, Y. H., Kim, S. M., Baek, I. C., Sohn, H. J., et al. (2021). Comprehensive analysis of CD4+ T cell responses to CMV pp65 antigen restricted by single HLA-DR, -DQ, and -DP allotype within an individual. Front. Immunol. 11, v11. doi:10.3389/fimmu.2020.602014
Robinson, J., Barker, D. J., Georgiou, X., Cooper, M. A., Flicek, P., and Marsh, S. G. E. (2020). IPD-IMGT/HLA Database. Nucleic Acids Res. 48 (D1), D948–D955. doi:10.1093/nar/gkz950
Knudsen, L. B., Nielsen, P. F., Huusfeldt, P. O., Johansen, N. L., Madsen, K., Pedersen, F. Z., et al. (2000). Potent derivatives of glucagon-like peptide-1 with pharmacokinetic properties suitable for once daily administration. J. Med. Chem. 43, 1664–1669. doi:10.1021/jm9909645
Lecchi, M., Lovisone, E., Genetta, C., Peruccio, D., Resegotti, L., and Richiardi, P. (1989). Gamma-IFN induces a differential expression of HLA-DR, DQ and DP antigens on peripheral blood myeloid leukemic blasts at various stages of differentiation. Leuk. Res. 13 (3), 221–226. doi:10.1016/0145-2126(89)90015-5
Lee, A. C., Harris, J. L., Khanna, K. K., and Hong, J. H. (2019). A comprehensive review on current advances in peptide drug development and design. Int. J. Mol. Sci. 20 (10), 2383. doi:10.3390/ijms20102383
Lund, O., Nielsen, M., Kesmir, C., Petersen, A. G., Lundegaard, C., Worning, P., et al. (2004). Definition of supertypes for HLA molecules using clustering of specificity matrices. Immunogenetics 55 (12), 797–810. doi:10.1007/s00251-004-0647-4
Jawa, V., Terry, F., Gokemeijer, J., Mitra-Kaushik, S., Roberts, B. J., Tourdot, S., et al. (2020). T-Cell Dependent Immunogenicity of Protein Therapeutics Pre-clinical Assessment and Mitigation-Updated Consensus and Review 2020. Front Immunol. 11, 1301. doi:10.3389/fimmu.2020.01301
Ozempic (2022). Ozempic (semaglutide) package insert. Kalundborg, Denmark: Novo Nordisk U.S. Food and Drug Administration. https://www.accessdata.fda.gov/drugsatfda_docs/label/2017/209637lbl.pdf. Revised Dec 2017. Accessed May 2022.
Ratanji, K. D., Derrick, J. P., Dearman, R. J., and Kimber, I. (2014). Immunogenicity of therapeutic proteins: Influence of aggregation. J. Immunotoxicol. 11, 99–109. doi:10.3109/1547691X.2013.821564
Rosenstock, J., Balas, B., Charbonnel, B., Bolli, G. B., Boldrin, M., Ratner, R., et al. (2013). The fate of taspoglutide, a weekly GLP-1 receptor agonist, versus twice-daily exenatide for type 2 diabetes: The T-emerge 2 trial. Diabetes Care 36, 498–504. doi:10.2337/dc12-0709
Rudolph, M. G., Stanfield, R. L., and Wilson, I. A. (2006). How TCRs bind MHCs, peptides, and coreceptors. Annu. Rev. Immunol. 24, 419–466. doi:10.1146/annurev.immunol.23.021704.115658
Rybelsus (2022). Rybelsus (semaglutide) package insert. Bagsvaerd, Denmark: Novo Nordisk U.S. Food and Drug Administration. https://www.accessdata.fda.gov/drugsatfda_docs/label/2019/213051s000lbl.pdf. Revised Sept 2019. Accessed May 2022.
Steere, A. C., Klitz, W., Drouin, E. E., Falk, B. A., Kwok, W. W., Nepom, G. T., et al. (2006). Antibiotic-refractory Lyme arthritis is associated with HLA-DR molecules that bind a Borrelia burgdorferi peptide. J. Exp. Med. 203 (4), 961–971. doi:10.1084/jem.20052471
Singh, S. K. (2010). Impact of product-related factors on immunogenicity of biotherapeutics. J. Pharm. Sci. 100, 354–387. doi:10.1002/jps.22276
Southwood, S., Sidney, J., Kondo, A., AppElla, E., Hoffman, S., Kubo, R. T., et al. (1998). Several common HLA-DR types share largely overlapping peptide binding repertoires. J. Immunol. 160 (7), 3363–3373.
Stern, L. J., Brown, J. H., Jardetzky, T. S., Gorga, J. C., Urban, R. G., Strominger, J. L., et al. (1994). Crystal structure of the human class II MHC protein HLA-DR1 complexed with an influenza virus peptide. Nature 368 (6468), 215–221. doi:10.1038/368215a0
Tan, H., Su, W., Zhang, W., Zhang, J., Sattler, M., and Zou, P. (2021). Albumin-binding domain extends half-life of glucagon-like peptide-1. Eur. J. Pharmacol. 890, 173650. doi:10.1016/j.ejphar.2020.173650
Terry, F. E., Moise, L., Martin, R. F., Torres, M., Pilotte, N., Williams, S. A., et al. (2015). Time for T? Immunoinformatics addresses vaccine design for neglected tropical and emerging infectious diseases. Expert Rev. Vaccines 14, 21–35. doi:10.1586/14760584.2015.955478
Victoza (2022). Victoza (liraglutide) package insert. Bagsvaerd, Denmark: Novo Nordisk. United States Food and Drug Administration. https://www.accessdata.fda.gov/drugsatfda_docs/label/2017/022341s027lbl.pdf. Revised Aug 2017. Accessed May 2022.
Vita, R., Mahajan, S., Overton, J. A., Dhanda, S. K., Martini, S., Cantrell, J. R., et al. (2018). The immune epitope Database (IEDB): 2018 update. Nucleic Acids Res. 47, D339–D343. doi:10.1093/nar/gky1006
Keywords: peptide drug, immunogenicity, immunoinformatic analysis, T cell epitope, HLA binding, D-amino acid, unnatural amino acid (UAA), impurity
Citation: Mattei AE, Gutierrez AH, Martin WD, Terry FE, Roberts BJ, Rosenberg AS and De Groot AS (2022) In silico immunogenicity assessment for sequences containing unnatural amino acids: A method using existing in silico algorithm infrastructure and a vision for future enhancements. Front. Drug. Discov. 2:952326. doi: 10.3389/fddsv.2022.952326
Received: 25 May 2022; Accepted: 21 September 2022;
Published: 10 October 2022.
Edited by:
José L. Medina-Franco, National Autonomous University of Mexico, MexicoReviewed by:
Marcin Poreba, Wrocław University of Science and Technology, PolanQingsheng Li, University of Nebraska-Lincoln, United States
Copyright © 2022 Mattei, Gutierrez, Martin, Terry, Roberts, Rosenberg and De Groot. This is an open-access article distributed under the terms of the Creative Commons Attribution License (CC BY). The use, distribution or reproduction in other forums is permitted, provided the original author(s) and the copyright owner(s) are credited and that the original publication in this journal is cited, in accordance with accepted academic practice. No use, distribution or reproduction is permitted which does not comply with these terms.
*Correspondence: Aimee E. Mattei, amattei@epivax.com