- 1Department of Endocrinology, The Third Affiliated Hospital of Zunyi Medical University, Zunyi City, Guizhou Province, China
- 2Bioresource Institute for Healthy Utilization, Zunyi Medical University, Zunyi City, Guizhou Province, China
- 3Nursing Department, Affiliated Hospital of Guizhou Medical University, Guiyang City, Guizhou Province, China
Although Siraitia grosvenorii (abbreviated as S.g.) is frequently used to prevent and cure diabetes problems, the precise mechanism underlying its ability to do so remains unknown. Through network pharmacology and molecular docking techniques, we studied the early molecular mechanisms of S.g in the treating of proliferative diabetic retinopathy (PDR) in this study. The Traditional Chinese Medicine Systems Pharmacology (TCMSP) database was used to screen the active compounds and related targets of S.g. Oral bioavailability (OB) 30% and drug likeness (DL) 0.18 were used as screening criteria. The active compounds without knowledge of a probable target were excluded. The Uniprot database included converted symbols for the associated targets. GEO2R was used to explore several genes related to PDR. Using jvenn web service to intersect targets of S.g and PDR. The Xiantao Academic Online website was used to examine the expression patterns of intersect targets in PDR samples. The STRING database was used to create a protein-protein interaction (PPI) network of intersecting targets. Cytoscape software was used to show the PPI network, MCODE software was used to evaluate the network’s core proteins, and CytoHubba software was used to extract the important networks of the top three targets. Omicshare platform carried a functional analysis using the Gene Ontology (GO) and pathway enrichment analysis using the Kyoto Encyclopedia of Genes and Genomes (KEGG). Pymol, AutoDock Vina software, Schrödinger Software were used to conduct molecular docking experiments or pockets search on the top three targets. The results showed that 85 targets were matched to six active compounds of S.g. 18 intersect targets were found. Seven DEGs were up-regulated and eleven genes were down-regulated when these targets were divided into two groups. TNF, PTGS2, and CASP3 were the main targets, according to the PPI network. The intersect targets were mostly related to angiogenesis, cell proliferation, oxidative stress, inflammatory response, and metabolism. It was discovered that the core targets TNF, PTGS2, and CASP3 had various levels of affinity for their respective compounds. Interestingly, multiple good drug-forming pockets for CASP3 and PTGS2 targets were identified through Schrödinger software. In particular, six compounds bind to the top three core targets to inhibit IL-17 signaling pathway, AGE-RAGE signaling pathway in diabetic complications, Pathways in cancer and 14 other signaling pathways to inhibit inflammation, apoptosis, oxidative stress, arachidonic acid metabolism, and angiogenesis to prevent and treat PDR. The study’s findings, which served as a guide for the widespread use of S.g in PDR clinical practise, included multi-substances and targets of S.g to prevent and cure PDR.
Introduction
Neovascularization or preretinal/vitreous bleeding are characteristics of proliferative diabetic retinopathy, or PDR, For all abbreviations involved in this document, please refer to Supplementary Table S1. In individuals with diabetes, hypoxia, oxidation, inflammation, neurodegeneration, and leukocyte suppression are the primary contributors to PDR pathogenesis. The diabetic retina is subject to pathological effects of the PDR vitreous, which promote further disease development (Nawaz et al., 2019). Although there have been significant advancements in the treatment of PDR (Tan et al., 2021), the prospective medications created in response to the intricate pathogenic mechanism of PDR are far from sufficient, especially given the absence of traditional Chinese medicine (TCM).
The therapeutic benefits of TCM on retinopathy have steadily come to light in recent years (Song and Zhu, 2019; Liu et al., 2022). S. g is a medicinal and edible related plant with anti-inflammatory, antioxidant, and blood sugar-lowering properties (Liu et al., 2018). Mogrosides, kaempferol, quercetin, succinic acid, vanillic acid, triterpenoid saponins, and other substances are among the primary components of S.g (Gong et al., 2019). For the therapy of diabetic retinopathy, S.g has been mentioned (Xu and Lin, 2009). PDR is a kind of diabetic retinopathy. This suggests that S.g may be a useful TCM for PDR prevention and therapy. How S.g could prevent and cure PDR is still a mystery.
TCM offers certain benefits in the prevention and specialised therapy of PDR due to the multi-target and multi-channel intervention techniques it uses, as well as its overall management and synergy. Therefore, Combined use of computer information technology and TCM will play a significant role in elucidating the mechanism of prevention and therapy of some diseases.
Chemoinformatics, bioinformatics, network biology, and pharmacology are all useful tools of network pharmacology for understanding how drugs operate (Chen et al., 2018; Zhang et al., 2019a). TCM has gathered numerous herbal formulations and has traditionally seen people or patients as systems with various states. The fundamental principles of network pharmacology and network biology, which are developing fields, are quite similar to the holistic philosophy of TCM. This philosophical theoretical framework may be used to meet the needs of treating difficult diseases like cancer. The research paradigm was changed from the present ‘one target, one drug’ approach to a ‘network target, multicomponent’ model in order to identify TCM from a systematic and molecular level (Li and Zhang, 2013). Network pharmacology assigns a higher priority to disease-related targets matching to the constituents of TCM. In addition, it explains combinatorial rules and network regulatory effects of TCM recipe while revealing drug-gene-disease co-modular linkages and screening synergistic multi-compounds from TCM formulations in a high-throughput manner. This approach has been validated (Li and Zhang, 2013) and illuminates the mechanisms of action of various TCM. A molecular level strategy for defining structure-activity relationships (SAR) or predicting ligand-target interactions is known as molecular docking. The identification of new compounds with therapeutic potential and widespread application in drug development is made possible by computational results from this approach (Pinzi and Rastelli, 2019). In this study, we explored the primary bioactive components of S.g and predicted its potential mechanism for preventing and therapy PDR using network pharmacology technique combined with GEO chip data and Molecular docking.
Materials and methods
Screening of active compounds of S.g
The TCMSP pharmacology database (TCMSP, http://tcmspw.com/tcmsp.php) was used to locate the active compounds of S. g Since traditional Chinese medicine requires the appropriate organs and tissues of the human body to go through the process of absorption, distribution, metabolism, and excretion (ADME) following oral administration. Retrieving pharmacokinetic data was utilised to screen active compounds. Oral bioavailability (OB) 30% and drug likeness (DL) 0.18 were the set criteria. Without knowing their possible targets, the active compounds were eliminated.
Construction of active compounds-target gene network of S.g
The TCMSP database was used to find the active compounds and the relevant targets. Following that, targets were performed using the UniProt database (https://www.uniprot.org) as a standard symbol. The species was set to ‟human” in the following parameter. The network of active compounds and S.g. targets visualisation was created using the Cytoscape 3.8.0 programme.
Analysis of DEGs of PDR
To analyse PDR’s differentially expressed genes (DEGs), Gene Expression Omnibus (GEO) data (GSE60436) was used. The data consists of 6 samples (NO. GSM1479907 to GSM1479912) in the experimental group and 3 samples in the control group (NO. GSM1479904 to GSM1479906). The GEO2R tool (https://www.ncbi.nlm.nih.gov/geo/geo2r/?acc=GSE60436) was used to examine the DEGs in this dataset. The following parameters are set: logFC>1 or −1 and a p-value of 0.05. Duplicates and entries lacking Gene symbol information were eliminated.
Determination of PDR-related targets of the active components
The Venn diagram network tool venny 2.1.0 (https://bioinfogp.cnb.csic.es/tools/venny/index.html) was used to import the chosen active compounds and PDR-related protein targets for analysis. The active compounds of S.g for the prevention and treatment of PDR were shown to have a intersecting target.
Differential expression analysis of active compounds targets in PDR samples
The distribution of targets in PDR was shown using the volcano plot analysis tool from Xiantao Academic. The intricate procedure involved using the DEGs discovered by GEO2R analysis as background genes and explicitly marking targets to track their up- or down-regulation. The following parameters are set: logFC>1 or −1 and a p-value of 0.05. At the same time, the expression patterns of targets in each sample were examined using the heat map online tool in Xiantao Academic (https://www.xiantao.love/products).
Construction protein and protein interaction network of targets
Using STRING 11.5 (https://cn.string-db.org/), interactions between target proteins were investigated. Inputting the target protein into the string database was the particular operation. The settings were set to “Homo sapiens” “0.4” for the confidence cutoff value, and “more” to link as many targets as feasible into the network. The output of the findings is in the form of “short tabular text output”. After that, the outcomes were examined using Cytoscape 3.8.0 software. The MCODE plugin was used to investigate the core proteins of the network. The top three important targets of the protein and protein interaction network were extracted using the plug-in CytoHubba.
GO and KEGG enrichment analysis of targets
The OmicShare platform has a thorough annotation feature (https://www.omicshare.com/tools/Home/Soft). The Kyoto Encyclopedia of Genes and Genomes (KEGG) pathway and Gene Ontology (GO) of targets were studied using the Omicshare platform. These objectives have values for Log2FC. All parameters were set as follows: “background gene files” was set to “model species,” “version/species/type” was set to “Ensemble 104 or 51”, “Homo sapiens (GRCh38. p13)”, and “gene” was set to “gene symbol”, p value less than 0.05 was set for plotting.
Molecular docking and molecular mechanism speculation involved in the core target
The top three core targets’ three-dimensional (3D) structures were downloaded from the RCSB PDB database at https://www.rcsb.org/. Through the TCMSP database, the active compounds matching to the 3−position core protein in MOL2 format were obtained. After removing the water molecules, isolating the proteins, adding the nonpolar hydrogen, and calculating Gasteiger charges for the structure, AutoDock Tools 1.5.7 software was used to save the structure as a PDBQT file. The active compounds’ MOL2 format file was saved as a PDBQT docking ligand in the AutoDock Tools 1.5.7 programme. The active compounds were employed as ligands and the receptors used were TNF,CASP3, and PEGS2. The ligand coordinate in the target protein complex determined the molecular docking’s active site. The receptor was designed to be stiff, whereas the ligand was flexible. The final docking conformation was chosen based on affinities, and Pymol 2.5.2 was used to visualise it. In order to solve the problem that these molecules may also be allosteric regulators, they may not bind to active sites, but bind to other sites. Schrodinger software is used to find all possible pockets on the protein surface and connect interested molecules to each pocket to find the best docking position in silico. Combining the results of two forms of molecular docking and the reported literature, the molecular mechanism of the core target participating in the prevention and treatment of PDR was predicted.
Results
Active compounds of S.g
After screening, six active compounds were obtained from the TCMSP database. The six compounds were Mandenol, ZINC03860434, Perlolyrine, beta-sitosterol, kaempferol, and Flazin (Table 1). Mandenol is a colorless to pale yellow oily liquid. Boiling point 212 °C (1.6 kPa), 175°C (0.33 kPa), 133°C (0.133 kPa), relative density 0.8865, refractive index 1.4675. Insoluble in water, soluble in ethanol and ether, miscible with aliphatic solvents and oils. This ingredient can be used to inhibit inflammation. ZINC03860434 is a light yellow oily liquid. Freezing point −55°C, boiling point 231°C (0.67 kPa), relative density 0.9861 (25/4°C), refractive index 1.483 (25°C). It is miscible with most organic solvents and insoluble in water. ZINC03860434 is a solid, the density is 1.4 ± 0.1 g/cm3, the boiling point is 533.9 ± 45.0°C at 760 mmHg, the molecular weight is 264.279, and the refractive index is 1.749. Beta-Sitosterol is a white scaly, needle-like crystal or crystalline powder. Odorless and tasteless. Mp136-140°C. It is easily soluble in chloroform and carbon disulfide, slightly soluble in ethanol or acetone, and insoluble in water. Kaempferol is a yellow crystalline powder, melting point 276°C, boiling point 348.61°C, density 1.2981, refractive index 1.4413, storage condition 2–8°C, solubility in ethanol: 20 mg/ml kaempferol has antioxidant, anti-inflammatory, anti-cancer, and anti-diabetic effects. Flazin is a solid powder with a density of 1.513 g/cm3, a boiling point of 657.1°C at 760 mmHg, a molecular formula of C17H12N2O4, a molecular weight of 308.28, a flash point of 351.2°C, and a refractive index of 1.771. Flazin can be used for researching diabetes and neuronal disorders.
Construction of ‘active compounds-targets’ network of S.g
In the TCMSP database, 85 potential targets for six S.g active compounds were found (Supplementary Table S2). Then, using the CytoScape 3.6.0 software, a “active compounds-targets” interaction network was created (Figure 1). The results showed that the components Flazin and Mandenol had two targets each, Perlolyrine had three targets, ZINC03860434 had four targets, kaempferol had 55 targets and beta-sitosterol had 35 targets. Kaempferol and beta-sitosterol clearly have more targets than other active compounds. Kaempferol and beta-sitosterol were therefore the main active compounds in S.g.
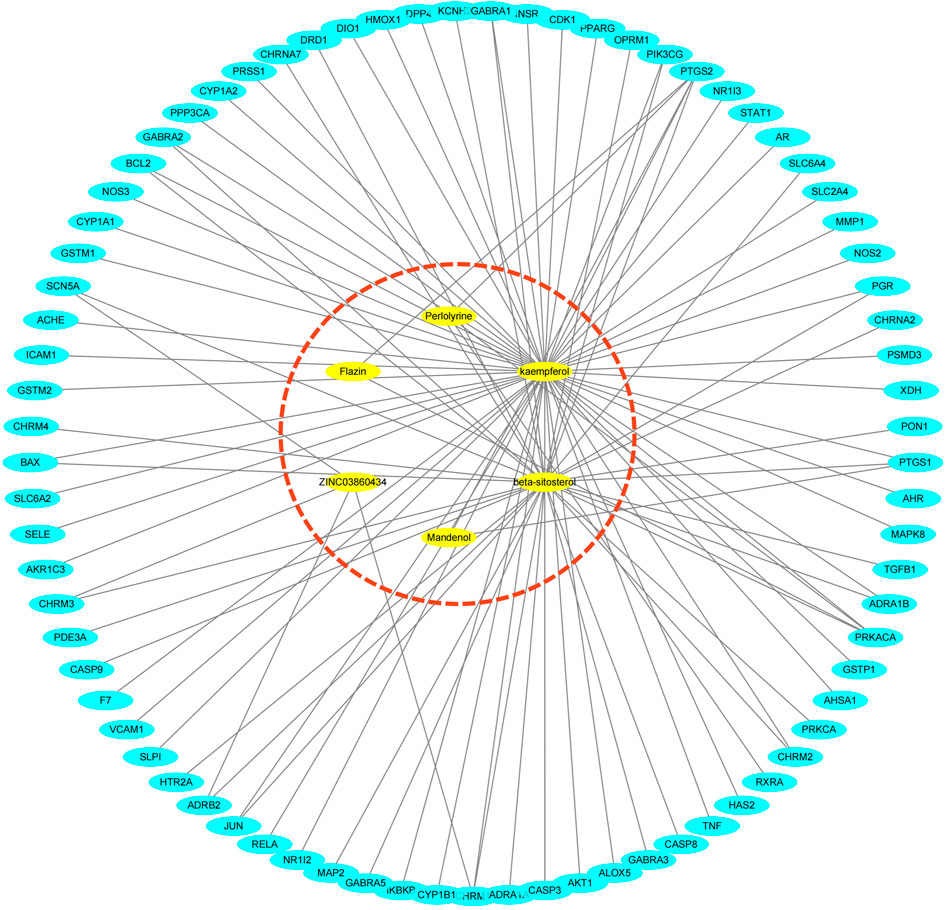
FIGURE 1. “Active compounds—targets” network, six active compounds are marked in red dashed circles.
Analysis of differential genes of PDR
After screening, 3055 DEGs were obtained. Then, 2642 DEGs were finally obtained after deduplication (Supplementary Table S3).
Determination of PDR-related targets of the active components
Following investigation, it was discovered that 18 intersect targets, including HMOX1, STAT1, PIK3CG, TNF, CASP3, PTGS1, NOS3, CDK1, PTGS2, ADRA1B, MMP1, ADRB2, GSTM2, ACHE, KCNH2, GSTM1, CYP1B1, and CHRM3, shared 85 targets of S.g and 2642 PDR’s DEGs (Figure 2). Further analysis will be conducted using these shared objectives.
Differential expression analysis of target genes
Using volcano plots, we further illustrated the distribution of these target genes’ up- or down-regulated expression over the complete expression profile of GES60436. The findings revealed that seven Targets—namely, CHRM3, KCNH2, ACHE, GSTM1, GSTM2, and CYP1B1—were down-regulated. There was an up-regulation of 11 Targets, including, successively, PIK3CG, CASP3, STAT1, TNF, NOS3, HMOX1, CDK1, PTGS1, ADRA1B, MMP1, and PTGS2 (Figure 3A). When we simultaneously conducted cluster analysis on the expression levels of these target genes in each GES60436 sample, we discovered that the 18 intersecting genes’ expression profiles were split into two groups, the first of which contained genes that were up-regulated. ACHE, KCNH2, and CHRM3 were grouped into one branch, while KCNH2 and CHRM3 were clustered into a smaller branch under this branch. GSTM2 and GSTM1 were clustered into one branch, ADRB2 and CYP1B1 were clustered into another branch, and ACHE, KCNH2, and GSTM1 were clustered into one branch. The genes in the second group were those that were up-regulated. There were two branches created within this gene group as well. The first branch included CDK1, NOS3, PTGS2, and PTGS1. Among them, TNF and CDK1 aggregated into a small branch and subsequently aggregated with PTGS2 into a branch. PTGS1 and NOS3 were grouped into a tiny branch underneath the branch. ADRA1B, CASP3, STAT1, PIR3CG, HMOX1, and MMP1 made up another branch of this type of clustering, of which MMP1 and HMOX1 aggregated into one branch, PIR3CG and STAT1 into one branch, CASP3 into one branch, and finally the aforementioned two branches into one branch. It aggregates with ADRA1B to create a branch after first creating one (Figure 3B).
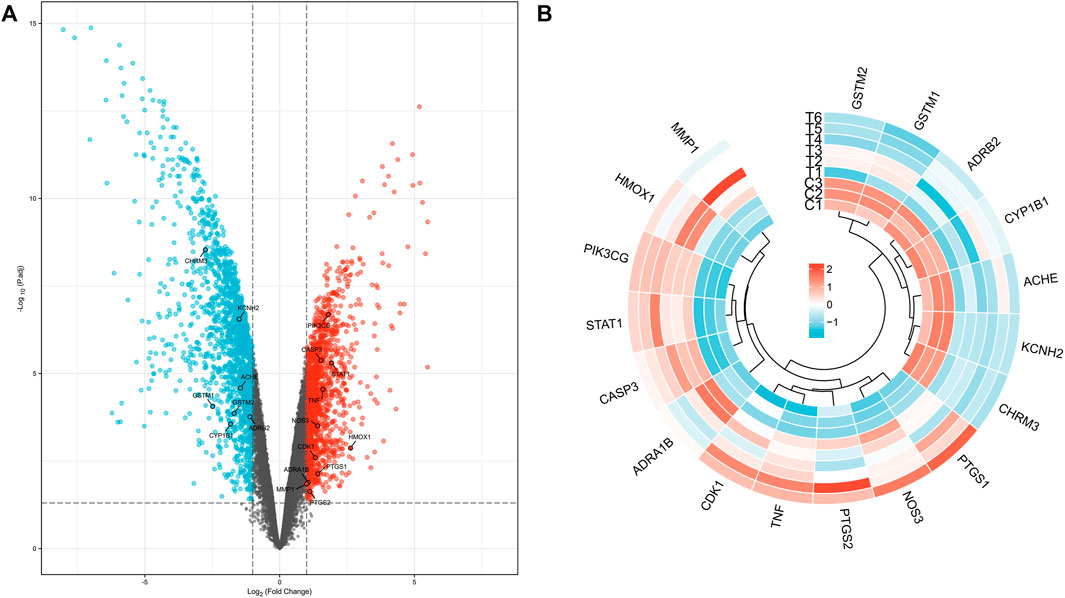
FIGURE 3. Distribution of target genes in volcano plot (A) and expression cluster analysis (B) in each PDR sample.
Construction of target protein and protein interaction network
The network revealed that TNF, CASP3, and PTGS2 were the top three core genes in this network (Figure 4A). Nine genes, including PTGS2, TIMP1, TNF, STAT1, MMP1, CASP3, NOS3, HMOX1, and TNFRSF1A, make up the network’s core. This core network’s nine genes have 31 edges between them (Figure 4B).
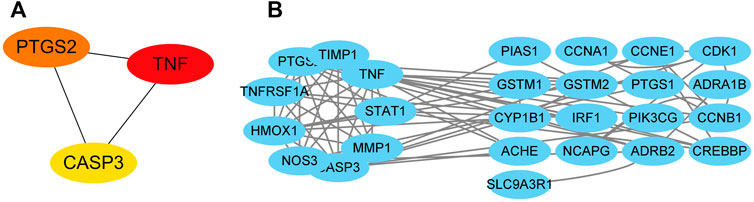
FIGURE 4. Targets’ protein-protein interaction network. The interactions among the top three core targets (A). The interaction diagram between targets (B).
GO and KEGG enrichment analysis of targets
18 targets were enriched in BP, CC, and MF by GO analysis. 23 of these BP elements were connected to biological processes such cell growth, detoxification, and positive regulation. Membrane, extracellular area, and membrane, extracellular region, and synapse parts all involved 14 CC components. 9 MF components were implicated in catalysis, molecular transducer, and antioxidant action (Figure 5).
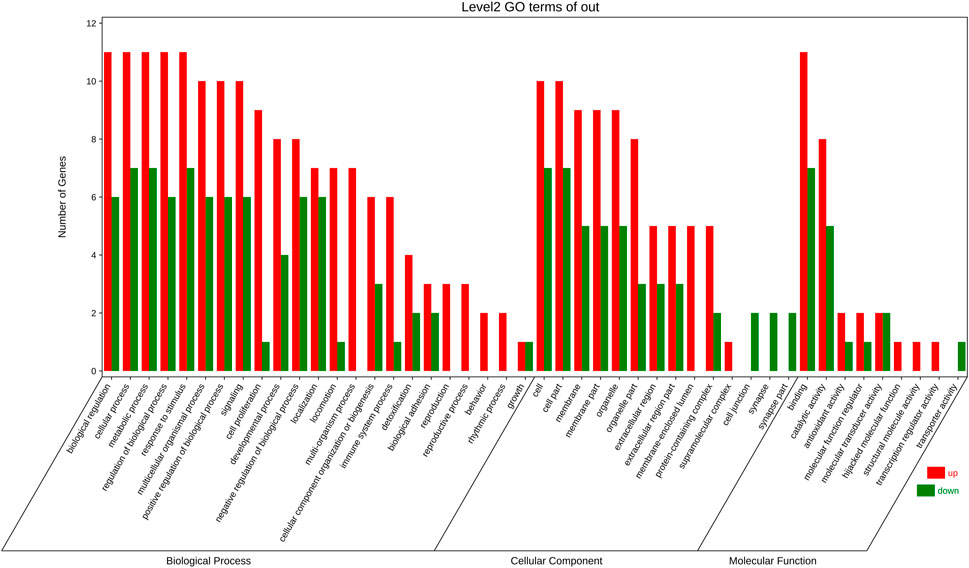
FIGURE 5. GO enrichment analysis Included are biological progress (BP) terms, molecular function (MF) terms, and (C) cellular component (CC) terms. The red bar is present number of up-regulated genes. The green one is present number of down-regulated genes.
Through KEGG enrichment analysis, 18 targets were mostly enriched in 20 items, such as Fluid shear stress and atherosclerosis, Chemical carcinogenesis—DNA adducts, IL-17 signalling pathway, AGE-RAGE signalling pathway in diabetic complications, and others. (Figure 6).
Molecular docking and molecular mechanism speculation involved in the core target
The top three targets were checked to see if they may combine with active substances using molecular docking. The findings demonstrated that each of the network’s important targets had a high affinity for the matching active component. TNF and kaempferol were interacting. Beta-sitosterol and kaempferol interact with CASP3. All six active substances interacted with PTGS2. According to the findings of the binding energy study, kaempferol’s energy required to bind TNF was -9.5 kcal/mol. Beta-sitosterol and kaempferol both bound to CASP3 with energies of −6.7 and −7.1 kcal/mol, respectively. The six active compounds had various PTGS2 binding energies. Beta-sitosterol (−9.6 kcal/mol), kaempferol (−9.5 kcal/mol), flazin (−8.1 kcal/mol), perlolyrine (−7.5 kcal/mol), ZINC03860434 (−6.7 kcal/mol), and mandenol (−4.1 kcal/mol) are listed in that sequence from small to big (Figure 7).
Schrodinger software is used to find all possible pockets on the protein surface and connect interested molecules to each pocket to find the best docking position in silico. The results showed that PTGS2 and CASP3 proteins had multiple active pockets and docked with different active molecules to generate different scores. Through these scores, many potential drug pockets were found. However, TNF has only one active pocket, which is only docked with kaempferol to form a score. This also seems to indicate that Chinese herbal medicine has the characteristics of multi-component and multi target treatment of diseases (Table 2 and Supplementary Figure S1).
After molecular docking, the molecular mechanism of key targets is mapped. The results showed that these three core targets controlled the process of PDR by inhibiting cell apoptosis, inflammation, angiogenesis and arachidonic acid metabolism through 14 key pathways such as AGE-RAGE signaling pathway in diabetic complexes (Figure 8).
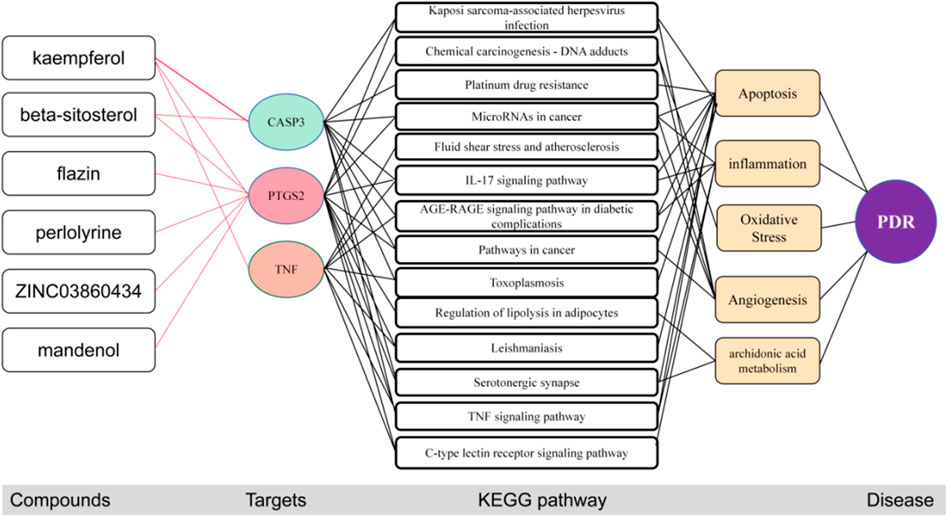
FIGURE 8. The potential molecular mechanisms of the three core targets. The red line represents the inhibition of activation of the target.
Discussion
Traditional Chinese medicine has a complicated mechanism of action since it has several components and targets. Analyzing the mechanism of action of Chinese medicine is particularly trickier when the pathophysiology of the condition is unclear. Systems network analysis and pharmacology are combined in network pharmacology. This advances our knowledge of the relationships between substances, targets, and pathways by enabling the systematic research of drug active substances, targets, and molecular pathways.
This study’s investigation of the TCM active chemicals’ network of targets revealed that Mandenol, ZINC03860434, Perlolyrine, kaempferol, Flazin, and beta-sitosterol can each have an effect on a number of different targets. This result raises the possibility that these substances are crucial to S. gtherapeutic's function in PDR. The most goals are for kaempferol, followed by beta-sitosterol. This suggests that these two components might be S. gprimary's active ingredients.
According to studies (Jorge et al., 2004), kaempferol can quickly lower blood glucose levels in diabetic mice by targeting mitochondrial calcium uptake and encouraging the metabolic/secretory coupling of pancreatic cells. By controlling the stability of gut flora, it can even reduce blood sugar (Bermont et al., 2020). More significantly, kaempferol targets VEGF and PGF to limit the activation of the Src-Akt1-Erk1/2 signalling pathway, which may effectively manage diabetic retinopathy. This prevents the angiogenesis of human retinal endothelial cells (HRECs) (Xu et al., 2017). This suggests that the PDR may be enhanced by kaempferol from S. g. The primary component of different phytosterols is -sitosterol (Babu and Jayaraman, 2020). According to Jayaraman et al. (2021), -Sitosterol can inhibit the IKK/NF-B and JNK signalling pathways in adipocytes from type 2 diabetic mice to prevent the inflammation and insulin resistance brought on by obesity. By increasing the protein expression of PPR and glucose transporter four in diabetic rats, (Ramalingam et al., 2020) discovered that -sitosterol sensitised the effects of insulin resistance on glucose homeostasis, and the long-term stability of plasma glucose may influence the development of diabetic retinopathy (DR). Since -sitosterol has been linked to diabetic retinopathy in several studies (Li et al., 2020), it is possible that this substance will help PDR progress in a similar manner.
18 DEGs intersections with the targets of S.g. active compounds were found, totaling 2642. The expression profiles of those 18 targets in each of the GES60436 samples were clustered, revealing that there were two main branches for each target’s expression: the up- and down-expression branches. Targets on each of the two major clades’ subclades may have had a complementary role in PDR development. Therefore, it was hypothesised that S.g may interact with these targets and cause their expression patterns to have the same expression pattern. The up-regulated expression branch of the PPI network, where PTGS2, TNF, and CASP3 were grouped, may be crucial in the way that S.g. treats PDR. The PPI network contained all targets other than KCNH2 and CHRM3. KCNH2 plays a part in regulating blood glucose homeostasis as a crucial component of the Kv11.1 voltage gate of cells (Engelbrechtsen et al., 2018). The pancreatic islet cells that express CHRM3 have the ability to stimulate the production of insulin (Guo et al., 2006). There is no question that both A and B have a role in controlling blood sugar, which may be relevant to PDR. However, there was no interaction between KCNH2 and CHRM3 and other proteins in the PPI network. It’s possible that those two targets interact indirectly with proteins in the PDR rather than directly, necessitating the addition of more proteins to the PPI. The network can respond logically.
For 18 targets, GO and KEGG enrichment analysis was utilised to forecast the underlying mechanism of the therapeutic action of S.g on PDR. GO analysis revealed that 18 targets, including cell proliferation, growth, and positive/negative regulation of biological processes, were enriched in 23 BP entries, indicating that these common target genes influenced PDR through a variety of biological processes. 14 items, comprising membrane part, extracellular area part, membrane, extracellular region, and synapse portion, are the major emphasis of CC entries. These targets are situated in a way that allows for direct interaction with external active substances. Nine elements—antioxidant activity, catalytic activity, hijacked molecular function, binding, molecular function regulator, structural molecule activity, transporter activity, and transcription regulator activity—made up the majority of the MF entries (Figure 5). The multimodality of S.g for the prevention and treatment of PDR is demonstrated by these enhanced entries.
According to the results of the KEGG enrichment study, 18 targets were mostly enriched in 20 items, including DNA adducts from chemical carcinogenesis, IL-17 signalling pathway, AGE-RAGE signalling pathway in diabetic complications, and others. (Figure 6). Vascular shape includes fluid shear stress and atherosclerosis pathways, which may be a key avenue for PDR vascular membrane development (Baeyens et al., 2016). Numerous tumours were linked to the DNA adducts-chemical carcinogenesis pathway. For instance, reduced GSTM2 expression in this pathway encourages cell division, cell cycle, and cell metastasis (Guo et al., 2020). Therefore, it was hypothesised that these targets are responsible for the aberrant growth of blood vessels in the diabetic retina. Inflammatory variables that may have a role in the remodelling of the retina and the tissues around it include the IL-17 signalling pathway. Diabetic problems and the AGE-RAGE signalling pathway Advanced glycation end products (AGEs) are important in cell proliferation, and RAGEs were AGEs’ receptors. This pathway also induces NF-kB activity, increases VEGF production, encourages angiogenesis or remodelling, inflammation, and death. Blood vessels are continuously created and multiplied as part of the cancer process. The toxoplasmosis pathway affected apoptosis, inflammation, and other processes. Lipid droplet production and arachidonic acid metabolism are impacted by lipolysis regulation in adipocytes. The cGMP-PKG signalling pathway was engaged in the contraction, proliferation, and differentiation of vascular smooth muscle cells. Angiogenesis and cell necrosis are also impacted by the TNF signalling pathway. MicroRNAs can cause aberrant apoptosis and unchecked cell growth in the cancer pathway. Xenobiotics were broken down by the cytochrome P450 pathway, which was engaged in the process. In vascular remodelling, angiogenesis, cell proliferation, the cell cycle, apoptosis, inflammation, and metabolism, these signalling pathways are crucial. These elements were the PDR’s known pathogenic components (Kelly et al., 2005; Zhang et al., 2005; Zhu et al., 2015; Bokhary et al., 2021; Hsiao et al., 2021).
The binding energy and locations of the active compounds corresponding to the top three target genes were examined using molecular docking in order to better understand the potential molecular mechanism of S.g preventative and therapeutic actions for PDR. The outcome demonstrated that the most trustworthy mixture was TNF and kaempferol. TNF can be divided into TNF-α and TNF-β. The secretion of inflammation-related cytokines (TNF-α, IL-6, etc.) in human retinal endothelial cells (HRECs) was increased in a high-glucose environment. It was suggested that TNF-α might be involved in the inflammatory response of retinal endothelial cells in a high-glucose environment (Zhang et al., 2019b). Studies have shown that TNF-α expression levels are reduced in diabetic retinopathy rats models after treatment (Gupta et al., 2020). kaempferol significantly reduced the expression of TNF to suppress the inflammatory response (Palacz-Wrobel et al., 2017). Kaempferol also inhibits angiogenesis by downregulating TNF-a expression (Yu et al., 2022). Inflammation and proliferation of blood vessels are important causes of PDR. Therefore, it is hypothesized that kaempferol can bind to TNF to inhibit inflammation and angiogenesis to combat PDR. This is consistent with the predicted molecular mechanism of the three core targets to combat PDR. CASP3 can be bound by beta-sitosterol and kaempferol. Kaempferol protects retinal cells from oxidative stress damage by downregulating CASP3 expression in vitro and in vivo (Yun et al., 2021). Treatment with Beta sitosterol inhibits CASP3 and thus protects cells from apoptosis (Lin et al., 2020). All six species have the ability to bind to PTGS2, with beta-sitosterol, kaempferol, flazin, perlolyrine, ZINC03860434, and mandenol having the highest binding energies. It has been shown that PTGS2 induces the synthesis of prostaglandins (PG) such as PGD2, PGE2, PGF2a, etc. PG in turn promotes the production of VEGF by paracrine, endocrine or autocrine means. The produced VEGF in turn stimulates PTGS2 to further trigger PG production thus increasing the level of PG to stimulate the expression of angiogenic factors and promote angiogenesis (Iñiguez et al., 2003). Therefore, it is hypothesized that the six active compounds inhibit the activity of PTGS2 through the active site, which in turn inhibits angiogenesis to prevent and treat PDR. However, these molecules could also be allosteric modulators so they might bind not the active site but the other sites. Schrodinger software was used to found all pockets of key targets and docked ingredients with pockets one by one. The results show that many active pockets were found. The six active compounds were scored differently with different pockets of activity for the three core targets. Among them, those scored less than—six by Schrodinger software are better drug design pockets. This was implies that the screened active ingredients have the potential to become drugs. By comparing the two molecular docking methods, the active sites of AutoDock Tools molecular docking are essentially in the active pocket predicted by Schrödinger’s software. It was also implied that a single active substance in S. g interacted with a number of PDR targets and that a single target interacted with a number of compounds, ultimately forming a treatment strategy with multiple compounds and many targets. When the active compounds bind to the target protein with the minimum free energy, the therapeutic impact of S.g may be dose-dependent. However, if the active compound dosage was insufficient, it may not be able to bind to other targets, leading to a poor therapeutic effect.
This study has several restrictions. The first is a summary of our findings at the network pharmacology, molecular docking, and bioinformatics levels. However, due to the current technology’s quick growth, the database was not updated in a timely manner, and the accuracy has to be increased. After the isolation and purification of therapeutic compounds, our results should be further validated by tests like Realtime-PCR, surface plasmon resonance and pharmacological research to confirm the bind effects. To improve the trustworthiness of the findings, more TCM target databases and freshly published literature component targets should be incorporated.
Conclusion
In conclusion, network pharmacology demonstrated that the primary active compounds in S. g, namely kaempferol and beta-sitosterol, might act on a variety of targets. S.g may have the ability to cure PDR mostly through the following pathways: cGMP-PKG signalling, TNF signalling, Metabolism, IL-17 signalling, AGE-RAGE signalling in diabetic complications, cancer, Toxoplasmosis, and lipolysis in adipocytes. In particular, six compounds bind to the top three core targets to inhibit IL-17 signaling pathway, AGE-RAGE signaling pathway in diabetic complications, Pathways in cancer and 14 other signaling pathways to inhibit inflammation, apoptosis, oxidative stress, arachidonic acid metabolism, and angiogenesis to prevent and treat PDR. The six active compounds’ therapeutic impact may be dose-dependent. Our findings provide as a guide for further investigation into the S. g preventative mechanism in PDR.
Data availability statement
Publicly available datasets were analyzed in this study. This data can be found here: The composition and target data of Luo Han Guo can be found in the public database TCMSP (https://old.tcmsp-e.com/tcmsp.php). Transcriptome data of PDR can be found from the GEO database retrieval number GSE60436 (https://www.ncbi.nlm.nih.gov/geo/geo2r/?acc=GSE60436).
Author contributions
YZ and FS contributed to conceptioni, design and wrote the first draft of the manuscript of the study. SS has revised the structure and English grammar of the full text. HJ organized the database. FS, CJ, and YZ collects and organizes data from the database. TQ and FS complete data analysis and visualization. YZ, FS, HJ, and RW wrote sections of the manuscript. All authors contributed to manuscript revision, read, and approved the submitted version. RW undertake the funding, design of the paper, check the quality of the paper, and communicate with the journal during the submission and peer review process.
Funding
This work was supported by Zunyi First People’s Hospital “123456” Future Talent Training Project, the project of Guizhou Provincial Administration of Traditional Chinese Medicine (grant number QZYY-2022-037), and project of Zunyi Science and Technology Bureau [grant number (2021) 261].
Conflict of interest
The authors declare that the research was conducted in the absence of any commercial or financial relationships that could be construed as a potential conflict of interest.
Publisher’s note
All claims expressed in this article are solely those of the authors and do not necessarily represent those of their affiliated organizations, or those of the publisher, the editors and the reviewers. Any product that may be evaluated in this article, or claim that may be made by its manufacturer, is not guaranteed or endorsed by the publisher.
Supplementary material
The Supplementary Material for this article can be found online at: https://www.frontiersin.org/articles/10.3389/fddsv.2022.1038224/full#supplementary-material
References
Babu, S., and Jayaraman, S. (2020). An update on β-sitosterol: A potential herbal nutraceutical for diabetic management. Biomed. Pharmacother. 131, 110702. doi:10.1016/j.biopha.2020.110702
Baeyens, N., Bandyopadhyay, C., Coon, B. G., Yun, S., and Schwartz, M. A. (2016). Endothelial fluid shear stress sensing in vascular health and disease. J. Clin. Invest. 126 (3), 821–828. doi:10.1172/JCI83083
Bermont, F., Hermant, A., Benninga, R., Chabert, C., Jacot, G., Santo-Domingo, J., et al. (2020). Targeting mitochondrial calcium uptake with the natural flavonol kaempferol, to promote metabolism/secretion coupling in pancreatic β-cells. Nutrients 12 (2), 538. doi:10.3390/nu12020538
Bokhary, K., Aljaser, F., Abudawood, M., Tabassum, H., Bakhsh, A., Alhammad, S., et al. (2021). Role of oxidative stress and severity of diabetic retinopathy in type 1 and type 2 diabetes. Ophthalmic Res. 64 (4), 613–621. doi:10.1159/000514722
Chen, L., Cao, Y., Zhang, H., Lv, D., Zhao, Y., Liu, Y., et al. (2018). Network pharmacology-based strategy for predicting active ingredients and potential targets of Yangxinshi tablet for treating heart failure. J. Ethnopharmacol. 219, 359–368. doi:10.1016/j.jep.2017.12.011
Engelbrechtsen, L., Mahendran, Y., Jonsson, A., Gjesing, A. P., Weeke, P. E., Jørgensen, M. E., et al. (2018). Common variants in the hERG (KCNH2) voltage-gated potassium channel are associated with altered fasting and glucose-stimulated plasma incretin and glucagon responses. BMC Genet. 19 (1), 15. doi:10.1186/s12863-018-0602-2
Gong, X., Chen, N., Ren, K., Jia, J., Wei, K., Zhang, L., et al. (2019). The fruits of Siraitia grosvenorii: A review of a Chinese food-medicine. Front. Pharmacol. 10, 1400. doi:10.3389/fphar.2019.01400
Guo, E., Wei, H., Liao, X., Wu, L., and Zeng, X. (2020). Clinical significance and biological mechanisms of glutathione S-transferase mu gene family in colon adenocarcinoma. BMC Med. Genet. 21 (1), 130. doi:10.1186/s12881-020-01066-2
Guo, Y., Traurig, M., Ma, L., Kobes, S., Harper, I., Infante, A. M., et al. (2006). CHRM3 gene variation is associated with decreased acute insulin secretion and increased risk for early-onset type 2 diabetes in Pima Indians. Diabetes 55 (12), 3625–3629. doi:10.2337/db06-0379
Gupta, S. K., Sharma, H. P., Das, U., Velpandian, T., and Saklani, R. (2020). Effect of rutin on retinal VEGF, TNF-α, aldose reductase, and total antioxidant capacity in diabetic rats: Molecular mechanism and ocular pharmacokinetics. Int. Ophthalmol. 40 (1), 159–168. doi:10.1007/s10792-019-01165-x
Hsiao, C. C., Chang, Y. C., Hsiao, Y. T., Chen, P. H., Hsieh, M. C., Wu, W. C., et al. (2021). Triamcinolone acetonide modulates TGF-β2-induced angiogenic and tissue-remodeling effects in cultured human retinal pigment epithelial cells. Mol. Med. Rep. 24 (5), 802. doi:10.3892/mmr.2021.12442
Iñiguez, M. A., Rodríguez, A., Volpert, O. V., Fresno, M., and Redondo, J. M. (2003). Cyclooxygenase-2: A therapeutic target in angiogenesis. Trends Mol. Med. 9 (2), 73–78. doi:10.1016/s1471-4914(02)00011-4
Jayaraman, S., Devarajan, N., Rajagopal, P., Babu, S., Ganesan, S. K., Veeraraghavan, V. P., et al. (2021). β-Sitosterol circumvents obesity induced inflammation and insulin resistance by down-regulating IKKβ/NF-κB and JNK signaling pathway in adipocytes of type 2 diabetic rats. Molecules 26 (7), 2101. doi:10.3390/molecules26072101
Jorge, A. P., Horst, H., de Sousa, E., Pizzolatti, M. G., and Silva, F. R. (2004). Insulinomimetic effects of kaempferitrin on glycaemia and on 14C-glucose uptake in rat soleus muscle. Chem. Biol. Interact. 149 (2-3), 89–96. doi:10.1016/j.cbi.2004.07.001
Kelly, D. J., Chanty, A., Gow, R. M., Zhang, Y., and Gilbert, R. E. (2005). Protein kinase Cbeta inhibition attenuates osteopontin expression, macrophage recruitment, and tubulointerstitial injury in advanced experimental diabetic nephropathy. J. Am. Soc. Nephrol. 16 (6), 1654–1660. doi:10.1681/ASN.2004070578
Li, H., Li, B., and Zheng, Y. (2020). Exploring the mechanism of action compound-xueshuantong capsule in diabetic retinopathy treatment based on network pharmacology. Evid. Based. Complement. Altern. Med. 2020, 8467046. doi:10.1155/2020/8467046
Li, S., and Zhang, B. (2013). Traditional Chinese medicine network pharmacology: Theory, methodology and application. Chin. J. Nat. Med. 11 (2), 110–120. doi:10.1016/S1875-5364(13)60037-0
Lin, F., Xu, L., Huang, M., Deng, B., Zhang, W., Zeng, Z., et al. (2020). β-Sitosterol protects against myocardial ischemia/reperfusion injury via targeting PPARγ/NF-κB signalling. Evid. Based. Complement. Altern. Med. 2020, 2679409. doi:10.1155/2020/2679409
Liu, H., Wang, C., Qi, X., Zou, J., and Sun, Z. (2018). Antiglycation and antioxidant activities of mogroside extract from Siraitia grosvenorii (Swingle) fruits.Siraitia grosvenorii (Swingle) fruits. J. Food Sci. Technol. 55, 1880–1888. doi:10.1007/s13197-018-3105-2
Liu, Q. P., Chen, Y. Y., Yu, Y. Y., An, P., Xing, Y. Z., Yang, H. X., et al. (2022). Bie-jia-ruan-mai-tang, a Chinese medicine formula, inhibits retinal neovascularization in diabetic mice through inducing the apoptosis of retinal vascular endothelial cells. Front. Cardiovasc. Med. 9, 959298. doi:10.3389/fcvm.2022.959298
Nawaz, I. M., Rezzola, S., Cancarini, A., Russo, A., Costagliola, C., Semeraro, F., et al. (2019). Human vitreous in proliferative diabetic retinopathy: Characterization and translational implications. Prog. Retin. Eye Res. 72, 100756. doi:10.1016/j.preteyeres.2019.03.002
Palacz-Wrobel, M., Borkowska, P., Paul-Samojedny, M., Kowalczyk, M., Fila-Danilow, A., Suchanek-Raif, R., et al. (2017). Effect of apigenin, kaempferol and resveratrol on the gene expression and protein secretion of tumor necrosis factor alpha (TNF-α) and interleukin-10 (IL-10) in RAW-264.7 macrophages. Biomed. Pharmacother. 93, 1205–1212. doi:10.1016/j.biopha.2017.07.054
Pinzi, L., and Rastelli, G. (2019). Molecular docking: Shifting paradigms in drug Discovery. Int. J. Mol. Sci. 20 (18), 4331. doi:10.3390/ijms20184331
Ramalingam, S., Packirisamy, M., Karuppiah, M., Vasu, G., Gopalakrishnan, R., Gothandam, K., et al. (2020). Effect of β-sitosterol on glucose homeostasis by sensitization of insulin resistance via enhanced protein expression of PPRγ and glucose transporter 4 in high fat diet and streptozotocin-induced diabetic rats. Cytotechnology 72 (3), 357–366. doi:10.1007/s10616-020-00382-y
Song, W., and Zhu, Y. W. (2019). Chinese medicines in diabetic retinopathy therapies. Chin. J. Integr. Med. 25 (4), 316–320. doi:10.1007/s11655-017-2911-0
Tan, Y., Fukutomi, A., Sun, M. T., Durkin, S., Gilhotra, J., and Chan, W. O. (2021). Anti-VEGF crunch syndrome in proliferative diabetic retinopathy: A review. Surv. Ophthalmol. 66 (6), 926–932. doi:10.1016/j.survophthal.2021.03.001
Xu, Wenju, and Lin, Yongchun (2009). Effect of luohan jiangtang granules on antioxidative damage in mice with diabetic retinopathy[J]. J. Mudanjiang Med. Coll. 30 (03), 13–15. doi:10.13799/j.cnki.mdjyxyxb.2009.03.009
Xu, X. H., Zhao, C., Peng, Q., Xie, P., and Liu, Q. H. (2017). Kaempferol inhibited VEGF and PGF expression and in vitro angiogenesis of HRECs under diabetic-like environment. Braz J. Med. Biol. Res. 50 (3), e5396. doi:10.1590/1414-431X20165396
Yu, R., Zhong, J., Zhou, Q., Ren, W., Liu, Z., and Bian, Y. (2022). Kaempferol prevents angiogenesis of rat intestinal microvascular endothelial cells induced by LPS and TNF-α via inhibiting VEGF/Akt/p38 signaling pathways and maintaining gut-vascular barrier integrity. Chem. Biol. Interact. 366, 110135. doi:10.1016/j.cbi.2022.110135
Yun, W., Dan, W., Liu, J., Guo, X., Li, M., and He, Q. (2021). Study on medication rules of traditional Chinese medicine against antineoplastic drug-induced cardiotoxicity based on network pharmacology and data mining. Evid. Based. Complement. Altern. Med. 2021, 7498525. doi:10.1155/2020/7498525
Zhang, J., Liang, R., Wang, L., and Yang, B. (2019). Effects and mechanisms of Danshen-Shanzha herb-pair for atherosclerosis treatment using network pharmacology and experimental pharmacology. J. Ethnopharmacol. 229, 104–114. doi:10.1016/j.jep.2018.10.004
Zhang, L., Yu, J., Ye, M., and Zhao, H. (2019). Upregulation of CKIP-1 inhibits high-glucose induced inflammation and oxidative stress in HRECs and attenuates diabetic retinopathy by modulating Nrf2/ARE signaling pathway: An in vitro study. Cell Biosci. 9, 67. doi:10.1186/s13578-019-0331-x
Zhang, X., Barile, G., Chang, S., Hays, A., Pachydaki, S., Schiff, W., et al. (2005). Apoptosis and cell proliferation in proliferative retinal disorders: PCNA, ki-67, caspase-3, and PARP expression. Curr. Eye Res. 30 (5), 395–403. doi:10.1080/02713680590956306
Keywords: Siraitia grosvenorii, proliferative diabetic retinopathy, network pharmacology, molecular docking, mechanism
Citation: Zhou Y, Shu F, Sarsaiya S, Jiang H, Jiang C, Qu T and Wang R (2022) Network pharmacology and molecular docking to explore Siraitia grosvenorii’s potential mechanism in preventing and treating proliferative diabetic retinopathy. Front. Drug. Discov. 2:1038224. doi: 10.3389/fddsv.2022.1038224
Received: 06 September 2022; Accepted: 13 October 2022;
Published: 25 October 2022.
Edited by:
Rajeev K. Tyagi, Institute of Microbial Technology (CSIR), IndiaReviewed by:
Jian Zhang, Shanghai Jiao Tong University, ChinaSergio Nemorio Hidalgo-Figueroa, Instituto Potosino de Investigación Científica y Tecnológica (IPICYT), Mexico
Copyright © 2022 Zhou, Shu, Sarsaiya, Jiang, Jiang, Qu and Wang. This is an open-access article distributed under the terms of the Creative Commons Attribution License (CC BY). The use, distribution or reproduction in other forums is permitted, provided the original author(s) and the copyright owner(s) are credited and that the original publication in this journal is cited, in accordance with accepted academic practice. No use, distribution or reproduction is permitted which does not comply with these terms.
*Correspondence: Ruixia Wang, NjMwMzkwNDI3QHFxLmNvbQ==
†These authors have contributed equally to this work