- Department of Cardiology, The Second Hospital of Hebei Medical University, Shijiazhuang, China
Background: Atrial fibrillation (AF) is the most common cardiac arrhythmia, significantly increasing the risk of death and stroke. The left atrial appendage (LAA) plays a crucial role in the development of AF. Reduced left atrial appendage emptying velocity (LAAEV) is an important indicator of nonvalvular AF, associated with thrombosis and recurrence after catheter ablation. This study aims to identify factors influencing LAAEV and construct a predictive model for LAAEV in nonvalvular AF patients.
Methods: This retrospective cohort study included 1,048 nonvalvular AF patients hospitalized at the Second Hospital of Hebei Medical University from January 1, 2015, to December 31, 2021. Patients underwent transthoracic and transesophageal echocardiography and had complete laboratory data. Statistical analyses included binary logistic regression and multiple linear regression to identify independent predictors of reduced LAAEV and construct a predictive model.
Results: Patients were divided into two groups: reduced LAAEV (<40 cm/s) and normal LAAEV (≥40 cm/s). The reduced LAAEV group included 457 patients (43.61%), with significant differences in age, gender, alcohol consumption, heart failure (HF), ischemic stroke, AF type, resting heart rate, CHA2DS2-VASc score, serum creatinine (SCR), serum uric acid (SUA), estimated glomerular filtration rate (eGFR), glycated hemoglobin (HbA1C), β2 macroglobulin (B2M), left atrial diameter (LAD), and left ventricular ejection fraction (LVEF) compared to the normal LAAEV group. Logistic regression analysis identified age (OR 0.974, 95% CI 0.951–0.997, P = 0.028), HF (OR 0.637, 95% CI 0.427–0.949, P = 0.027), AF type [Persistent AF vs. PAF (OR 0.063, 95% CI 0.041–0.095, P = 0) Long-standing Persistent AF vs. PAF (OR 0.077, 95% CI 0.043–0.139, P = 0)], LAD (OR 0.872, 95% CI 0.836–0.91, P < 0.001), and LVEF (OR 1.057, 95% CI 1.027–1.089, P = 0) as independent predictors of reduced LAAEV. Multiple linear regression analysis included age, AF type, LAD, and LVEF in the final predictive model, explaining 43.5% of the variance in LAAEV (adjusted R² = 0.435).
Conclusion: Age, HF, type of AF, LAD, and LVEF are independent predictors of reduced LAAEV. The predictive model (LAAEV = 96.567–15.940 × AFtype–1.309 × LAD–0.18 × Age + 37.069 × LVEF) demonstrates good predictive value, aiding in the initial assessment and management of nonvalvular AF patients.
Introduction
Atrial fibrillation (AF) is the most common cardiac arrhythmia (1), increases the risk of death by 1.5–1.9 times (2) and stroke risk by fivefold (3). The left atrial appendage (LAA) plays a crucial role in the development of AF. As a part of the left atrium (LA), the LAA has both contractile and endocrine functions, but it differs from the LA in developmental, structural, and physiological characteristics, contributing significantly to arrhythmogenesis and thrombosis (4). Approximately 90% of thrombi in nonvalvular AF patients originate from the LAA (5). Left atrial appendage emptying velocity (LAAEV) is an important indicator of nonvalvular AF, measured via transesophageal echocardiography, an invasive procedure (6). Decreased LAAEV is a known risk factor for LAA thrombosis in patients with nonvalvular AF (7). Catheter ablation is becoming the first-line treatment for nonvalvular AF (8). Decreased left atrial appendage emptying velocity is an independent predictor of recurrence after catheter ablation in patients with nonvalvular AF (9).
Several factors influence LAAEV. Previous studies have shown that LAAEV is related to the degree of LAA fibrosis (10), serum uric acid levels (11), nocturnal intermittent hypoxia (12), low voltage area in the anterior LA wall (13) and the morphology and opening area of the LAA (14). Retrospective studies identified non-paroxysmal AF, heart failure (HF), and age >65 years as predictors of LAAEV <20 cm/s and LAA thrombosis (15). Another retrospective study showed that LAAEV was not associated with LAA morphology and type of AF but with a higher CH2DS2 score and greater LAA volume (16). However, the sample size of studies on the factors influencing LAAEV is small and still controversial. Therefore, this study aims to investigate the potential factors influencing LAAEV and construct a predictive model for easier estimation of LAAEV, aiding in thromboprophylaxis and recurrence prevention post-catheter ablation in AF patients.
Methods
This single-center retrospective cohort study collected laboratory tests and echocardiographic examinations of nonvalvular AF patients hospitalized at the Second Hospital of Hebei Medical University from January 1, 2015, to December 31, 2021, using the electronic medical record system.
Inclusion criteria for patients were as follows. (1) Age >18 years old. (2) Non-valvular AF type. (3) Underwent transthoracic and transesophageal echocardiography with complete laboratory data. The exclusion criteria were as follows. (1) Moderate or severe aortic or mitral stenosis. (2) History of surgery on the mitral or aortic valve. (3) Missing clinical data.
AF was diagnosed based on electrocardiographic features of AF on a 12-lead electrocardiogram, a duration of AF >30 s on a 24-hour dynamic electrocardiogram, or a previous episode of AF. Paroxysmal AF(PAF) was defined as AF that terminated spontaneously or with intervention within seven days, persistent AF(PeAF) was defined as AF lasting more than seven days, and long-standing persistent was defined as continuous AF of >12 months’ duration (17). Diabetes mellitus (DM) was defined by random blood glucose ≥200 mg/dl, fasting blood glucose ≥126 mg/dl, or 2-hour blood glucose ≥200 mg/dl based on a 75 g oral glucose tolerance test, or the use of hypoglycemic medications or insulin. Hypertension was defined as systolic blood pressure ≥140 mmHg, or diastolic blood pressure ≥90 mmHg, or use of antihypertensive drugs. HF included past or current signs and symptoms of HF with both low (<40%) and preserved ejection fraction (≥40%) and other clinical evidence of cardiac dysfunction. Ischemic stroke was diagnosed based on CT or MRI evidence or a history of ischemic stroke. Peripheral arterial disease (PAD) was diagnosed via vascular Doppler ultrasound or past medical history. Coronary heart disease (CHD) was diagnosed according to relevant guidelines or history of CHD. Transthoracic echocardiograms and transesophageal echocardiograms were measured by an experienced sonographer and reviewed by an experienced senior sonographer. Left atrial diameters (LAD) were measured from the anteroposterior diameter of the LA, and left ventricular ejection fraction(LVEF) was measured using the modified Simpson method (18). LAAEV was obtained by transesophageal echocardiography and the pulsed doppler sample volume was typically placed 1–2 cm from the orifice within the chamber (6) (Figure 1). LAAEV of <40 cm/s were considered to be reduced (19, 20). LAAEV <20 cm/s were associated with embolic events (21). Therefore, a subgroup analysis was conducted. The estimated glomerular filtration rate (eGFR) was calculated by the CKD-EPI formula based on serum creatinine (SCr) (22). Patients’ CHA2DS2-VASc scores were calculated upon admission. The Clinical, imaging, and laboratory data were collected at the time of admission.
Statistical analysis
Statistical analyses were performed using SPSS (version 26.0, SPSS Inc., Chicago, IL, USA). The Shapiro-Wilk test assessed whether continuous variables were normally distributed. Non-normally distributed continuous variables were expressed as medians and interquartile ranges, with the Mann-Whitney U-test used for sample comparisons. Categorical variables were presented as numbers and percentages, compared using chi-square tests. Binary logistic regression identified independent risk factors, with the odds ratio (OR) value and 95% confidence interval calculated. Multiple linear regression analysis, performed using stepwise regression with αin ≤0.05 and αout ≥0.1, constructed a predictive model. Pearson correlation analysis and Spearman correlation analysis in bivariate correlation analysis selected variables for the multiple linear regression model. Two-tailed P-values less than 0.05 were considered statistically significant.
Result
Patients with non-valvular AF hospitalized at the Second Hospital of Hebei Medical University from January 1, 2015, to December 31, 2021, meeting the inclusion criteria, were included in this study. A total of 1,048 patients were analyzed. Patients were categorized into a reduced LAAEV group (LAAEV <40 cm/s) and a normal LAAEV group (LAAEV ≥40 cm/s). The reduced LAAEV group comprised 457 patients (43.61%), including 346 males (61.02%) and 221 females (38.98%). The normal LAAEV group consisted of 591 patients (56.39%), including 270 males (56.13%) and 211 females (43.87%) (Table 1).
Baseline data
Patients with reduced LAAEV were older compared to those with normal LAAEV [64(57–69) vs. 62(55–69) P = 0.008] (unit, years old), had a higher percentage of males [285(62.36%) vs. 331 (56.01%) P = 0.038], had a faster resting heart rate [80(70–94.5) vs. 71(61–82) P = 0] (bpm) and had a higher rate of alcohol consumption [90(19.96%) vs. 87(14.72%) P = 0.033]. There was no statistically significant difference in the proportion of smokers between the two groups. In terms of underlying diseases, the reduced LAAEV group had a higher rate of HF [279(61.05%) vs. 143(24.20%) P = 0] and ischemic stroke [185(40.48%) vs. 156(26.40%) P = 0]. No statistically significant differences were observed in the prevalence of hypertension, DM, PAD, and CHD between the groups. In addition, the reduced LAAEV group also had a higher percentage of PeAF [257(60.2%) vs. 50(8.5%) P = 0] and long-standing persistent AF [97(21.2%) vs. 20(3.4%) p = 0], and a higher CHA2DS2-VASc score [3(2–5) vs. 3(1–4) P = 0.001].
In laboratory tests, transthoracic echocardiography and transesophageal echocardiography, compared to the normal LAAEV group, the reduced LAAEV group had higher levels of SCr [73(64–84) vs. 69(60–80.4) P = 0] (unit, mmol/L), glycated hemoglobin(HbA1C) [5.9%(5.6%−6.4%) vs. 5.8%(5.5%−6.2%) P = 0], β2 macroglobulin(B2M) [1.9(1.6–2.3) vs. 1.8(1.55–2.1) P = 0] (unit, mg/L), and serum uric acid(SUA) [335(281–398.5) vs. 304(259–357) P = 0] (unit, mmol/L). The reduced LAAEV group also had longer LAD [41 (38–44) vs. 35 (33–38), P = 0] (unit, mm), but lower eGFR [88.01 (76.93–96.79) vs. 92.45 (82.06–100.27), P = 0] (unit, ml/min/1.73m2) and LVEF [60.7% (55.55%−63.08%) vs. 63.2% (61.4%−66.1%), P = 0]. Differences in high-sensitivity C-reactive protein (hs-CRP), triglycerides, cholesterol (TC), low-density lipoprotein (LDL), high-density lipoprotein (HDL), apolipoprotein A (ApoA), and apolipoprotein B (ApoB) were not statistically significant between the two groups (Table 1).
Logistic regression analysis
Based on the baseline data comparison, statistically significant differences were found in age, gender, alcohol consumption, HF, ischemic stroke, AF type, CHA2DS2-VASc score, resting heart rate, SCr, SUA, eGFR, HbA1C, B2M, LAD, and LVEF between the reduced and normal LAAEV groups. Ischemic stroke was excluded from the binary logistic regression analysis as it was not a factor in LAAEV. The CHA2DS2-VASc scores were also excluded because they include factors such as age, gender, HF, and ischemic stroke. eGFR was calculated from SCr values, so only eGFR was selected for inclusion in the binary logistic regression analysis. Since eGFR was calculated from SCr values, only eGFR was included in the binary logistic regression analysis. Thus, age, gender, alcohol consumption, HF, AF type, resting heart rate, eGFR, HbA1C, SUA, B2M, LAD, and LVEF were included in the binary logistic regression analysis.
Binary logistic regression results indicated that advanced age (OR 0.974, 95% CI 0.951–0.997, P = 0.028), suffering from HF (OR 0.637, 95% CI 0.427–0.949, P = 0.027), PeAF (PeAF vs. PAF (OR 0.063, 95% CI 0.041–0.095, P = 0), long-standing persistent AF (Long-standing Persistent AF vs. PAF (OR 0.077, 95% CI 0.043–0.139, P = 0), and lager LAD (OR 0.872, 95% CI 0.836–0.91, P = 0) were independent predictors of reduced LAAEV, while higher LVEF (OR 1.055, 95%CI 1.024–1.086 P = 0) were independent predictors of normal LAAEV. However, gender, alcohol consumption, eGFR, HbA1C, resting heart rate, SUA, and B2M were confounding factors (Table 2).
Multiple linear regression model of LAAEV
Correlation analysis indicated that age (r = −0.106, P = 0.001), alcohol consumption (r = −0.080, P = 0.01), AF Type (r = −0.661, P = 0), HF (r = −0.349, P = 0), resting heart rate (−0.220 P = 0), SUA (r = −0.14, P = 0), hs-CRP (r = −0.076, P = 0), HbA1C (r = −0.126, P = 0), B2M (r = −0.067 P = 0.029), and LAD (r = −0.513, P = 0) were negatively correlated with LAAEV, while eGFR (r = 0.162, P = 0) and LVEF (r = 0.333, P = 0) were positively correlated. Gender, Smoking, DM, hypertension, PAD, CAD, TC, TG, LDL, HDL, ApoA, and ApoB had no significant correlation with LAAEV (Supplementary Table 1). Scatter plots of age, hs-CRP, HbA1C, resting heart rate, SUA, B2M, LAD, eGFR, and LVEF vs. LAAEV demonstrated linear relationships (Figure 2).
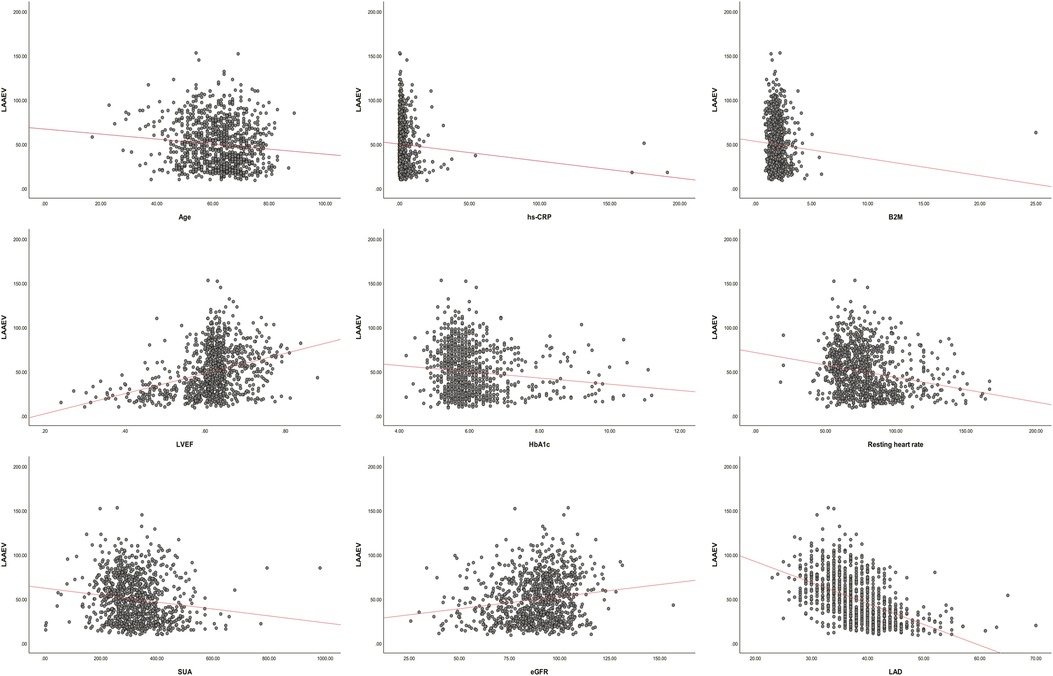
Figure 2. LAAEV, left atrial appendage emptying velocity; LVEF, left ventricular ejection fraction; SUA, serum uric acid; hs-CRP, high-sensitivity C-reactive protein; eGFR, estimated glomerular filtration rate; B2M, β2-microglobulin; LAD, left atrium diameter; HbA1C, glycated hemoglobin.
Independent variables including age, alcohol consumption, AF type, HF, hs-CRP, HbA1C, resting heart rate, SUA, B2M, LAD, eGFR, and LVEF were selected for stepwise regression to construct a multiple linear regression model of LAAEV. After stepwise regression, age, type of AF, LAD, and LVEF were included in the regression model. The final constructed multiple linear regression model was statistically significant (F = 200.661 P = 0), and 43.5% of the variance in the dependent variable LAASE could be explained by changes in the type of AF, LAD, age, and LVEF (corrected R2 = 0.435). The regression coefficients (B) and 95% confidence intervals of the regression model are shown in Table 3. The regression equation is: LAAEV = 96.567–15.940 × AFtype–1.309 × LAD–0.18 × Age + 37.069 × LVEF, where PAF is coded as 0, PeAF as 1, Long-standing Persistent AF as 2, LAD is in millimeters, and age is in years.
Subgroup analysis
In the subgroup analysis, patients with LAAEV <20 cm/s were older [64(59–70) vs. 63(55–69) P = 0.031] (unit, years old), had a higher rate of HF [72(61.5%) vs. 350(37.6%) P = 0], a higher proportion of PeAF [80(68.4%) vs. 245(26.3%) P = 0] and long-standing persistent AF [24(20.5%) vs. 93(10%) P = 0], a higher CHA2DS2-VASc score [3(2–5) vs. 3(1–4) P = 0.001], and a faster resting heart rate [84(70–93.5) vs. 75(64–86) P = 0] (bpm). However, there were no statistically significant differences between the two groups in terms of gender, proportion of smokers, alcohol consumption, and the prevalence of hypertension, diabetes, PAD, ischemic stroke, and CHD. In terms of laboratory tests and ultrasound examinations, patients with LAAEV <20 cm/s had higher levels of hs-CRP [1.8(1–4.6) vs. 1.4(0.7–2.7) P = 0.001] (unit, mg/L), SCr [73(63.2–88) vs. 70(61–81) P = 0.032] (unit μmmol/L) and SUA [332(291.5–408) vs. 316(264–375) P = 0.008] (unit, μmmol/L) as well as larger LAD [42 (30–46) vs. 37 (34–40), P = 0] (unit,mm), while lower eGFR [86.72 (74.55–96.54) vs. 91.25 (81.09–99.14), P = 0.008] (unit, ml/min/1.73m2), TC levels [3.8(3.265–4.285) vs. 4(3.39–4.68) P = 0.034] (unit, mmol/L), and LVEF [58.33% (51%−62.885%) vs. 62.03% (60.23%−64.79%), P = 0] (Supplementary Table 2). Since the CHA2DS2-VASc score includes variables such as age and HF and eGFR is calculated from SCr, the CHA2DS2-VASc score and SCr were excluded from the binary logistics regression analysis. Ultimately, age, HF, AF type, resting heart rate, eGFR, SUA, TC, LAD and LVEF were included in the regression analysis. Binary logistic regression analysis showed that PeAF [PeAF vs. PAF (OR 0.119, 95% CI 0.061–0.229, P = 0], long-standing Persistent AF [Long-standing Persistent AF vs. PAF (OR 0.174, 95% CI 0.08–0.379, P = 0] and lager LAD(OR 0.886, 95% CI 0.851–0.923, P = 0) were independent predictors of LAAEV <20 cm/s, while higher LVEF(OR 1.029, 95% CI 1.002–1.085, P = 0.037) were independent predictors of LAAEV2 ≥20 cm/s (Supplementary Table 3).
Discussion
This retrospective study analyzed non-valvular AF patients hospitalized at the Second Hospital of Hebei Medical University from January 1, 2015, to December 31, 2021, to identify factors influencing reduced LAAEV and construct a prediction model. The results indicated that age, HF, AF type, LAD, and LVEF are independent predictors of reduced LAAEV, and the constructed model has good predictive value.
Advanced age is a known independent predictor of cardiogenic stroke in patients with nonvalvular AF patients (23), and with a lower age threshold for stroke in Asian populations (24). In patients with PeAF, advanced age correlates with LAA thrombosis, which is less likely to resolve in older patients (25). Age remains an independent predictor of LAA thrombosis even after anticoagulation therapy (26). In healthy subjects, thrombus formation is detected in patients with lower LAAEV, and age is negatively correlated with LAAEV (27), corroborating our finding that higher age predicts lower LAAEV. In patients with nonvalvular AF, age-induced reduction in LAAEV appears to be an important reason why older patients are more susceptible to cardiac stroke. Aging is often accompanied by a decline in LA function (28), and increasing age is associated with a higher burden of LA fibrosis (29). In contrast, LA fibrosis strongly correlates with LAA fibrosis (30). This may be one of the reasons for the age-induced decrease in LAAEV, warranting further investigation.
HF is another independent predictor of cardiogenic stroke in patients with nonvalvular AF (23), and increases stroke risk even in sinus rhythm (31). In patients with nonvalvular AF with LAA thrombus, HF is not only an independent predictor of LAA thrombosis (32) but also an independent risk factor for the failure of the thrombus to resolve (33). Reduced LVEF also predicts LAA thrombosis in these patients (32). Our study confirmed HF and LVEF as independent predictors of reduced LAAEV, with LVEF negatively correlated with LAAEV. Patients with reduced LVEF or HF in nonvalvular AF are more likely to develop cardiac stroke or LAA thrombus, possibly related to the reduced LAAEV they cause. HF leads to decreased LA function, exacerbated in reduced ejection fraction HF (34). An animal experiment illustrated that HF can lead to remodeling and fibrosis of the LA (35). This explains, to some extent, the reduced LVEF in patients with HF and reduced LVEF, but further studies are needed.
PeAF is associated with more severe cardiac stroke (36). In addition, a real-world study showed that PeAF was independently associated with LAA thrombosis (37). PeAF patients have a higher stroke and systemic embolism risk than PAF patients, even with anticoagulation therapy (38). The greater susceptibility to LAA thrombus formation in patients with PeAF may be related to the reduced LAAEV. In this study, PeAF was an independent predictor of reduced LAAEV. A retrospective study showed that the extent of LA fibrosis assessed by gadolinium-enhanced MRI was greater in patients with PeAF than in PAF (39), which may contribute to their reduced LAAEV. The LAAEV serves as a predictive marker for the extent of LA fibrosis in patients with long-term persistent atrial fibrillation (40). A decreased LAAEV in these patients is potentially indicative of more advanced LA fibrosis. Still, the exact mechanism of reduced LAAEV in patients with Non-paroxysmal AF is unknown.
LA enlargement is more common in patients with AF, and AF is a cause of LA enlargement (41) and is also an independent predictor of stroke and systemic embolism in patients with nonvalvular AF (42). It is an independent predictor of recurrent cardiac stroke in the Chinese population (43). Retrospective studies had shown LA size as an independent predictor of reduced LAAEV (44)., with our study confirming this relationship. A study showed that LAD was independently associated with LA fibrosis in patients with nonvalvular AF (45). Patients with larger LAD have greater LA fibrosis, which may explain its association with reduced LAAEV.
LAAEV, measured via invasive transesophageal echocardiography, is crucial in nonvalvular AF (6). Our study provides a predictive model for LAAEV, with easily obtained variables like age, AF type, LAD, and LVEF, offering excellent predictive value for initial LAAEV assessment in nonvalvular AF patients, aiding in treatment evaluation.
This study demonstrated that age, AF type, HF, LAD, and LVEF independently predict reduced LAAEV, explaining the susceptibility to stroke in these patients. The predictive model has good value but requires further improvement. As a single-center retrospective study with patients from the Hebei region, findings may not generalize to other populations. Since this is a retrospective study, other indicators that may affect LAAEV, such as LA emptying fraction, LA volume, brain natriuretic peptide, brain natriuretic peptide and atrial fibrillation burden, were not available. So future research should include more variables and diverse populations to enhance the model’s predictive value.
Conclusion
Age, heart failure, type of AF, LAD, and LVEF were identified as independent predictors of reduced LAAEV. The constructed model (LAAEV = 96.567–15.940 × AFtype–1.309 × LAD–0.18 × Age + 37.069 × LVEF) has demonstrated good predictive value, where PAF is coded as 0, PeAF as 1, Long-standing Persistent AF as 2, LAD is in millimeters, and age is in years.
Data availability statement
The raw data supporting the conclusions of this article will be made available by the authors, without undue reservation.
Author contributions
WH: Data curation, Methodology, Software, Writing – original draft. LEY: Software, Writing – review & editing. QL: Methodology, Writing – review & editing. YZ: Software, Writing – review & editing. YLZ: Validation, Writing – review & editing. LW: Data curation, Writing – review & editing. LIY: Methodology, Project administration, Resources, Supervision, Writing – review & editing.
Funding
The author(s) declare that no financial support was received for the research, authorship, and/or publication of this article.
Conflict of interest
The authors declare that the research was conducted in the absence of any commercial or financial relationships that could be construed as a potential conflict of interest.
Publisher's note
All claims expressed in this article are solely those of the authors and do not necessarily represent those of their affiliated organizations, or those of the publisher, the editors and the reviewers. Any product that may be evaluated in this article, or claim that may be made by its manufacturer, is not guaranteed or endorsed by the publisher.
Supplementary material
The Supplementary Material for this article can be found online at: https://www.frontiersin.org/articles/10.3389/fcvm.2024.1468379/full#supplementary-material
References
1. Tsao CW, Aday AW, Almarzooq ZI, Anderson CAM, Arora P, Avery CL, et al. Heart disease and stroke statistics−2023 update: a report from the American Heart Association. Circulation. (2023) 147(8):e93–e621. doi: 10.1161/CIR.0000000000001123
2. Benjamin EJ, Wolf PA, D'Agostino RB, Silbershatz H, Kannel WB, Levy D. Impact of atrial fibrillation on the risk of death: the framingham heart study. Circulation. (1998) 98(10):946–52. doi: 10.1161/01.CIR.98.10.946
3. Wolf PA, Kannel WB, McGee DL, Meeks SL, Bharucha NE, McNamara PM. Duration of atrial fibrillation and imminence of stroke: the framingham study. Stroke. (1983) 14(5):664–7. doi: 10.1161/01.STR.14.5.664
4. Al-Saady NM, Obel OA, Camm AJ. Left atrial appendage: structure, function, and role in thromboembolism. Heart (British Cardiac Society). (1999) 82(5):547–54. doi: 10.1136/hrt.82.5.547
5. Schotten U, Verheule S, Kirchhof P, Goette A. Pathophysiological mechanisms of atrial fibrillation: a translational appraisal. Physiol Rev. (2011) 91(1):265–325. doi: 10.1152/physrev.00031.2009
6. Hahn RT, Abraham T, Adams MS, Bruce CJ, Glas KE, Lang RM, et al. Guidelines for performing a comprehensive transesophageal echocardiographic examination: recommendations from the American society of echocardiography and the society of cardiovascular anesthesiologists. J Am Soc Echocardiogr. (2013) 26(9):921–64. doi: 10.1016/j.echo.2013.07.009
7. Pollick C, Taylor D. Assessment of left atrial appendage function by transesophageal echocardiography. Implications for the development of thrombus. Circulation. (1991) 84(1):223–31. doi: 10.1161/01.CIR.84.1.223
8. Morillo CA, Verma A, Connolly SJ, Kuck KH, Nair GM, Champagne J, et al. Radiofrequency ablation vs antiarrhythmic drugs as first-line treatment of paroxysmal atrial fibrillation (RAAFT−2): a randomized trial. JAMA. (2014) 311(7):692–700. doi: 10.1001/jama.2014.467
9. You L, Zhang X, Yang J, Wang L, Zhang Y, Xie R. The long-term results of three catheter ablation methods in patients with paroxysmal atrial fibrillation: a 4-year follow-up study. Front Cardiovasc Med. (2021) 8:719452. doi: 10.3389/fcvm.2021.719452
10. Miyauchi S, Tokuyama T, Uotani Y, Miyamoto S, Ikeuchi Y, Okamura S, et al. Association between left atrial appendage fibrosis and thrombus formation: a histological approach. J Cardiovasc Electrophysiol. (2022) 33(4):677–87. doi: 10.1111/jce.15384
11. Celik M, Yalcinkaya E, Yuksel UC, Gokoglan Y, Bugan B, Kabul HK, et al. Increased serum uric acid levels are correlated with decreased left atrial appendage peak flow velocity in patients with atrial fibrillation. Medical principles and practice: international journal of the Kuwait university. Health Science Centre. (2015) 24(3):263–8. doi: 10.1159/000373892
12. Kimura T, Kohno T, Nakajima K, Kashimura S, Katsumata Y, Nishiyama T, et al. Effect of nocturnal intermittent hypoxia on left atrial appendage flow velocity in atrial fibrillation. Can J Cardiol. (2015) 31(7):846–52. doi: 10.1016/j.cjca.2014.12.032
13. Hori Y, Nakahara S, Nishiyama N, Fukuda R, Ukaji T, Sato H, et al. Impact of low-voltage zones on the left atrial anterior wall on the reduction in the left atrial appendage flow velocity in persistent atrial fibrillation patients. J Interv Card Electrophysiol. (2019) 56(3):299–306. doi: 10.1007/s10840-019-00532-z
14. Chen L, Xu C, Chen W, Zhang C. Left atrial appendage orifice area and morphology is closely associated with flow velocity in patients with nonvalvular atrial fibrillation. BMC Cardiovasc Disord. (2021) 21(1):442. doi: 10.1186/s12872-021-02242-9
15. Gawałko M, Budnik M, Uziębło-Życzkowska B, Krzesiński P, Scisło P, Kochanowski J, et al. Decreased left atrial appendage emptying velocity as a link between atrial fibrillation type, heart failure and older age and the risk of left atrial thrombus in atrial fibrillation. Int J Clin Pract. (2020) 74(11):e13609. doi: 10.1111/ijcp.13609
16. Ouchi K, Sakuma T, Higuchi T, Yoshida J, Narui R, Nojiri A, et al. Computed tomography findings associated with the reduction in left atrial appendage flow velocity in patients with atrial fibrillation. Heart Vessels. (2022) 37(8):1436–45. doi: 10.1007/s00380-022-02041-y
17. Hindricks G, Potpara T, Dagres N, Arbelo E, Bax JJ, Blomström-Lundqvist C, et al. 2020 ESC guidelines for the diagnosis and management of atrial fibrillation developed in collaboration with the European association for cardio-thoracic surgery (EACTS): the task force for the diagnosis and management of atrial fibrillation of the European Society of Cardiology (ESC) developed with the special contribution of the European heart rhythm association (EHRA) of the ESC. Eur Heart J. (2021) 42(5):373–498. doi: 10.1093/eurheartj/ehaa612
18. Lang RM, Badano LP, Mor-Avi V, Afilalo J, Armstrong A, Ernande L, et al. Recommendations for cardiac chamber quantification by echocardiography in adults: an update from the American society of echocardiography and the European association of cardiovascular imaging. Eur Heart J Cardiovasc Imaging. (2015) 16(3):233–70. doi: 10.1093/ehjci/jev014
19. Fatkin D, Kelly RP, Feneley MP. Relations between left atrial appendage blood flow velocity, spontaneous echocardiographic contrast and thromboembolic risk in vivo. J Am Coll Cardiol. (1994) 23(4):961–9. doi: 10.1016/0735-1097(94)90644-0
20. Sadanandan S, Sherrid MV. Clinical and echocardiographic characteristics of left atrial spontaneous echo contrast in sinus rhythm. J Am Coll Cardiol. (2000) 35(7):1932–8. doi: 10.1016/S0735-1097(00)00643-4
21. Zabalgoitia M, Halperin JL, Pearce LA, Blackshear JL, Asinger RW, Hart RG. Transesophageal echocardiographic correlates of clinical risk of thromboembolism in nonvalvular atrial fibrillation. Stroke prevention in atrial fibrillation III investigators. J Am Coll Cardiol. (1998) 31(7):1622–6. doi: 10.1016/S0735-1097(98)00146-6
22. Levey AS, Stevens LA, Schmid CH, Zhang YL, Castro AF 3rd, Feldman HI, et al. A new equation to estimate glomerular filtration rate. Ann Intern Med. (2009) 150(9):604–12. doi: 10.7326/0003-4819-150-9-200905050-00006
23. Olesen JB, Lip GY, Hansen ML, Hansen PR, Tolstrup JS, Lindhardsen J, et al. Validation of risk stratification schemes for predicting stroke and thromboembolism in patients with atrial fibrillation: nationwide cohort study. BMJ (Clinical Research ed. (2011) 342:d124. doi: 10.1136/bmj.d124
24. Kim TH, Yang PS, Yu HT, Jang E, Uhm JS, Kim JY, et al. Age threshold for ischemic stroke risk in atrial fibrillation. Stroke. (2018) 49(8):1872–9. doi: 10.1161/STROKEAHA.118.021047
25. Hautmann M, Zacher M, Fuchs S, Pérez CM, Ahmidou A, Kerber S, et al. Left atrial appendage thrombus formation, potential of resolution and association with prognosis in a large real-world cohort. Sci Rep. (2023) 13(1):889. doi: 10.1038/s41598-023-27622-3
26. Da Costa A, Delolme C, Guichard JB, Gerbay A, Pierrard R, Romeyer-Bouchard C, et al. Comparison of prevalence and management of left atrial appendage thrombi under old and new anticoagulants prior to left atrial catheter ablation. Am Heart J. (2017) 193:8–15. doi: 10.1016/j.ahj.2017.07.016
27. Tabata T, Oki T, Fukuda N, Iuchi A, Manabe K, Kageji Y, et al. Influence of aging on left atrial appendage flow velocity patterns in normal subjects. J Am Soc Echocardiogr. (1996) 9(3):274–80. doi: 10.1016/S0894-7317(96)90140-6
28. Singh A, Carvalho Singulane C, Miyoshi T, Prado AD, Addetia K, Bellino M, et al. Normal values of left atrial size and function and the impact of age: results of the world alliance societies of echocardiography study. J Am Soc Echocardiogr. (2022) 35(2):154–64.e3. doi: 10.1016/j.echo.2021.08.008
29. Akoum N, Mahnkopf C, Kholmovski EG, Brachmann J, Marrouche NF. Age and sex differences in atrial fibrosis among patients with atrial fibrillation. Europace. (2018) 20(7):1086–92. doi: 10.1093/europace/eux260
30. Hopman L, Frenaij IM, Solis-Lemus JA, Mathari SEI, Bhagirath P, Niederer S, et al. Cardiac magnetic resonance imaging to study the relation between left atrial fibrosis and left atrial appendage fibrosis in atrial fibrillation patienTS. J Am Coll Cardiol. (2023) 81(8_Supplement):82. doi: 10.1016/S0735-1097(23)00526-0
31. Hjalmarsson C, Fu M, Zverkova Sandström T, Schaufelberger M, Ljungman C, Andersson B, et al. Risk of stroke in patients with heart failure and sinus rhythm: data from the Swedish heart failure registry. ESC heart Failure. (2021) 8(1):85–94. doi: 10.1002/ehf2.13091
32. Shah M, Mobaligh N, Niku A, Shiota T, Siegel RJ, Rader F. Predictors of left atrial appendage thrombus despite NOAC use in nonvalvular atrial fibrillation and flutter. Int J Cardiol. (2020) 317:86–90. doi: 10.1016/j.ijcard.2020.04.070
33. Yang S, Zhang Y, Chen N, Shi J, Ju W, Chen H, et al. Characteristics, thrombus resolution, and long-term outcomes in patients with nonvalvular atrial fibrillation and atrial thrombus. Clin Appl Thromb Hemost. (2023) 29:10760296231168097. doi: 10.1177/10760296231168097
34. Jin X, Nauta JF, Hung CL, Ouwerkerk W, Teng TK, Voors AA, et al. Left atrial structure and function in heart failure with reduced (HFrEF) versus preserved ejection fraction (HFpEF): systematic review and meta-analysis. Heart Fail Rev. (2022) 27(5):1933–55. doi: 10.1007/s10741-021-10204-8
35. Hanif W, Alex L, Su Y, Shinde AV, Russo I, Li N, et al. Left atrial remodeling, hypertrophy, and fibrosis in mouse models of heart failure. Cardiovasc Pathol. (2017) 30:27–37. doi: 10.1016/j.carpath.2017.06.003
36. Hagii J, Metoki N, Saito S, Shiroto H, Sasaki S, Takahashi K, et al. Persistent or permanent atrial fibrillation is associated with severe cardioembolic stroke in patients with non-valvular atrial fibrillation. Thromb J. (2021) 19(1):22. doi: 10.1186/s12959-021-00276-9
37. Kapłon-Cieślicka A, Budnik M, Gawałko M, Peller M, Gorczyca I, Michalska A, et al. Atrial fibrillation type and renal dysfunction as important predictors of left atrial thrombus. Heart (British Cardiac Society). (2019) 105(17):1310–5. doi: 10.1136/heartjnl-2018-314492
38. Zhang W, Xiong Y, Yu L, Xiong A, Bao H, Cheng X. Meta-analysis of stroke and bleeding risk in patients with Various atrial fibrillation patterns receiving oral anticoagulants. Am J Cardiol. (2019) 123(6):922–8. doi: 10.1016/j.amjcard.2018.11.055
39. Lee DK, Shim J, Choi JI, Kim YH, Oh YW, Hwang SH. Left atrial fibrosis assessed with cardiac MRI in patients with paroxysmal and those with persistent atrial fibrillation. Radiology. (2019) 292(3):575–82. doi: 10.1148/radiol.2019182629
40. Kiedrowicz RM, Wielusinski M, Wojtarowicz A, Kazmierczak J. Left and right atrial appendage functional features as predictors for voltage-defined left atrial remodelling in patients with long-standing persistent atrial fibrillation. Heart Vessels. (2021) 36(6):853–62. doi: 10.1007/s00380-020-01752-4
41. Sanfilippo AJ, Abascal VM, Sheehan M, Oertel LB, Harrigan P, Hughes RA, et al. Atrial enlargement as a consequence of atrial fibrillation. A prospective echocardiographic study. Circulation. (1990) 82(3):792–7. doi: 10.1161/01.CIR.82.3.792
42. Hamatani Y, Ogawa H, Takabayashi K, Yamashita Y, Takagi D, Esato M, et al. Left atrial enlargement is an independent predictor of stroke and systemic embolism in patients with non-valvular atrial fibrillation. Sci Rep. (2016) 6:31042. doi: 10.1038/srep31042
43. Xue J, Lin Y, Huang W, Chen X, Li Q, Cai Z, et al. Left atrial size and risk of recurrent ischemic stroke in a Chinese population. Brain Behav. (2017) 7(5):e00702. doi: 10.1002/brb3.702
44. Wang G, Li G, Hu F, Zang M, Pu J. Predicting a decrease in left atrial appendage flow velocity using left atrial diameter and CHA(2)DS(2)-VASc score in patients with non-valvular atrial fibrillation. BMC Cardiovasc Disord. (2023) 23(1):180. doi: 10.1186/s12872-022-03033-6
Keywords: atrial fibrillation, left atrial appendage emptying velocity, predictive model, echocardiography, retrospective study
Citation: He W, Yin L, Liu Q, Zhang Y, Zhao Y, Wang L and You L (2024) Influencing factors and predictive model for left atrial appendage emptying velocity in nonvalvular AF patients. Front. Cardiovasc. Med. 11:1468379. doi: 10.3389/fcvm.2024.1468379
Received: 21 July 2024; Accepted: 3 September 2024;
Published: 19 September 2024.
Edited by:
Rui Providencia, University College London, United KingdomReviewed by:
Yuta Kikuchi, Thomas Jefferson University, United StatesDimitris Tsiachris, Athens Medical Center, Greece
Copyright: © 2024 He, Yin, Liu, Zhang, Zhao, Wang and You. This is an open-access article distributed under the terms of the Creative Commons Attribution License (CC BY). The use, distribution or reproduction in other forums is permitted, provided the original author(s) and the copyright owner(s) are credited and that the original publication in this journal is cited, in accordance with accepted academic practice. No use, distribution or reproduction is permitted which does not comply with these terms.
*Correspondence: Ling You, eW91bGluZ0BoZWJtdS5lZHUuY24=