- 1Department of Nursing, The 2nd Affiliated Hospital, Jiangxi Medical College, Nanchang University, Nanchang, China
- 2School of Nursing, Jiangxi Medical College, Nanchang University, Nanchang, China
Introduction: The morbidity and mortality rates of coronary heart disease are significant, with PCI being the primary treatment. The high incidence of ISR following PCI poses a challenge to its effectiveness. Currently, there are numerous studies on ISR risk prediction models after PCI, but the quality varies and there is still a lack of systematic evaluation and analysis.
Methods: To systematically retrieve and evaluate the risk prediction models for ISR after PCI. A comprehensive search was conducted across 9 databases from inception to March 1, 2024. The screening of literature and extraction of data were independently carried out by two investigators, utilizing the checklist for critical appraisal and data extraction for systematic reviews of prediction modeling studies (CHARMS). Additionally, the risk of bias and applicability were evaluated using the Prediction Model Risk of Bias Assessment Tool (PROBAST).
Results: A total of 17 studies with 29 models were included, with a sample size of 175–10,004 cases, and the incidence of outcome events was 5.79%–58.86%. The area under the receiver operating characteristic curve was 0.530–0.953. The top 5 predictors with high frequency were diabetes, number of diseased vessels, age, LDL-C and stent diameter. Bias risk assessment into the research of the risk of higher bias the applicability of the four study better.
Discussion: The overall risk of bias in the current ISR risk prediction model post-PCI is deemed high. Moving forward, it is imperative to enhance study design and specify the reporting process, optimize and validate the model, and enhance its performance.
1 Introduction
Coronary heart disease (CHD) poses a significant threat to human life and health as a chronic disease, with its morbidity and mortality showing an increasing trend year by year (1). Percutaneous coronary intervention (PCI) is the primary treatment for CHD (2), offering the advantages of minimal trauma and fewer complications. In-stent restenosis (ISR) following PCI refers to the narrowing of the stent lumen or 5 mm segments at both ends of the stent detected by coronary angiography, resulting in a stenosis degree ≥50% (3). The main pathophysiological mechanism involves vascular endothelial injury and neointimal hyperplasia (4). ISR is a major cause of stent failure and repeated revascularization, serving as a key limitation for PCI treatment (5) and significantly impacting postoperative efficacy. Despite the reduction in ISR occurrence with drug-eluting stents (DES), its incidence remains high at 5%–10% (6). Furthermore, there is currently no standardized clinical treatment plan for ISR (7). Therefore, risk stratification based on risk factors plays a crucial role in identifying high-risk populations for ISR after PCI early on and implementing timely intervention measures. Clinical prediction model (8), as an assessment tool, can evaluate the current health status of patients, help medical staff quantify the risk value of a patient's future disease, and also provide individualized medical evidence for patients, which is beneficial to improve medical cost efficiency. It may even affect the diagnosis and outcome (9). Combining multiple variables or features to construct a prediction model for ISR is helpful to understand the pathogenic mechanism of the combined effect of multiple risk factors. This method helps to have more accurate on the onset and progress of ISR evidence-based knowledge, also is advantageous to the medical staff to make the most benefit at the patient's clinical decision, to optimize clinical outcomes (10). Researchers both domestically and internationally have conducted studies on ISR risk factors after PCI, continuously developing or validating various risk prediction models; however, these models vary in research quality. Thus, this study aims to analyze and compare the fundamental characteristics, modeling methods, predictive variables, and methodological quality of ISR risk prediction models for patients after PCI to offer guidance for clinical medical staff when selecting or developing appropriate ISR risk prediction models after PCI.
2 Methods
In this study, the PICOTS model, as suggested by the Cochrane Prognostic Methods Group, was employed to formulate evidence-based inquiries (11). The target population (P) comprised patients experiencing restenosis after PCI. The index prediction model (I) under investigation was the ISR risk prediction model. There was no specific comparator (C) identified. The primary outcome (O) of interest was the occurrence of stent restenosis post-PCI. The model's application timeframe (T) was during angiography following PCI. The setting (S) for utilizing the model was within the domain of cardiovascular medicine. Apart from that, we use the Checklist for Critical Appraisal and Data Extraction for Systematic Reviews of Risk Prediction Models (CHARMS) by Moons et al. (12) was employed for screening literature and extracting data. Furthermore, the Prediction Model Risk of Bias Assessment Tool (PROBAST) developed by Moons et al. (13) was utilized to evaluate the risk of bias and the applicability of quality assessment for research on ISR prediction models.
2.1 Retrieval strategy
We conducted a thorough computerized search across multiple databases, including PubMed, Web of Science, Embase, Cochrane Library, CINAHL, Chinese Biomedical Literature Database (CBM), China National Knowledge Infrastructure (CNKI), Wanfang, and Weipu (VIP). The search spanned from the inception of each respective database up to March 1, 2024.
The search strategy is as follows: using medical subject headings (MeSH), subject headings, abstract and keyword combinations, Key words include: “Percutaneous Coronary Intervention/Coronary Intervention, Percutaneous/Intervention,Percutaneous Coronary/Coronary Revascularization,Percutaneous/PCI""Coronary restenosis/Restenosis, Coronary/restenosis/restenosis lesions/instent restenosis""risk assessment/predict*/prediction model/risk prediction/risk factors”. In addition, we manually searched the references of the included studies. Taking PubMed as an example, the search strategy is as follows: (((((Percutaneous Coronary Intervention[MeSH Terms]) OR (Percutaneous Coronary Intervention[Title/Abstract])) OR (Coronary Intervention*,Percutaneous[Title/Abstract])) OR (Intervention,Percutaneous Coronary*[Title/Abstract])) OR (Coronary Revascularization,Percutaneous[Title/Abstract])) OR (PCI[Title/Abstract]))))) AND ((((((Coronary restenosis[MeSH Terms]) OR (Restenosis*, Coronary[MeSH Terms])) OR (Coronary restenosis[Title/Abstract])) OR (Restenosis*, Coronary[Title/Abstract])) OR (restenosis[Title/Abstract])) OR (restenosis lesions[Title/Abstract])) OR (instent restenosis[Title/Abstract])))))) AND (((((risk assessment[MeSH Terms]) OR (risk assessment[Title/Abstract])) OR (predict*[Title/Abstract])) OR (prediction model[Title/Abstract])) OR (risk prediction[Title/Abstract])) OR (risk factors*[Title/Abstract])))))).
2.2 Study screening and inclusion and exclusion criteria
Use the Endnote software delete found in the database to retrieve all repeated study, two researchers independent screening the rest of the title and abstract, the full text of selected independent review to ensure that the report up to the standard. Study inclusion criteria were as follows: (1) Patients aged ≥18 years old with ISR after PCI; (2) The research content was the construction and/or validation of ISR risk prediction model after PCI; (3) Study types were cross-sectional study, case-control study or cohort study; (4) Chinese and English studies. Exclusion criteria: (1) The model included less than 2 predictors; (2) Unable to extract complete data; (3) unable to get the full text; (4) Repeated publication; (5) The specific process of modeling was not described. Study screening and data extraction were cross-checked by two researchers independently, and a third party was consulted in case of disagreement. See Figure 1 for detail.
2.3 Data extraction
Data from the included studies were independently extracted by two investigators and subsequently cross-checked by a third investigator. Any discrepancies or inconsistencies were resolved through discussion among the investigators or by seeking professional consultation. Utilizing a standardized data collection form, CHARMS data were extracted from the included studies. The form comprises the following details: Year of publication, country of study, study design type, research object, study site, and outcome definition. Additionally, it includes information on the number of candidate variables, continuous variable processing method, sample size, proportion of restenosis events and other information.
2.4 Risk of bias in the studies
Two researchers independently utilized the Prediction Model Risk of Bias Assessment Tool (PROBAST) (13) to assess the risk of bias and applicability of the included studies. In instances of disagreement, a third party was consulted for resolution. The risk of bias assessment encompassed four aspects: subjects, predictors, outcomes, and data analysis, with a total of 20 questions. Applicability was evaluated across three dimensions: subjects, predictors, and outcomes. Each question could answer “Yes (Y)/Probably Yes (PY),” “Probably Not (PN)/No (N),” or “No Information (NI).” Each domain and overall will be assessed as “high risk of bias,” “low risk of bias,” or “unclear risk of bias.” Applicability is rated as “good applicability,” “poor applicability,” or “unclear applicability.” When the evaluation results of all domains are low risk of bias or good applicability, the overall risk of bias and applicability are judged to be low risk of bias or good applicability.
2.5 Statistical analysis
Throughout the study, descriptive statistics and narrative reviews were utilized for organizing and synthesizing research findings (14), while thematic analysis (15) was employed for categorizing influencing factors.
3 Results
3.1 Data search and included literature
A total of 10,422 relevant studies were initially retrieved, from which 17 were ultimately included following screening (16–32). This comprised 13 studies in English and 4 studies in Chinese. The literature screening process is illustrated in Figure 1.
3.2 Study characteristics
Studies were published between 2013 and 2024, with the majority originating from China (n = 12), followed by Germany (n = 2), and one each from Romania, Spain, and South Korea. Among these, three (16, 18, 21) were prospective studies, while the remaining were retrospective. A total of 29 models were included, with sample sizes ranging from 175 to 10,004, and the incidence of outcome events varying from 5.79% to 58.86%. Logistic regression methods were employed in 11 studies (16–21, 23, 25, 27–30, 32) see Table 1.
3.3 Model development and performance
Among the 29 included models, 27 (16–21, 23–25, 27–32) reported areas under the receiver operating characteristic curve (AUC) ranging from 0.460 to 0.953. Except for the 7 models constructed by Scafa-udriste et al. (20), Lin et al. (25), He et al. (28), and Kang et al. (32), the AUC values of the other models exceeded 0.7. The calibration of five models 27 (17, 24, 28, 29) was evaluated using the Hosmer-Lemeshow test. Three studies (16, 28, 31) reported missing data, with two studies (28, 31) employing specific treatment methods such as direct deletion and data imputation, respectively, while six studies (17, 18, 20, 27, 30, 32) did not report the presence or absence of missing values. Table 2 shows the performance and predictors of all models.
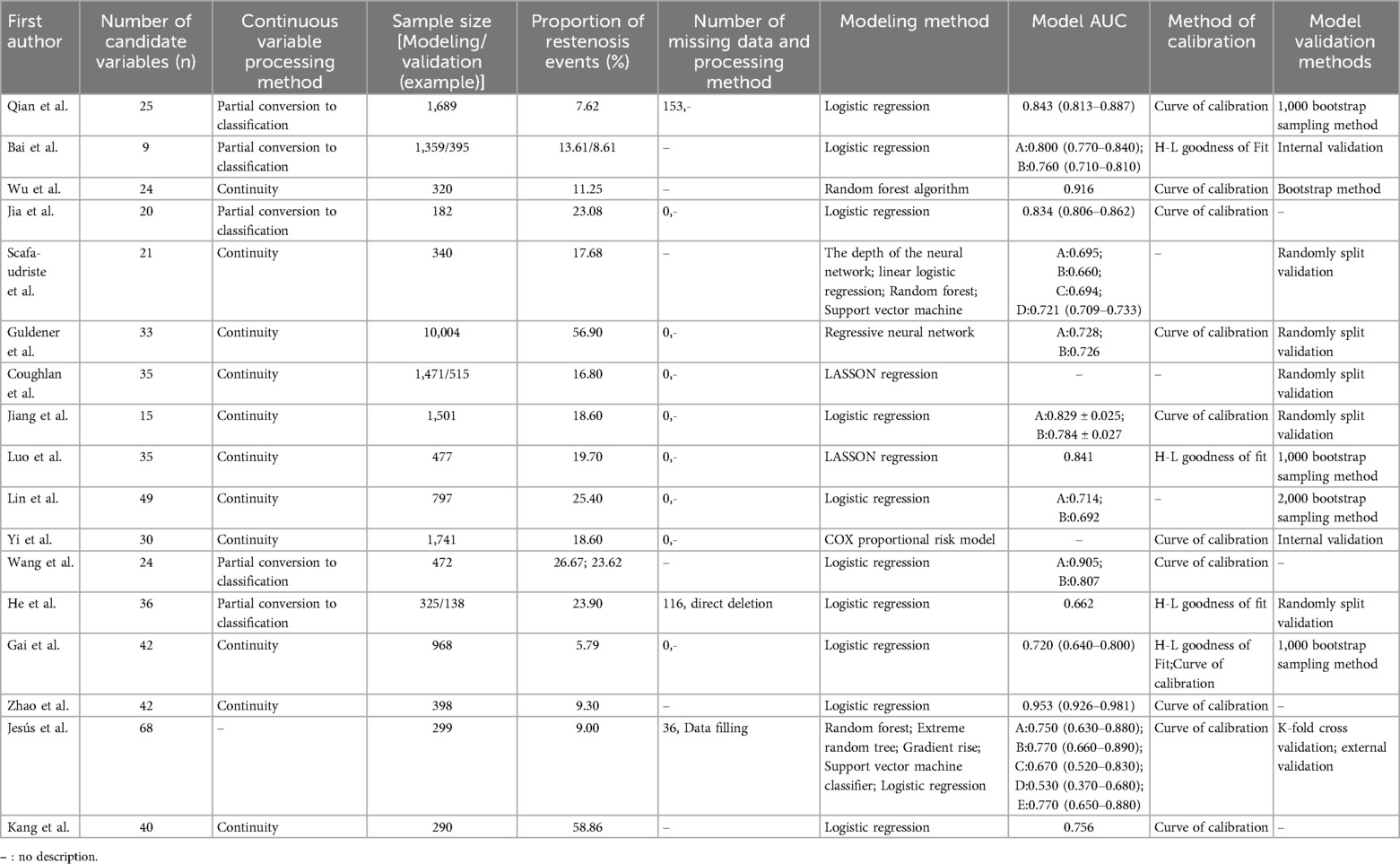
Table 2. Establishment, validation, variables and model performance of ISR risk prediction model for patients after PCI (n = 29).
3.4 Predictive variables included in the prediction model for ISR after PCI
The number of predictors included in the models ranged from 3 to 19. According to the thematic analysis method, the risk factors involved in the included studies were classified and described, which could be divided into three categories: disease and treatment factors, laboratory indicators, and demographic characteristics. The top 5 predictive variables consistently reported by the models were diabetes mellitus (n = 8), number of diseased vessels (n = 6), age (n = 6), LDL-C (n = 5), and stent diameter (n = 5). see Tables 3, 4 for more details.
3.5 Quality assessment
Among the 17 included studies, 15 (16–25, 27, 28, 30–32) were deemed to have a high risk of bias, while 2 (26, 29) could not be definitively assessed for bias. Concerning study subjects, nine studies (16, 20–25, 28, 32) were rated as high risk, seven studies (17–19, 26, 27, 29, 30) as low risk, and one study (31) as unclear risk. Regarding predictors, four studies (18–20, 25) were judged to have a high risk of bias, ten studies (17, 21, 22, 26–32) as low risk, and three studies (16, 23, 24) as unclear. Concerning outcomes, seven studies (16, 18, 19, 25, 30–32) were deemed to have a high risk of bias, four studies (24, 26, 27, 29) as unclear, and the remainder as low risk. For data analysis, five studies (17, 27, 28, 31) were rated as high risk, six studies (16, 18, 19, 21, 22, 29, 32) had unclear risk, and the rest were deemed low risk. Four studies (21, 23, 24, 30) received favorable applicability evaluations across study subjects, predictors, and outcomes, indicating high overall applicability, while the remaining studies scored low in applicability assessments. See Table 5 and Supplementary Appendix 1.
4 Discussion
4.1 Summary of main findings
We conducted a systematic review focusing on the application of clinical prediction models in studies concerning the diagnosis and prognosis prediction of in-stent restenosis (ISR) following percutaneous coronary intervention (PCI). Upon screening the 17 finally included studies, we found that the area under the receiver operating characteristic curve (AUC) of ISR prediction models after PCI ranged from 0.530 to 0.953. With the exception of four models (20, 25, 28, 31) with AUC values below 0.70, the remaining models exhibited satisfactory prediction performance (AUC > 0.70). However, it's worth noting that all 17 studies included in this review demonstrated a high risk of bias. This indicates that there's a need for future studies to enhance their study design and reporting processes, optimize and validate the models, and ultimately improve their performance.
4.2 High bias risk analysis of ISR risk prediction model after PCI
All the 17 studies included in this study showed a high risk of bias. The main reasons for this phenomenon are as follows: (1) Inadequate data size: Ten studies (18–20, 24, 25, 27, 28, 30–32) suffered from small sample sizes (sample size/candidate variables < 20), potentially predisposing the models to overfitting. (2) Inadequate handling of missing data: Among the studies included, six (17, 18, 20, 27, 30, 32) did not report any missing data, three (16, 28, 31) specified the presence of missing data, and one (16) did not detail the specific handling methods for missing data. Proper handling of missing data is crucial for reducing bias. Currently, various methods exist for addressing missing data, such as weighting methods, among others. Employing appropriate techniques tailored to the specific circumstances can mitigate the adverse effects of missing data on statistical analysis and model reliability. (3) Improvement needed in validation methods: While five studies (16, 18, 24, 25, 29) utilized the Bootstrap self-sampling method, the majority of included studies relied on internal validation methods, primarily random split validation. However, this validation approach not only diminishes the effective sample size for model development but also heightens the risk of overfitting. (4) Reliance on a single data analysis method: Eleven studies (16, 17, 19, 20, 23, 25, 27–30, 32) employed logistic regression analysis to construct models. However, contemporary approaches encompass various data analysis methods, including deep learning and decision trees, for handling complex data. Hence, alongside traditional prediction methods, integrating alternative techniques can enhance the robustness of prediction models. The initial step in prevention involves conducting risk assessment and prediction. The accuracy of predictive results will directly impact the selection and effectiveness of preventive management measures. Bias is usually defined as the presence of a systematic error that may affect the study's validity (33). A high overall bias in risk prediction models may lead to the following issues: inaccurate predictive results; suboptimal decision-making; bias in resource allocation; trust issues. Suggestions for future research involve augmenting sample sizes, enhancing the standardization of data reporting and processing, refining validation methods, and incorporating diverse data analysis methodologies. Adhering to standardized research methods and reporting processes can bolster the quality of investigations, furnishing a more scientific and reliable foundation for clinical decision-making.
4.3 High risk factors of ISR risk prediction model after PCI
Among the 17 predictors finally included in the study, spanning from 3 to 18, they can be categorized into three types. Analysis revealed that among the 22 models considered, the most prevalent predictors were diabetes mellitus, the number of diseased vessels, age, LDL-C, and stent diameter.
Diabetes is widely recognized as a risk factor for intrastent restenosis after PCI (34), and was also noted in 10 studies (16, 18, 19, 21, 23, 25, 27, 30–32) in this study. This association can be attributed to several factors. Firstly, in individuals with diabetes, chronically elevated blood glucose levels can directly or indirectly stimulate the generation of reactive oxygen species, inflammation, and metabolic factors within the body. These physiological processes can adversely affect the repair of blood vessels where stents are placed. Secondly, insulin resistance in patients with type II diabetes mellitus can easily lead to lipid deposition in the intima of blood vessels, trigger atherosclerosis and inflammatory reaction of the vessel wall, thereby stimulating the inflammatory reaction of the vessel wall, promoting the formation of thrombosis and accelerating the occurrence of ISR. In patients with abnormal lipid metabolism, lipids are more likely to be deposited in the vessel wall, thereby increasing the risk of ISR (35, 36).
The risk of ISR is high in elderly patients. Due to the relatively high number of underlying diseases, the compensatory capacity of various organs is decreased, and the repair of vascular endothelial function is impaired. In addition, the arterial wall of elderly patients is relatively thickened, the anticoagulant ability is weakened, and they are more likely to suffer from atherosclerosis (37).
Elevated levels of LDL-C can disrupt the structure and function of vascular endothelial cells, trigger inflammation, while reducing LDL-C concentration can delay the onset of ISR (34).
Stent implantation itself can cause vascular damage, and with the more and longer stents inserted, the greater vascular resistance and the greater pressure required for stent release will further damage vascular endothelial cells, leading to platelet adhesion and intimal hyperplasia, leading to ISR (34). In addition, bare metal stents can greatly increase the risk of ISR, and drug-eluting stents can inhibit endothelial cell proliferation for a long time (34).
Surgical history is a widely accepted risk factor for in-stent restenosis after PCI (38, 39). Surgery can cause different degrees of damage to the vascular wall, which leads to endothelial cell stripping, inflammatory reaction, intimal repair proliferation and smooth muscle cell proliferation. The more the number of operations, the greater the damage to the vascular wall, and inflammation and repair lead to stent restenosis.
In addition, patients with smaller vessels have a high risk of restenosis due to the fact that they are more likely to develop diseases such as diabetes or multivessel coronary artery disease, resulting from multiple factors (40).
Despite the increasing number of studies on in-stent restenosis after PCI, recognized risk factors include: the type of implanted stent, the presence of diabetes mellitus, previous bypass surgery, and small vessel caliber. However, the exact cause of in-stent restenosis after PCI is still controversial, and the risk factors are complex and diverse. Therefore, it is particularly important to carry out differential risk stratification (39) and accurately identify the predictors related to patients, stents and lesions, which is also the deficiency of existing studies.
Hence, in clinical practice, it is imperative to conduct a comprehensive assessment of individual risk factors, closely monitor these high-risk patients, and devise personalized multidisciplinary intervention programs to preempt the occurrence and progression of ISR.
4.4 Application of prediction models in clinical practice
The risk score within the prediction model relies on readily accessible clinical and laboratory data, facilitating easy calculation and implementation (41). This offers a swift and convenient means for clinics to quantify risk, aiding in the formulation of individualized patient treatments. In clinical practice, prediction models serve to unveil the risk of ISR post-PCI, and accurate models empower medical professionals to make optimal clinical decisions or guide adjunctive therapies. Hence, it is essential for them to grasp the strengths and limitations of these models.
Currently, research on ISR risk prediction models post-PCI is progressively advancing. Our study underscores that while the included ISR risk prediction models post-PCI demonstrate partial applicability, they exhibit an overall high risk of bias. This underscores the imperative for further investigations in the future, such as augmenting sample sizes and extending follow-up periods. Such endeavors are pivotal for refining the accuracy and reliability of these predictive models.
There are a variety of machine learning algorithms that can be used to establish risk prediction models in computer and medical fields, and it has been confirmed that models established by machine algorithms have better prediction performance (42, 43). Therefore, in the future, modeling methods should be used reasonably to improve model performance. To facilitate clinical practice.
Furthermore, the absence of external validation in some studies hampers the universality of prediction models. Limited clinical research exists on whether these prediction models for ISR post-PCI are conducive to early identification and intervention. These shortcomings impede the refinement of prediction models in the post-PCI population and in clinical practice. Future research endeavors should focus on enhancing the clinical utility and simplifying the prediction model for ISR post-PCI, such as refining risk calculation formulas and graphical representations.
Establishing a comprehensive clinical medical decision system through prediction models can assist clinicians in diagnosing and devising personalized treatment plans for post-PCI patients. Based on our study, we recommend that healthcare practitioners adhere strictly to PROBAST report specifications when constructing future models. Additionally, there is a need to bolster multicenter, large-sample external validation efforts and integrate clinical insights to further validate the feasibility and applicability of these models in clinical settings. Moreover, continual updates, optimization, and calibration of the model's predictive power are imperative to furnish a robust foundation for high-quality clinical decision-making.
5 Strengths and limitations
This study has several significant advantages. Firstly, it provides a comprehensive review encompassing all relevant studies on ISR prediction models post-PCI, ensuring a thorough examination of existing literature. Secondly, by employing the PROBAST tool, we meticulously assess model bias and applicability, thereby providing a robust framework for evaluating various diagnostic or prognostic prediction models.
However, we must also acknowledge some limitations. While our primary focus is on evaluating the predictive performance and bias of the included models, we did not directly assess the applicability of the clinical prediction tool in real-world clinical settings. Additionally, the formulation of inclusion and exclusion criteria, along with subjective judgments made by investigators, may lead to omissions in the reviewed literature. Finally, due to language barriers, our study is limited to published Chinese or English literature, which may introduce a certain degree of selection bias.
6 Conclusions
A total of 17 studies, encompassing 29 prediction models, were included in the analysis. The findings suggest that the current prediction models for in-stent restenosis (ISR) following PCI exhibit a high risk of bias overall, with only a minority of the models undergoing external validation. Hence, it is recommended that future research strictly adhere to the reporting steps outlined in PROBAST during model construction. Furthermore, there is a need to strengthen external validation through multi-center studies with large sample sizes. It is essential to further assess the applicability and feasibility of these models in clinical practice, considering the actual clinical context. By doing so, we can furnish a high-quality foundation for clinical decision-making.
Data availability statement
The original contributions presented in the study are included in the article/Supplementary Material, further inquiries can be directed to the corresponding author.
Author contributions
QX: Writing – review & editing, Writing – original draft, Resources, Methodology, Conceptualization. XY-X: Writing – review & editing, Supervision, Funding acquisition. SL: Writing – original draft, Methodology, Conceptualization. MJ-Z: Writing – review & editing. YJ-L: Writing – review & editing, Software, Methodology. HW-W: Writing – review & editing, Resources, Methodology. RW: Writing – review & editing, Software, Resources. LC: Writing – review & editing, Software, Resources, Conceptualization.
Funding
The author(s) declare financial support was received for the research, authorship, and/or publication of this article. This study was supported by the National Natural Science Foundation of China (No.72064028) and the Scientific Research Project approved by the Chinese Nursing Association in 2023 (ZHKY202321).
Acknowledgments
We would like to thank all the authors and editorial board for their help.
Conflict of interest
The authors declare that the research was conducted in the absence of any commercial or financial relationships that could be construed as a potential conflict of interest.
Publisher's note
All claims expressed in this article are solely those of the authors and do not necessarily represent those of their affiliated organizations, or those of the publisher, the editors and the reviewers. Any product that may be evaluated in this article, or claim that may be made by its manufacturer, is not guaranteed or endorsed by the publisher.
Supplementary material
The Supplementary Material for this article can be found online at: https://www.frontiersin.org/articles/10.3389/fcvm.2024.1445076/full#supplementary-material
References
1. Myers J, Fonda H, Vasanawala M, Chung K, Segall G, Chan K, et al. PCI alternative using sustained exercise (PAUSE): rationale and trial design. Contemp Clin Trials. (2019) 79:37–43. doi: 10.1016/j.cct.2019.02.010
2. Demyanets S, Tentzeris I, Jarai R, Katsaros K-M, Farhan S, Wonnerth A, et al. An increase of interleukin-33 serum levels after coronary stent implantation is associated with coronary in-stent restenosis. Cytokine. (2014) 2:65–70. doi: 10.1016/j.cyto.2014.02.014
3. Zhang Q, Li T, Chen R, Zeng Z-H. Research progress of SGLT2i in the prevention of in-stent restenosis after percutaneous coronary intervention. J Pract Med. (2024) 40(08):1175–80. doi: 10.3969/j.issn.1006-5725.2024.08.027
4. Yang S-M, Li S-C, Geng C-Q, Gao F. Research progress of in-stent restenosis after percutaneous coronary intervention. Combine Trad Chin West Med Jo Cardio Cerebrovasc Dis. (2023) 21(20):3754–60. doi: 10.12102/j.issn.1672-1349.2023.20.013
5. Wang F, Li C, Ding F-H, Shen Y, Gao J, Liu Z-H, et al. Increased serum TREM-1 level is associated with in-stent restenosis, and activation of TREM-1 promotes inflammation, proliferation and migration in vascular smooth muscle cells. Atherosclerosis. (2017):26710–18. doi: 10.1016/j.atherosclerosis.2017.10.015
6. Erdogan E, Bajaj R, Lansky A, Mathur A, Baumbach A, Bourantas C-V. Intravascular imaging for guiding in-stent restenosis and stent thrombosis therapy. J Am Heart Assoc. (2022) 11(22):e26492. doi: 10.1161/JAHA.122.026492
7. Ma W-L, Liu Q-F, Bao F-M, Cai Y, Zhang F-M. The application value of NLR, MHR and their combination in the diagnosis of in-stent restenosis after percutaneous coronary intervention in patients with coronary heart disease. Guangxi Med. (2021) 43(8):913–6. doi: 10.11675/j.issn.0253-4304.2021.08.02
8. Meehan A-J, Lewis S-J, Fazel S, Fusar-Poli P, Steyerberg E-W, Stahl D, et al. Clinical prediction models in psychiatry: a systematic review of two decades of progress and challenges. Mol Psychiatry. (2022) 27(6):2700–8. doi: 10.1038/s41380-022-01528-4
9. Mvs A, Jbr A, Rdr B, Gscc D, Kgm A. Clinical prediction models: diagnosis versus prognosis. J Clin Epidemiol. (2021) 132:142–5. doi: 10.1016/j.jclinepi.2021.01.009
10. Collins G-S, Reitsma J-B, Altman D-G, Moons K-G. Transparent reporting of a multivariable prediction model for individual prognosis or diagnosis (TRIPOD): the TRIPOD statement. Br Med J. (2015) 350:7594. doi: 10.1136/bmj.g7594
11. Moons K-G, Hooft L, Williams K, Hayden J-A, Damen J-A-A-G, Riley R-D. Implementing systematic reviews of prognosis studies in Cochrane. Cochrane Database Syst Rev. (2018) 10(10):ED129. doi: 10.1002/14651858
12. Moons K-G, De Groot J-A, Bouwmeester W, Vergouwe Y, Mallett S, Altman D-G, et al. Critical appraisal and data extraction for systematic reviews of prediction modelling studies: the CHARMS checklist. PLoS Med. (2014) 11(10):e1001744. doi: 10.1371/journal.pmed.1001744
13. Moons K-G-M, Wolff R-F, Riley R-D, Whiting P-F, Westwood M, Collins G-S, et al. PROBAST: a tool to assess risk of bias and applicability of prediction model studies: explanation and elaboration. Ann Intern Med. (2019) 170(1):W1–33. doi: 10.7326/M18-1377
14. Stang A. Critical evaluation of the Newcastle-Ottawa scale for the assessment of the quality of nonrandomized studies in meta-analyses. Eur J Epidemiol. (2010) 25(9):603–5. doi: 10.1007/s10654-010-9491-z
15. Braun V, Clarke V. Using thematic analysis in psychology. Qual Res Psychol. (2006) 3(2):77–101. doi: 10.1191/1478088706qp063oa
16. Qian J, Gu S-Z, Yan Y-J, Lu Y. Prevalence of in-stent restenosis after percutaneous coronary intervention (PCI) in coronary heart disease in Hai’an and construction of risk prediction model. J Integr Tradit Chin West Med Cardiocerebral Vasc Dis. (2024) 22(03):542–7. doi: 10.12102/j.issn.1672-1349.2024.03.027
17. Bai X-L, Jiang X, Pang J, Yang Z-Z, Wei Q. To construct and validate a risk prediction model for restenosis or recurrent myocardial infarction after percutaneous coronary intervention (PCI). Mil Nurs. (2024) 41(02):11–5. doi: 10.3969/j.issn.2097-1826.2024.02.003
18. Wu S-B, Wang W-Z, Wu C. To construct a risk prediction model for in-stent restenosis (ISR) in percutaneous coronary intervention (PCI) patients based on glucose and lipid metabolism. J Integr Tradit Chin West Med Cardiocerebral Vasc Dis. (2023) 21(08):1459–64. doi: 10.12102/j.issn.1672-1349.2023.08.019
19. Jia L-L, Zhang B. Establishment and validation of a nomogram model for predicting in-stent restenosis after percutaneous coronary intervention (PCI) in hypertensive patients with coronary heart disease. Chin J Arterioscler. (2023) 31(02):148–56. doi: 10.3969/j.issn.1007-3949.2023.02.008
20. Scafa-udriste A, Itu L, Puiu A, Stoian A, Moldovan H, Popa-Fotea N-M. In-stent restenosis in acute coronary syndrome—a classic and a machine learning approach. Front Cardiovasc Med. (2023) 10:1270986. doi: 10.3389/fcvm.2023.1270986
21. Guidener U, Kessler T, Von Scheidt M, Scheidt M-V, Hawe J-S, Gerhard B, et al. Machine learning identifies new predictors on restenosis risk after coronary artery stenting in 10,004 patients with surveillance angiography. J Clin Med. (2023) 12(8):2941. doi: 10.3390/jcm12082941
22. Coughan J-J, Aytekin A, Lahu S, Scalamogna M, Wiebe J, Pinieck S, et al. Derivation and validation of the ISAR score to predict the risk of repeat percutaneous coronary intervention for recurrent drug-eluting stent restenosis. EuroIntervention. (2023) 18(16):e1328–38. doi: 10.4244/EIJ-D-22-00860
23. Jiang Z, Tian L, Liu W, Song B, Xue C, Li T-Z, et al. Random forest vs. logistic regression: predicting angiographic in-stent restenosis after second-generation drug-eluting stent implantation. PLoS One. (2022) 17(5):e268757. doi: 10.1371/journal.pone.0268757
24. Luo Y, Tan N, Zhao J, Li Y-H. A nomogram for predicting in-stent restenosis risk in patients undergoing percutaneous coronary intervention: a population-based analysis. Int J Gen Med. (2022) 15:2451–61. doi: 10.2147/IJGM.S357250
25. Lin X-L, Li Q-Y, Zhao D-H, Liu J-H, Fan Q. Serum glycated albumin is associated with in-stent restenosis in patients with acute coronary syndrome after percutaneous coronary intervention with drug-eluting stents: an observational study. Front Cardiovasc Med. (2022) 9:943185. doi: 10.3389/fcvm.2022.943185
26. Yi M, Tang W-H, Xu S, Ke X, Liu Q. Investigation into the risk factors related to in-stent restenosis in elderly patients with coronary heart disease and type 2 diabetes within 2 years after the first drug-eluting stent implantation. Front Cardiovasc Med. (2022) 9:837330. doi: 10.3389/fcvm.2022.837330
27. Wang J, Yang Y-C, Zhang L, He P-Y, Mu H. Predictors of stent restenosis in Han and Uygur patients with coronary heart disease after PCI in the Xinjiang region. Cardiol Res Pract. (2022) 2022:7845108. doi: 10.1155/2022/7845108
28. He W, Xu C, Wang X, Lei J-Y, Qiu Q-F, Hu Y-Y, et al. Development and validation of a risk prediction nomogram for in-stent restenosis in patients undergoing percutaneous coronary intervention. BMC Cardiovasc Disord. (2021) 21(1):435. doi: 10.1186/s12872-021-02255-4
29. Gai M-T, Zhu B, Chen X-C, Liu F, Xie X, Gao X-M, et al. A prediction model based on platelet parameters, lipid levels, and angiographic characteristics to predict in-stent restenosis in coronary artery disease patients implanted with drug-eluting stents. Lipids Health Dis. (2021) 20(1):118. doi: 10.1186/s12944-021-01553-2
30. Zhao J, Wang X, Wang H-Y, Zhao Y, Fu X-H. Occurrence and predictive factors of restenosis in coronary heart disease patients underwent sirolimus-eluting stent implantation. Ir J Med Sci. (2020) 189(3):907–15. doi: 10.1007/s11845-020-02176-9
31. Sampedro-Gomez J, Dorado-Diza P-I, Vicente-Palacios V, Sánchez-Puente A, Jiménez-Navarro M, Roman J-A-S, et al. Machine learning to predict stent restenosis based on daily demographic, clinical, and angiographic characteristics. Can J Cardiol. (2020) 36(10):1624–32. doi: 10.1016/j.cjca.2020.01.027
32. Kang S-J, Cho Y-R, Park G-M, Ahn J-M, Han S-B, Lee J-Y, et al. Predictors for functionally significant in-stent restenosis: an integrated analysis using coronary angiography, IVUS, and myocardial perfusion imaging. JACC Cardiovasc Imaging. (2013) 6(11):1183–90. doi: 10.1016/j.jcmg.2013.09.006
33. De-Jong Y, Ramspek C-L, Zoccali C, Jager K-J, Dekker F-W, Van-Diepen M. Appraising prediction research: a guide and meta-review on bias and applicability assessment using the prediction model risk of bias ASsessment tool (PROBAST). Nephrology (Carlton). (2021) 26(12):939–47. doi: 10.1111/nep.13913
34. Wang L-Y, Zhang L-M, Li J-N, Du T-H. Risk factors of in-stent restenosis after percutaneous coronary intervention in patients with coronary heart disease: a meta-analysis. Nurs Res. (2021) 35(10):1711–9. doi: 10.12102/j.issn.1009-6493.2021.10.003
35. Shigematsu S, Takahashi N, Hara M, Hara M, Yoshimatsu H, Saikawa T. Increased incidence of coronary in-stent restenosis in type 2 diabetic patients is related to elevated serum malondialdehyde-modified low-density lipoprotein. Circ J. (2007) 71(11):1697–702. doi: 10.1253/circj.71.1697
36. Chai D, Yang X, Wang A, Lu S, Dai Y-X, Zhou J. Usefulness of platelet distribution width and fibrinogen in predicting in-stent restenosis with stable angina and type 2 patients with diabetes Mellitus. Front Cardiovasc Med. (2022) 9:710804. doi: 10.3389/fcvm.2022.710804
37. Zheng J-F, Guo T-T, Wang Y, Hu X-Y, Chang Y, Tian Y, et al. Short-term outcomes and prognostic risk factors of late drug-eluting stent restenosis patients undergoing repeated percutaneous coronary intervention. Chin Circ J. (2020) 35(4):349–54. doi: 10.3969/j.issn1000-3614.2020.04.006
38. Cassese S, Byrne R-A, Tada T, Pinieck S, Joner M, Ibrahim T, et al. Incidence and predictors of restenosis after coronary stenting in 10 004 patients with surveillance angiography. Heart. (2014) 100(2):153–9. doi: 10.1136/heartjnl-2013-304933
39. Ullrich H, Olschewski M, Münzel T, Gori T. Coronary in-stent restenosis: predictors and treatment. Dtsch Arztebl Int. (2021) 118(38):637–44. doi: 10.3238/arztebl.m2021.0254
40. Elezi S, Dibra A, Mehilli J, Pache J, Wessely R, Schömig A, et al. Vessel size and outcome after coronary drug-eluting stent placement: results from a large cohort of patients treated with sirolimus- or paclitaxel-eluting stents. J Am Coll Cardiol. (2006) 48(7):1304–9. doi: 10.1016/j.jacc.2006.05.068
41. Gan T, Guan H, Li P, Huang X, Li Y, Zhang R, et al. Risk prediction models for cardiovascular events in hemodialysis patients: a systematic review. Semin Dial. (2024) 37(2):101–9. doi: 10.1111/sdi.13181
42. Akazawa M, Hashimoto K, Katsuhiko N, Kaname N. Machine learning approach for the prediction of postpartum hemorrhage in vaginal birth. Sci Rep. (2021) 1:22620. doi: 10.1038/s41598-021-02198-y
Keywords: coronary heart disease, PCI, restenosis, prediction model, systematic review
Citation: Xiang Q, Xiong X-Y, Liu S, Zhang M-J, Li Y-J, Wang H-W, Wu R and Chen L (2024) Risk prediction model for in-stent restenosis following PCI: a systematic review. Front. Cardiovasc. Med. 11:1445076. doi: 10.3389/fcvm.2024.1445076
Received: 11 June 2024; Accepted: 19 August 2024;
Published: 29 August 2024.
Edited by:
Rajeev Gupta, Spectrum Medical Center, United Arab EmiratesReviewed by:
Mohammed Najeeb Osman, University Hospitals Cleveland Medical Center, United StatesVito Maurizio Parato, Marche Polytechnic University, Italy
Copyright: © 2024 Xiang, Xiong, Liu, Zhang, Li, Wang, Wu and Chen. This is an open-access article distributed under the terms of the Creative Commons Attribution License (CC BY). The use, distribution or reproduction in other forums is permitted, provided the original author(s) and the copyright owner(s) are credited and that the original publication in this journal is cited, in accordance with accepted academic practice. No use, distribution or reproduction is permitted which does not comply with these terms.
*Correspondence: Xiao-Yun Xiong, xxy6692@163.com