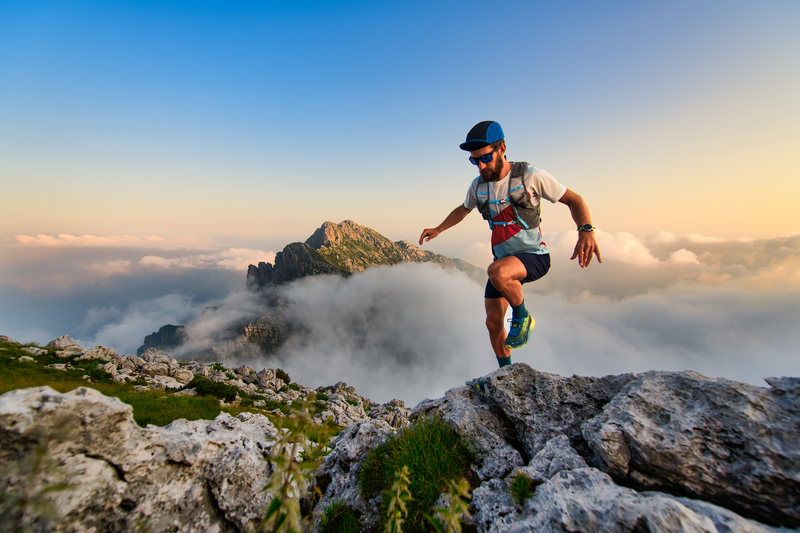
94% of researchers rate our articles as excellent or good
Learn more about the work of our research integrity team to safeguard the quality of each article we publish.
Find out more
ORIGINAL RESEARCH article
Front. Cardiovasc. Med. , 19 December 2024
Sec. Lipids in Cardiovascular Disease
Volume 11 - 2024 | https://doi.org/10.3389/fcvm.2024.1433884
Background: The association between obstructive sleep apnea (OSA) and plasma lipid concentrations is not consistent. This study aimed to investigate the association of plasma lipid concentrations with the prevalence of OSA among US adults, with an additional examination of the mediating effect of body mass index (BMI).
Methods: This cross-sectional study included 8,086 individuals who participated in the National Health and Nutrition Examination Survey (NHANES), conducted from 2005 to 2008 and 2015–2018. Multivariable logistic regression analysis was conducted to compute the odds ratios (ORs) and 95% confidence intervals (CIs) for the association between plasma lipid concentrations and the prevalence of OSA. Additionally, subgroup analysis was used to explore the potential interactions. Generalized additive models (GAM) were constructed to evaluate the nonlinear relationships between lipid concentrations and OSA. Furthermore, mediation analysis was performed to assess the potential mediating role of BMI.
Results: In the fully adjusted model, when comparing the lowest quartile, the ORs for the prevalence of OSA among participants in the highest quartile were 1.367 (95% CI, 1.107–1.688) for triglyceride and 1.212 (95% CI, 1.004–1.462) for low-density lipoprotein cholesterol (LDL-C). However, total cholesterol (TC) and high-density lipoprotein cholesterol (HDL-C) were not associated with OSA. Notably, the relationship between triglyceride and OSA differed in the subgroups of gender, race, and body mass index (BMI) (P for interaction <0.05). Furthermore, we discovered an inverted U-shaped association between triglyceride and OSA (inflection point: 0.813 mmol/L). Causal mediation analysis revealed that BMI significantly mediated the relationship between triglyceride and the prevalence of OSA.
Conclusions: This study revealed that an elevated level of triglyceride increased the prevalence of OSA, and this effect was potentially mediated through BMI. Lowering triglyceride concentration may help to reduce the prevalence of OSA.
Obstructive sleep apnea (OSA) is a chronic intermittent sleep disorder characterized by recurrent apneas, sleep disruptions, and intermittent hypoxia (1). Although OSA is a breathing disorder that occurs during sleep, the relevant pathophysiological consequences of recurrent apnea tend not to regress upon awakening (2). The episode of apnea usually persists for a minute or less. When it remains stable night after night for years, there can be long-term consequences for patients with OSA. OSA leads to increased oxidative stress, sympathetic activity, and chronic low-grade inflammation that contribute to metabolic alterations such as dyslipidemia (3, 4), hypertension (5), diabetes (6), and cardiovascular diseases (7). Recent evidence suggests that individuals suffering from OSA are at an elevated risk for developing cardiovascular diseases (CVDs). A robust investigation indicates that nocturnal oxygen desaturation significantly contributes to the development of cardiovascular complications associated with OSA (8). This association is consequently related to higher mortality rates observed in patients with OSA (9).
Patients with OSA frequently suffer from dyslipidemia (10). An animal study showed that the alterations in lipid metabolism triggered by hypoxia were facilitated through the action of hepatic stearoyl coenzyme A desaturase 1 (11). This enzyme plays a crucial role in the biosynthesis of cholesterol esters and triglycerides, contributing to dyslipidemia by enhancing the secretion of lipoproteins (12). Another cohort study described the association of severe OSA with increased triglyceride concentrations and decreased high-density lipoprotein cholesterol (HDL-C) concentrations (13). Intermittent hypoxemia may disrupt lipid metabolism by enhancing the breakdown of adipose tissue, leading to an increased flow of free fatty acids to the liver. This process occurs through the upregulation of hepatic triglyceride biosynthesis and the secretion of lipoproteins, while simultaneously suppressing the clearance of these lipoproteins from the bloodstream (14). However, other systematic reviews have provided uncertain evidence to support a direct association between lipid concentration and OSA (15, 16).
The causal relationship between lipid profiles and OSA requires further investigation due to several challenges. One significant issue is the potential confounding by other factors. Obesity has been established as a factor that affects lipid profiles (17), and it is also acknowledged as a significant contributor to OSA (18). This dual association complicates the understanding of whether the observed relationship between lipid profiles and OSA is indeed causal or if it comes from their shared connection with obesity. Thus, unravelling the interrelated factor is essential for clarifying the nature of the relationship between lipid profiles and OSA. Limited research has been undertaken regarding this relationship, and its nature remains to be fully clarified. Therefore, this research endeavors to address a significant gap in the current understanding of the interplay between OSA and lipid metabolism and obesity. By elucidating the lipidomic changes associated with OSA, it is anticipated that the study will provide valuable insights that can enhance diagnostic and therapeutic strategies, ultimately improving outcomes for individuals affected by this prevalent sleep disorder.
NHANES is a continuous, cross-sectional health and nutrition survey conducted by selecting representative non-institutionalized American residents. Data are publicly available and can be obtained at https://www.cdc.gov/nchs/nhanes. In this study, we extracted and integrated data from the NHANES 2005–2008 and 2015–2018 circles, which include a more comprehensive questionnaire about sleep disorders than any other cycles. Our initial data sample comprises 22,202 individuals aged 20 years or older. After excluding participants with missing data for plasma lipid concentrations and OSA symptoms, 8,086 participants (3,950 men and 4,136 women) were finally included in our analysis.
The definition of OSA symptoms is based on the answers to three dichotomous questions (19). These include the following: (1) Snoring three nights or more per week; (2) gasping, snorting, or stopping breathing three nights or more per week; or (3) Feeling overly sleepy during the daytime 16–30 times per month. Participants who responded yes to at least two of the three questions were considered positive for having OSA symptoms.
Serum levels of triglyceride, HDL-C, TC (Total cholesterol), and LDL-C (low-density lipoprotein cholesterol) for each specimen were measured using Roche Cobas 6,000 and Roche modular P chemistry analyzers.
According to previous findings, confounders with theoretically plausible explanations were selected as covariates. Self-reported demographic data included age, gender, race (Mexican-American, other Hispanic, non-Hispanic White, non-Hispanic Black, and other race), income, and education. The poverty income ratio (PIR) referred to the ratio of household income to poverty threshold income (20). As indicators of socioeconomic status, PIR was classified as <1.3, 1.3–1.8, and >1.8 (21). Education level was categorized as less than high school, high school or equivalent, and greater than high school (22). We classified BMI (weight/height2) into three categories: normal/underweight (BMI < 25 kg/m2), overweight (BMI between 25 and 30 kg/m2), and obese (BMI ≥ 30 kg/m2) (23). Alcohol consumption was categorized according to the National Institute on Alcohol Abuse and Alcoholism (NIAAA) criteria in the National Institute of Health, with binge drinking defined as ≥ 5 drinks/day for men or ≥4 drinks/day for women, heavy drinking defined as 3–4 drinks day for men or 2–3 drinks day for women, moderate drinking defined as 1–2 drinks/day for men or 1 drink/day for women, and none (24). Smoking status was categorized as current, prior, or never (25). Physical activity was determined by whether the participants were walking or bicycling (26). Individuals with a mean systolic blood pressure of at least 140 mmHg, a mean diastolic blood pressure of at least 90 mmHg, or current use of antihypertensive agents were defined as having hypertension (27). Cardiovascular events were defined by self-reported history of cardiovascular diseases (CVDs). The outcome was a composite of heart failure (HF), coronary heart disease (CHD), myocardial infarction (MI), angina pectoris, or stroke (28). Participants who responded with “yes” to any of the following questions were defined as CVDs: “Has a doctor or other health professional ever told you that you had a heart attack (also called myocardial infarction) coronary heart disease congestive heart failure angina (also called angina pectoris) a stroke?”. The hepatic steatosis index (HSI) was applied to assess the extent of steatosis in individuals with non-alcoholic fatty liver disease (NAFLD). The formula for calculating HSI is represented as follows: HSI = 8 × (ALT/AST) + BMI (+2 for individuals with diabetes and +2 for females). A standard threshold value of 36 for HSI is utilized for the diagnosis of NAFLD (29). Use of lipid-lowering drugs was obtained from the Drug Questionnaire. Laboratory analyses were conducted, including the assessment of alanine aminotransferase (ALT), serum albumin (ALB), blood urea nitrogen (BUN), and serum creatinine (Scr) at baseline.
Data were recorded using categorical or continuous variables. Distributed continuous variables were expressed as weighted mean ± standard deviations (mean ± SD). For categorical variables, proportions and weighted percentages with corresponding 95% confidence intervals (CIs) were computed. Categorical data were compared using the chi-square test, while continuous data were compared using Student's t-test. Multivariable logistic regression analysis was conducted to assess the association between plasma lipid concentrations and OSA. Odds ratio (OR) was used to determine the strength of the association. Multicollinearity was evaluated by calculating the variance inflation factors (VIF). Only those with VIF < 10 was included in the model. P-value of Goodness-of-fit test was >0.05, indicating that the established model fits well. The confounding factor was selected based on clinically relevance or when added it to this model, changed the matched odds ratio on the outcome measure by at least 10% or it was significantly associated with OSA. Furthermore, to ensure parsimony of the final logistic regression model, variables for inclusion were determined based on the number of events available. Model 1 was the unadjusted model. Model 2 was adjusted for demographic characteristics (age, gender, and race). Model 3 included adjustment for demographic information (age, gender, and race), individual characteristics (PIR, BMI, and level of education), health behaviors (daily alcohol consumption, physical activity status, and smoking status), comorbid conditions (history of diabetes, hypertension, CVDs, and NAFLD), laboratory tests (ALT, ALB, and Scr), and medication history (use of lipid-lowering agents). Given the significant health implications associated with plasma lipids, it is essential to investigate the various factors that affect them. Non-genetic determinants, such as socio-demographic variables and lifestyle choices—including age, gender, alcohol consumption, smoking, physical activity, BMI, comorbidities, and medication regimens—play a crucial role. Lifestyle factors such as physical activity, alcohol consumption, smoking, and BMI are modifiable and can be effectively managed to regulate lipid levels. Therefore, we reported stratified results of triglyceride, LDL-C, and HDL-C by age (<60 or ≥60 years), gender (male or female), race (non-Hispanic White or other), BMI (<30 or ≥30), smoking status (never, ever or current), alcohol consumption (nondrinker or drinker), physically active (inactive or active), hypertension (yes or no), diabetes (yes or no), NAFLD(yes or no), and use of lipid-lowering agents (yes or no). Potential interactions were performed using the likelihood ratio test. To further assess the nonlinear relationships, generalized additive models (GAM) were employed. P-values for the log-likelihood ratio test were used to compare the linear regression model with a two-piecewise linear regression model. Nonlinearity was indicated when the P-value for the log-likelihood ratio was less than 0.05. Subsequently, we determined the inflection point to develop a two-piecewise linear regression model using a recursive algorithm. The corresponding threshold level was estimated based on the maximum likelihood method. Causal mediation analyses were conducted to investigate whether BMI mediates the associations of plasma lipid concentrations with OSA. Mediation analyses could quantify the total effect (association between plasma lipid concentrations and OSA), natural direct effect (total effect without the influence of BMI), and natural indirect effect (effect of plasma lipid concentrations on OSA attributed to BMI). All statistical analyses were performed by R version 4.2.3 (R Foundation for Statistical Computing, Vienna), STATA 16.0 (Stata Corp, College Station, TX, USA), and Empower stats (http://www.empowerstats.com, X&Y Solutions, Inc, CA, USA).
Baseline characteristics of the 8,086 study participants stratified by OSA are presented in Table 1 based on weighted analyses. Compared to participants without OSA, those with OSA were generally older, predominantly male, and more likely to be obese. Additionally, they had a higher likelihood of being current smokers and had a history of conditions such as diabetes, hypertension, CVDs, and NAFLD. These individuals were also more likely to be receiving lipid-lowering treatment. Furthermore, they also had higher levels of ALT, BUN, Scr, triglyceride, TC, and LDL-C, while showing decreased levels of ALB and HDL-C.
Table 1. Weighted characteristics of participants stratified by OSA, NHANES 2005–2008 and 2015–2018.
To investigate the relationship between plasma lipid concentrations and the prevalence of OSA, three multivariable logistic regression models were constructed for analysis (Table 2). Compared to the lowest quartile in the non-adjusted model (model 1), individuals from the highest quartile of triglyceride and HDL-C had a 116% [OR, 2.162 (1.783, 2.621)] increased risk and a 55% [OR, 0.455 (0.377, 0.550)] decreased risk for the prevalence of OSA. Similar results were observed in Model 2. After adjusting for all confounding factors (model 3), compared with the lowest quartile group, the ORs with 95%CI for the prevalence of OSA were 1.277(1.037,1.572), 1.452(1.182,1.784), and 1.367(1.107,1.688) for triglyceride, and 1.180(0.976,1.426), 1.078(0.891,1.303), and 1.212(1.004,1.462) for LDL-C in the second, third, and highest quartile groups, respectively. The high levels of triglyceride and LDL-C (>75th percentile) had significant associations with the prevalence of OSA. However, TC and HDL-C were not associated with the prevalence of OSA.
We found significant interactions of triglyceride levels with gender, race, and BMI on the prevalence of OSA (Table 3). When stratified by gender (P-interaction = 0.039), female participants in the third and fourth quartile had a 52% [OR, 1.522 (1.151, 2.012)] and a 48% [OR, 1.48 (1.117, 1.962)] higher prevalence of OSA risk odds than those in the first quartile. Race also yielded a significant interaction effect (P-interaction = 0.047). Compared with the first triglyceride quartile group, the prevalence of OSA from other races in the third and fourth quartile indicated high risk odds (OR, 1.635 [1.264, 2.116], OR, 1.435 [1.095, 1.880). However, this phenomenon was not noted in non-Hispanic White individuals. In patients with BMI ≥ 30, the relationship between triglyceride and the prevalence of OSA was more prominent (P-interaction = 0.043). The fourth quartile group had a 92% higher risk odd than the first quartile group, with an OR of 1.922 (1.389, 2.660). However, no significant interactions were found between LDL-C and HDL-C and stratified variables (Supplementary Table S1).
Table 3. Associations of TG and LDL-C With the prevalence of OSA in Various subgroups, NHANES 2005–2008 and 2015–2018.
By GAM, we discovered an inverted U-shaped association between triglyceride concentration and OSA (Figure 1), and the test for non-linearity was significant (P = 0.002 for log-likelihood ratio). When the triglyceride concentration was less than the turning point (0.813 mmol/L), per unit increase in the triglyceride concentration was correlated with a 249% greater adjusted OR of developing OSA [OR, 3.493 (1.663, 7.334); Table 4]. When serum triglyceride concentration exceeded the inflection point, there was no association between the triglyceride and the prevalence of OSA [OR, 1.051 (0.955, 1.555)]. Furthermore, the LDL-C level was not associated with the prevalence of OSA when it was entered in the analysis as a continuous variable (Table 4).
Figure 1. Plots of estimated smoothing spline functions with 95% confidence band for the generalized additive model between triglyceride and the prevalence of OSA. Analyses were adjusted for: age, gender, race, education, poverty-income ratio, BMI, alcohol drinking status, smoking status, physical activity level, diabetes, hypertension, CVD, NAFLD, lipid-lowering agents, ALT, ALB, and Scr. Solid lines represent trend lines and dotted lines represent 95% confidence intervals. OSA, obstructive sleep apnea; TG, triglyceride; BMI, body mass index; CVD, cardiovascular disease; NAFLD, non-alcoholic fatty liver disease; ALT, alanine aminotransferase; ALB, albumin; Scr, Serum creatinine.
A significant mediation effect of BMI was observed in the association of triglyceride with the prevalence of OSA. The mediated proportion (%) of BMI in the relationship of triglyceride with OSA was 44% (95%CI: 33%, 63%) (Figure 2). No mediating effects of BMI were observed in HDL, LDL, and TC.
Figure 2. Mediation effect of BMI in the association of triglyceride with the prevalence of OSA. TG, triglyceride. ACME, average causal mediation effects (indirect effect); ADE, average direct effects. BMI, body mass index. *** P < 0.001.
This study examined the relationship between serum lipid concentrations and the prevalence of OSA in a large-scale U.S. general population. We discovered a significant association between triglyceride and the prevalence of OSA, as illustrated by an inverted U-shaped pattern. Significant interactions of triglyceride with gender, race, and BMI on the prevalence of OSA were also observed. The high level of LDL-C (>75th percentile) also had a significant association with the prevalence of OSA. Nevertheless, this study did not show that TC and HDL-C were correlated with OSA. Furthermore, causal mediation analysis demonstrated a significant mediation effect of BMI in the association of triglyceride with the prevalence of OSA, with the mediated proportion of 44%.
We also performed stratified analyses of the effects of serum lipid concentrations on the prevalence of OSA in several categories, and the findings showed that the results differed among groups. A significant interaction between gender and triglyceride levels was observed on the odds of developing OSA. Our study established an unexpected association between triglyceride and females, but not males. The differentiation of body composition between sexes predominantly occurs during the stages of puberty and is influenced by the effects of sex hormones (30). Generally, females exhibit a greater overall fat content in their bodies, which is associated with insulin resistance (31). An increase in body fat percentage has been linked to an elevation in plasma triglyceride levels (32). We hypothesize that elevated overall adiposity could diminish the subcutaneous adipose tissue's ability to accommodate additional fat, resulting in an increased accumulation of fat in visceral and other ectopic locations. This metabolically active visceral fat is known to secrete various inflammatory cytokines and release free fatty acids into the portal circulation, which may subsequently disrupt hepatic metabolism. This disruption could lead to diminished hepatic insulin clearance, heightened synthesis of triglyceride-rich lipoproteins, and augmented hepatic glucose production (33). Furthermore, an increase in overall adiposity also leads to a larger reserve of abdominal subcutaneous fat, which exhibits significant lipolytic activity, thereby increasing the flow of free fatty acids and further contributing to insulin resistance and the risk of cardiovascular disease (34). Studies have reported that increased triglyceride levels are associated with high CVD risks in specific subgroups such as women (35–38). Meanwhile, hypertriglyceridemia was not correlated with CVD risks in men (37). A meta-analysis of prospective studies has also suggested that for per 1 mmol/L increase in triglyceride concentrations, the risk of CVD increases by 37% in women and 12% in men regardless of other risk factors (38). OSA and CVD are closely linked, as evidenced by accumulating findings. Further investigations of gender-specific pathways that affect triglyceride are needed to understand the prevalence of OSA.
Race also yielded a significant interaction effect on triglyceride. Compared with the first triglyceride quartile group, the prevalence of OSA increased 1.635 times with the 3rd and 1.435 times with the 4th quartile group in other races. However, this phenomenon was not observed in non-Hispanic White individuals, which indicates that various races have distinct effects on OSA. Racial differences in triglyceride concentrations may be influenced by a combination of lifestyle, genetic, and cultural factors (39). It is widely recognized that Black and Hispanic Americans experience lower rates of insurance coverage and face challenges in accessing healthcare, which is particularly evident in their increased dependence on emergency departments for medical services (40). Therefore, Black and Hispanic Americans had prevalence of dyslipidemia that was comparable to that of non-Hispanic whites but were less likely to be treated and controlled (41). A previous study also showed that African Americans have a higher prevalence of OSA than white patients (42).
Women generally exhibit higher levels of HDL compared to men, which can be attributed to the effects of estrogen and testosterone on hepatic lipase activity. Hepatic lipase is crucial in HDL metabolism, and its levels are inversely related to HDL levels (43). Specifically, estrogen tends to lower hepatic lipase levels, while testosterone tends to raise them. The relationship between age and HDL levels has been demonstrated in various studies. One study indicates that older African American women tend to have higher HDL levels (44). However, in the current study, no significant interactions were observed between HDL-C and the stratified variables identified in these previous studies. This suggests that further research with larger sample sizes is necessary to better understand the mechanisms that contribute to the variations in HDL levels.
Our study revealed that elevated triglyceride was significantly associated with an increased prevalence of OSA. Furthermore, the GAM model illustrated a nonlinear pattern, with a critical threshold identified at a value of 0.813. However, HDL, LDL, and TC had no causal effects on OSA. There are several potential interpretations. Triglyceride is characterized as hydrophobic, non-polar, neutral compounds. This structural composition renders triglyceride insoluble in water, facilitating their aggregation and subsequent deposition within extracellular fluid. Elevated blood triglyceride levels lead to an accumulation of triglyceride in different areas of the body. Oral and pharyngeal triglyceride accumulations can directly narrow the airway (45, 46). Patients can compensate for narrowing of the upper airway by enhancing the activity of the upper airway muscles while they are awake. However, this protective mechanism diminishes during sleep due to muscle relaxation (45). A study conducted by Tang et al. (47) indicated that triglyceride might have a causal role in the development of OSA. This is attributed to the hydrophobic nature of triglyceride, which leads to their aggregation and accumulation in extracellular fluids. In contrast, HDL and TC possess hydrophilic polar functional groups. The hydrophilic properties of these substances make them soluble in the saline-rich environment of the body (48). As a result, even when present in high concentrations, they are less likely to deposit and accumulate in areas such as the oral cavity, pharynx, neck, and abdomen, which could potentially block the airway. Additionally, these substances do not have the capacity to form adipose tissue, a key factor that can lead to metabolic dysregulation, as seen with triglycerides. Therefore, it can be concluded that the hydrophilic nature of these compounds suggests they do not have a significant direct causal link to OSA, like triglyceride. In addition, LDL and HDL primarily function to transport TC rather than triglyceride. Variations in the levels of LDL and HDL directly affect TC levels, while having minimal impact on triglyceride. TC, which is the main lipid component of both LDL and HDL, plays a crucial role as a structural element of cell membranes, helping to maintain proper membrane permeability and fluidity (49). Additionally, TC is vital for the synthesis of steroid hormones, bile acids, and vitamin D (50). Its hydrophilic group (-OH) makes TC less prone to accumulation compared to triglyceride. Consequently, the physiological roles of HDL, TC, and LDL indicate that they are unlikely to contribute to OSA indirectly through triglyceride pathways.
The observed nonlinear relationship between triglyceride and the prevalence of OSA could be attributed to the fact that individuals without dyslipidemia might demonstrate an increased vulnerability to the accumulation of visceral fat. This accumulation of visceral fat may subsequently elevate their risk of developing OSA in comparison to individuals with dyslipidemia (51). Interestingly, Deng H et al. (51) demonstrated that among participants without dyslipidemia, parameters such as body fat percentage, waist-to-hip ratio, lipid accumulation product, and resting metabolic rate exhibited a stronger correlation with the occurrence of OSA compared to those with dyslipidemia. Another explanation is that these patients were receiving lipid-lowering treatment, which may explain the relatively low triglyceride concentrations noted in quartile 1 and quartile 2.
So far, studies conducted on the evaluation of lipid status and sleep have mainly adopted OSA and lipid abnormalities through logistic/linear regression, which might result in potential residual confounding (52, 53). By contrast, our study reported a solid non-linear association between triglyceride and OSA in a large sample of participants. A strength of our study was the application of GAM in detecting the nonlinear curved-shape correlation. Several studies have demonstrated GAM could improve the risk adjustment compared to linear models (54, 55). Our results may provide a plausible theoretical foundation for future research in OSA prevention.
The result of mediation analysis suggested that part of the effect of the triglyceride on OSA was realized through its effect on BMI. Furthermore, in the subgroup analyses regarding the association between triglyceride concentration and OSA, the strength of this relationship was found to be more pronounced in participants who were obese (P for interaction = 0.043). Obesity is a significant contributor to the development of OSA, which has been validated by numerous studies (56, 57). A review highlighted that obesity was a significant factor contributing to the increased prevalence of hypertriglyceridemia (58). Consequently, we evaluated how obesity influences the causal relationship between triglyceride levels and the susceptibility to OSA. We demonstrated that the causal impact of triglyceride on the prevalence of OSA was significant with the confounding effects of obesity. Abdominal fat accumulation, which includes both subcutaneous fat and visceral fat surrounding the organs in the abdominal cavity, significantly raises abdominal pressure. This increase in pressure can lead to a reduction in lung volume. When lung volume decreases, it may result in diminished longitudinal tracheal traction forces and reduced tension in the pharyngeal walls. These changes can predispose individuals to airway narrowing, potentially impacting respiratory function (59). Neck fat accumulation contributes to the increased collapsibility of the upper airway, which in turn reduces the effectiveness of the dilator muscles during contraction (60). This process can lead to sarcopenia, denervation, and dysfunction of skeletal muscles. Such alterations are associated with upper airway narrowing and the development of OSA. Classification of BMI may give more reliable evidence and provide novel targets for the prevention of obesity-related OSA.
As a clinical implication of our findings, physicians should pay more attention to the association between dyslipidemia and OSA. Subgroup analysis also showed that the prevalence of OSA is markedly greater among individuals who have not undergone lipid-lowering therapy when compared with those who have received such treatment. This observation indicates that lipid-lowering therapy plays a crucial role in diminishing the prevalence of OSA. Physicians can enhance the identification of individuals with elevated triglyceride levels for OSA screening, as triglyceride serves as an effective diagnostic biomarker. Additionally, clinicians can create more tailored treatment plans for patients by utilizing triglyceride levels as a basis for their therapeutic strategies.
Our study has several limitations to consider: (1) We could only identify some typical symptoms of OSA via the sleep questionnaire, such as snoring and daytime sleep. The individuals were not assessed by home or laboratory sleep testing (61), so some of the respondents with OSA might go undiagnosed. Using the STOP-Bang questionnaire may be a good supplementary method for screening suspected patients with OSA in the future (62). (2) Relying solely on data from US adults may restrict the applicability of the findings to other populations. (3) The cross-sectional design limits the ability to observe changes over time in physical indicators. Further researches are needed to elucidate the underlying mechanism of interaction on the complex balance between OSA symptoms and plasma lipid concentration.
In conclusion, this study using NHANES data shows a significant relationship between triglyceride levels and the prevalence of OSA, indicating that its potential as a predictive indicator for OSA. These association was particularly prominent in female individuals, individuals from other races, and those who were obese. Causal mediation analysis demonstrated a significant mediation effect of BMI in the association of triglyceride with OSA. Maintaining a normal or low BMI could be a valuable recommendation for decreasing the prevalence of OSA in the adult population.
The raw data supporting the conclusions of this article will be made available by the authors, without undue reservation.
XH: Writing – original draft. JX: Writing – review & editing. YG: Writing – review & editing.
The author(s) declare financial support was received for the research, authorship, and/or publication of this article. This work was supported by the National Natural Science Foundation of China (82370089).
The data used in this study were from the NHANES 2005-2008 and 2015–2018. We would like to appreciate National Center for Health Statistics of the Center for Disease Control and Prevention (CDC) for the availability of the data.
The authors declare that the research was conducted in the absence of any commercial or financial relationships that could be construed as a potential conflict of interest.
All claims expressed in this article are solely those of the authors and do not necessarily represent those of their affiliated organizations, or those of the publisher, the editors and the reviewers. Any product that may be evaluated in this article, or claim that may be made by its manufacturer, is not guaranteed or endorsed by the publisher.
The Supplementary Material for this article can be found online at: https://www.frontiersin.org/articles/10.3389/fcvm.2024.1433884/full#supplementary-material
OSA, obstructive sleep apnea; BMI, body mass index; CVD, cardiovascular disease; NAFLD, non-alcoholic fatty liver disease; ALT, alanine aminotransferase; ALB, albumin; BUN, blood urea nitrogen; TC, total cholesterol; TG, triglyceride; HDL-C, high-density lipoprotein-cholesterol; LDL-C, low-density lipoprotein-cholesterol; NHANES, National Health and Nutrition Examination Survey; OR, odds ratio; CI, confidence interval; GAM, generalized additive models.
1. Sforza E, Thomas T, Barthélémy JC, Collet P, Roche F. Obstructive sleep apnea is associated with preserved bone mineral density in healthy elderly subjects. Sleep. (2013) 36:1509–15. doi: 10.5665/sleep.3046
2. Floras JS. Sleep apnea and cardiovascular disease: an enigmatic risk factor. Circ Res. (2018) 122:1741–64. doi: 10.1161/CIRCRESAHA.118.310783
3. Kim NH, Cho NH, Yun CH, Lee SK, Yoon DW, Cho HJ, et al. Association of obstructive sleep apnea and glucose metabolism in subjects with or without obesity. Diabetes Care. (2013) 36:3909–15. doi: 10.2337/dc13-0375
4. Kohler M, Stradling JR. Mechanisms of vascular damage in obstructive sleep apnea. Nat Rev Cardiol. (2010) 7:677–85. doi: 10.1038/nrcardio.2010.145
5. Brown J, Yazdi F, Jodari-Karimi M, Owen JG, Reisin E. Obstructive sleep apnea and hypertension: updates to a critical relationship. Curr Hypertens Rep. (2022) 24:173–84. doi: 10.1007/s11906-022-01181-w
6. Reutrakul S, Mokhlesi B. Obstructive sleep apnea and diabetes: a state of the art review. Chest. (2017) 152:1070–86. doi: 10.1016/j.chest.2017.05.009
7. Javaheri S, Barbe F, Campos-Rodriguez F, Dempsey JA, Khayat R, Javaheri S, et al. Sleep apnea: types, mechanisms, and clinical cardiovascular consequences. J Am Coll Cardiol. (2017) 69:841–58. doi: 10.1016/j.jacc.2016.11.069
8. Punjabi NM, Caffo BS, Goodwin JL, Gottlieb DJ, Newman AB, O'Connor GT, et al. Sleep-disordered breathing and mortality: a prospective cohort study. PLoS Med. (2009) 6:e1000132. doi: 10.1371/journal.pmed.1000132
9. Korkalainen H, Töyräs J, Nikkonen S, Leppänen T. Mortality-risk-based apnea-hypopnea index thresholds for diagnostics of obstructive sleep apnea. J Sleep Res. (2019) 28:e12855. doi: 10.1111/jsr.12855
10. Hao H, Chen Y, Xiaojuan J, Siqi Z, Hailiang C, Xiaoxing S, et al. The association between METS-IR and serum ferritin level in United States female: a cross-sectional study based on NHANES. Front Med (Lausanne). (2022) 9:925344. doi: 10.3389/fmed.2022.925344
11. Li J, Thorne LN, Punjabi NM, Sun CK, Schwartz AR, Smith PL, et al. Intermittent hypoxia induces hyperlipidemia in lean mice. Circ Res. (2005) 97:698–706. doi: 10.1161/01.RES.0000183879.60089.a9
12. Cohen P, Miyazaki M, Socci ND, Hagge-Greenberg A, Liedtke W, Soukas AA, et al. Role for stearoyl-CoA desaturase-1 in leptin-mediated weight loss. Science (New York, NY). (2002) 297:240–3. doi: 10.1126/science.1071527
13. Trzepizur W, Le Vaillant M, Meslier N, Pigeanne T, Masson P, Humeau MP, et al. Independent association between nocturnal intermittent hypoxemia and metabolic dyslipidemia. Chest. (2013) 143:1584–9. doi: 10.1378/chest.12-1652
14. Drager LF, Jun JC, Polotsky VY. Metabolic consequences of intermittent hypoxia: relevance to obstructive sleep apnea. Best Pract Res Clin Endocrinol Metab. (2010) 24:843–51. doi: 10.1016/j.beem.2010.08.011
15. Drager LF, Jun J, Polotsky VY. Obstructive sleep apnea and dyslipidemia: implications for atherosclerosis. Curr Opin Endocrinol Diabetes Obes. (2010) 17:161–5. doi: 10.1097/MED.0b013e3283373624
16. Adedayo AM, Olafiranye O, Smith D, Hill A, Zizi F, Brown C, et al. Obstructive sleep apnea and dyslipidemia: evidence and underlying mechanism. Sleep Breath. (2014) 18:13–8. doi: 10.1007/s11325-012-0760-9
17. Iyer A, Fairlie DP, Prins JB, Hammock BD, Brown L. Inflammatory lipid mediators in adipocyte function and obesity. Nat Rev Endocrinol. (2010) 6:71–82. doi: 10.1038/nrendo.2009.264
18. Kasasbeh A, Kasasbeh E, Krishnaswamy G. Potential mechanisms connecting asthma, esophageal reflux, and obesity/sleep apnea complex–a hypothetical review. Sleep Med Rev. (2007) 11:47–58. doi: 10.1016/j.smrv.2006.05.001
19. Cavallino V, Rankin E, Popescu A, Gopang M, Hale L, Meliker JR. Antimony and sleep health outcomes5 2009–2016. Sleep Health. (2022) 8:373–9. doi: 10.1016/j.sleh.2022.05.005
20. Fisher GM. The development and history of the poverty thresholds. Soc Secur Bull. (1992) 55:3–14.1300640
21. Bailey RL, Pac SG, Fulgoni VL 3rd, Reidy KC, Catalano PM. Estimation of total usual dietary intakes of pregnant women in the United States. JAMA Network Open. (2019) 2:e195967. doi: 10.1001/jamanetworkopen.2019.5967
22. Jackson CL, Hu FB, Kawachi I, Williams DR, Mukamal KJ, Rimm EB. Black-White differences in the relationship between alcohol drinking patterns and mortality among US men and women. Am J Public Health. (2015) 105(Suppl 3):S534–43. doi: 10.2105/AJPH.2015.302615
23. Ho FK, Celis-Morales C, Gray SR, Petermann-Rocha F, Lyall D, Mackay D, et al. Child maltreatment and cardiovascular disease: quantifying mediation pathways using UK biobank. BMC Med. (2020) 18:143. doi: 10.1186/s12916-020-01603-z
24. Willenbring ML, Massey SH, Gardner MB. Helping patients who drink too much: an evidence-based guide for primary care clinicians. Am Fam Physician. (2009) 80:44–50.19621845
25. Cao Z, Xu C, Yang H, Li S, Wang Y. The role of healthy lifestyle in cancer incidence and temporal transitions to cardiometabolic disease. JACC CardioOncology. (2021) 3:663–74. doi: 10.1016/j.jaccao.2021.09.016
26. Gu X, Tang D, Xuan Y, Shen Y, Lu LQ. Association between obstructive sleep apnea symptoms and gout in US population, a cross-sectional study. Sci Rep. (2023) 13:10192. doi: 10.1038/s41598-023-36755-4
27. Chobanian AV, Bakris GL, Black HR, Cushman WC, Green LA, Izzo JL Jr, et al. Seventh report of the joint national committee on prevention, detection, evaluation, and treatment of high blood pressure. Hypertension. (2003) 42:1206–52. doi: 10.1161/01.HYP.0000107251.49515.c2
28. Abdalla SM, Yu S, Galea S. Trends in cardiovascular disease prevalence by income level in the United States. JAMA Network Open. (2020) 3:e2018150. doi: 10.1001/jamanetworkopen.2020.18150
29. Lee JH, Kim D, Kim HJ, Lee CH, Yang JI, Kim W, et al. Hepatic steatosis index: a simple screening tool reflecting nonalcoholic fatty liver disease. Dig Liver Dis. (2010) 42:503–8. doi: 10.1016/j.dld.2009.08.002
30. Stevens J, Katz EG, Huxley RR. Associations between gender, age and waist circumference. Eur J Clin Nutr. (2010) 64:6–15. doi: 10.1038/ejcn.2009.101
31. Viitasalo A, Schnurr TM, Pitkänen N, Hollensted M, Nielsen TRH, Pahkala K, et al. Abdominal adiposity and cardiometabolic risk factors in children and adolescents: a Mendelian randomization analysis. Am J Clin Nutr. (2019) 110:1079–87. doi: 10.1093/ajcn/nqz187
32. Grundy SM, Adams-Huet B, Vega GL. Variable contributions of fat content and distribution to metabolic syndrome risk factors. Metab Syndr Relat Disord. (2008) 6:281–8. doi: 10.1089/met.2008.0026
33. Blüher S, Schwarz P. Metabolically healthy obesity from childhood to adulthood—does weight status alone matter? Metabolism. (2014) 63:1084–92. doi: 10.1016/j.metabol.2014.06.009
34. Patel P, Abate N. Role of subcutaneous adipose tissue in the pathogenesis of insulin resistance. J Obes. (2013) 2013:489187. doi: 10.1155/2013/489187
35. Miller M, Stone NJ, Ballantyne C, Bittner V, Criqui MH, Ginsberg HN, et al. Triglycerides and cardiovascular disease: a scientific statement from the American Heart Association. Circulation. (2011) 123:2292–333. doi: 10.1161/CIR.0b013e3182160726
36. Mazza A, Tikhonoff V, Schiavon L, Casiglia E. Triglycerides+high-density-lipoprotein-cholesterol dyslipidaemia, a coronary risk factor in elderly women: the CArdiovascular STudy in the ELderly. Intern Med J. (2005) 35:604–10. doi: 10.1111/j.1445-5994.2005.00940.x
37. Abdel-Maksoud MF, Eckel RH, Hamman RF, Hokanson JE. Risk of coronary heart disease is associated with triglycerides and high-density lipoprotein cholesterol in women and non-high-density lipoprotein cholesterol in men. J Clin Lipidol. (2012) 6:374–81. doi: 10.1016/j.jacl.2012.02.011
38. Abdel-Maksoud MF, Hokanson JE. The complex role of triglycerides in cardiovascular disease. Semin Vasc Med. (2002) 2:325–33. doi: 10.1055/s-2002-35403
39. Pu J, Romanelli R, Zhao B, Azar KM, Hastings KG, Nimbal V, et al. Dyslipidemia in special ethnic populations. Endocrinol Metab Clin N Am. (2016) 45:205–16. doi: 10.1016/j.ecl.2015.09.013
40. Blackwell DL, Lucas JW, Clarke TC. Summary health statistics for U.S. Adults: national health interview survey, 2012. Vital Health Stat 10. (2014) 260:1–161.
41. Goff DC Jr, Bertoni AG, Kramer H, Bonds D, Blumenthal RS, Tsai MY, et al. Dyslipidemia prevalence, treatment, and control in the multi-ethnic study of atherosclerosis (MESA): gender, ethnicity, and coronary artery calcium. Circulation. (2006) 113:647–56. doi: 10.1161/CIRCULATIONAHA.105.552737
42. Redline S, Tishler PV, Hans MG, Tosteson TD, Strohl KP, Spry K. Racial differences in sleep-disordered breathing in African-Americans and caucasians. Am J Respir Crit Care Med. (1997) 155:186–92. doi: 10.1164/ajrccm.155.1.9001310
43. Jansen H, Verhoeven AJ, Sijbrands EJ. Hepatic lipase: a pro- or anti-atherogenic protein? J Lipid Res. (2002) 43:1352–62. doi: 10.1194/jlr.R200008-JLR200
44. Harman JL, Griswold ME, Jeffries NO, Sumner AE, Sarpong DF, Akylbekova EL, et al. Age is positively associated with high-density lipoprotein cholesterol among African Americans in cross-sectional analysis: the Jackson heart study. J Clin Lipidol. (2011) 5:173–8. doi: 10.1016/j.jacl.2011.02.002
45. Schwab RJ, Pasirstein M, Pierson R, Mackley A, Hachadoorian R, Arens R, et al. Identification of upper airway anatomic risk factors for obstructive sleep apnea with volumetric magnetic resonance imaging. Am J Respir Crit Care Med. (2003) 168:522–30. doi: 10.1164/rccm.200208-866OC
46. Shelton KE, Woodson H, Gay S, Suratt PM. Pharyngeal fat in obstructive sleep apnea. Am Rev Respir Dis. (1993) 148:462–6. doi: 10.1164/ajrccm/148.2.462
47. Tang H, Zhou Q, Zheng F, Wu T, Tang YD, Jiang J. The causal effects of lipid profiles on sleep apnea. Front Nutr. (2022) 9:910690. doi: 10.3389/fnut.2022.910690
48. Ginsberg HN. Lipoprotein physiology. Endocrinol Metab Clin N Am. (1998) 27:503–19. doi: 10.1016/S0889-8529(05)70023-2
49. Luo J, Yang H, Song BL. Mechanisms and regulation of cholesterol homeostasis. Nat Rev Mol Cell Biol. (2020) 21:225–45. doi: 10.1038/s41580-019-0190-7
50. Schade DS, Shey L, Eaton RP. Cholesterol review: a metabolically important molecule. Endocr Pract. (2020) 26:1514–23. doi: 10.4158/ep-2020-0347
51. Deng H, Duan X, Huang J, Zheng M, Lao M, Weng F, et al. Association of adiposity with risk of obstructive sleep apnea: a population-based study. BMC Public Health. (2023) 23:1835. doi: 10.1186/s12889-023-16695-4
52. Broussard J, Brady MJ. The impact of sleep disturbances on adipocyte function and lipid metabolism. Best Pract Res Clin Endocrinol Metab. (2010) 24:763–73. doi: 10.1016/j.beem.2010.08.007
53. Gunduz C, Basoglu OK, Hedner J, Zou D, Bonsignore MR, Hein H, et al. Obstructive sleep apnoea independently predicts lipid levels: data from the European sleep apnea database. Respirology. (2018) 23:1180–9. doi: 10.1111/resp.13372
54. Benedetti A, Abrahamowicz M. Using generalized additive models to reduce residual confounding. Stat Med. (2004) 23:3781–801. doi: 10.1002/sim.2073
55. Smiley A, King D, Harezlak J, Dinh P, Bidulescu A. The association between sleep duration and lipid profiles: the NHANES 2013–2014. J Diabetes Metab Disord. (2019) 18:315–22. doi: 10.1007/s40200-019-00415-0
56. Ischander MM, Lloyd RD Jr. Severe paediatric obesity and sleep: a mutual interactive relationship!. J Sleep Res. (2021) 30:e13162. doi: 10.1111/jsr.13162
57. Shechter A. Obstructive sleep apnea and energy balance regulation: a systematic review. Sleep Med Rev. (2017) 34:59–69. doi: 10.1016/j.smrv.2016.07.001
58. Laufs U, Parhofer KG, Ginsberg HN, Hegele RA. Clinical review on triglycerides. Eur Heart J. (2020) 41:99–109c. doi: 10.1093/eurheartj/ehz785
59. Isono S. Obesity and obstructive sleep apnoea: mechanisms for increased collapsibility of the passive pharyngeal airway. Respirology. (2012) 17:32–42. doi: 10.1111/j.1440-1843.2011.02093.x
60. Lévy P, Kohler M, McNicholas WT, Barbé F, McEvoy RD, Somers VK, et al. Obstructive sleep apnoea syndrome. Nat Rev Dis Primers. (2015) 1:15015. doi: 10.1038/nrdp.2015.15
61. Patel SR. Obstructive sleep apnea. Ann Intern Med. (2019) 171:Itc81–itc96. doi: 10.7326/AITC201912030
Keywords: obstructive sleep apnea, triglycerides, HDL-C, LDL-C, BMI
Citation: Hu X, Xu J and Gu Y (2024) Body mass index mediates the association between plasma lipid concentrations and the prevalence of obstructive sleep apnea among US adults: a cross-sectional study. Front. Cardiovasc. Med. 11:1433884. doi: 10.3389/fcvm.2024.1433884
Received: 16 May 2024; Accepted: 11 December 2024;
Published: 19 December 2024.
Edited by:
Athanasia Pataka, Aristotle University of Thessaloniki, GreeceReviewed by:
Arianna Pannunzio, Sapienza University of Rome, ItalyCopyright: © 2024 Hu, Xu and Gu. This is an open-access article distributed under the terms of the Creative Commons Attribution License (CC BY). The use, distribution or reproduction in other forums is permitted, provided the original author(s) and the copyright owner(s) are credited and that the original publication in this journal is cited, in accordance with accepted academic practice. No use, distribution or reproduction is permitted which does not comply with these terms.
*Correspondence: Yang Gu, Z3V5YW5nMTAyOEAxNjMuY29t
Disclaimer: All claims expressed in this article are solely those of the authors and do not necessarily represent those of their affiliated organizations, or those of the publisher, the editors and the reviewers. Any product that may be evaluated in this article or claim that may be made by its manufacturer is not guaranteed or endorsed by the publisher.
Research integrity at Frontiers
Learn more about the work of our research integrity team to safeguard the quality of each article we publish.