- Department of Geriatrics, Tongji Hospital, Tongji Medical College, Huazhong University of Science and Technology, Wuhan, China
Subclinical cardiovascular disease (Sub-CVD) is an early stage of cardiovascular disease and is often asymptomatic. Risk factors, including hypertension, diabetes, obesity, and lifestyle, significantly affect Sub-CVD. Progress in imaging technology has facilitated the timely identification of disease phenotypes and risk categorization. The critical function of dual-energy x-ray absorptiometry (DXA) in predicting Sub-CVD was the subject of this research. Initially used to evaluate bone mineral density, DXA has now evolved into an indispensable tool for assessing body composition, which is a pivotal determinant in estimating cardiovascular risk. DXA offers precise measurements of body fat, lean muscle mass, bone density, and abdominal aortic calcification, rendering it an essential tool for Sub-CVD evaluation. This study examined the efficacy of DXA in integrating various risk factors into a comprehensive assessment and how the application of machine learning could enhance the early discovery and control of cardiovascular risks. DXA exhibits distinct advantages and constraints compared to alternative imaging modalities such as ultrasound, computed tomography, magnetic resonance imaging, and positron emission tomography. This review advocates DXA incorporation into cardiovascular health assessments, emphasizing its crucial role in the early identification and management of Sub-CVD.
1 Introduction
Subclinical cardiovascular disease (Sub-CVD) is an early stage of cardiovascular disease (CVD) that is frequently asymptomatic. It is commonly observed in patients with sedentary lifestyles, hypertension, diabetes, or obesity, which are all risk factors (1–4). The confluence of health variables and lifestyle behaviors contributes to the development of Sub-CVD. Smoking, physical inactivity, unhealthy diet, and obesity are major contributors to its pathogenesis. High cholesterol levels, specifically low-density lipoprotein cholesterol, hypertension, and poor glucose control, play key roles in developing Sub-CVD, contributing to arterial plaque formation and vascular damage (2, 5). Numerous studies have documented how these behaviors aggravate problems such as arterial stiffness, atherosclerosis, and metabolic abnormalities (1, 2, 6–8). Clinical diseases, including coronary and peripheral artery diseases, can result from Sub-CVDs. At the outset, atherosclerosis develops because of the accumulation of plaques in the arteries. The risk factors include hypertension, obesity, and hypercholesterolemia. Patients may experience heart failure, arrhythmias, valve disorders, and stroke in the later stages (7, 9). CVD represents a major public health concern on a global scale, leading to a great clinical disease burden, high mortality rates, and a substantial financial burden on healthcare systems (10). Timely diagnosis and intervention are crucial for managing and preventing risk factors from developing into clinically apparent cardiovascular conditions.
Recent studies have demonstrated that, besides conventional risk factors, body composition measures such as excessive body fat, sarcopenia, and osteoporosis significantly increase the prevalence of Sub-CVD (11–13). Systemic inflammation and atherogenic dyslipidemia are both effects of visceral fat excess (14, 15). Sarcopenia, characterized by muscle loss and decreased function, is associated with reduced physical activity and an elevated risk of cardiovascular incidents (16, 17). Osteoporosis and Sub-CVD have similar risk factors (12, 18, 19), and osteoporosis may also influence arterial calcium deposition. Furthermore, abdominal aortic calcification (AAC) serves as an indicator of advanced atherosclerosis, which may manifest before coronary artery calcification, be detected in young persons, and reflects an elevated cardiovascular risk (19–24). Mounting data underscores the necessity for further studies and integration of these factors into clinical assessments and therapeutic strategies for cardiovascular health.
Initially employed to determine bone mineral density (BMD), dual-energy x-ray absorptiometry (DXA) (25) has evolved into a valuable tool for evaluating body composition (26). The conventional use of DXA to assess bone density may indirectly indicate cardiovascular risk owing to the correlation between osteoporosis and CVD. Recent studies have highlighted a potential link between bone health and cardiovascular risks. The accuracy of DXA in determining lean muscle mass and body fat assists in detecting excessive visceral fat, a major risk factor for Sub-CVD (27–29). The efficiency of DXA in diagnosing sarcopenia by measuring lean muscle mass highlights its role in cardiovascular risk assessment, given the established link between muscle mass loss and increased cardiovascular risk (16, 30–33). Additionally, DXA can detect AAC, a significant marker for atherosclerosis, consequently predicting Sub-CVD (28, 34–37).
Typically, physical examinations, blood tests, and imaging modalities are used to diagnose Sub-CVD (10). The thorough evaluation of body fat, sarcopenia, osteoporosis, and AAC by DXA renders it a crucial tool for predicting and assessing Sub-CVD (37–40). DXA aids in the early detection and management of cardiovascular risk by providing comprehensive data on various risk factors, potentially preventing progression from subclinical to overt CVD. The multifaceted applications of DXA are expanding beyond bone health to include a wider spectrum of cardiovascular risks (Figure 1).
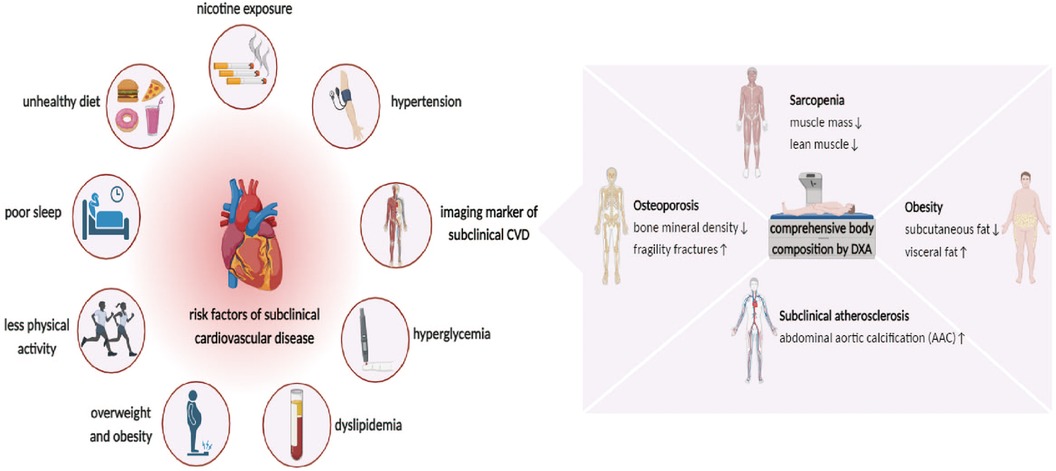
Figure 1. Risk factor for subclinical cardiovascular disease (Sub-CVD) and the prediction of dual-energy x-ray absorptiometry (DXA). The risk factor of Sub-CVD include lifestyle indicators such as unhealthy diet, nicotine exposure, poor sleep, and reduced physical activity, and clinical indicators such as hypertension, hyperglycemia, dyslipidemia, overweight, and obesity. It further highlights the utility of DXA in providing a comprehensive assessment of abdominal aortic calcification (AAC) and body composition, such as osteoporosis, sarcopenia, and obesity, which is instrumental in the early prediction and management of Sub-CVD, emphasizing its expanded role in cardiovascular risk assessment beyond bone health.
2 Risks of subclinical CVD detected by DXA
2.1 Body fat
Numerous studies have emphasized the significance of adipose tissue in maintaining cardiovascular health. Systemic inflammation, atherogenic dyslipidemia, and insulin resistance are induced by excessive body fat, particularly visceral adiposity. These well-established risk factors for CVD demonstrate a substantial association between obesity and increased risk of Sub-CVD (13, 14, 27, 41). Moreover, DXA can distinguish excess visceral fat, which is a significant risk factor for Sub-CVD (27, 28, 31). Aleksandra Radecka et al. demonstrated the potential of DXA in assessing body composition, such as in Cushing's syndrome, which is directly relevant to cardiovascular risk (32).
Obesity is characterized by excessive free fatty acids and triglycerides, leading to ectopic lipid deposition in various tissues, such as the liver, skeletal muscle, and myocardium. This deposition results in insulin resistance, hypertension, metabolic syndrome, type 2 diabetes mellitus, atherosclerosis, and CVD. Obesity-related dysfunctional visceral white adipose tissue generates oxidative stress and pro-inflammatory adipokines and activates the renin-angiotensin-aldosterone system, thereby increasing cardiovascular risk. Inflammation may be a potential molecular mechanism, and systemic inflammation is induced by excessive body fat, particularly visceral fat, by secreting pro-inflammatory cytokines, tumor necrosis factor-α (TNF-α), and interleukin-6 (IL-6) from adipose tissue. These cytokines significantly influence atherosclerosis and endothelial dysfunction, which are critical components of Sub-CVDs (14, 41, 42).
Obesity is associated with insulin resistance, which serves as a precursor to type 2 diabetes mellitus and metabolic syndrome, both of which are recognized risk factors for CVD. Increased cardiovascular risk is further exacerbated by dyslipidemia and hypertension resulting from insulin resistance. Adipose tissue actively secretes adipokines such as leptin, resistin, and adiponectin. Leptin and resistin upregulate oxidative stress and pro-inflammatory cytokine production, thereby promoting atherosclerosis and inflammation. Conversely, adiponectin has anti-inflammatory properties; however, its levels decrease with obesity. Lipotoxicity, caused by ectopic fat accumulation in non-adipose tissues, leads to endothelial dysfunction and cellular damage, significantly contributing to atherosclerosis, a precursor of subclinical CVD (43). Furthermore, obesity and type 2 diabetes mellitus orchestrate changes in substrate usage, tissue metabolism, oxidative stress, and inflammation, which collectively promote myocardial fibrosis and cardiac dysfunction (15).
The whole-body scan is performed using DXA. Discovery QDR software (version 13.5.3.2) then differentiates between fat and lean tissues to generate a comprehensive body fat distribution. DXA measures total body fat with a particular focus on visceral fat, which is closely related to cardiovascular risk.
2.2 Sarcopenia
Sarcopenia is characterized by reduced muscle mass and dysfunction, leading to decreased physical activity. Reduction in physical inactivity can potentially promote the progression of atherosclerosis and insulin resistance. Ke Gao et al. demonstrated a correlation between muscle mass loss and the rising prevalence of CVD (17). Recent research has uncovered the molecular mechanisms underlying the relationship between sarcopenia and Sub-CVD, providing evidence for this connection (16). Sarcopenia involves a reduction in muscle mass that inhibits the synthesis of nitric oxide (NO), an essential vasodilator. Decreased NO levels contribute to vascular stiffness and hypertension (44). Furthermore, muscle loss impairs glucose and lipid metabolism, exacerbating insulin resistance, a known risk factor for CVD (43, 45–47). Elevated levels of pro-inflammatory cytokines, such as C-reactive protein, IL-1, IL-6, and TNF-α, in sarcopenia lead to mitochondrial dysfunction in skeletal muscle. This dysfunction results in increased production of reactive oxygen species, which trigger the ubiquitin-proteasome cascade and amplify muscle proteolysis (48–50). Similarly, IL-6 inhibits muscle protein synthesis and activation of the Akt/mTOR pathway by inducing insulin resistance (51–54). Sarcopenia profoundly affects cardiovascular health, accelerates CVD progression, and increases the risk of mortality.
DXA determines the muscle mass, particularly in the extremities (arms and legs), which is crucial for diagnosing sarcopenia. The evaluation involves analyzing the distribution of lean muscle mass, with sarcopenia diagnosed using specific cut-off values based on established guidelines. The ability of DXA to detect sarcopenia is significantly linked to the prediction of Sub-CVD risk (30, 38).
2.3 Osteoporosis
Cohort research from the UK biobank has suggested a possible correlation between DXA-determined bone health and cardiovascular risk (55). Increased cardiovascular risk is associated with osteoporosis and decreased BMD, as reported in epidemiological studies (56). Notable correlations have also been identified between fragility fractures, which often result in osteoporosis, and later onset of cardiovascular conditions, including, but not limited to, heart failure, stroke, and ischemic heart disease. Osteoporosis and Sub-CVD share common risk factors, such as aging, diabetes mellitus, obesity, smoking, sedentary lifestyle, and advancing inflammation and hormonal changes. Additionally, the accumulation of calcium in the arterial walls highlights osteoporosis as a potential indicator of Sub-CVD (55, 57–59). This relationship could fundamentally alter our understanding of osteoporosis and cardiovascular health.
Multiple mechanisms link osteoporosis to CVD, and systemic inflammation plays a key role in both conditions. The Wnt signaling pathway is critical for the maintenance of cardiovascular homeostasis. Romosozumab, which inhibits sclerostin and is used in the treatment of osteoporosis, activates the Wnt pathway and may affect cardiovascular remodeling, potentially increasing the risk of cardiovascular events. The calcium paradox describes the inverse correlation between vascular calcification and bone density. Calcium from bone deterioration can exacerbate arterial calcification, which is a risk factor for Sub-CVD. The RANK/RANKL/OPG pathway, essential for bone remodeling, is also involved in vascular calcification (60, 61). Osteoprotegerin (OPG), a RANKL decoy receptor, inhibits bone resorption and contributes to vascular disease (62–65). Additionally, vitamin D deficiency and secondary hyperparathyroidism in osteoporosis may lead to vascular calcification and endothelial dysfunction (60, 66).
Furthermore, anti-osteoporotic medications may influence CVD. Bisphosphonates, which inhibit bone resorption, may impact lipid profiles and atherogenesis. Some anti-osteoporosis drugs could have beneficial cardiovascular effects, whereas certain vasoactive agents might positively affect bone health. The interplay between these domains requires further research (12, 18).
DXA is considered the gold standard for measuring BMD and is crucial for osteoporosis diagnosis. It typically scans the spine and hip and areas where fractures are the most common. The T- or Z-score, calculated by comparing BMD values with age- and sex-adjusted normative data, helps to identify osteoporosis or osteopenia. Early osteoporosis screening with DXA may also detect Sub-CVD in its early stages (55, 56).
2.4 AAC
Calcium deposition on the walls of the abdominal aorta is a defining feature of AAC. This process is driven by the osteogenic transformation of vascular smooth muscle cells (VSMCs) and is influenced by various factors such as inflammation, oxidative stress, and dysregulated mineral metabolism. Calcification in the aorta can reduce elasticity, cause endothelial dysfunction, impair blood flow, and increase the risk of cardiovascular events (19, 34, 35, 67). Inflammation can stimulate VSMCs within the arterial wall to undergo osteogenic differentiation, leading to calcification. Matrix vesicles, which are extracellular vesicles derived from VSMCs, play a crucial role in initiating and propagating calcification in the arterial wall (37, 68, 69).
The molecular mechanisms underlying the role of body fat, sarcopenia, osteoporosis, and AAC in the development of Sub-CVD are intricate and multifaceted. These conditions are involved in various biochemical and cellular pathways, including inflammation, metabolic dysregulation, endothelial dysfunction, and osteogenic transformation. This complexity underscores the impact of these factors on cardiovascular health. The referenced studies provide a comprehensive understanding of these mechanisms, highlighting the importance of addressing these risk factors in the prevention and treatment of Sub-CVD.
DXA can detect AAC, a marker of Sub-CVD (34, 67, 70, 71). AAC was scored on a 0–24 point scale based on baseline lateral lumbar spine x-rays. This calcification is indicative of advanced atherosclerosis and is associated with an increased risk of cardiovascular events (34, 70).
3 Comparison of DXA with other non-invasive imaging tools in predicting sub-CVD
Advances in digital imaging and biomarker technologies have greatly enhanced our ability to phenotype populations, providing detailed insights into the structural and functional dynamics of the cardiac and vascular systems. Developing modern non-invasive and invasive imaging technologies has led to significant advancements in diagnostic imaging (72, 73). This progress is crucial for early disease phenotyping, risk stratification, and management of CVD. Techniques such as carotid ultrasound, coronary cardiac computed tomography (CT), magnetic resonance imaging (MRI), and positron emission tomography (PET) are essential for detecting Sub-CVD risk factors, including early atherosclerosis and myocardial perfusion anomalies (74).
Carotid ultrasound measures intima-media thickness (IMT), a crucial early biomarker of atherosclerosis and arterial fibrosis that helps predict future CVD risk (75–78). The correlation between IMT and atherosclerotic histology makes it a reliable marker. Echocardiography, another ultrasound-based technique, is crucial for detecting and monitoring cardiac remodeling. Additionally, ultrasound also assesses body composition by measuring subcutaneous fat thickness and muscle echogenicity, which indicate skeletal muscle quality (77, 79–81). Although ultrasound may not be as comprehensive as MRI or CT for these evaluations, its non-invasive nature, cost-effectiveness, efficiency, and lack of radiation make it a valuable tool in clinical settings.
CT plays a crucial role in predicting CVD and evaluating body composition (82). The coronary artery calcium (CAC) score, obtained through CT, is an essential prognostic indicator of CVD (83). This score quantifies calcium deposition in coronary arteries and serves as a marker of arterial rigidity and potential atherosclerosis. CAC scoring is vital for CVD risk stratification (84, 85). CT angiography enhances diagnostic precision by visualizing the coronary architecture, detecting severe stenosis, and identifying subclinical coronary artery disease. In body composition studies, CT is instrumental in determining total body adipose tissue and muscle mass (86, 87). However, the use of CT is often limited due to concerns regarding radiation exposure.
MRI is invaluable for forecasting CVD and analyzing body composition (88–90). High-resolution MRI effectively assesses plaque volume and composition in the carotid arteries and abdominal aorta of patients with CVD. MRI discerns plaque components, such as the fibrous cap, necrotic core, and areas of hemorrhage or calcification. MRI excels in three-dimensional (3D) assessments of adipose tissue, muscle volume, and fat fraction across various organs when analyzing the body composition. MRI exhibits high spatial resolution and reproducibility, making it the clinical gold standard for evaluating cardiac structure and function (91, 92). The ability of MRI to identify cardiac fibrosis enhances its diagnostic capability. However, concerns about cost and accessibility have limited their widespread use.
PET is a sophisticated tool in cardiovascular diagnostics, particularly for detecting microvascular dysfunction (MVD) in patients with angina without obstructive coronary disease (93, 94). PET assesses coronary flow and myocardial perfusion reserve and provides information on epicardial stenosis and MVD. It accurately quantifies myocardial blood flow, making it invaluable for diagnosing MVD and evaluating cardiac perfusion in various cardiac pathologies. Although PET offers improved diagnostic accuracy for MVD through a comprehensive analysis of myocardial perfusion, limitations such as reduced temporal resolution and radiation exposure must be considered (95, 96).
DXA is a multifunctional imaging modality that offers a comprehensive assessment of body composition while minimizing radiation exposure. It is crucial for evaluating early-stage CVD risk factors, including osteoporosis, sarcopenia, and obesity (26, 28, 29, 31). DXA can also detect AAC, which is a precursor to coronary artery calcification and is significantly associated with CVD mortality, incident coronary heart disease, myocardial infarction, and stroke (34, 37, 97). Electron beam and multidetector CT are exceptionally precise for quantitative assessment, and the Agatston score is used to estimate the AAC. AAC is a readily available imaging marker that can be used to diagnose early stages of CVD. AAC can also be identified using MRI and transesophageal echocardiography. However, x-ray-based imaging technologies, including DXA, are most commonly used for detecting and diagnosing AAC owing to their accessibility and relatively low radiation exposure.
In conclusion, each imaging modality offers distinct advantages and disadvantages for predicting Sub-CVD. DXA has been recognized for its comprehensive assessment of body composition and minimal radiation exposure. However, CT and MRI provide a more direct visualization of cardiac structures and atherosclerosis, which can be crucial for detailed diagnostics. Ultrasound presents a balance between accessibility, safety, and functional assessment but does not offer the same level of comprehensive risk profiling as DXA (Table 1). The choice of imaging tool should be based on the specific clinical scenario, available resources, and the patient's overall risk profile.
4 Future perspectives
With advances in artificial intelligence and modern medicine, many researchers have investigated the critical function of machine learning (ML) in predicting Sub-CVD. Numerous studies have suggested that ML, particularly deep learning (DL), could improve the predictive and evaluative skills of DXA for Sub-CVD (98–101). ML models can integrate diverse datasets, including demographic, lifestyle, and disease history data, with detailed DXA data to more accurately forecast the progression of Sub-CVD. The predictive potential is critical for the timely management of Sub-CVD. By combining ML with DXA, personalized risk profiles for cardiovascular diseases can be developed. Algorithms that analyze individual DXA scans along with broader clinical data can facilitate tailored risk stratification, thereby enhancing the precision of preventive measures.
Conventional risk prediction scores are frequently employed in cardiovascular risk assessment and are supported by several national cardiovascular authorities, as well as by the World Health Organization. Commonly used scores for predicting CVD, such as the Framingham risk score, QRISK, systematic coronary risk evaluation, UK prospective diabetes study risk engine, and cardiovascular health score (2, 102–104), are combined with traditional risk factors, such as age, sex, ethnicity, socioeconomic status, family history, diet, physical activity, nicotine exposure, sleep health, body mass index, lipid profile, blood glucose, and blood pressure. Traditional scores, which are derived from statistical models based on cohort studies, employ set algorithms that do not account for the expansion of patient data. Conversely, ML operates without predetermined rules, utilizes data dynamically, and is independent of statistical assumptions, thereby enabling the discovery of complex data interactions. ML, encompassing supervised, unsupervised, and reinforcement learning, differs from traditional methods in that it focuses on predictive accuracy over hypothesis-driven inference.
ML offers diverse and powerful techniques for predicting CVDs. Supervised learning algorithms, such as neural networks and decision trees, efficiently exploit annotated data to forecast CVD by leveraging recognized risk variables. Unsupervised learning is paramount in identifying novel risk factors by unveiling hidden patterns within the data. Reinforcement learning is promising for the development of individualized treatment techniques via iterative trial-and-error methodologies (101, 105). DL, a subset of ML, excels in analyzing medical images and detecting complex indicators of Sub-CVD (106, 107). ML technology can predict and manage cardiovascular health, which is a significant advancement.
The integration of ML, particularly DL, with DXA can enhance diagnostic precision and personalize risk assessment, thereby making a substantial contribution to proactive and individualized patient care in cardiology (Figure 2).
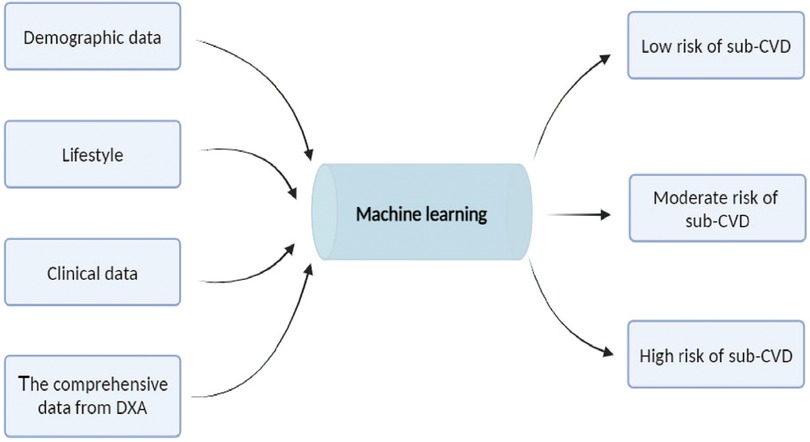
Figure 2. Machine learning model for cardiovascular risk stratification. Combining demographic data (such as age, gender, race, education, marital status and financial status), lifestyle (such as height, weight, diet, alcohol consumption, nicotine exposure, sleep, and physical activity and clinical indicators, hypertension, hyperglycemia, dyslipidemia, overweight, and obesity), clinical data (such as abdominal circumference, hip circumference, body mass index, blood pressure, glycemia, and lipidemia) and the comprehensive data from dual-energy x-ray absorptiometry (DXA) to the machine learning model. The model process these inputs to categorize individuals into three risk groups for subclinical cardiovascular disease (Sub-CVD): low, moderate, and high risk. This predictive model aims to enhance early detection and intervention strategies for Sub-CVD.
5 Conclusion
Our review highlights the distinct capacity of DXA to precisely assess body composition, particularly body fat and lean muscle mass, which are key indicators of cardiovascular health. Besides, its effectiveness in detecting osteoporosis and AAC establishes it as a vital instrument for the stratification and management of Sub-CVD.
In summary, DXA has emerged as a diagnostic tool essential in predicting cardiovascular health (11, 38, 74). This review also underscores the necessity of a multidisciplinary approach for managing Sub-CVD, advocating the use of DXA alongside other diagnostic tools and clinical evaluations. Such an integrative strategy ensures a more comprehensive cardiovascular risk assessment, fostering more effective prevention and management tactics.
Author contributions
SY: Writing – original draft, Writing – review & editing. QC: Writing – review & editing. YF: Writing – review & editing. CZ: Writing – review & editing. MC: Writing – review & editing.
Funding
The author(s) declare financial support was received for the research, authorship, and/or publication of this article. The publication fees and literature review costs for this article were supported by the Department of Science and Technology, Hubei Provincial China, under grant number 2022CFB742, with SY as the grant leader.
Conflict of interest
The authors declare that the research was conducted in the absence of any commercial or financial relationships that could be construed as a potential conflict of interest.
Publisher's note
All claims expressed in this article are solely those of the authors and do not necessarily represent those of their affiliated organizations, or those of the publisher, the editors and the reviewers. Any product that may be evaluated in this article, or claim that may be made by its manufacturer, is not guaranteed or endorsed by the publisher.
References
1. Acquah I, Hagan K, Javed Z, Taha MB, Valero-Elizondo J, Nwana N, et al. Social determinants of cardiovascular risk, subclinical cardiovascular disease, and cardiovascular events. J Am Heart Assoc. (2023) 12(6):e025581. doi: 10.1161/JAHA.122.025581
2. Lloyd-Jones DM, Allen NB, Anderson CAM, Black T, Brewer LC, Foraker RE, et al. Life’s essential 8: updating and enhancing the American Heart Association’s construct of cardiovascular health: a presidential advisory from the American Heart Association. Circulation. (2022) 146(5):e18–43. doi: 10.1161/CIR.0000000000001078
3. Enserro DM, Vasan RS, Xanthakis V. Twenty-year trends in the American Heart Association cardiovascular health score and impact on subclinical and clinical cardiovascular disease: the Framingham offspring study. J Am Heart Assoc. (2018) 7(11):e008741. doi: 10.1161/JAHA.118.008741
4. Fang M, Wang D, Tang O, McEvoy JW, Echouffo-Tcheugui JB, Christenson RH, et al. Subclinical cardiovascular disease in US adults with and without diabetes. J Am Heart Assoc. (2023) 12:e029083. doi: 10.1161/JAHA.122.029083
5. Magnussen CG, Venn A, Thomson R, Juonala M, Srinivasan SR, Viikari JSA, et al. The association of pediatric low- and high-density lipoprotein cholesterol dyslipidemia classifications and change in dyslipidemia Status with carotid intima-Media thickness in adulthood evidence from the cardiovascular risk in young Finns study, the Bogalusa heart study, and the CDAH (childhood determinants of adult health) study. J Am Coll Cardiol. (2009) 53:860–9. doi: 10.1016/j.jacc.2008.09.061
6. Bergstrom G, Persson M, Adiels M, Bjornson E, Bonander C, Ahlstrom H, et al. Prevalence of subclinical coronary artery atherosclerosis in the general population. Circulation. (2021) 144:916–29. doi: 10.1161/CIRCULATIONAHA.121.055340
7. Berry JD, Liu K, Folsom AR, Lewis CE, Carr JJ, Polak JF, et al. Prevalence and progression of subclinical atherosclerosis in younger adults with low short-term but high lifetime estimated risk for cardiovascular disease the coronary artery risk development in young adults study and multi-ethnic study of atherosclerosis. Circulation. (2009) 119:382–9. doi: 10.1161/CIRCULATIONAHA.108.800235
8. Fiuza-Luces C, Santos-Lozano A, Joyner M, Carrera-Bastos P, Picazo O, Zugaza JL, et al. Exercise benefits in cardiovascular disease: beyond attenuation of traditional risk factors. Nat Rev Cardiol. (2018) 15:731–43. doi: 10.1038/s41569-018-0065-1
9. Devesa A, Ibanez B, Malick WA, Tinuoye EO, Bustamante J, Peyra C, et al. Primary prevention of subclinical atherosclerosis in young adults: JACC review topic of the week. J Am Coll Cardiol. (2023) 82:2152–62. doi: 10.1016/j.jacc.2023.09.817
10. Ibanez B, Fernandez-Ortiz A, Fernandez-Friera L, Garcia-Lunar I, Andres V, Fuster V. Progression of early subclinical atherosclerosis (PESA) study: JACC focus seminar 7/8. J Am Coll Cardiol. (2021) 78:156–79. doi: 10.1016/j.jacc.2021.05.011
11. Alcantara JMA, Idoate F, Labayen I. Medical imaging in the assessment of cardiovascular disease risk. Curr Opin Clin Nutr Metab Care. (2023) 26:440–6. doi: 10.1097/MCO.0000000000000960
12. Asadipooya K, Weinstock A. Cardiovascular outcomes of romosozumab and protective role of alendronate. Arterioscler Thromb Vasc Biol. (2019) 39:1343–50. doi: 10.1161/ATVBAHA.119.312371
13. Lee C-D, Jacobs DR Jr., Schreiner PJ, Iribarren C, Hankinson A. Abdominal obesity and coronary artery calcification in young adults: the coronary artery risk development in young adults (CARDIA) study. Am J Clin Nutr. (2007) 86:48–54. doi: 10.1093/ajcn/86.1.48
14. Lopez-Jimenez F, Almahmeed W, Bays H, Cuevas A, Di Angelantonio E, le Roux CW, et al. Obesity and cardiovascular disease: mechanistic insights and management strategies. A joint position paper by the world heart federation and world obesity federation. Eur J Prev Cardiol. (2022) 29:2218–37. doi: 10.1093/eurjpc/zwac187
15. Piche ME, Tchernof A, Despres JP. Obesity phenotypes, diabetes, and cardiovascular diseases. Circ Res. (2020) 126:1477–500. doi: 10.1161/CIRCRESAHA.120.316101
16. Damluji AA, Alfaraidhy M, AlHajri N, Rohant NN, Kumar M, Al Malouf C, et al. Sarcopenia and cardiovascular diseases. Circulation. (2023) 147:1534–53. doi: 10.1161/CIRCULATIONAHA.123.064071
17. Gao K, Cao LF, Ma WZ, Gao YJ, Luo MS, Zhu J, et al. Association between sarcopenia and cardiovascular disease among middle-aged and older adults: findings from the China health and retirement longitudinal study. EClinicalMedicine. (2022) 44:101264. doi: 10.1016/j.eclinm.2021.101264
18. Fuggle NR, Cooper C, Harvey NC, Al-Daghri N, Brandi ML, Bruyere O, et al. Assessment of cardiovascular safety of anti-osteoporosis drugs. Drugs. (2020) 80:1537–52. doi: 10.1007/s40265-020-01364-2
19. Bartstra JW, Mali WP, Spiering W, de Jong PA. Abdominal aortic calcification: from ancient friend to modern foe. Eur J Prev Cardiol. (2021) 28:1386–91. doi: 10.1177/2047487320919895
20. Fusaro M, Schileo E, Crimi G, Aghi A, Bazzocchi A, Barbanti Brodano G, et al. A novel quantitative computer-assisted score can improve repeatability in the estimate of vascular calcifications at the abdominal aorta. Nutrients. (2022) 14(20):4276. doi: 10.3390/nu14204276
21. Jurgens PT, Carr JJ, Terry JG, Rana JS, Jacobs DR Jr., Duprez DA. Association of abdominal aorta calcium and coronary artery calcium with incident cardiovascular and coronary heart disease events in black and white middle-aged people: the coronary artery risk development in young adults study. J Am Heart Assoc. (2021) 10(24):e023037. doi: 10.1161/JAHA.121.023037
22. Gidding SS, Rana JS, Prendergast C, McGill H, Carr JJ, Liu K, et al. Pathobiological determinants of atherosclerosis in youth (PDAY) risk score in young adults predicts coronary artery and abdominal aorta calcium in middle age the CARDIA study. Circulation. (2016) 133:139–46. doi: 10.1161/CIRCULATIONAHA.115.018042
23. Leow K, Szulc P, Schousboe JT, Kiel DP, Teixeira-Pinto A, Shaikh H, et al. Prognostic value of abdominal aortic calcification: a systematic review and meta-analysis of observational studies. J Am Heart Assoc. (2021) 10(2):e017205. doi: 10.1161/JAHA.120.017205
24. Levitzky YS, Cupples LA, Murabito JM, Kannel WB, Kiel DP, Wilson PWF, et al. Prediction of intermittent claudication, ischemic stroke, and other cardiovascular disease by detection of abdominal aortic calcific deposits by plain lumbar radiographs. Am J Cardiol. (2008) 101:326–31. doi: 10.1016/j.amjcard.2007.08.032
25. Löffler MT, Sollmann N, Mei K, Valentinitsch A, Noël PB, Kirschke JS, et al. x-ray-based quantitative osteoporosis imaging at the spine. Osteoporos Int. (2019) 31:233–50. doi: 10.1007/s00198-019-05212-2
26. Gonera-Furman A, Bolanowski M, Jedrzejuk D. Osteosarcopenia-The role of dual-energy x-ray absorptiometry (DXA) in diagnostics. J Clin Med. (2022) 11(9):2522. doi: 10.3390/jcm11092522
27. Kim SR, Lee G, Choi S, Oh YH, Son JS, Park M, et al. Changes in predicted lean body mass, appendicular skeletal muscle mass, and body fat mass and cardiovascular disease. J Cachexia Sarcopenia Muscle. (2022) 13:1113–23. doi: 10.1002/jcsm.12962
28. Laddu DR, Qin F, Hedlin H, Stefanick ML, Manson JE, Zaslavsky O, et al. DXA versus clinical measures of adiposity as predictors of cardiometabolic diseases and all-cause mortality in postmenopausal women. Mayo Clin Proc. (2021) 96:2831–42. doi: 10.1016/j.mayocp.2021.04.027
29. McHugh C, Hind K, Wyse J, Davey D, Wilson F. Increases in DXA-derived visceral fat across one season in professional rugby union players: importance of visceral fat monitoring in athlete body composition assessment. J Clin Densitom. (2021) 24:206–13. doi: 10.1016/j.jocd.2020.09.001
30. Bauer J, Morley JE, Schols A, Ferrucci L, Cruz-Jentoft AJ, Dent E, et al. Sarcopenia: a time for action. An SCWD position paper. J Cachexia Sarcopenia Muscle. (2019) 10:956–61. doi: 10.1002/jcsm.12483
31. Fukuda T, Bouchi R, Takeuchi T, Tsujimoto K, Minami I, Yoshimoto T, et al. Sarcopenic obesity assessed using dual energy x-ray absorptiometry (DXA) can predict cardiovascular disease in patients with type 2 diabetes: a retrospective observational study. Cardiovasc Diabetol. (2018) 17(1):55. doi: 10.1186/s12933-018-0700-5
32. Radecka A, Lubkowska A. The significance of dual-energy x-ray absorptiometry (DXA) examination in Cushing’s syndrome—a systematic review. Diagnostics. (2023) 13(9):1576. doi: 10.3390/diagnostics13091576
33. Shin A, Choi SR, Han M, Ha YJ, Lee YJ, Lee EB, et al. Association between sarcopenia defined as low lean mass by dual-energy x-ray absorptiometry and comorbidities of rheumatoid arthritis: results of a nationwide cross-sectional health examination. Semin Arthritis Rheum. (2022) 57:152090. doi: 10.1016/j.semarthrit.2022.152090
34. Schousboe JT, Lewis JR, Kiel DP. Abdominal aortic calcification on dual-energy x-ray absorptiometry: methods of assessment and clinical significance. Bone. (2017) 104:91–100. doi: 10.1016/j.bone.2017.01.025
35. Schousboe JT, Vo TN, Langsetmo L, Adabag S, Szulc P, Lewis JR, et al. Abdominal aortic calcification (AAC) and ankle-brachial index (ABI) predict health care costs and utilization in older men, independent of prevalent clinical cardiovascular disease and each other. Atherosclerosis. (2020) 295:31–7. doi: 10.1016/j.atherosclerosis.2020.01.012
36. Sotomayor CG, Benjamens S, Gomes-Neto AW, Pol RA, Groothof D, Te Velde-Keyzer CA, et al. Bone mineral density and aortic calcification: evidence for a bone-vascular axis after kidney transplantation. Transplantation. (2021) 105:231–9. doi: 10.1097/TP.0000000000003226
37. Szulc P. Abdominal aortic calcification: a reappraisal of epidemiological and pathophysiological data. Bone. (2016) 84:25–37. doi: 10.1016/j.bone.2015.12.004
38. Al-Absi HRH, Islam MT, Refaee MA, Chowdhury MEH, Alam T. Cardiovascular disease diagnosis from DXA scan and retinal images using deep learning. Sensors (Basel). (2022) 22(12):4310. doi: 10.3390/s22124310
39. Sa C, Burgos M, Rabelo Filho LV, Calado CKM, Costa MDC, Silva TCA, et al. Body composition assessed by dual-energy x-ray absorptiometry on metabolic profile and cardiovascular risk in obese patients prior to bariatric surgery. Arq Bras Cir Dig. (2023) 36:e1734. doi: 10.1590/0102-672020230016e1734
40. Sawicki P, Talalaj M, Zycinska K, Zgliczynski WS, Wierzba W. Current applications and selected technical details of dual-energy x-ray absorptiometry. Med Sci Monit. (2021) 27:e930839. doi: 10.12659/MSM.930839
41. Valenzuela PL, Carrera-Bastos P, Castillo-Garcia A, Lieberman DE, Santos-Lozano A, Lucia A. Obesity and the risk of cardiometabolic diseases. Nat Rev Cardiol. (2023) 20:475–94. doi: 10.1038/s41569-023-00847-5
42. Mouton AJ, Li X, Hall ME, Hall JE. Obesity, hypertension, and cardiac dysfunction: novel roles of immunometabolism in macrophage activation and inflammation. Circ Res. (2020) 126:789–806. doi: 10.1161/CIRCRESAHA.119.312321
43. Kawai T, Autieri MV, Scalia R. Adipose tissue inflammation and metabolic dysfunction in obesity. Am J Physiol Cell Physiol. (2021) 320:C375–91. doi: 10.1152/ajpcell.00379.2020
44. Borlaug BA. The pathophysiology of heart failure with preserved ejection fraction. Nat Rev Cardiol. (2014) 11:507–15. doi: 10.1038/nrcardio.2014.83
45. Feng S, Zhu Y, Yan C, Wang Y, Zhang Z. Retinol binding protein 4 correlates with and is an early predictor of carotid atherosclerosis in type 2 diabetes mellitus patients. J Biomed Res. (2015) 29(6):451–5. doi: 10.7555/JBR.29.20140087
46. Koenen M, Hill MA, Cohen P, Sowers JR. Obesity, adipose tissue and vascular dysfunction. Circ Res. (2021) 128:951–68. doi: 10.1161/CIRCRESAHA.121.318093
47. Taqueti VR, Di Carli MF. Coronary microvascular disease pathogenic mechanisms and therapeutic options. JACC state-of-the-art review. J Am Coll Cardiol. (2018) 72:2625–41. doi: 10.1016/j.jacc.2018.09.042
48. Liang Z, Zhang T, Liu H, Li Z, Peng L, Wang C, et al. Inflammaging: the ground for sarcopenia? Exp Gerontol. (2022) 168:111931. doi: 10.1016/j.exger.2022.111931
49. Mendes J, Simoes CD, Martins JO, Sousa AS. Inflammatory bowel disease and sarcopenia: a focus on muscle strength—narrative review. Arq Gastroenterol. (2023) 60:373–82. doi: 10.1590/s0004-2803.230302023-45
50. Gonzalez A, Simon F, Achiardi O, Vilos C, Cabrera D, Cabello-Verrugio C. The critical role of oxidative stress in sarcopenic obesity. Oxid Med Cell Longev. (2021) 2021:4493817. doi: 10.1155/2021/4493817
51. Toda G, Soeda K, Okazaki Y, Kobayashi N, Masuda Y, Arakawa N, et al. Insulin- and lipopolysaccharide-mediated signaling in adipose tissue macrophages regulates postprandial glycemia through akt-mTOR activation. Mol Cell. (2020) 79:43–53.e4. doi: 10.1016/j.molcel.2020.04.033
52. Oh HJ, Jin H, Lee BY. Hesperidin ameliorates sarcopenia through the regulation of inflammaging and the AKT/mTOR/FoxO3a signaling pathway in 22–26-month-old mice. Cells. (2023) 12(15):2015. doi: 10.3390/cells12152015
53. Gingras AA, White PJ, Chouinard PY, Julien P, Davis TA, Dombrowski L, et al. Long-chain omega-3 fatty acids regulate bovine whole-body protein metabolism by promoting muscle insulin signalling to the akt-mTOR-S6K1 pathway and insulin sensitivity. J Physiol. (2007) 579:269–84. doi: 10.1113/jphysiol.2006.121079
54. Jimenez-Gutierrez GE, Martínez-Gómez LE, Martínez-Armenta C, Pineda C, Martínez-Nava GA, Lopez-Reyes A. Molecular mechanisms of inflammation in sarcopenia: diagnosis and therapeutic update. Cells. (2022) 11(15):2359. doi: 10.3390/cells11152359
55. Rodriguez-Gomez I, Gray SR, Ho FK, Petermann-Rocha F, Welsh P, Cleland J, et al. Osteoporosis and its association with cardiovascular disease, respiratory disease, and cancer: findings from the UK biobank prospective cohort study. Mayo Clin Proc. (2022) 97:110–21. doi: 10.1016/j.mayocp.2021.07.019
56. Pickering ME. Cross-talks between the cardiovascular disease-sarcopenia-osteoporosis triad and magnesium in humans. Int J Mol Sci. (2021) 22(16):9102. doi: 10.3390/ijms22169102
57. Reiss AB, Miyawaki N, Moon J, Kasselman LJ, Voloshyna I, D'Avino R Jr., et al. CKD, arterial calcification, atherosclerosis and bone health: inter-relationships and controversies. Atherosclerosis. (2018) 278:49–59. doi: 10.1016/j.atherosclerosis.2018.08.046
58. Lampropoulos CE, Papaioannou I, D'Cruz DP. Osteoporosis–a risk factor for cardiovascular disease? Nat Rev Rheumatol. (2012) 8:587–98. doi: 10.1038/nrrheum.2012.120
59. Polyzos SA, Anastasilakis AD, Efstathiadou ZA, Yavropoulou MP, Makras P. Postmenopausal osteoporosis coexisting with other metabolic diseases: treatment considerations. Maturitas. (2021) 147:19–25. doi: 10.1016/j.maturitas.2021.02.007
60. Kenkre JS, Bassett J. The bone remodelling cycle. Ann Clin Biochem. (2018) 55:308–27. doi: 10.1177/0004563218759371
61. Daoussis D, Andonopoulos AP, Liossis SN. Wnt pathway and IL-17: novel regulators of joint remodeling in rheumatic diseases. Looking beyond the RANK-RANKL-OPG axis. Semin Arthritis Rheum. (2010) 39:369–83. doi: 10.1016/j.semarthrit.2008.10.008
62. Fitzpatrick J, Kim ED, Sozio SM, Jaar BG, Estrella MM, Monroy-Trujillo JM, et al. Calcification biomarkers, subclinical vascular disease, and mortality among multiethnic dialysis patients. Kidney Int Rep. (2020) 5:1729–37. doi: 10.1016/j.ekir.2020.07.033
63. Van Campenhout A, Golledge J. Osteoprotegerin, vascular calcification and atherosclerosis. Atherosclerosis. (2009) 204:321–9. doi: 10.1016/j.atherosclerosis.2008.09.033
64. Rochette L, Meloux A, Rigal E, Zeller M, Cottin Y, Vergely C. The role of osteoprotegerin and its ligands in vascular function. Int J Mol Sci. (2019) 20(3):705. doi: 10.3390/ijms20030705
65. Sattler AM, Schoppet M, Schaefer JR, Hofbauer LC. Novel aspects on RANK ligand and osteoprotegerin in osteoporosis and vascular disease. Calcif Tissue Int. (2004) 74:103–6. doi: 10.1007/s00223-003-0011-y
66. Pirrotta F, Cavati G, Mingiano C, Merlotti D, Nuti R, Gennari L, et al. Vitamin D deficiency and cardiovascular mortality: retrospective analysis “siena osteoporosis” cohort. Nutrients. (2023) 15(15):3303. doi: 10.3390/nu15153303
67. Mazziotti G, Tupputi U, Ferrante G, Guglielmi G. Abdominal aortic calcification as a marker of relationship between atherosclerosis and skeletal fragility. J Clin Densitom. (2020) 23:539–42. doi: 10.1016/j.jocd.2020.05.001
68. Chen Y, Zhao X, Wu H. Arterial stiffness: a focus on vascular calcification and its link to bone mineralization. Arterioscler Thromb Vasc Biol. (2020) 40:1078–93. doi: 10.1161/ATVBAHA.120.313131
69. Kockx MM, De Meyer GRY, Muhring J, Jacob W, Bult H, Herman AG. Apoptosis and related proteins in different stages of human atherosclerotic plaques. Circulation. (1998) 97:2307–15. doi: 10.1161/01.CIR.97.23.2307
70. Elmasri K, Hicks Y, Yang X, Sun X, Pettit R, Evans W. Automatic detection and quantification of abdominal aortic calcification in dual energy x-ray absorptiometry. Procedia Comput Sci. (2016) 96:1011–21. doi: 10.1016/j.procs.2016.08.116
71. Kim E-D, Kim JS, Kim S-S, Jung J-G, Yun S-J, Kim J-Y, et al. Association of abdominal aortic calcification with lifestyle and risk factors of cardiovascular disease. Korean J Fam Med. (2013) 34:213–20. doi: 10.4082/kjfm.2013.34.3.213
72. Vemuri P, Decarli C, Duering M. Imaging markers of vascular brain health: quantification, clinical implications, and future directions. Stroke. (2022) 53:416–26. doi: 10.1161/STROKEAHA.120.032611
73. Mikail N, Rossi A, Bengs S, Haider A, Stahli BE, Portmann A, et al. Imaging of heart disease in women: review and case presentation. Eur J Nucl Med Mol Imaging. (2022) 50:130–59. doi: 10.1007/s00259-022-05914-6
74. Varadarajan V, Gidding SS, Wu C, Carr JJ, Lima JAC. Imaging early life cardiovascular phenotype. Circ Res. (2023) 132:1607–27. doi: 10.1161/CIRCRESAHA.123.322054
75. Hodis HN, Mack WJ, LaBree L, Selzer RH, Liu CR, Liu CH, et al. The role of carotid arterial intima-media thickness in predicting clinical coronary events. Ann Intern Med. (1998) 128:262. -+. doi: 10.7326/0003-4819-128-4-199802150-00002
76. Juonala M, Magnussen CG, Venn A, Dwyer T, Burns TL, Davis PH, et al. Influence of age on associations between childhood risk factors and carotid intima-Media thickness in adulthood the cardiovascular risk in young Finns study, the childhood determinants of adult health study, the Bogalusa heart study, and the Muscatine study for the international childhood cardiovascular cohort (i3C) consortium. Circulation. (2010) 122:2514–20. doi: 10.1161/CIRCULATIONAHA.110.966465
77. Lorenz MW, Markus HS, Bots ML, Rosvall M, Sitzer M. Prediction of clinical cardiovascular events with carotid intima-media thickness—a systematic review and meta-analysis. Circulation. (2007) 115:459–67. doi: 10.1161/CIRCULATIONAHA.106.628875
78. Nwabuo CC, Yano Y, Moreira HT, Appiah D, Vasconcellos HD, Aghaji QN, et al. Long-term blood pressure variability in young adulthood and coronary artery calcium and carotid intima-media thickness in midlife the CARDIA study. Hypertension. (2020) 76:404–9. doi: 10.1161/HYPERTENSIONAHA.120.15394
79. Joakimsen O, Bonaa KH, StenslandBugge E. Reproducibility of ultrasound assessment of carotid plaque occurrence, thickness, and morphology—the Tromso study. Stroke. (1997) 28:2201–7. doi: 10.1161/01.STR.28.11.2201
80. Wei K, Skyba DM, Firschke C, Jayaweera AR, Lindner JR, Kaul S. Interactions between microbubbles and ultrasound: in vitro and in vivo observations. J Am Coll Cardiol. (1997) 29:1081–8. doi: 10.1016/S0735-1097(97)00029-6
81. Velazquez F, Berna JD, Abellan JL, Serrano L, Escribano A, Canteras M. Reproducibility of sonographic measurements of carotid intima-media thickness. Acta Radiol. (2008) 49:1162–6. doi: 10.1080/02841850802438520
82. Antonopoulos AS, Angelopoulos A, Tsioufis K, Antoniades C, Tousoulis D. Cardiovascular risk stratification by coronary computed tomography angiography imaging: current state-of-the-art. Eur J Prev Cardiol. (2022) 29:608–24. doi: 10.1093/eurjpc/zwab067
83. Arbab-Zadeh A, Di Carli MF, Cerci R, George RT, Chen MY, Dewey M, et al. Accuracy of computed tomographic angiography and single-photon emission computed tomography-acquired myocardial perfusion imaging for the diagnosis of coronary artery disease. Circulation-Cardiovascular Imaging. (2015) 8(10):e003533. doi: 10.1161/CIRCIMAGING.115.003533
84. Faggiano P, Dasseni N, Gaibazzi N, Rossi A, Henein M, Pressman G. Cardiac calcification as a marker of subclinical atherosclerosis and predictor of cardiovascular events: a review of the evidence. Eur J Prev Cardiol. (2019) 26:1191–204. doi: 10.1177/2047487319830485
85. Mortensen MB, Gaur S, Frimmer A, Botker HE, Sorensen HT, Kragholm KH, et al. Association of age with the diagnostic value of coronary artery calcium score for ruling out coronary stenosis in symptomatic patients. Jama Cardiology. (2022) 7:36–44. doi: 10.1001/jamacardio.2021.4406
86. Troschel AS, Troschel FM, Best TD, Gaissert HA, Torriani M, Muniappan A, et al. Computed tomography-based body composition analysis and its role in lung cancer care. J Thorac Imaging. (2020) 35:91–100. doi: 10.1097/RTI.0000000000000428
87. Nicholson JM, Orsso CE, Nourouzpour S, Elangeswaran B, Chohan K, Orchanian-Cheff A, et al. Computed tomography-based body composition measures in COPD and their association with clinical outcomes: a systematic review. Chron Respir Dis. (2022) 19:14799731221133387. doi: 10.1177/14799731221133387
88. Cai JM, Hastukami TS, Ferguson MS, Small R, Polissar NL, Yuan C. Classification of human carotid atherosclerotic lesions with in vivo multicontrast magnetic resonance imaging. Circulation. (2002) 106:1368–73. doi: 10.1161/01.CIR.0000028591.44554.F9
89. Rider OJ, Lewandowski A, Nethononda R, Petersen SE, Francis JM, Pitcher A, et al. Gender-specific differences in left ventricular remodelling in obesity: insights from cardiovascular magnetic resonance imaging. Eur Heart J. (2013) 34:292–9. doi: 10.1093/eurheartj/ehs341
90. Wust RCI, Calcagno C, Daal MRR, Nederveen AJ, Coolen BF, Strijkers GJ. Emerging magnetic resonance imaging techniques for atherosclerosis imaging high magnetic field, relaxation time mapping, and fluorine-19 imaging. Arterioscler Thromb Vasc Biol. (2019) 39:841–9. doi: 10.1161/ATVBAHA.118.311756
91. Lemos T, Gallagher D. Current body composition measurement techniques. Curr Opin Endocrinol Diabetes Obes. (2017) 24:310–4. doi: 10.1097/MED.0000000000000360
92. Berg EA, Huang Z, Wang Y, Woo Baidal J, Fennoy I, Lavine JE, et al. Magnetic resonance imaging to assess body composition change in adolescents with obesity after sleeve gastrectomy. J Pediatr Gastroenterol Nutr. (2022) 75:761–7. doi: 10.1097/MPG.0000000000003607
93. Slomka P, Berman DS, Alexanderson E, Germano G. The role of PET quantification in cardiovascular imaging. Clin Transl Imaging. (2014) 2:343–58. doi: 10.1007/s40336-014-0070-2
94. Dar T, Radfar A, Abohashem S, Pitman RK, Tawakol A, Osborne MT. Psychosocial stress and cardiovascular disease. Curr Treat Options Cardiovasc Med. (2019) 21(5):23. doi: 10.1007/s11936-019-0724-5
95. Marinescu MA, Loffler AI, Ouellette M, Smith L, Kramer CM, Bourque JM. Coronary microvascular dysfunction, microvascular angina, and treatment strategies. JACC Cardiovasc Imaging. (2015) 8:210. doi: 10.1016/j.jcmg.2014.12.008
96. Schindler TH, Fearon WF, Pelletier-Galarneau M, Ambrosio G, Sechtem U, Ruddy TD, et al. Myocardial perfusion PET for the detection and reporting of coronary microvascular dysfunction: a JACC: cardiovascular imaging expert panel statement. JACC Cardiovasc Imaging. (2023) 16:536–48. doi: 10.1016/j.jcmg.2022.12.015
97. Xie F, Xiao Y, Li X, Wu Y. Association between the weight-adjusted-waist index and abdominal aortic calcification in United States adults: results from the national health and nutrition examination survey 2013–2014. Front Cardiovasc Med. (2022) 9:948194. doi: 10.3389/fcvm.2022.948194
98. Alaa AM, Bolton T, Di Angelantonio E, Rudd JHF, van der Schaar M. Cardiovascular disease risk prediction using automated machine learning: a prospective study of 423,604 UK biobank participants. PLoS One. (2019) 14:e0213653. doi: 10.1371/journal.pone.0213653
99. Davenport T, Kalakota R. The potential for artificial intelligence in healthcare. Fut Healthc J. (2019) 6:94–8. doi: 10.7861/futurehosp.6-2-94
100. Dey D, Slomka PJ, Leeson P, Comaniciu D, Shrestha S, Sengupta PP, et al. Artificial intelligence in cardiovascular imaging: JACC state-of-the-art review. J Am Coll Cardiol. (2019) 73:1317–35. doi: 10.1016/j.jacc.2018.12.054
101. Swain D, Parmar B, Shah H, Gandhi A, Pradhan MR, Kaur H, et al. Cardiovascular disease prediction using Various machine learning algorithms. J Comput Sci. (2022) 18:993–1004. doi: 10.3844/jcssp.2022.993.1004
102. Hippisley-Cox J, Coupland C, Vinogradova Y, Robson J, May M, Brindle P. Derivation and validation of QRISK, a new cardiovascular disease risk score for the United Kingdom: prospective open cohort study. Br Med J. (2007) 335:136. doi: 10.1136/bmj.39261.471806.55
103. Hemann BA, Bimson WF, Taylor AJ. The Framingham risk score: an appraisal of its benefits and limitations. Am Heart Hosp J. (2007) 5:91–6. doi: 10.1111/j.1541-9215.2007.06350.x
104. Shivakumar V, Kandhare AD, Rajmane AR, Adil M, Ghosh P, Badgujar LB, et al. Estimation of the long-term cardiovascular events using UKPDS risk engine in metabolic syndrome patients. Indian J Pharm Sci. (2014) 76:174–8. PMID: 2484319
105. Sofogianni A, Stalikas N, Antza C, Tziomalos K. Cardiovascular risk prediction models and scores in the era of personalized medicine. J Pers Med. (2022) 12(7):1180. doi: 10.3390/jpm12071180
106. Swathy M, Saruladha K. A comparative study of classification and prediction of cardio-vascular diseases (CVD) using machine learning and deep learning techniques. ICT Express. (2022) 8:109–16. doi: 10.1016/j.icte.2021.08.021
Keywords: cardiovascular risk assessment, subclinical cardiovascular disease, body composition analysis, abdominal aortic calcification, dual-energy x-ray absorptiometry
Citation: Yang S, Chen Q, Fan Y, Zhang C and Cao M (2024) The essential role of dual-energy x-ray absorptiometry in the prediction of subclinical cardiovascular disease. Front. Cardiovasc. Med. 11:1377299. doi: 10.3389/fcvm.2024.1377299
Received: 27 January 2024; Accepted: 15 August 2024;
Published: 29 August 2024.
Edited by:
Tanvir Alam, Hamad bin Khalifa University, QatarReviewed by:
Mohammad Islam, Southern Connecticut State University, United StatesMuhammad Arif, Hamad Bin Khalifa University, Qatar
Copyright: © 2024 Yang, Chen, Fan, Zhang and Cao. This is an open-access article distributed under the terms of the Creative Commons Attribution License (CC BY). The use, distribution or reproduction in other forums is permitted, provided the original author(s) and the copyright owner(s) are credited and that the original publication in this journal is cited, in accordance with accepted academic practice. No use, distribution or reproduction is permitted which does not comply with these terms.
*Correspondence: Cuntai Zhang, Y3R6aGFuZ0B0amgudGptdS5lZHUuY24=; Ming Cao, Y2FvLW1pbmdAdGpoLnRqbXUuZWR1LmNu
†These authors have contributed equally to this work and share first authorship