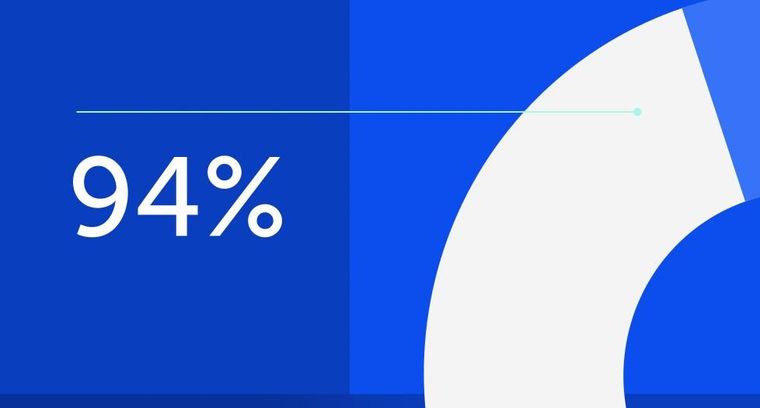
94% of researchers rate our articles as excellent or good
Learn more about the work of our research integrity team to safeguard the quality of each article we publish.
Find out more
ORIGINAL RESEARCH article
Front. Cardiovasc. Med., 26 August 2024
Sec. General Cardiovascular Medicine
Volume 11 - 2024 | https://doi.org/10.3389/fcvm.2024.1364126
This article is part of the Research TopicMendelian Randomization and Cardiovascular DiseasesView all 16 articles
Background: Observational clinical studies suggest an association between dilated cardiomyopathy (DCM) and various factors including titin, cardiac troponin I (CTnI), desmocollin-2, the perinatal period, alcoholism, Behçet's disease, systemic lupus erythematosus, hyperthyroidism and thyrotoxicosis, hypothyroidism, carnitine metabolic disorder, and renal insufficiency. The causal nature of these associations remains uncertain. This study aims to explore these correlations using the Mendelian randomization (MR) approach.
Objective: To investigate the etiology of DCM through Mendelian randomization analysis.
Methods: Data mining was conducted in genome-wide association study databases, focusing on variant target proteins (titin, CTnI, desmocollin-2), the perinatal period, alcoholism, Behçet's disease, systemic lupus erythematosus, hyperthyroidism and thyrotoxicosis, hypothyroidism, carnitine metabolic disorder, and renal insufficiency, with DCM as the outcome. The analysis employed various regression models, namely, the inverse-variance weighted (IVW), MR-Egger, simple mode, weighted median, and weighted mode methods.
Results: The IVW results showed a correlation between titin protein and DCM, identifying titin as a protective factor [OR = 0.856, 95% CI (0.744–0.985), P = 0.030]. CTnI protein correlated with DCM, marking it as a risk factor [OR = 1.204, 95% CI (1.010–1.436), P = 0.040]. Desmocollin-2 also correlated with DCM and was recognized as a risk factor [OR = 1.309, 95% CI (1.085–1.579), P = 0.005]. However, no causal relationship was found between the perinatal period, alcoholism, Behçet's disease, systemic lupus erythematosus, hyperthyroidism and thyrotoxicosis, hypothyroidism, carnitine metabolic disorder, renal insufficiency, and DCM (P > 0.05). The MR-Egger intercept test indicated no pleiotropy (P > 0.05), affirming the effectiveness of Mendelian randomization in causal inference.
Conclusion: Titin, CTnI, and desmocollin-2 proteins were identified as independent risk factors for DCM. Contrasting with previous observational studies, no causal relationship was observed between DCM and the perinatal period, alcoholism, Behçet's disease, systemic lupus erythematosus, hyperthyroidism and thyrotoxicosis, hypothyroidism, carnitine metabolic disorder, or renal insufficiency.
Dilated cardiomyopathy (DCM) is a common disease that leads to heart failure, arrhythmias, and sudden cardiac death. It is the most prevalent form of cardiomyopathy globally, affecting individuals of all ages and genders (1). Since 2013, the prevalence of DCM has risen from 1/2,500 to 1/250 (2). Associated with cardiogenic sudden death and heart failure, DCM results in high hospital admission rates and a potential need for heart transplantation, causing considerable suffering and financial burdens for patients.
Despite its global public health impact, no effective cures for DCM exist. Treatment focuses on relieving heart failure symptoms, improving myocardial remodeling, and even implantation of LVADs or heart transplants in the terminal stage (3).The lack of a comprehensive understanding of DCM's pathogenesis and risk factors contributes to this challenge. Previous studies have reported several risk factors for DCM (4, 5), including functional variants in target proteins [titin, cardiac troponin I (CTnI), and desmocollin-2]; the perinatal period; alcoholism; secondary cardiomyopathy due to autoimmune diseases [Behçet's disease and systemic lupus erythematosus (SLE)]; metabolic, endocrine, and nutritional diseases (hyperthyroidism and thyrotoxicosis, hypothyroidism, and carnitine metabolic disorder); and cardiomyopathy secondary to other organ diseases (uremia). The etiology of DCM is complex and multifaceted. The cure rate of DCM based on risk factors is not very satisfactory (4, 5). Genetic studies can provide new ideas to further clarify the etiology of DCM. Therefore, understanding DCM's etiology is vital. Mendelian randomization (MR) uses genetic variations as instrumental variables (IVs) (6), offering an approach akin to randomized controlled trials (7). This statistical model, free from the confounding and reverse causation biases of observational studies, assesses the causal relationship between exposure and outcome factors (8). Our aim was to demonstrate a causal relationship between DCM and related causes using Mendelian randomization.
MR analysis adheres to three key assumptions: (1) the relevance assumption—genetic IVs should be closely associated with exposure factors; (2) the independence assumption—genetic IVs should not be linked with potential confounders; and (3) the exclusion restriction assumption—genetic IVs should influence the outcome solely through exposure factors (Figure 1). This study uses MR to examine the causal relationship between existing etiologies and DCM, employing variant target proteins (titin, CTnI, and desmocollin-2), perinatal period, alcoholism, Behçet's disease, systemic lupus erythematosus, hyperthyroidism and thyrotoxicosis, hypothyroidism, carnitine metabolic disorder, and renal insufficiency as exposure factors, and DCM as the outcome factor.
We obtained genetic data for exposure and outcome factors from genome-wide association studies (GWAS). Genetic data for titin, CTnI, and desmocollin-2 came from the study by Sun et al. (9), comprising 3,301 samples and 10,534,735 single nucleotide polymorphisms (SNPs); perinatal period genetic data from the Integrative Epidemiology Unit (IEU) Open GWAS database, with 218,792 samples and 16,380,466 SNPs; alcoholism genetic data from the IEU Open GWAS database, encompassing 217,277 samples and 16,380,440 SNPs; lupus genetic data from the study by Sakaue et al. (10), featuring 482,911 samples and 24,198,877 SNPs; Behçet's disease genetic data also from Sakaue et al. (10), including 317,252 samples and 19,084,009 SNPs; hyperthyroidism and thyrotoxicosis genetic data from Dönertaş et al. (11), with 484,598 samples and 9,587,836 SNPs; hypothyroidism genetic data again from Sakaue et al. (10), comprising 410,141 samples and 24,138,872 SNPs; carnitine metabolic disorder genetic data from Shin et al. (12), including 7,797 samples and 2,545,563 SNPs; and finally, renal insufficiency genetic data from Sakaue et al. (10), featuring 482,858 samples and 24,185,976 SNPs. Genetic data for the outcome factor, DCM, were obtained from Sakaue et al. (10), encompassing 355,381 samples and 19,080,278 SNPs (Table 1).
IVs for exposure factors must meet these criteria: (1) All IVs should be genome-wide significant (P < 5 × 10−6/P < 5 × 10−8); (2) Linkage disequilibrium parameter (R2) = 0.001, with a regional range within 10,000 kb; (3) The F-statistic assesses weak instrument bias, representing the strength of individual SNPs. An SNP is considered strong enough to avoid biases if its F-statistic is >10. The F-statistic formula is F = β2/SE2 (13). We calculated the F-statistic and excluded SNPs with F <10 to prevent bias; (4) Knock out any palindromic SNPs (14) or incompatible alleles; (5) SNPs linked to confounding factors are removed. This involves consulting the PhenoScanner database and excluding SNPs associated with exposure or outcomes. The refined SNPs were used as IVs in this study. This removed confounding factors including malnutrition, strenuous exercise, overexertion, and radiation exposure (4).
The primary analysis method in this study was the inverse-variance weighted (IVW) method, which offers robust results without pleiotropy. Supplementary methods included MR-Egger, simple mode, weighted median (WM), and weighted mode to verify the robustness of the main analysis. For sensitivity analyses, we used a leave-one-out analysis approach and the outlier detection (MR-PRESSO) method. The outlier method identifies and interprets significant outliers that may increase heterogeneity and horizontal pleiotropy. The leave-one-out sensitivity analysis examines the impact of individual SNPs on the causal relationship. Sensitivity analysis for each SNP helps exclude variables that may significantly affect the results. Pleiotropy was assessed via the MR-Egger intercept test; a P <0.05 indicates pleiotropy. Heterogeneity was evaluated using Cochran's Q-value from the IVW and MR-Egger tests, with Q-Pval <0.05 signaling heterogeneity. In cases of heterogeneity, a random-effects model is applied in MR analysis. We conducted MR analysis using the “TwoSampleMR,” “MRPRESSO,” and “forestploter” packages in R software (version 4.3.2, R Foundation, Vienna, Austria).
In this study, a complete GWAS database was used to screen and remove confounding factors, and SNPs with a strong association with exposure factors were obtained. To avoid the phenomenon of linkage disequilibrium and reduce the bias caused by weak instruments and other problems, screening was further carried out according to the filter conditions of the instrumental variables mentioned above. We further excluded any palindromic SNPs. A palindromic SNP is an SNP in which the possible alleles for that SNP are the same alleles that would pair with each other in the double helix structure. When this occurs, alleles on the forward strand are the same as on the reverse strand. In two-sample MR, it can be difficult to harmonize palindromic SNPs if it is unclear which way the alleles are presented in the data sources providing information on the IV-exposure association and the IV-outcome associations. Therefore, it is necessary to delete the palindromic SNPs to ensure the accuracy of the results (14). Finally, a total of 164 SNPs (Supplementary Table S1) were included, all with an F-statistic greater than 10, and the results of MR analysis were not affected by weak instrumental variables. According to the MR-Egger intercept test, none of the data had any pleiotropy (P > 0.05) and the MR analysis could be performed.
The titin protein analysis showed a consistent direction across all five methods (Figure 2). Before MR analysis, palindromic SNP rs62127764 was removed. The heterogeneity test showed no heterogeneity (Q-Pval > 0.05), and a fixed-effects model IVW method was applied. Sensitivity analysis with leave-one-out analysis and the MR-PRESSO method identified no impactful SNPs on the causal relationship (Figure 3). The MR-Egger test indicated no pleiotropy (P = 0.783). The IVW results revealed a correlation between titin protein and DCM (Table 2), suggesting it as a protective factor [OR = 0.856, 95% CI (0.744–0.985), P = 0.030].
CTnI protein analysis displayed a consistent direction across all five methods (Figure 2). No palindromic SNPs were found prior to MR analysis. The heterogeneity test indicated no heterogeneity (Q-Pval > 0.05), utilizing a fixed-effects model IVW method. Sensitivity analysis with leave-one-out analysis and the MR-PRESSO method showed no significant SNPs affecting the causal relationship (Figure 3). The MR-Egger test revealed no pleiotropy (P = 0.680). The IVW results demonstrated a correlation between the CTnI protein and DCM (Figure 4), identifying it as a risk factor [OR = 1.204, 95% CI (1.009–1.436), P = 0.039].
Desmocollin-2 analysis indicated a consistent direction in all five methods (Figure 2). Palindromic SNPs rs147947012 and rs1789063 were excluded before MR analysis. The heterogeneity test showed no heterogeneity (Q-Pval > 0.05), leading to a fixed-effects model IVW approach. Sensitivity analysis with leave-one-out analysis and the MR-PRESSO method did not find any impactful SNPs on the causal relationship (Figure 3). The MR-Egger test indicated no pleiotropy (P = 0.396). The IVW results suggested desmocollin-2 was a risk factor for DCM (Table 2) [OR = 1.309, 95% CI (1.085–1.579), P = 0.005].
The perinatal period analysis showed a consistent direction across all five methods (Figure 2). Before MR analysis, no palindromic SNPs were detected. The heterogeneity test indicated no heterogeneity (Q-Pval > 0.05), employing a fixed-effects model IVW method. Sensitivity analysis with leave-one-out analysis and the MR-PRESSO method identified no significant SNPs affecting the causal relationship (Figure 3). The MR-Egger test showed no pleiotropy (P = 0.977). The IVW results suggested the perinatal period was a risk factor for DCM (Table 2) [OR = 1.006, 95% CI (0.929–1.090), P = 0.876], but this was not statistically significant (P > 0.05).
Alcoholism analysis demonstrated consistent direction in all five methods (Figure 2). Before MR analysis, palindromic SNP rs57490218 was excluded. The heterogeneity test showed no heterogeneity (Q-Pval > 0.05), utilizing a fixed-effects model IVW method. Sensitivity analysis with leave-one-out analysis and MR-PRESSO method found no impactful SNPs on the causal relationship (Figure 3). MR-Egger test indicated no pleiotropy (P = 0.830). IVW results suggested alcoholism as a protective factor for DCM (Table 2) [OR = 0.982, 95% CI (0.935–1.031), P = 0.467], but this was not statistically significant (P > 0.05).
SLE analysis showed a consistent direction in all five methods (Figure 2). No palindromic SNPs were found before MR analysis. The heterogeneity test indicated no heterogeneity (Q-Pval > 0.05), applying a fixed-effects model IVW method. Sensitivity analysis with leave-one-out analysis and MR-PRESSO method did not reveal significant SNPs affecting the causal relationship (Figure 3). The MR-Egger test showed no pleiotropy (P = 0.286). The IVW results indicated SLE was a risk factor for DCM (Table 2) [OR = 0.957, 95% CI (0.879–1.043), P = 0.317], but this was not statistically significant (P > 0.05).
The analysis of Behçet's disease showed a consistent direction in three methods (Figure 2). Before MR analysis, palindromic SNP rs2697704 and weak instrumental variable rs72735844 with an F <10 were excluded. The heterogeneity test showed no heterogeneity (Q-Pval > 0.05), using a fixed-effects model IVW method. Sensitivity analysis with leave-one-out analysis and the MR-PRESSO method found no significant SNPs affecting the causal relationship (Figure 3). The MR-Egger test indicated no pleiotropy (P = 0.711). The IVW results suggested Behçet's disease was a risk factor for DCM (Table 2) [OR = 1.004, 95% CI (0.966–1.043), P = 0.840], but this was not statistically significant (P > 0.05).
The analysis of hyperthyroidism and thyrotoxicosis showed a consistent direction in all five methods (Figure 2). Before MR analysis, palindromic SNPs rs12999008 and rs4903961 were excluded. The heterogeneity test indicated heterogeneity (Q-Pval < 0.05), and a random-effect model IVW method was applied. Sensitivity analysis with leave-one-out analysis and the MR-PRESSO method identified no significant SNPs on the causal relationship (Figure 3). The MR-Egger test showed no pleiotropy (P = 0.3324255). The IVW results indicated hyperthyroidism and thyrotoxicosis were protective factors for DCM (Table 2) [OR = 1.58 × 10−2, 95% CI (3.332 × 109 to 7.538 × 104), P = 0.597], but this was not statistically significant (P > 0.05).
The analysis of hypothyroidism showed a consistent direction across all five methods (Figure 2). Before MR analysis, palindromic SNPs rs1065386, rs114285740, rs187707293, rs2114702, rs2412976, rs2921053, rs3118469, and rs34536443, and incompatible allele rs11406335 were excluded. The heterogeneity test showed no heterogeneity (Q-Pval > 0.05), utilizing a fixed-effects model IVW method. Sensitivity analysis with leave-one-out analysis and the MR-PRESSO method revealed no significant SNPs impacting the causal relationship (Figure 3). The MR-Egger test indicated no pleiotropy (P = 0.755607). The IVW results suggested hypothyroidism was a risk factor for DCM (Table 2) [OR = 1.016, 95% CI (0.933–1.107), P = 0.710], but this was not statistically significant (P > 0.05).
The carnitine metabolic disorder analysis showed a consistent direction in all five methods (Figure 2). Before MR analysis, palindromic SNP rs13182512 was excluded. The heterogeneity test showed no heterogeneity (Q-Pval > 0.05), employing a fixed-effects model IVW method. Sensitivity analysis with leave-one-out analysis and the MR-PRESSO method identified no significant SNPs affecting the causal relationship (Figure 3). The MR-Egger test indicated no pleiotropy (P = 0.2428895). The IVW results suggested carnitine metabolic disorder was a risk factor for DCM (Table 2) [OR = 4.973, 95% CI (0.520–47.583), P = 0.164], but this was not statistically significant (P > 0.05).
The renal insufficiency analysis showed a consistent direction in two methods (Figure 2). Before MR analysis, palindromic SNPs rs11146948, rs12793321, rs3115665, rs4936552, and rs77638060 were excluded. The heterogeneity test indicated no heterogeneity (Q-Pval > 0.05), using a fixed-effects model IVW method. Sensitivity analysis with leave-one-out analysis and the MR-PRESSO method found no significant SNPs impacting the causal relationship (Figure 3). The MR-Egger test showed no pleiotropy (P = 0.279). The IVW results indicated renal insufficiency was a protective factor for DCM (Table 2) [OR = 0.955, 95% CI (0.728–1.254), P = 0.741], but this was not statistically significant (P > 0.05).
This study involved multiple exposures. To reduce the probability of a type I error, the FDR (Benjamini–Hochberg) method was used to appropriately correct the test P-value. The corrected results were 0.039, 0.039, and 0.015, respectively, and the FDR values were lower than 0.05, indicating significant differences among the three positive exposures.
To rule out a reverse causal relationship between DCM and a known etiology and meet the third key assumption of MR, two samples were used for univariate reverse MR analysis. The F-statistic for each SNP of the DCM was calculated to be >10 before MR analysis. Except for carnitine metabolic disorder, palindromic SNPs rs12051750 and rs62240972 were eliminated before MR analysis of the remaining 10 exposures (carnitine metabolic disorder had no palindromic SNPs). Heterogeneity tests showed no heterogeneity (Q-Pval > 0.05) using the fixed-effects model IVW method for analysis. Sensitivity analysis using the leave-one-out sensitivity analysis and the MR-PRESSO method did not identify SNPs that had a significant impact on the causal relationships. The MR-Egger test showed no pleiotropy, with P-values >0.05. The results of IVW method showed that DCM was not an influencing factor of known etiology and there is no causal relationship between them (Figure 4).
In this study, we utilized the GWAS database to select SNPs closely related to DCM and its etiologies. We employed five methods (MR-Egger, weighted median, fixed-effects model IVW, random-effect Model IVW, simple mode, weighted mode) to analyze the causal relationships. Mutations in genes linked to DCM leading to functional abnormalities in the target proteins (titin, CTnI, and desmocollin-2) demonstrated a causal relationship with DCM. Our findings contrast with previous observational clinical studies, demonstrating that factors such as alcoholism, the perinatal period, lupus, Behçet's disease, hyperthyroidism, hypothyroidism, carnitine metabolic disorder, and renal insufficiency showed no causal relationship with DCM. Our MR analysis indicates that decreased titin levels and increased CTnI and desmocollin-2 levels heighten DCM risk, confirming a significant causal relationship and aligning with existing research (5, 15). Previous studies have suggested that primary DCM is an autosomal genetic disorder caused by gene mutations. In this study, MR was used to explore the etiology of DCM at the genetic level, which is of great significance for deepening the understanding of the etiology of DCM. Titin is an important sarcomere protein, the largest protein known to be expressed in the heart, and maintains the structural integrity and elasticity of myocardial cells, provides power for myocardium, and participates in the regulation of myocardial sarcomere contraction and signaling (16). It also plays an active role in myocardial contraction, diastole, and passive tension. Titin truncating variants (TTNtvs) represent the most common cause of familial and sporadic DCM (17).Over the years, a variety of hypotheses have been proposed to explain the pathogenicity of TTNtvs; however, the two most popular have been haploinsufficiency (18) and the poison peptide hypothesis (19). In the case of the former, titin production from the single unaffected allele is insufficient to maintain appropriate cardiac function, causing a decrease in the left ventricular ejection fraction. The latter posits that the truncated protein acts in a manner that disrupts normal heart function. The findings of recent studies indicate that both mechanisms contribute to the development of DCM in TTNtv carriers (20).The pathophysiological changes of DCM are mainly cardiomyocyte hypertrophy, fibrous tissue hyperplasia, and non-specific degenerative changes and interstitial fibrosis. The lesion is diffuse and affects the whole heart, but the left ventricle is mainly dilated. The hypertrophy of the ventricular wall is relatively insignificant or even thin, and the systolic function of the heart is reduced. Thus, the titin protein plays a protective role in DCM. This is in line with our findings. CTnI, the striated muscle troponin complex's inhibitory subunit, mediates muscle relaxation by preventing myosin-actin binding and regulates Ca2+ sensitivity (21, 22). Increased CTnI levels can reduce cardiac muscle cell contractility. Studies have shown a potential link between CTnI and the prognosis of patients with DCM. Elevated serum CTnI is the preferred marker for diagnosing myocardial injury due to its excellent sensitivity and specificity, and its elevated levels are an independent predictor of increased all-cause mortality in patients with DCM (23). Cardiac desmosomes are cell–cell junctions that connect the intercalated disc to the intermediate filament system, containing a total of five desmosomal proteins that have important mechanical and signaling functions. Desmocollin-2, as one of the five desmosomal transmembrane proteins in the heart, assembles a desmosome junction with the other four desmosomal proteins to ensure the structural and functional integrity of the myocardium. It is also involved in cell signaling, apoptosis, and cell-to-cell adhesion (24). Heart-specific overexpression of desmocollin-2 induces myocardial necrosis, acute inflammation, and patchy cardiac fibrotic remodeling, leading to fulminant cardiomyopathy with extensive cardiac fibrosis with necrosis and calcification, resulting in shortened ventricular fraction and reduced ejection fraction (25). Furthermore, mutations in the desmocollin-2 gene may cause cardiomyopathy and related arrhythmias (26, 27). These results suggest that CTnI and desmocollin-2 can damage cardiomyocytes and cause or aggravate DCM. These may explain our findings that CTnI and desmocollin-2 are risk factors for cardiopathy.
Previous observational clinical studies have suggested that cardiac disease is associated with alcoholism, perinatal period, lupus erythematosus, Behcet's disease, hyperthyroidism and thyrotoxicosis, hypothyroidism, carnitine metabolic disorder, and renal insufficiency, but this study ruled out a causal relationship with them using Mendelian randomization. The reasons may be related to the following: (1) MR analysis, based on the GWAS database, selects genetic variants (SNPs) as instrumental variables, effectively avoiding biases from lifestyle, social environment, and other confounding factors; (2) Previous studies have only included myocardial damage as the cause of cardiac disease, but have not further clarified whether there is a causal relationship between them; (3) This study employed large-sample MR analysis, offering more convincing results compared to previous small-sample observational studies.
Limitations include the following: (1) The GWAS data for the MR analysis population are from Europeans, and racial differences in allele frequencies and disease prevalence might affect the applicability of the findings; (2) GWAS database limitations mean detailed data such as disease severity is unavailable; (3) SNPs for hyperthyroidism, thyrotoxicosis, and carnitine metabolic disorder with an F-statistic of <10 were used and such weak instrumental variables could introduce bias; (4) The GWAS database lacks data on the main familial dilated cardiomyopathy (FDCM) related gene target proteins (α/β-Myosin heavy chain, lamin A/C, cardiac actin, etc.) with a frequency of >5%, precluding MR analysis of these etiologies.
In summary, this study employs MR analysis to examine potential etiological factors of DCM and the causal relationship between myocardial-related proteins and DCM. It was found that alcoholism, perinatal factors, lupus erythematosus, Behçet's disease, hyperthyroidism and thyrotoxicosis, hypothyroidism, and carnitine metabolic disorder have no significant causal relationship with DCM. A causal relationship exists between myocardial-related proteins and DCM, among which titin protein is a protective factor for DCM, whereas cardiac troponin I and desmocollin-2 are risk factors. The application of myocardial cell proteomics in DCM offers promising prospects in the understanding of DCM progression at the molecular and cellular pathway levels (28), facilitating earlier diagnosis of DCM. This also uncovers new molecular therapeutic targets, providing innovative approaches for the treatment of DCM.
The datasets presented in this study can be found in online repositories. The names of the repository/repositories and accession number(s) can be found in the article/Supplementary Material.
The raw data for Mendelian randomization were all derived from network-shared databases, so they did not require review and approval by an ethics committee.
SX: Writing – original draft, Writing – review & editing. HJ: Writing – original draft, Writing – review & editing, Methodology, Supervision.
The authors declare financial support was received for the research, authorship, and/or publication of this article. This study was supported by the Shandong Provincial Medical and Health Science and Technology Development Project (202103011004).
The authors declare that the research was conducted in the absence of any commercial or financial relationships that could be construed as a potential conflict of interest.
All claims expressed in this article are solely those of the authors and do not necessarily represent those of their affiliated organizations, or those of the publisher, the editors and the reviewers. Any product that may be evaluated in this article, or claim that may be made by its manufacturer, is not guaranteed or endorsed by the publisher.
The Supplementary Material for this article can be found online at: https://www.frontiersin.org/articles/10.3389/fcvm.2024.1364126/full#supplementary-material
1. Reichart D, Magnussen C, Zeller T, Blankenberg S. Dilated cardiomyopathy: from epidemiologic to genetic phenotypes. J Intern Med. (2019) 286(4):362–72. doi: 10.1111/joim.12944
2. Fatkin D, Calkins H, Elliott P, James CA, Peters S, Kovacic JC. Contemporary and future approaches to precision medicine in inherited cardiomyopathies: JACC focus seminar 3/5. J Am Coll Cardiol. (2021) 77:2551–72. doi: 10.1016/j.jacc.2020.12.072
3. McDonagh TA, Metra M, Adamo M, Gardner RS, Baumbach A, Böhm M, et al. 2021 ESC guidelines for the diagnosis and treatment of acute and chronic heart failure. Eur Heart J. (2021) 42:3599–726. doi: 10.1093/eurheartj/ehab368
4. Heymans S, Lakdawala NK, Tschöpe C, Klingel K. Dilated cardiomyopathy: causes, mechanisms, and current and future treatment approaches. Lancet. (2023) 402(10406):998–1011. doi: 10.1016/S0140-6736(23)01241-2
5. Yang J, Liao Y, Yuan J, Wang C, Cheng X, Zhao D. Guidelines for the diagnosis and treatment of dilated cardiomyopathy in China. J Clin Cardiovasc Dis. (2018) 34(5):421–34. doi: 10.13201/j.issn.1001-1439.2018.05.001
6. Yu T, Xu W, Su Y, Li J. Fundamental principles, methods, and limitations of Mendelian randomization studies. Chin J Evid Based Med. (2021) 21(10):1227–34. https://kns.cnki.net/kcms2/article/abstract?v=koCHBfbQEdYHNfZ0d3 CgZpsgqo_j5DG2rKnMALFhcsseakE3AUaFtynYAv1TLuKsAw8ocR8W89AxINq63WLLvYsYX1iWOX58L9IY9An1NaMs5MQoWO2cCN9-rd5Bw1g2BUH3H8JzAH5yNzKd1xVVzMfQVpDEUn0BNwJX2joac9tPiZ25V5rGEC606C28kzNMECo55zsaBw=&uniplatform=NZKPT&language=CHS
7. Biostatistics Expert Committee of CSCO RWS Methodology Group. Statistical consensus on the Mendelian randomization model and its standardized application. Chin Health Stat. (2021) 38(3):471–5, 480. https://kns.cnki.net/kcms2/article/abstract?v=9koCHBfbQEeXup7-QJU1xGetl6DRI_MOLEXq3jbwWQ-euX88NZRIW__ILD1fd12F_bn6krWrcAAbG69sZ3TnzvMwfejh1razHHp7ow_gJ268VpliWHRv78jaOJKT0SD1gITmLFvXBxSbvlNcga0qqhi53Rg0yzkIGzcTXPHIbutHucseQtwN3fTacMQqPhkdfllLUMxdlQ=&uniplatform=NZKPT&language=CHS
8. Richmond RC, Davey smith G. Mendelian randomization: concepts and scope. Cold Spring Harbor Perspect Med. (2022) 12(1):a040501. doi: 10.1101/cshperspect.a040501
9. Sun BB, Maranville JC, Peters JE, Stacey D, Staley JR, Blackshaw J, et al. Genomic atlas of the human plasma proteome. Nature. (2018) 558(7708):73–9. doi: 10.1038/s41586-018-0175-2
10. Sakaue S, Kanai M, Tanigawa Y, Karjalainen J, Kurki M, Koshiba S, et al. A cross-population atlas of genetic associations for 220 human phenotypes. Nat Genet. (2021) 53(10):1415–24. doi: 10.1038/s41588-021-00931-x
11. Dönertas HM, Fabian DK, Valenzuela MF, Partridge L, Thornton JM. Common genetic associations between age-related diseases. Nat Aging. (2021) 1(4):400–12. doi: 10.1038/s43587-021-00051-5
12. Shin SY, Fauman EB, Petersen AK, Krumsiek J, Santos R, Huang J, et al. An atlas of genetic influences on human blood metabolites. Nat Genet. (2014) 46(6):543–50. doi: 10.1038/ng.2982
13. Xiong Y, Zhong X, Zhang F, Wang W, Zhang Y, Wu C, et al. Genetic evidence supporting a causal role of snoring in erectile dysfunction. Front Endocrinol (Lausanne). (2022) 13:896369. doi: 10.3389/fendo.2022.896369
14. Hemani G, Zheng J, Elsworth B, Wade KH, Haberland V, Baird D, et al. The MR-Base platform supports systematic causal inference across the human phenome. eLife. (2018) 7:e34408. doi: 10.7554/eLife.34408
15. Wang Y, Wang X, Yang Y, Xu H, Li J. Long-term prognostic value of high-sensitivity cardiac troponin-I in patients with idiopathic dilated cardiomyopathy. Open Med (Wars). (2023) 18(1):20230837. doi: 10.1515/med-2023-0837
16. Loescher CM, Hobbach AJ, Linke WA. Titin (TTN): from molecule to modifications, mechanics, and medical significance. Cardiovasc Res. (2022) 118(14):2903–18. doi: 10.1093/cvr/cvab328
17. Mazzarotto F, Tayal U, Buchan RJ, Midwinter W, Wilk A, Whiffin N, et al. Reevaluating the genetic contribution of monogenic dilated cardiomyopathy. Circulation. (2020) 141(5):387–98. doi: 10.1161/CIRCULATIONAHA.119.037661
18. Fomin A, Gärtner A, Cyganek L, Tiburcy M, Tuleta I, Wellers L, et al. Truncated titin proteins and titin haploinsufficiency are targets for functional recovery in human cardiomyopathy due to TTN mutations. Sci Transl Med. (2021) 13(618):eabd3079. doi: 10.1126/scitranslmed.abd3079
19. Yotti R, Seidman CE, Seidman JG. Advances in the genetic basis and pathogenesis of sarcomere cardiomyopathies. Annu Rev Genomics Hum Genet. (2019) 20:12–153. doi: 10.1146/annurev-genom-083118-015306
20. Stroik D, Gregorich ZR, Raza F, Ge Y, Guo W. Titin: roles in cardiac function and diseases. Front Physiol. (2024) 15:1385821. doi: 10.3389/fphys.2024.1385821
21. Davies E, Gawad Y, Takahashi M, Shi Q, Lam P, Styba G, et al. Analytical performance and utility of a sensitive immunoassay for determination of human cardiac troponin I. Clin Biochem. (1997) 30:479–90. doi: 10.1016/S0009-9120(97)00111-2
22. Arifulin EA, Sheval EV. Non-canonical localization of cardiac troponins: expanding functions or causing pathologies? Int J Mol Sci. (2024) 25(6):3117. doi: 10.3390/ijms25063117
23. Li X, Luo R, Jiang R, Kong H, Tang Y, Shu Y, et al. The prognostic use of serum concentrations of cardiac troponin-I, CK-MB and myoglobin in patients with idiopathic dilated cardiomyopathy. Heart Lung. (2014) 43(3):219–24. doi: 10.1016/j.hrtlng.2014.03.001
24. Murray B. Arrhythmogenic right ventricular dysplasia/cardiomyopathy (ARVD/C): a review of molecular and clinical literature. J Genet Couns. (2012) 21(4):494–504. doi: 10.1007/s10897-012-9497-7
25. Brodehl A, Belke DD, Garnett L, Martens K, Abdelfatah N, Rodriguez M, et al. Transgenic mice overexpressing desmocollin-2 (DSC2) develop cardiomyopathy associated with myocardial inflammation and fibrotic remodeling. PLoS One. (2017) 12(3):e0174019. doi: 10.1371/journal.pone.0174019
26. McNally E, MacLeod H, Dellefave-Castillo L. Arrhythmogenic right ventricular cardiomyopathy overview. 2005 [updated May 11, 2023]. In: Adam MP, Feldman J, Mirzaa GM, Pagon RA, Wallace SE, Bean LJH, et al., editors. GeneReviews® [Internet]. Seattle, WA: University of Washington, Seattle (1993–2023). Available from: https://www.ncbi.nlm.nih.gov/books/NBK1131/ (accessed May 11, 2023).
27. Gerull B, Brodehl A. Insights into genetics and pathophysiology of arrhythmogenic cardiomyopathy. Curr Heart Fail Rep. (2021) 18(6):378–90. doi: 10.1007/s11897-021-00532-z
Keywords: Mendelian randomization, dilated cardiomyopathy (DCM), genome-wide association study (GWAS), etiology, single nucleotide polymorphism (SNP)
Citation: Xue S and Jiang H (2024) Exploring the etiology of dilated cardiomyopathy using Mendelian randomization. Front. Cardiovasc. Med. 11:1364126. doi: 10.3389/fcvm.2024.1364126
Received: 1 January 2024; Accepted: 25 July 2024;
Published: 26 August 2024.
Edited by:
Panpan Hao, Shandong University, ChinaReviewed by:
Ruijian Li, Shandong University, ChinaCopyright: © 2024 Xue and Jiang. This is an open-access article distributed under the terms of the Creative Commons Attribution License (CC BY). The use, distribution or reproduction in other forums is permitted, provided the original author(s) and the copyright owner(s) are credited and that the original publication in this journal is cited, in accordance with accepted academic practice. No use, distribution or reproduction is permitted which does not comply with these terms.
*Correspondence: HongJu Jiang, eWl6aGV6YWl0dUAxNjMuY29t
Disclaimer: All claims expressed in this article are solely those of the authors and do not necessarily represent those of their affiliated organizations, or those of the publisher, the editors and the reviewers. Any product that may be evaluated in this article or claim that may be made by its manufacturer is not guaranteed or endorsed by the publisher.
Research integrity at Frontiers
Learn more about the work of our research integrity team to safeguard the quality of each article we publish.