- Department of Cardiology, Affiliated Hospital of Nanjing University of Chinese Medicine, Nanjing, Jiangsu, China
Background and objectives: Heart failure (HF) is a disease with numerous genetic and environmental factors that affect it. The results of previous studies indicated that immune phenotypes are associated with HF, but there have been inconclusive studies regarding a causal relationship. Therefore, Mendelian randomization (MR) analyses were undertaken to confirm the causal connections between immune phenotypes and HF, providing genetic evidence supporting the association of immune cell factors with HF risk.
Methods: We selected instrumental variables that met the criteria based on data from the results of genome-wide association studies (GWAS) of immune phenotype and all-cause HF. An evaluation of the causal association between 731 immune cell factors and HF risk was carried out using the inverse variance weighted (IVW), MR-Egger regression (MR-Egger), and weighted median (WM) analysis methods. To determine the horizontal pleiotropy, heterogeneity, and stability of the genetic variants, the MR-Egger intercept test, Cochran's Q test, MR-PRESSO, and leave-one-out sensitivity analysis were performed.
Results: MR principal method (IVW) analysis showed that a total of 38 immune cell-related factors were significantly causally associated with HF. Further analyses combining three methods (IVW, MR-Egger and WME) showed that six exposure factors significantly associated with heart failure, as shown below. The effect of Dendritic cell Absolute Count, CD62l- CD86+ myeloid Dendritic cell Absolute Count, CD62l- CD86+ myeloid Dendritic cell% Dendritic cell, CD39+ CD8+ T cell% CD8+ T cell, CD3 on Central Memory CD4+ T cell on heart failure was positive. Whereas, a reverse effect was observed for CD14+ CD16+ monocyte% monocyte.
Conclusion: We investigated the causal relationship between immune phenotypes and all-cause HF. According to the results, Dendritic cell Absolute Count, CD62l- CD86+ myeloid Dendritic cell Absolute Count, CD62l- CD86+ myeloid Dendritic cell% Dendritic cell, CD39+ CD8+ T cell% CD8+ T cell, CD3 on Central Memory CD4+ T cell aggravate HF, and the risk of HF is decreased by CD14+ CD16+ monocyte% monocyte. These phenotypes may serve as new biomarkers, providing new therapeutic insights for the prevention and treatment of all-cause HF.
1 Introduction
In general, heart failure (HF) is caused by various structural or functional disorders of the heart that result in impaired ventricular filling and (or) ejection capacity, represented as dyspnea, limitation of physical activity and fluid retention (1). At present, approximately 64 million people are thought to suffer from HF worldwide, 20% of total cardiovascular cases, and have a prevalence of around 1%–12% (2–4). In 2019, a meta-analysis involving 1.5 million chronic heart failure patients revealed that the comprehensive survival rates for these patients at one year, two years, five years, and ten years were 86.5%, 72.6%, 56.7%, and 34.9%, respectively (5). HF has the characteristics of high morbidity, high hospitalization rate, and high mortality, and it accounts for a large economic and societal burden. Therefore, early diagnosis and treatment of HF to improve the condition of patients with HF and to reduce morbidity and mortality has become a significant public health concern around the world (6).
With increasing research into the pathophysiological mechanisms of HF, evidence is mounting that immune activation is involved in the process of HF. When the heart is damaged, various stimuli lead to the activation of effector immune cells, penetrating the vascular wall. Different subgroups of immune cells release various cytokines, including interleukin-6, interleukin-17, interleukin-10, tumor necrosis factor-alpha, and interferon-gamma. These cytokines target the regulation of vascular aging, degradation of elastic lamina, and the process of myocardial fibrosis. While HF generates cellular damage and structural remodeling, it can secrete related cytokines and substances, further intensifying this immune-inflammatory response. This, in turn, leads to additional damage to the body's heart and other organ tissues, creating a chronic malignant cycle. Consequently, this accelerates the progression of HF and leads to an adverse prognosis (7). In relation to HF, immune phenotypes have been shown to influence host immunity (8–10). Some studies suggest in the onset of HF, activation of NFKb and NLRP3 inflammasome triggers downstream production of IL-1b and IL-6, while clonal hematopoiesis mediated by TET2 is recognized as an accelerating factor in HF deterioration (11, 12). In Houssari M's investigation into the influence of infiltrating T cells on post-infarction cardiac lymphatic remodeling, the findings indicate that CD4 and CD8T cells, to a certain extent, effectively suppress the generation of cardiac lymphatic vessels through interferon-gamma, consequently slowing down cardiac remodeling and myocardial fibrosis following myocardial infarction (13). However, there are many factors that may affect the results of current research, including sample size, limitations in study design, and confounding variables. To date, there is still no consensus on the correlation between immune phenotypes and overall HF.
In recent years, genomics-wide association studies (GWAS) have provided the opportunity to uncover HF's genetic background, the linkage disequilibrium score regression (LDSC) and genomic structural equation modeling (Genomic SEM) can evaluate genetic correlations and partial correlations based on aggregated-level data (14, 15). HF is a complex disorder with an estimated heritability of around 26%. The Mendelian randomization (MR) method uses measured genetic variants as instrumental variables for exposures to infer the causation of the effects of exposures (16). Large-scale GWAS have identified numerous single nucleotide polymorphism (SNP) loci, providing a wealth of genetic variation instrumental variables for MR analyses, effectively reducing bias in causal relationship estimates (17). At the same time, because genotype precedes disease and is primarily independent of postnatal habits and environment, the theory ensures that confounding factors are balanced between different genetic variants. Thus, an analysis of MR has been used to determine potentially causal relationships between exposures and diseases (18–20).
In this study, all the current GWAS data related to 731 immune cells were collected and the causal effects of all immune cells and related factors on all-cause HF were assessed using a two-sample MR approach, which systematically screened out immune cells that may contribute to all-cause HF and provided new ideas for preventing and treating HF from an immune perspective.
2 Materials and methods
2.1 Study design
In this study, we systematically analyzed the potential causal effects of 731 immune cell phenotypes associated with all-cause HF using a two-sample MR analysis and evaluated the reliability of the results.
2.2 Data sources for exposure and outcomes
The summary data of the GWAS for immune cells is sourced from IEU Open GWAS (https://gwas.mrcieu.ac.uk/). The total sample size is 2,919 cases, with a total of 14,849,624 SNPs (21). The summary data regarding the GWAS for overall HF originates from the 9th edition of research data conducted by the FinnGen Consortium. This comprehensive study involves 376,233 Finnish adult participants, comprising 26,872 cases and 349,361 controls, encompassing a total of 5,747,754 SNPs (https://r9.finngen.fi/pheno/I9_HEARTFAIL_ALLCAUSE) (22). Data aggregated from publicly available sources was used in the study and, therefore, did not require ethical approval and consent for participation.
2.3 Instrument selection
In MR, genetic variants must meet three core assumptions of an instrumental variable (23). (1) The relevance assumption: genetic variants used as instrumental variables should be associated with the risk factor. (2) Independence assumption: the selected SNPs do not interact with other variables. (3) Exclusionary restriction assumption: instrumental variables affect all factors—the only factors contributing to HF are immune cell exposure factors and not alternative pathways.
To meet the above assumptions, we developed specific screening criteria for the instrumental variables. We analyzed the linkage disequilibrium of each risk factor based on the 1,000 genomes reference panel (European population). In the next step, a set of parameters was used to LD-clump the processed summary files (PLINK software, p1 = 1 × 10-5, r2 = 0.1, and distance 500 kb). Finally, we removed any outliers that may have been affected by pleiotropic bias using MR—PRESSO and Radial MR (24). The F statistic was approximated to determine whether the instrumental variable had a weak bias. Weak instrumental variables were defined as those with an F statistic less than 10 (25) (Figure 1).
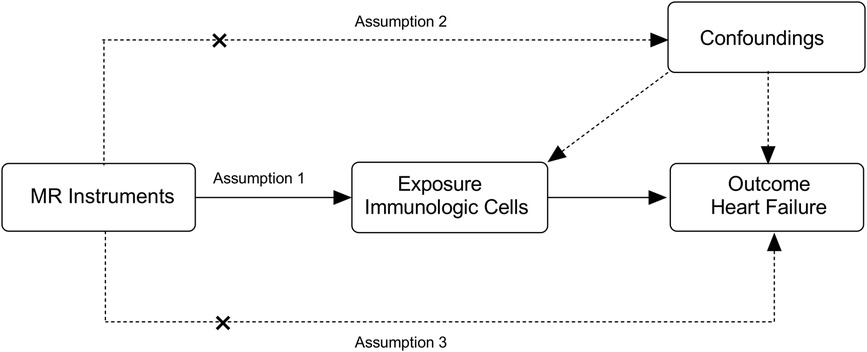
Figure 1 Overview of the overall MR design. (1) Assumption 1: the relevance assumption, genetic variants used as instrumental variables should be associated with the risk factor. (2) Assumption 2: the independence assumption, the selected genetic variants do not interact with other variables. (3) Assumption 3: the exclusion restriction assumption, instrumental variables affect all—cause HF only through immune cell exposure factors and not through additional pathways.
In order to remove SNPs associated with confounding factors and outcomes of HF, we manually screened and deleted them using PhenoScanner (http://www.phenoscanner.medschl.cam.ac.uk/) (26).
2.4 MR analysis
As a primary analysis, we used IVW for its efficiency in estimating the causal effect of immune phenotypes on the HF (27), combining the WM approach and MR-Eggerwas used as complementary analyses (28, 29). The joint analysis of the above multiple MR methods can test the stability and reliability of the association under different assumptions.
2.5 Sensitivity analysis
We performed heterogeneity tests, pleiotropy tests, and leave-one-out tests during the sensitivity analysis. The transverse pleiotropy was tested using the MR-Egger intercept. In the MR-Egger analysis with an intercept of 0.05, there probably isn't a horizontal pleiotropic pathway (30). We evaluated SNP heterogeneity using Cochran' s Q test. The fixed-effects model was applied for SNPs with no evidence of heterogeneity between studies (Cochran's Q test P < 0.05), and the random effects model was applied for SNPs exhibiting heterogeneity between studies (Cochran's Q test P > 0.05) (31). For the detection of outliers, we used the MR-pleiotropy residual sum and outlier (MR—PRESSO) test. If outliers were present, they were removed, and we re-evaluated the MR causal estimates. Additionally, we conducted a leave-one-out analysis to test whether one SNP had a significant effect (32). Odds ratios (ORs) and 95% confidence intervals (CIs) are presented to show the association between the potential associated factors and HF. A significant difference was considered when P was <0.05.
2.6 Reverse MR analysis
To assess whether HF has a causal effect on the levels of immune cells in the body, the MR mentioned above analysis method was employed. In this approach, systemic HF was considered as the exposure, and individual immune cell phenotypes were separately examined as outcomes in a reverse MR analysis. Two-sample MR and MR-PRESSO packages (version 1.0 and 0.5.4) of the R program (version 4.3.1) were used for statistical analysis (33).
3 Results
3.1 Instrumental variable selection results
This study utilized GWAS data for 731 immune cell phenotypes and associated factors as the focus of investigation. Employing criteria including a significance threshold of P < 1 × 10-05 and linkage disequilibrium analysis, a total of 38 immune cell phenotypes were identified through MR analysis, with an average F-statistic exceeding 10 (Table 1).
3.2 Main results of the MR analysis
In the primary analysis using the IVW method, a positive correlation was observed between 25 immune cell-related factors and the risk of HF (OR > 1). Conversely, 13 immune cell-related factors showed a negative correlation (OR < 1) with the incidence of HF (Figure 2).
3.3 Combined results of the three methods in MR analysis
In the combined analysis using IVW, MR-Egger regression, and WM, Dendritic cell Absolute Count, CD62l- CD86+ myeloid Dendritic cell Absolute Count, and CD62l- CD86+ myeloid Dendritic Cell% Dendritic cell exhibited potential positive causal effects on HF (0.001 < P < 0.05). Specifically, an increase in Dendritic cell Absolute Count levels is associated with an approximately 3% higher risk of HF incidence (IVW: OR = 1.03, 95% CI: 1.01–1.06). Similarly, CD62l- CD86+ myeloid Dendritic cell Absolute Count (IVW: OR = 1.013, 95% CI: 1.00–1.03) and CD62l- CD86+ myeloid Dendritic cell% Dendritic cell (IVW: OR = 1.01, 95% CI: 1.00–1.02) are also positively correlated with the onset of HF.
For HF, there is a significant positive causal effect for CD39+ CD8+ T cell% CD8+ T cell [OR = 1.01, 95% CI (1.00–1.02), P = 0.039], as well as CD3 on Central Memory CD4+ T cell [OR = 1.02, 95% CI (1.01–1.03), P = 0.002] (0.001 < P < 0.05). On the contrary, there is a negative correlation between CD14+ CD16+ monocyte% monocyte [OR = 0.98, 95% CI (0.96–0.99), P = 0.002] and the incidence risk of HF. In other words, for each increase of one standard deviation in CD14+ CD16+ monocyte% monocyte, the average risk of developing systemic HF may decrease by 2% (Figure 3).
3.4 Reverse MR analysis
The findings from the reverse MR study suggest that systemic HF does not appear to be a significant factor causing alterations in immune cells. Additionally, the likelihood ratio, MR-Egger intercept, and WM did not reveal a causal link between these variables.
3.5 Sensitivity analysis
Using MR-Eggerto assess horizontal pleiotropy between SNPs and outcomes, the results indicate that Dendritic cell Absolute Count (intercept P = 0.136), CD62l- CD86+ myeloid Dendritic cell Absolute Count (intercept P = 0.159), CD62l- CD86+ myeloid Dendritic cell% Dendritic cell (intercept P = 0.075), CD14+ CD16 + monocyte% monocyte (intercept P = 0.176), CD39+ CD8+ T cell% CD8+ T cell (intercept P = 0.156), and CD3 on Central Memory CD4+ T cell (intercept P = 0.947) show no evidence of horizontal pleiotropy with respect to systemic HF (P > 0.05) (Table 2).
Assessing the heterogeneity of SNPs selected through Cochran's test, the results indicate that for Dendritic cell Absolute Count, CD62l- CD86+ myeloid Dendritic cell Absolute Count, CD62l- CD86+ myeloid Dendritic cell% Dendritic cell, CD14+ CD16+ monocyte% monocyte, CD39+ CD8+ T cell% CD8+ T cell, and CD3 on Central Memory CD4+ T cell, both IVW and MR-Egger's Q_pval are >0.05, suggesting the absence of heterogeneity. Additionally, the MR-PRESSO method did not identify any outliers (P > 0.05) (Table 2).
The leave-one-out analysis demonstrated a close alignment between the included effect size and the overall effect size, indicating the absence of a single SNP exerting a dominant influence on the overall assessment. This further enhances the stability and reliability of the MR statistics (Figure 4).
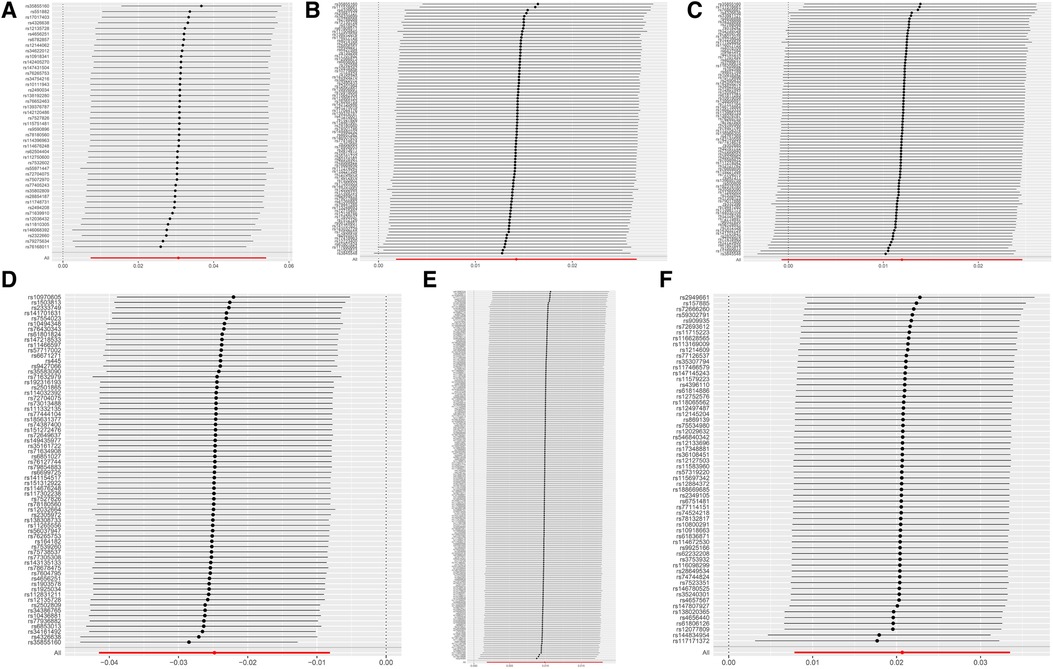
Figure 4 MR leave-one-out sensitivity analysis results. (A) DC Absolute Count. (B) CD62l- CD86+ MDC Absolute Count. (C) CD62l- CD86+ MDC %DC. (D) CD14+ CD16+ monocyte %monocyte. (E) CD39+ CD8+ T cell %CD8+ T cell. (F) CD3 on CM CD4+ T cell.
4 Discussion
Drawing upon an extensive dataset of publicly available genetic information, this study pioneered using a two-sample MR method to systematically assess the causal relationship between all discernible immune cell phenotypes and the overall incidence of HF. In the two-sample MR study, 38 immune cell-related factors were collectively identified as relevant to overall HF, including phenotypes associated with T cells, B cells, monocytes, and dendritic cells. Following stringent SNP quality control to mitigate confounding factors and reverse causation, we identified six immune cell-related exposure factors-Dendritic cell Absolute Count, CD62l- CD86+ Myeloid Dendritic cell Absolute Count, CD62l- CD86+ Myeloid Dendritic cell %Dendritic cell, CD14+ CD16+ Monocyte% Monocyte, CD39+ CD8+ T cell% CD8+ T cell, and CD3 on Central Memory CD4+ T cell-significantly associated with the overall risk of HF across three MR analysis methods (IVW, MR-Egger, WM). And the results of reverse MR showed no reverse causality between the six immune phenotypes and HF, suggesting that the effect of HF on these immune phenotypes was not statistically significant (P > 0.05).
Randomized controlled trial (RCT) stands as the gold standard in biomedical research for inferring causality (34). Nevertheless, RCTs are commonly hindered by their high costs, prolonged durations, and challenges in implementation, often stemming from ethical considerations and participant constraints. Observational studies, although more accessible for preliminary causation determination in etiological research, frequently encounter recognition challenges, especially when dealing with potential confounding factors and reverse causation (35). MR provides an effective solution to the aforementioned challenges by leveraging genetic variation as an instrumental variable. This approach adeptly mitigates confounding factors introduced by the environment. Furthermore, in contrast to the immediate outcomes derived from RCTs, exposure factors obtained from a genetic standpoint tend to endure over a lifetime and can even be hereditary to the next generation (36). HF, a chronic disease caused by multiple factors, remains poorly understood in its etiology despite extensive research into the mechanisms underlying its pathogenesis (37). Recent studies have revealed profound changes in the immune system during HF, encompassing the recruitment, activation of immune cells, and secretion of immune molecules induced by various factors. This intricate process of immune remodeling persists throughout the entire development and maintenance of HF (38). It is noteworthy that recent research emphasizes the significant impact of immune cells on the incidence, progression, and risk of HF (39, 40). Cells in the bloodstream, including neutrophils, macrophages, natural killer cells, eosinophils, and mast cells, demonstrate this effect by coordinating changes in tissue immune-inflammatory responses. This observation is corroborated by the upregulation of molecules such as PD-1 in regulatory T cells and oncostatin M in inflammatory macrophages. However, these observational studies only confirm the involvement of immune cells and inflammation in the pathogenesis of HF without providing reliable and significant causal evidence. Leveraging recent extensive GWAS on immune cell phenotypes and HF, two-sample MR studies provide a systematic evaluation of the causal relationship, offering an immunological perspective for clinical diagnosis and intervention in HF.
Dendritic cells, composed of diverse subsets from lymphoid and non-lymphoid organs, are specialized antigen-presenting cells that recognize pathogens in the innate immune system and activate immune cells in the adaptive immune system (41). Myeloid DCs and lymphoid DCs constitute the two primary sources of DCs, with myeloid DCs further categorized into precursor stage, immature DCs (iDCs), migratory phase, and mature DCs (mDCs) (42). The generation of autoimmune T cell responses to cardiac self-antigens requires direct interaction between antigen-presenting cells and T cells in lymphoid tissues; dendritic cells (DCs), as primary antigen-presenting cells, play a crucial role in regulating immune responses to foreign antigens and peripheral self-tolerance (43, 44). Our research uncovered a positive association between the immune phenotypes of Dendritic cell Absolute Count, CD62l- CD86+ Myeloid Dendritic cell Absolute Count, and CD62l- CD86+ Myeloid Dendritic cell% Dendritic cell, and the onset of HF. The upregulation of co-stimulatory molecule expression on the surface of DCs can activate T cells, inducing the occurrence of immune response and exacerbating the progression of HF (45). Furthermore, in studies related to myocardial ischemia, it has been observed that circulating DCs decrease in acute myocardial infarction patients due to their migration into myocardial tissue, confirming the involvement of DCs in the inflammatory processes of HF (46). MDCs (myeloid dendritic cells) are immune cells differentiated from myeloid stem cells under the stimulation of GM-CSF. Under different conditions, mDCs and iDCs (immature dendritic cells) can mediate the differentiation of CD+4 T cells into Th17 or Treg. This imbalance in the Th17/Treg cell ratio can lead to a close relationship with the imbalance of Th17 and Treg cells in the body, which is closely related to cardiac function (47–49). This suggests that mDCs play a crucial role in the immune mechanism of HF, and their involvement may accelerate the occurrence and progression of HF. Additional research has revealed that mDCs, by secreting a large amount of pro-inflammatory cytokine interleukin-12 (IL-12), promote the activation of T cells, leading to myocardial tissue remodeling and deterioration of heart function (50).
Monocytes, as a heterogeneous group of cells, are produced in the bone marrow, stored in the spleen, and circulate for 1–3 days in a balanced state (51). According to the expression of CD14, CD16 (Fcγ RIII), CD64 (Fcγ RI), and chemokine receptors CD192 and CX3CR1, peripheral blood monocytes can be classified into three distinct subgroups: Classical monocytes (CD14++CD16-): expressing high levels of CD14 and not expressing CD16; Intermediate monocytes (CD14+CD16+): expressing intermediate levels of both CD14 and CD16; Non-classical monocytes (CD14dimCD16+): expressing low levels of CD14 while expressing high levels of CD16 (52, 53). It is now acknowledged that classical monocytes (CD14++CD16-) mature along a continuum to intermediate monocytes (CD14++CD16+) and then to non-classical monocytes (CD14+CD16++) (54). Compared to the classical CD16- monocytes, the CD16+ subset possesses a stronger ability to secrete pro-inflammatory factors. Therefore, in many inflammatory diseases such as HF and tumors, there is an increase in the number of CD16+ monocytes, playing a role in eliminating damaging factors and protecting tissue cells to some extent (55). Rogacev and colleagues conducted a prospective follow-up study over an average of 4.9 years on 119 patients with chronic kidney disease. They found that the CD14++/CD16+ (intermediate) monocyte subset could predict combined mortality and major cardiovascular events (myocardial infarction, stroke, HF, vascular revascularization), independent of other risk factors, highlighting a significant correlation between the CD14+/CD16+ (intermediate) monocyte subset and the onset of HF (56). However, the article did not mention whether the authors discussed whether this correlation is positive or negative. The intermediate subset may represent a stage of differentiation towards immature classical and non-classical monocyte subsets (57). Benjamin J suggests that in both acute and chronic HF, the quantity and percentage of CD14+ CD16+ monocytes in patients increase, which correlates with the severity of the disease (58). Due to their potent phagocytic ability, CD14+ CD16+ monocytes play a crucial role in clearing apoptotic cells and endogenous ligands within the myocardium (59). Observational studies indicate that an increase in total monocyte count is associated with a poorer prognosis in HF (60). However, a lower count of CD14+ CD16+ monocytes is an independent predictor of increased mortality and repeated hospitalizations (58). This aligns with our conclusion: the percentage of CD14+ CD16+ monocytes among total monocytes is negatively correlated with HF. In terms of specific mechanisms, research has identified that CD14+ CD16+ monocytes possess a distinct gene expression profile, promoting angiogenesis and tissue remodeling through the upregulation of genes such as VCAM-1R (58, 61, 62). It is noteworthy that, although CD14++CD16+ monocytes exhibit dual functionality, being both pro-inflammatory and anti-inflammatory (63, 64), the CD14+ CD16+ intermediate subset effectively mitigates the harmful pro-inflammatory effects of classical and non-classical monocytes. This is achieved through pathways such as the production of interleukin-10 (IL-10), stimulation of angiogenesis, and promotion of tissue cell repair (65, 66).
The adaptive immune system's potential role in HF has been extensively explored, and T cells, crucial immune cells in adaptive immunity and chronic inflammation, participate in the development of HF. After maturing in the thymus, these cells enter the bloodstream and differentiate into two major subsets based on the surface expression of antigens: CD4+ and CD8+ T cells (67). Immunological basic theory research has revealed that the CD4+ subset includes effector T cells, involved in immune responses, and helper T cells, influencing antibody generation in cells. On the other hand, the CD8+ subset comprises suppressor cells, affecting B cell production, and cytotoxic cells, participating in the destruction of target cells (68). The interdependent interactions among cellular subsets in the body either promote or constrain each other, forming an appropriate immune response and maintaining the stability of the body's own immune system. According to the magnetic resonance (MR) results, we found that the proportion of CD39+ CD8+ T cells within CD8+ T cells is a significant factor in the onset of HF (P < 0.05). The CD39 molecule, a significant extracellular nucleotide hydrolase, is broadly expressed on endothelial and diverse immune cell surfaces (lymphocytes, neutrophils, monocytes/macrophages, dendritic cells, regulatory T cells, myeloid-derived suppressor cells, etc.), playing a critical role in immune responses, cell apoptosis, tumor immunity, and lymphocyte activation, emphasizing its crucial significance (69). Joe Yeong analyzed CD8+ T cells from healthy donors and found that only a minority of CD8+ T cells expressed CD39; however, during chronic infection, virus-specific CD8+ T cells exhibited significant CD39 expression. This suggests that CD8+ T cell expression of CD39 is a pathological phenomenon associated with the development of T cell exhaustion (70). Moreover, Mendel's randomized findings reveal a positive association between CD3 expression on Central Memory CD4+ T cells and the onset of HF. Specifically, each additional standard deviation of CD3 on Central Memory CD4+ T cells corresponds to a 2% increase in the risk of HF. Consequently, targeting the modulation of specific T cell subsets could represent a promising approach to enhance cardiac function, potentially slowing.
The early changes in immune cells are closely related to the pathology of HF. This suggests that we can develop more reliable immune-related biomarkers to aid in the early identification and diagnosis of HF. Additionally, various cells in the immune system participate in the body's inflammatory response, with peripheral immune cells such as monocytes, neutrophils, and T cells influencing the progression of central inflammatory responses through the release of pro-inflammatory factors. By modulating or intervening in specific immune cells, such as monocytes, dendritic cells, and T cells, we can slow down the emergence of early pathological features of HF, thus impeding its further development or reversing ventricular remodeling.
5 Strengths and limitations
Compared to previous studies, our study has several strengths. First, using data from immuno- phenotypic exposure factors and population HF studies has helped to mitigate biases arising from reverse causation and confounding factors. Second, we systematically sifted through a broad spectrum of 731 common immune phenotypes, delving deeper into the analysis of 6 exposure factors that exhibited significant causal relationships. Third, the causal relationships identified in our MR analysis could serve as promising candidates for further functional studies of immune phenotypes, contributing to the development of innovative approaches to target specific immune modulations and intervene in overall HF.
There are some limitations to the study we conducted. First, the study population was exclusively composed of individuals of European descent, emphasizing the need to further validate of the causal relationship between immune cells and relevant factors in the overall HF across diverse ethnic groups. Next, further stratified analysis of the population is not possible due to the lack of individual information. Third, the outcome data used in the study were aggregated, and the causal association between immune phenotypes and specific subtypes of HF is still unclear due to the lack of investigation of exposure to categorical diseases. Finally, this study solely assessed the causal association between immune cells and overall HF without delving into specific underlying mechanisms.
6 Conclusion
This study systematically assessed the causal effect of 38 immune cells and related factors on HF using a two-sample MR. The results identified potential causal relationships of specific immune phenotypes, such as Dendritic cell Absolute Count and CD62l- CD86+ Myeloid Dendritic cell, providing novel avenues for therapeutic exploration and insights into the pathogenic mechanisms of HF. However, these findings warrant further experimental validation and subsequent in-depth exploration of molecular mechanisms, laying the foundation for preventive and therapeutic strategies for all-cause HF and the potential discovery of drug targets.
Data availability statement
The original contributions presented in the study are included in the article/supplementary material, further inquiries can be directed to the corresponding authors.
Author contributions
JL: Conceptualization, Investigation, Software, Writing – original draft, Writing – review & editing. LL: Data curation, Methodology, Writing – original draft, Writing – review & editing. QL: Writing – original draft. WZ: Data curation, Methodology, Supervision, Writing – original draft. YZ: Data curation, Methodology, Resources, Supervision, Writing – original draft, Writing – review & editing. WJ: Funding acquisition, Resources, Supervision, Visualization, Writing – original draft, Writing – review & editing.
Funding
The author(s) declare financial support was received for the research, authorship, and/or publication of this article.
This research was provided by the Natural Science Foundation of Jiangsu Province (Grant No. BK 20221422), the Foundation from Jiangsu, Provincial Administration of Traditional Chinese Medicine (Grant No. k2021j17-3 and No. ZT202104).
Conflict of interest
The authors declare that the research was conducted in the absence of any commercial or financial relationships that could be construed as a potential conflict of interest.
Publisher's note
All claims expressed in this article are solely those of the authors and do not necessarily represent those of their affiliated organizations, or those of the publisher, the editors and the reviewers. Any product that may be evaluated in this article, or claim that may be made by its manufacturer, is not guaranteed or endorsed by the publisher.
Supplementary material
The Supplementary Material for this article can be found online at: https://www.frontiersin.org/articles/10.3389/fcvm.2024.1363200/full#supplementary-material
References
1. Greene SJ, Fonarow GC, Butler J. Risk profiles in heart failure: baseline, residual, worsening, and advanced heart failure risk. Circ Heart Fail. (2020) 13:e007132. doi: 10.1161/CIRCHEARTFAILURE.120.007132
2. Roger VL. Epidemiology of heart failure: a contemporary perspective. Circ Res. (2021) 128:1421–34. doi: 10.1161/CIRCRESAHA.121.318172
3. van Riet EES, Hoes AW, Wagenaar KP, Limburg A, Landman MAJ, Rutten FH. Epidemiology of heart failure: the prevalence of heart failure and ventricular dysfunction in older adults over time. A systematic review. Eur J Heart Fail. (2016) 18:242–52. doi: 10.1002/ejhf.483
5. Jones NR, Roalfe AK, Adoki I, Hobbs FDR, Taylor CJ. Survival of patients with chronic heart failure in the community: a systematic review and meta-analysis. Eur J Heart Fail. (2019) 21:1306–25. doi: 10.1002/ejhf.1594
6. Savarese G, Becher PM, Lund LH, Seferovic P, Rosano GMC, Coats AJS. Corrigendum to: global burden of heart failure: a comprehensive and updated review of epidemiology. Cardiovasc Res. (2023) 119:1453. doi: 10.1093/cvr/cvad026
7. Rurik JG, Aghajanian H, Epstein JA. Immune cells and immunotherapy for cardiac injury and repair. Circ Res. (2021) 128:1766–79. doi: 10.1161/CIRCRESAHA.121.318005
8. Ridker PM, Rane M. Interleukin-6 signaling and anti-interleukin-6 therapeutics in cardiovascular disease. Circ Res. (2021) 128:1728–46. doi: 10.1161/CIRCRESAHA.121.319077
9. Steffens S, Van Linthout S, Sluijter JPG, Tocchetti CG, Thum T, Madonna R. Stimulating pro-reparative immune responses to prevent adverse cardiac remodelling: consensus document from the joint 2019 meeting of the ESC working groups of cellular biology of the heart and myocardial function. Cardiovasc Res. (2020) 116:1850–62. doi: 10.1093/cvr/cvaa137
10. Goonewardena SN, Stein AB, Tsuchida RE, Rattan R, Shah D, Hummel SL. Monocyte subsets and inflammatory cytokines in acute decompensated heart failure. J Card Fail. (2016) 22:358–65. doi: 10.1016/j.cardfail.2015.12.014
11. Mann DL. Innate immunity and the failing heart: the cytokine hypothesis revisited. Circ Res. (2015) 116:1254–68. doi: 10.1161/CIRCRESAHA.116.302317
12. Mezzaroma E, Toldo S, Farkas D, Seropian IM, Van Tassell BW, Salloum FN, et al. The inflammasome promotes adverse cardiac remodeling following acute myocardial infarction in the mouse. Proc Natl Acad Sci USA. (2011) 108:19725–30. doi: 10.1073/pnas.1108586108
13. Houssari M, Dumesnil A, Tardif V, Kivelä R, Pizzinat N, Boukhalfa I, et al. Lymphatic and immune cell cross-talk regulates cardiac recovery after experimental myocardial infarction. Arterioscler Thromb Vasc Biol. (2020) 40:1722–37. doi: 10.1161/ATVBAHA.120.314370
14. Bulik-Sullivan BK, Loh P-R, Finucane HK, Ripke S, Yang J, Patterson N, et al. LD Score regression distinguishes confounding from polygenicity in genome-wide association studies. Nat Genet. (2015) 47:291–5. doi: 10.1038/ng.3211
15. Grotzinger AD, Rhemtulla M, de Vlaming R, Ritchie SJ, Mallard TT, Hill WD, et al. Genomic structural equation modelling provides insights into the multivariate genetic architecture of complex traits. Nat Hum Behav. (2019) 3:513–25. doi: 10.1038/s41562-019-0566-x
16. Boef AGC, Dekkers OM, le Cessie S. Mendelian randomization studies: a review of the approaches used and the quality of reporting. Int J Epidemiol. (2015) 44:496–511. doi: 10.1093/ije/dyv071
17. Tang M, Wang T, Zhang X. A review of SNP heritability estimation methods. Brief Bioinform. (2022) 23:bbac067. doi: 10.1093/bib/bbac067
18. Larsson SC, Michaëlsson K, Burgess S. Mendelian randomization in the bone field. Bone. (2019) 126:51–8. doi: 10.1016/j.bone.2018.10.011
19. Harroud A, Richards JB. Mendelian randomization in multiple sclerosis: a causal role for vitamin D and obesity? Mult Scler. (2018) 24:80–5. doi: 10.1177/1352458517737373
20. Xia J, Xie S-Y, Liu K-Q, Xu L, Zhao P-P, Gai S-R, et al. Systemic evaluation of the relationship between psoriasis, psoriatic arthritis and osteoporosis: observational and Mendelian randomisation study. Ann Rheum Dis. (2020) 79:1460–7. doi: 10.1136/annrheumdis-2020-217892
21. Elsworth B, Lyon M, Alexander T, Liu Y, Matthews P, Hallett J, et al. The MRC IEU OpenGWAS Data Infrastructure. BioRxiv. Available online at: https://www.biorxiv.org/content/10.1101/2020.08.10.244293v1 (accessed November 17, 2023).
22. Kurki MI, Karjalainen J, Palta P, Sipilä TP, Kristiansson K, Donner KM, et al. Finngen provides genetic insights from a well-phenotyped isolated population. Nature. (2023) 613:508–18. doi: 10.1038/s41586-022-05473-8
23. Sekula P, Del Greco MF, Pattaro C, Köttgen A. Mendelian randomization as an approach to assess causality using observational data. J Am Soc Nephrol. (2016) 27:3253–65. doi: 10.1681/ASN.2016010098
24. Bowden J, Spiller W, Del Greco MF, Sheehan N, Thompson J, Minelli C, et al. Improving the visualization, interpretation and analysis of two-sample summary data Mendelian randomization via the radial plot and radial regression. Int J Epidemiol. (2018) 47:1264–78. doi: 10.1093/ije/dyy101
25. Burgess S, Thompson SG, CRP CHD Genetics Collaboration. Avoiding bias from weak instruments in Mendelian randomization studies. Int J Epidemiol. (2011) 40:755–64. doi: 10.1093/ije/dyr036
26. Kamat MA, Blackshaw JA, Young R, Surendran P, Burgess S, Danesh J, et al. Phenoscanner V2: an expanded tool for searching human genotype-phenotype associations. Bioinformatics. (2019) 35:4851–3. doi: 10.1093/bioinformatics/btz469
27. Pierce BL, Burgess S. Efficient design for Mendelian randomization studies: subsample and 2-sample instrumental variable estimators. Am J Epidemiol. (2013) 178:1177–84. doi: 10.1093/aje/kwt084
28. Bowden J, Del Greco MF, Minelli C, Davey Smith G, Sheehan NA, Thompson JR. Assessing the suitability of summary data for two-sample Mendelian randomization analyses using MR-egger regression: the role of the I2 statistic. Int J Epidemiol. (2016) 45:1961–74. doi: 10.1093/ije/dyw220
29. Bowden J, Davey Smith G, Burgess S. Mendelian randomization with invalid instruments: effect estimation and bias detection through Egger regression. Int J Epidemiol. (2015) 44:512–25. doi: 10.1093/ije/dyv080
30. Burgess S, Thompson SG. Interpreting findings from Mendelian randomization using the MR-Egger method. Eur J Epidemiol. (2017) 32:377–89. doi: 10.1007/s10654-017-0255-x
31. Xiao G, He Q, Liu L, Zhang T, Zhou M, Li X, et al. Causality of genetically determined metabolites on anxiety disorders: a two-sample Mendelian randomization study. J Transl Med. (2022) 20:475. doi: 10.1186/s12967-022-03691-2
32. Geroldinger A, Lusa L, Nold M, Heinze G. Leave-one-out cross-validation, penalization, and differential bias of some prediction model performance measures-a simulation study. Diagn Progn Res. (2023) 7:9. doi: 10.1186/s41512-023-00146-0
33. Hemani G, Zheng J, Elsworth B, Wade KH, Haberland V, Baird D, et al. The MR-Base platform supports systematic causal inference across the human phenome. Elife. (2018) 7:e34408. doi: 10.7554/eLife.34408
34. Jones DS, Podolsky SH. The history and fate of the gold standard. Lancet. (2015) 385:1502–3. doi: 10.1016/S0140-6736(15)60742-5
35. Millwood IY, Bennett DA, Walters RG, Clarke R, Waterworth D, Johnson T, et al. Lipoprotein-associated phospholipase A2 loss-of-function variant and risk of vascular diseases in 90,000 Chinese adults. J Am Coll Cardiol. (2016) 67:230–1. doi: 10.1016/j.jacc.2015.10.056
36. Birney E. Mendelian randomization. Cold Spring Harb Perspect Med. (2022) 12:a041302. doi: 10.1101/cshperspect.a041302
37. Ling S, Xu J-W. NETosis as a pathogenic factor for heart failure. Oxid Med Cell Longev. (2021) 2021:6687096. doi: 10.1155/2021/6687096
38. Moskalik A, Niderla-Bielińska J, Ratajska A. Multiple roles of cardiac macrophages in heart homeostasis and failure. Heart Fail Rev. (2022) 27:1413–30. doi: 10.1007/s10741-021-10156-z
39. Baci D, Bosi A, Parisi L, Buono G, Mortara L, Ambrosio G, et al. Innate immunity effector cells as inflammatory drivers of cardiac fibrosis. Int J Mol Sci. (2020) 21:7165. doi: 10.3390/ijms21197165
40. Martini E, Kunderfranco P, Peano C, Carullo P, Cremonesi M, Schorn T, et al. Single-cell sequencing of mouse heart immune infiltrate in pressure overload-driven heart failure reveals extent of immune activation. Circulation. (2019) 140:2089–107. doi: 10.1161/CIRCULATIONAHA.119.041694
41. Balan S, Saxena M, Bhardwaj N. Dendritic cell subsets and locations. Int Rev Cell Mol Biol. (2019) 348:1–68. doi: 10.1016/bs.ircmb.2019.07.004
42. Schraml BU, Reis e Sousa C. Defining dendritic cells. Curr Opin Immunol. (2015) 32:13–20. doi: 10.1016/j.coi.2014.11.001
43. Théry C, Amigorena S. The cell biology of antigen presentation in dendritic cells. Curr Opin Immunol. (2001) 13:45–51. doi: 10.1016/s0952-7915(00)00180-1
44. Steinman RM. The dendritic cell system and its role in immunogenicity. Annu Rev Immunol. (1991) 9:271–96. doi: 10.1146/annurev.iy.09.040191.001415
45. Watanabe M, Fujihara C, Radtke AJ, Chiang YJ, Bhatia S, Germain RN, et al. Co-stimulatory function in primary germinal center responses: CD40 and B7 are required on distinct antigen-presenting cells. J Exp Med. (2017) 214:2795–810. doi: 10.1084/jem.20161955
46. Kretzschmar D, Betge S, Windisch A, Pistulli R, Rohm I, Fritzenwanger M, et al. Recruitment of circulating dendritic cell precursors into the infarcted myocardium and pro-inflammatory response in acute myocardial infarction. Clin Sci (Lond). (2012) 123:387–98. doi: 10.1042/CS20110561
47. Lee GR. The balance of Th17 versus treg cells in autoimmunity. Int J Mol Sci. (2018) 19:730. doi: 10.3390/ijms19030730
48. Pathak M, Sharma P, Sharma A, Verma M, Srivastava M, Misra-Bhattacharya S. Regulatory T-cell neutralization in mice during filariasis helps in parasite clearance by enhancing T helper type 17-mediated pro-inflammatory response. Immunology. (2016) 147:190–203. doi: 10.1111/imm.12550
49. Zhou Y, Leng X, Luo S, Su Z, Luo X, Guo H, et al. Tolerogenic dendritic cells generated with tofacitinib ameliorate experimental autoimmune encephalomyelitis through modulation of Th17/treg balance. J Immunol Res. (2016) 2016:5021537. doi: 10.1155/2016/5021537
50. Athanassopoulos P, Vaessen LMB, Maat APWM, Balk AHMM, Weimar W, Bogers AJJC. Peripheral blood dendritic cells in human end-stage heart failure and the early post-transplant period: evidence for systemic Th1 immune responses. Eur J Cardiothorac Surg. (2004) 25:619–26. doi: 10.1016/j.ejcts.2004.01.032
51. Duncan SE, Gao S, Sarhene M, Coffie JW, Linhua D, Bao X, et al. Macrophage activities in myocardial infarction and heart failure. Cardiol Res Pract. (2020) 2020:4375127. doi: 10.1155/2020/4375127
52. Collin M, Bigley V. Monocyte, macrophage, and dendritic cell development: the human perspective. Microbiol Spectr. (2016) 4(5):MCHD-0015-2015. doi: 10.1128/microbiolspec.MCHD-0015-2015
53. Ziegler-Heitbrock L, Ancuta P, Crowe S, Dalod M, Grau V, Hart DN, et al. Nomenclature of monocytes and dendritic cells in blood. Blood. (2010) 116:e74–80. doi: 10.1182/blood-2010-02-258558
54. Stirrat CG, Alam SR, MacGillivray TJ, Gray CD, Dweck MR, Raftis J, et al. Ferumoxytol-enhanced magnetic resonance imaging assessing inflammation after myocardial infarction. Heart. (2017) 103:1528–35. doi: 10.1136/heartjnl-2016-311018
55. Hristov M, Weber C. Differential role of monocyte subsets in atherosclerosis. Thromb Haemost. (2011) 106:757–62. doi: 10.1160/TH11-07-0500
56. Rogacev KS, Cremers B, Zawada AM, Seiler S, Binder N, Ege P, et al. CD14++CD16+ monocytes Independently predict cardiovascular events: a cohort study of 951 patients referred for elective coronary angiography. J Am Coll Cardiol. (2012) 60:1512–20. doi: 10.1016/j.jacc.2012.07.019
57. Connaughton EP, Naicker S, Hanley SA, Slevin SM, Eykelenboom JK, Lowndes NF, et al. Phenotypic and functional heterogeneity of human intermediate monocytes based on HLA-DR expression. Immunol Cell Biol. (2018). doi: 10.1111/imcb.12032
58. Wrigley BJ, Shantsila E, Tapp LD, Lip GYH. CD14++CD16+ monocytes in patients with acute ischaemic heart failure. Eur J Clin Invest. (2013) 43:121–30. doi: 10.1111/eci.12023
59. Heine GH, Ortiz A, Massy ZA, Lindholm B, Wiecek A, Martínez-Castelao A, et al. Monocyte subpopulations and cardiovascular risk in chronic kidney disease. Nat Rev Nephrol. (2012) 8:362–9. doi: 10.1038/nrneph.2012.41
60. Vaduganathan M, Greene SJ, Butler J, Sabbah HN, Shantsila E, Lip GYH, et al. The immunological axis in heart failure: importance of the leukocyte differential. Heart Fail Rev. (2013) 18:835–45. doi: 10.1007/s10741-012-9352-9
61. Wrigley BJ, Shantsila E, Tapp LD, Lip GYH. Increased expression of cell adhesion molecule receptors on monocyte subsets in ischaemic heart failure. Thromb Haemost. (2013) 110:92–100. doi: 10.1160/TH13-02-0088
62. Zawada AM, Rogacev KS, Rotter B, Winter P, Marell R-R, Fliser D, et al. SuperSAGE evidence for CD14++CD16+ monocytes as a third monocyte subset. Blood. (2011) 118:e50–61. doi: 10.1182/blood-2011-01-326827
63. Rossol M, Kraus S, Pierer M, Baerwald C, Wagner U. The CD14(bright) CD16+ monocyte subset is expanded in rheumatoid arthritis and promotes expansion of the Th17 cell population. Arthritis Rheum. (2012) 64:671–7. doi: 10.1002/art.33418
64. Azeredo EL, Neves-Souza PC, Alvarenga AR, Reis SRNI, Torrentes-Carvalho A, Zagne S-MO, et al. Differential regulation of toll-like receptor-2, toll-like receptor-4, CD16 and human leucocyte antigen-DR on peripheral blood monocytes during mild and severe dengue fever. Immunology. (2010) 130:202–16. doi: 10.1111/j.1365-2567.2009.03224.x
65. Shantsila E, Wrigley B, Tapp L, Apostolakis S, Montoro-Garcia S, Drayson MT, et al. Immunophenotypic characterization of human monocyte subsets: possible implications for cardiovascular disease pathophysiology. J Thromb Haemost. (2011) 9:1056–66. doi: 10.1111/j.1538-7836.2011.04244.x
66. Eljaszewicz A, Bolkun L, Grubczak K, Rusak M, Wasiluk T, Dabrowska M, et al. Very small embryonic-like stem cells, endothelial progenitor cells, and different monocyte subsets are effectively mobilized in acute lymphoblastic leukemia patients after G-CSF treatment. Stem Cells Int. (2018) 2018:1943980. doi: 10.1155/2018/1943980
67. Bradshaw AD, DeLeon-Pennell KY. T-cell regulation of fibroblasts and cardiac fibrosis. Matrix Biol. (2020) 91–92:167–75. doi: 10.1016/j.matbio.2020.04.001
68. Go RR, Hm K, Cj D, As S, Mm W, Am R-R, et al. Fundamentals of T cell metabolism and strategies to enhance cancer immunotherapy. Front Immunol. (2021) 12:645242. doi: 10.3389/fimmu.2021.645242
69. Timperi E, Barnaba V. CD39 regulation and functions in T cells. Int J Mol Sci. (2021) 22:8068. doi: 10.3390/ijms22158068
Keywords: immune cell, all-cause heart failure, Mendelian randomization, single nucleotide polymorphism, causal effect
Citation: Li J, Liu L, Luo Q, Zhou W, Zhu Y and Jiang W (2024) Exploring the causal relationship between immune cell and all-cause heart failure: a Mendelian randomization study. Front. Cardiovasc. Med. 11:1363200. doi: 10.3389/fcvm.2024.1363200
Received: 21 January 2024; Accepted: 6 June 2024;
Published: 13 June 2024.
Edited by:
Dragos Cretoiu, Carol Davila University of Medicine and Pharmacy, RomaniaReviewed by:
Amirmohammad Khalaji, Tehran University of Medical Sciences, IranDebashish Dey, Vidyasagar University, India
© 2024 Li, Liu, Luo, Zhou, Zhu and Jiang. This is an open-access article distributed under the terms of the Creative Commons Attribution License (CC BY). The use, distribution or reproduction in other forums is permitted, provided the original author(s) and the copyright owner(s) are credited and that the original publication in this journal is cited, in accordance with accepted academic practice. No use, distribution or reproduction is permitted which does not comply with these terms.
*Correspondence: Yao Zhu, zy910113@njucm.edu.cn; Weimin Jiang, jwm0410@njucm.edu.cn