- 1Department of Cardiovascular Medicine, First Affiliated Hospital of Gannan Medical University, Ganzhou, Jiangxi, China
- 2National Heart Research Institute Singapore, National Heart Centre Singapore, Singapore, Singapore
- 3Duke-NUS Medical School, Singapore, Singapore
- 4Department of Endocrinology, Singapore General Hospital, Singapore, Singapore
- 5KK Research Centre, KK Women’s and Children’s Hospital, Singapore, Singapore
- 6Department of Cardiology, The Second Affiliated Hospital of Nanchang University, Nanchang, Jiangxi, China
- 7Department of Radiology, Mayo Clinic, Rochester, MN, United States
Background: Pulmonary artery (PA) strain is associated with structural and functional alterations of the vessel and is an independent predictor of cardiovascular events. The relationship of PA strain to metabolomics in participants without cardiovascular disease is unknown.
Methods: In the current study, community-based older adults, without known cardiovascular disease, underwent simultaneous cine cardiovascular magnetic resonance (CMR) imaging, clinical examination, and serum sampling. PA global longitudinal strain (GLS) analysis was performed by tracking the change in distance from the PA bifurcation to the pulmonary annular centroid, using standard cine CMR images. Circulating metabolites were measured by cross-sectional targeted metabolomics analysis.
Results: Among n = 170 adults (mean age 71 ± 6.3 years old; 79 women), mean values of PA GLS were 16.2 ± 4.4%. PA GLS was significantly associated with age (β = −0.13, P = 0.017), heart rate (β = −0.08, P = 0.001), dyslipidemia (β = −2.37, P = 0.005), and cardiovascular risk factors (β = −2.49, P = 0.001). Alanine (β = −0.007, P = 0.01) and proline (β = −0.0009, P = 0.042) were significantly associated with PA GLS after adjustment for clinical risk factors. Medium and long-chain acylcarnitines were significantly associated with PA GLS (C12, P = 0.027; C12-OH/C10-DC, P = 0.018; C14:2, P = 0.036; C14:1, P = 0.006; C14, P = 0.006; C14-OH/C12-DC, P = 0.027; C16:3, P = 0.019; C16:2, P = 0.006; C16:1, P = 0.001; C16:2-OH, P = 0.016; C16:1-OH/C14:1-DC, P = 0.028; C18:1-OH/C16:1-DC, P = 0.032).
Conclusion: By conventional CMR, PA GLS was associated with aging and vascular risk factors among a contemporary cohort of older adults. Metabolic pathways involved in PA stiffness may include gluconeogenesis, collagen synthesis, and fatty acid oxidation.
1 Introduction
Similar to the aorta, the pulmonary artery (PA) is susceptible to vascular remodeling against a background of age- and risk factor-related insults over time (1–3). In the PA, age-related vascular remodeling increases PA pressure due to decreased vascular compliance (2, 4, 5), leading to diseases such as pulmonary arterial hypertension (PAH) and heart failure.
While age-associated increases in PA pressure and diameter are well appreciated, PA stiffness in aging is rarely characterized. PA stiffness has been largely reported among clinical cardiovascular disease cohorts, utilizing advanced techniques such as invasive hemodynamics (6) or surrogate measures via echocardiogram (7–9). These methods are inherently disadvantageous for understanding PA stiffness in non-disease cohorts as they are invasive, variable—due to short transit time in the pulmonary trunk—or rely on complicated phase-contrast imaging, making them impractical for community-based studies. Among aging cohorts that may have unstable renal function, non-contrast-enhanced conventional cardiovascular magnetic resonance (CMR) appears advantageous over other techniques.
PA stiffness is a key disturbance associated with poor outcomes in both left and right heart diseases (10–13). Underscoring the important mechanical relationship between the PA and right ventricle (RV), the movement of the pulmonary valve plane arising from RV contraction causes longitudinal movement of the PA. Together with the expansibility of the artery, longitudinal movement contributes to PA deformation over a cardiac cycle. As the PA is mechanically connected to the RV, assessing PA stiffness across the longitudinal length of the PA may be useful for understanding diseases of the right heart (10, 13). This might be especially important in the absence of elevated pulmonary pressures, which calls for more sensitive tools to ascertain the state of PA stiffness before hemodynamic pressures rise.
Evidence-based therapies for the field of pulmonary hypertension remain less developed compared to therapeutics for left heart diseases, such as left ventricular heart failure. A key barrier lies in insufficient mechanistic understanding of the pathogenesis of pulmonary arterial diseases at the molecular level in human cohorts. Mapping molecular signals to sensitive quantitative measures of arterial properties can potentially facilitate exploratory interrogation of the complex vascular biology mechanisms that underpin the development of symptomatic vascular disease. The feasibility of this approach is supported by mechanistic studies that have shown changes in circulating metabolic intermediates in subjects with asymptomatic arterial stiffness (14, 15) as well as in subjects with PAH (16, 17). Whether disruptions in metabolic pathways, as indicated by metabolic intermediates, are observed in the pulmonary vasculature remains under-investigated.
With these considerations in mind, we proposed a method for assessing PA global longitudinal strain (GLS) based on CMR feature tracking methods and demonstrated significant correlations between PA GLS and surrogates of PA stiffness, including PA relative area change and pulse wave velocity (PWV) (18). Analogous to GLS of the ascending aorta as a measure of aortic stiffness (19), we further hypothesize that PA GLS obtained by this method may be associated with clinical factors such as age and vascular risk factors that alter PA stiffness. Guided by our prior work that detected associations between vascular stiffness and targeted metabolites in the acylcarnitines and related pathways, we studied serum metabolomics in conjunction with PA GLS to discover key metabolic pathways involved in PA stiffness.
2 Materials and methods
2.1 Study population
One hundred and seventy subjects were recruited from the Cardiac Aging Study, a prospective study initiated in 2014 that examines characteristics and determinants of cardiovascular function in older adults (20) without known cardiovascular disease. All participants had no self-reported history of physician-diagnosed cardiovascular diseases (such as coronary heart disease, atrial fibrillation), stroke, or cancer. The study adhered to the principles outlined in the Declaration of Helsinki, and the SingHealth Centralised Institutional Review Board approved the study protocol. Participants provided written informed consent upon enrollment.
All participants were examined and interviewed during a single study visit. They completed a standardized questionnaire that included medical history and coronary risk factors. Hypertension was defined by the current use of antihypertensive drugs or physician-diagnosed hypertension. Diabetes mellitus was defined by the current use of anti-diabetic agents or physician-diagnosed diabetes mellitus. Dyslipidemia was defined by the current use of lipid-lowering agents or physician-diagnosed dyslipidemia. Smoking history was categorized as ever smokers (former or current smoking) or never smokers. Sinus rhythm status was ascertained through a resting electrocardiogram. Clinical data were obtained on the same day as the assessment of CMR imaging and serum collection.
A validated non-exercise prediction model was employed to estimate peak oxygen uptake, VO2 (ml/kg/min) (21). The physical activity questionnaire included the frequency of exercise, length of time, and intensity for each workout. This model is closely linked to specific measures of cardiovascular structure and function (22).
2.2 CMR acquisition
Cine CMR scans were performed using a balanced fast field echo sequence. All subjects were imaged on a 3 T magnetic resonance imaging system (Ingenia, Philips Healthcare, The Netherlands). The CMR acquisition protocol and typical parameters were published previously (23). Standard end-expiratory breath-hold cine images were obtained using a steady-state free precession pulse sequence, retrospective electrocardiographic gating, and a typical temporal resolution of 30 frames per cardiac cycle. These images were acquired in the PA bifurcation, RV outflow tract (RVOT), coronal RVOT, and RV 3-chamber views (Figure 1A). Additionally, end-expiratory breath-hold cine images were acquired in multi-planar long-axis views, including 2-, 3-, and 4-chamber views.
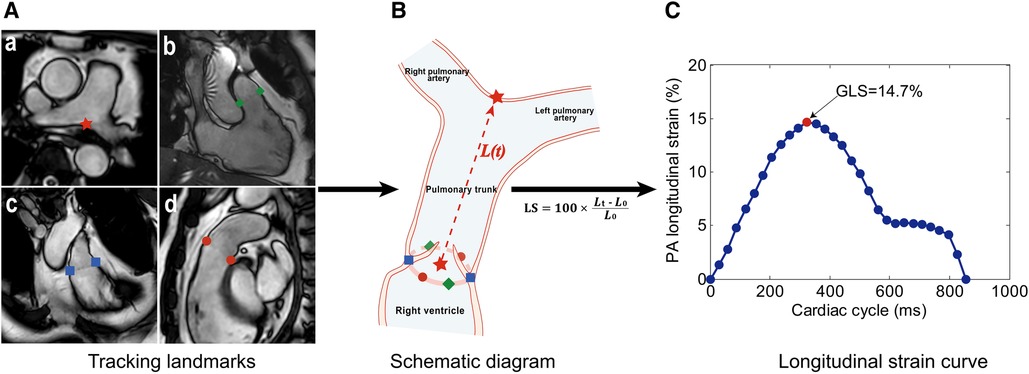
Figure 1. Measurement method of pulmonary artery (PA) global longitudinal strain (GLS). (A) Landmark tracking on the PA bifurcation (a, red star), right ventricular (RV) 3-chamber (b, green diamonds), coronal RV outflow tract (RVOT) (c, blue squares), and RVOT (d, red dots) views. (B) Schematic diagram of feature tracking. The trajectory of the centroid (red star) derived from six pulmonary annular points was calculated. The distance [L(t), the red dotted line] between two red stars was automatically tracked throughout the cardiac cycle in 3D space. (C) Longitudinal strain (LS) curve. LS at a time point (t) relative to the initial time point (t = 0) at end-diastole was calculated as 100 × (Lt – L0)/L0, and the PA GLS was defined as the maximal absolute strain value.
2.3 PA global longitudinal strain
PA GLS was assessed by one operator (H.Z.Z.) using an in-house semi-automatic algorithm (18) while remaining blinded to the clinical characteristics of participants and other CMR measurements. The motion of the PA bifurcation and the pulmonary valve annulus was automatically tracked over the cardiac cycle in PA bifurcation, RVOT, coronal RVOT, and RV 3-chamber views (Figure 1A). The trajectory of the centroid derived from six pulmonary annular points was obtained. The distance (L) from PA bifurcation to the centroid was calculated throughout the cardiac cycle. Longitudinal strain at a time point (t) relative to the initial time point (time 0) at end-diastole was calculated as 100 × (Lt–L0)/L0 (Figure 1B), and the PA GLS was defined as the maximal absolute strain value (Figure 1C). Details on the measurement of PA GLS can be found in the Supplementary Material.
Reproducibility was assessed in 20 randomly selected subjects. Inter-observer variability was evaluated by two independent operators (H.Z.Z. & S.L.), while intra-observer variability was assessed twice, with an interval of one month, by the first operator (H.Z.Z.). Correlation and Bland-Altman analyses were conducted to investigate intra- and inter-observer agreement.
2.4 RV function and global longitudinal strain
RV function was assessed by cardiologists blinded to other CMR measurements. The endocardium of the RV was automatically tracked on short-axis cine images at end-systole and end-diastole to obtain RV end-diastolic volume (EDV) and end-systolic volume (ESV), and to calculate stroke volume (SV) and ejection fraction (EF). Using cine 4-chamber CMR images, the RV endocardium was tracked by one reader (X.D.Z.), who was blinded to all participant characteristics. RV GLS was automatically obtained using dedicated, validated QStrain software (Version 2.0, Medis BV, Leiden, The Netherlands).
2.5 Metabolomic profiling
2.5.1 Blood collection and serum processing
Antecubital venous blood samples (20–30 ml) were collected in the morning from consenting participants; fasting was not required. After collection, the blood samples were immediately placed on ice for transportation and processed within 6 h to obtain serum samples. The serum metabolomic profiling analysis was conducted at the Duke-NUS Metabolomics Facility.
2.5.2 Targeted metabolomics profiling
Serum samples (50 μl) were spiked with a 10 μl deuterium labelled amino acid mixture and diluted with 400 μl methanol. After centrifugation of the mixture at 17,000 g for 5 min at 4°C, the supernatant fraction (10 μl) was collected for amino acid analysis. A pooled quality control sample was prepared by mixing equal amounts (10 μl) of each extracted serum sample. Extraction and measurement of amino acid panels (quantified in units of μM) were performed as previously described (24). Analysis was done on the MultiQuant™ 3.0.3 software (AB Sciex, DC, USA). For acylcarnitines, serum samples (100 μl) were similarly prepared as the amino acid analysis, but were instead spiked with 20 μl deuterium-labelled acyl-carnitine mixture and diluted with 800 µl methanol. Extraction and measurement of acyl-carnitine were performed as previously described (25). Data acquisition and analysis were conducted on an Agilent MassHunter Workstation B.06.00 Software.
During initial data analysis, standard analytical chemistry procedures were employed to assess data quality, including accuracy and precision. Accuracy was determined through low and high concentration quality control runs against the standard calibration curve. A sample of the pooled biologic quality control sample was measured repeatedly during the sample run to detect drift in the signal and assess precision. Additionally, the coefficient of variation was evaluated for each analyte in the pooled biologic quality control runs. Analytes with a coefficient of variation greater than 20% were excluded from further interpretation.
2.6 Statistical analysis
Clinical characteristics are presented as mean and standard deviation (SD) for continuous data and frequency and percentage for categorical data. Cardiovascular risk factor 2 (CVRF2) was defined as the presence of any two or more cardiovascular risk factors (hypertension, dyslipidemia, ever smoked).
The association between PA GLS and clinical risk factors was assessed using linear regression. All clinical risk factors that showed an association with PA GLS at P < 0.05 in univariate analysis were entered into a multiple linear regression analysis that related PA GLS to significant clinical risk factors, adjusting for metabolite effects.
The identification of metabolites associated with PA GLS occurred in two steps. First, simple linear regression with PA GLS was conducted to identify significant metabolites (P < 0.05). Second, multiple linear regression was performed on each metabolite associated with PA GLS (P < 0.05 in univariate analysis), adjusting for the effects of identified significant clinical risk factors.
Subjects were assigned to groups with higher or lower than average PA GLS—i.e., “high PA GLS” and “low PA GLS”, respectively—based on sex-specific mean values (16.3% for females and 16.1% for males). A heatmap, visualizing the normalized intensities of metabolites, was generated using Mass Profiler Professional software (Agilent, USA).
All statistical analyses were conducted using STATA 15 (College Station, Texas, USA). For all analyses, a two-tailed P-value of <0.05 was considered significant.
3 Results
We analyzed 170 participants (mean ± SD age 71 ± 6.3 years; 79 women) to obtain PA GLS (mean ± SD 16.2 ± 4.4%). The most common associated comorbidities of participants were hypertension (51.8%), diabetes mellitus (48.2%), dyslipidemia (20%), and smoking (18.2%). Additionally, 25.3% of participants had two or more risk factors (hypertension, dyslipidemia, ever smoked) (Table 1). All participants were in New York Heart Association Class I and were in sinus rhythm. Mean VO2 levels were 34 ± 5.9 ml/kg/min for the whole cohort, 37.7 ml/kg/min for men, and 29.9 ml/kg/min for women.
PA GLS analysis was successfully performed in all subjects. The intra- and inter-observer correlation coefficients were r = 0.94 and r = 0.91, respectively, while the intra- and inter-observer coefficients of variation were 4.4% and 5.0%, respectively (Supplementary Figure S1).
Univariate linear regression was performed on PA GLS with clinical parameters as dependent variables (Table 2). Age, heart rate, dyslipidemia, smoking, and CVRF2 were negatively associated with PA GLS. Additionally, RV EDV index (β = 0.10, P = 0.001) and RV ESV index (β = 0.12, P = 0.002) showed positive associations with PA GLS. However, there was no significant correlation between RVEF or RV GLS and PA GLS. PA GLS correlated with aerobic capacity among women (r = 0.31, P = 0.0049), but not among men (r = 0.038, P = 0.723).
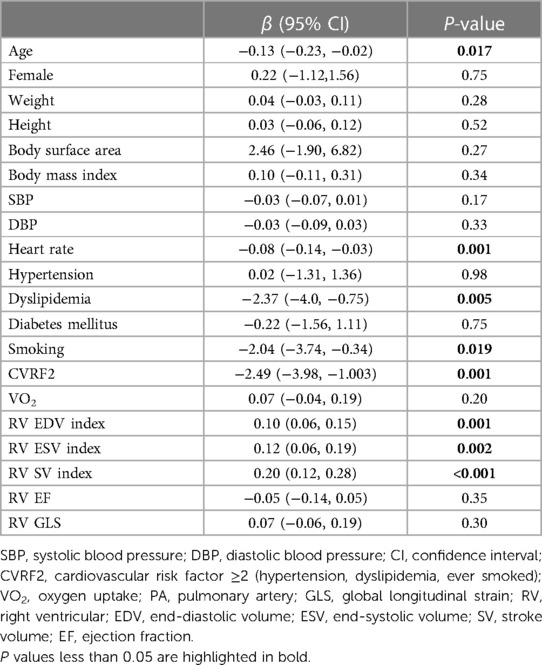
Table 2. Summary of univariate linear regression coefficients for PA GLS regressed on demographic and clinical variables.
We analyzed 86 metabolites, including 69 acylcarnitines and 17 amino acid metabolites. The list of measured metabolites is presented in Supplementary Table S1.
Linear regression analysis revealed associations between amino acids and PA GLS (Table 3). In univariate analysis, PA GLS was associated with alanine (β = −0.009, P = 0.001) and proline (β = −0.01, P = 0.008). Other amino acids showed no association with PA GLS. In multiple regression analysis between individual amino acids and PA GLS, adjusting for prior clinical covariates, both alanine and proline remained significantly associated with PA GLS.
We then conducted linear regression analysis between PA GLS and acylcarnitines (Table 4). Negative correlations were observed between C2, C4-OH, C12:1, C12, C12-OH/C10-DC, C14:3, C14:2, C14:1, C14, C14-OH/C12-DC, C16:3, C16:2, C16:1, C16:3-OH/C14:3-DC, C16:2-OH, C16:1-OH/C14:1-DC, C18:1-OH/C16:1-DC, C18-OH/C16-DC, C20:1-OH/C18:1-DC, C20-OH/C18-DC, and PA GLS. Multiple linear regression, adjusting for clinical covariates, was performed on PA GLS with significant acylcarnitines as predictor variables (Table 4). Medium and long-chain acylcarnitines remained significantly associated with PA GLS (C12, P = 0.027; C12-OH/C10-DC, P = 0.018; C14:2, P = 0.036; C14:1, P = 0.006; C14, P = 0.006; C14-OH/C12-DC, P = 0.027; C16:3, P = 0.019; C16:2, P = 0.006; C16:1, P = 0.001; C16:2-OH, P = 0.016; C16:1-OH/C14:1-DC, P = 0.028; C18:1-OH/C16:1-DC, P = 0.032).
A heatmap was generated to examine metabolite patterns in subjects with high vs. low PA GLS (Figure 2). Subjects with low PA GLS (indicative of high PA stiffness) exhibited higher levels of long-chain acylcarnitines, as evident from the increased prevalence of red for this class of metabolites on the heat map.
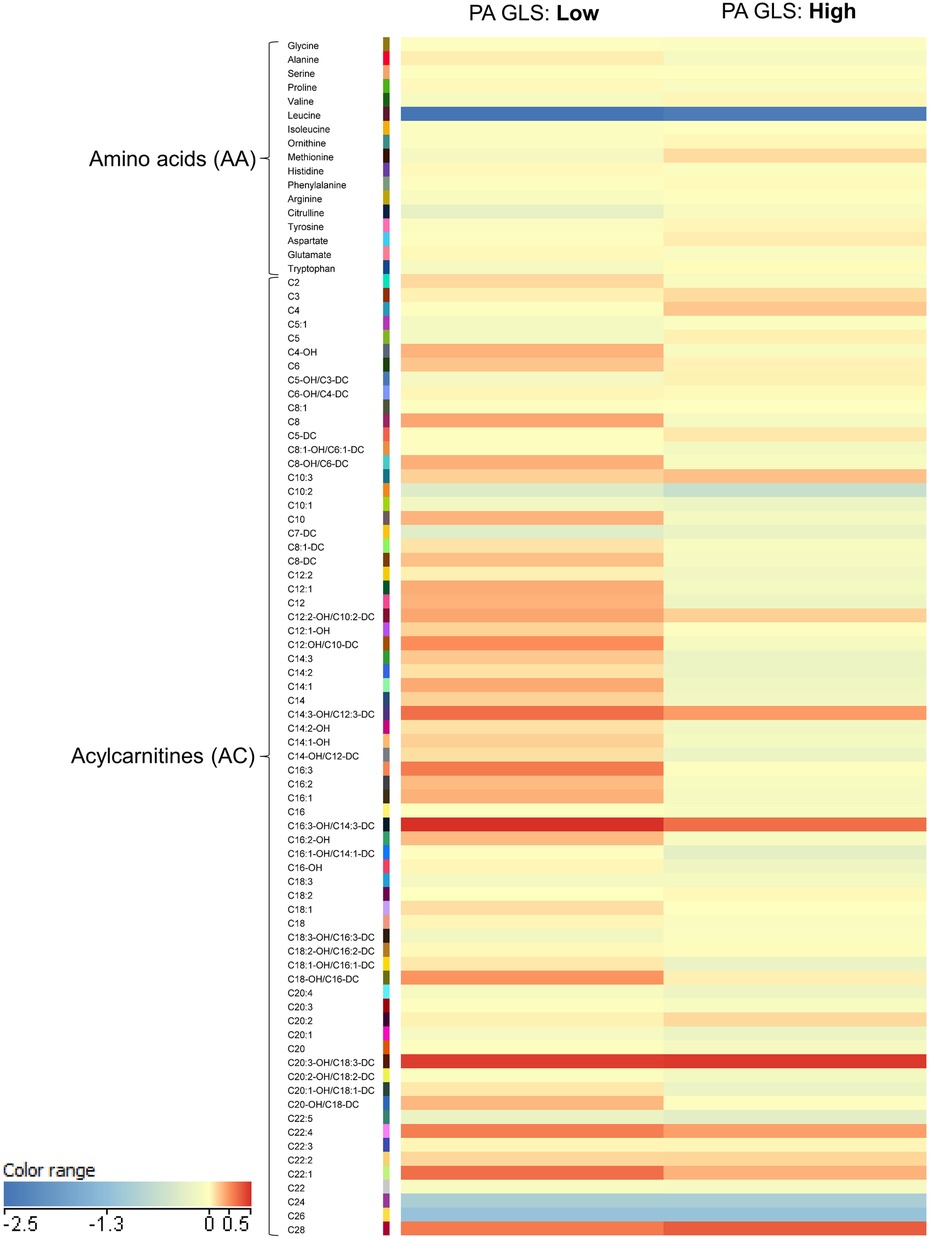
Figure 2. Heatmap illustrating metabolomics results in plot illustrating mean levels of key acylcarnitine.
We quantified levels of key metabolites that differed between participants with high vs. low PA strain (Figure 3). Participants with low PA GLS had higher levels of C12, C12-OH/C10-DC, C14, C14:1, C14:2, C14-OH/C12-DC, C16:2, C16:3, C16:1-OH/C14:1-DC, and C18:1-OH/C16:1-DC compared to participants with high PA GLS (P < 0.05 for all).
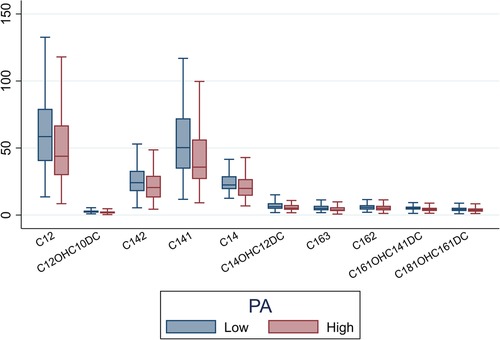
Figure 3. Box plot illustrating mean levels of key acylcarnitine metabolites that differentiate between high PA strain and low PA strain (P < 0.05 for all). PA, pulmonary artery.
4 Discussion
PA GLS was evaluated as a novel method for assessing PA stiffness using conventional cine CMR images. Decreased PA GLS was characterized by specific clinical factors and metabolites among older adults. PA GLS was independently associated with aging, elevated heart rate, dyslipidemia, smoking, long-chain acylcarnitines, and amino acid metabolites.
4.1 PA GLS and clinical risk factors
This study lays the groundwork for future clinical applications of PA GLS in assessing PA stiffness. The correlation between PA GLS and clinical cardiovascular risk factors aligns with previous studies on PA stiffness (11, 26).
Age has been positively associated with PA PWV (11) and PA pressures (2, 5) in healthy subjects. Similarly, our study demonstrated that, like PWV and pressure, PA GLS decreased with age in a community cohort of older adults, indicating that aging is associated with longitudinal length changes in the PA.
Elevated heart rate is another risk factor associated with adverse cardiovascular events (27). In a porcine model, PA pressure was found to increase with heart rate, which was attributed to increase in flow and/or downstream flow resistance (28). A study of patients with pulmonary hypertension showed positive linear relationship between mean PA pressure and heart rate (29). Similar effects of heart rate on atrial distensibility were observed in earlier studies (30–32). PA distensibility was lower among patients with left heart disease and pulmonary hypertension vs. no pulmonary hypertension, and negatively correlated with heart rate (33). PA stiffness, assessed by the pulse pressure to stroke volume index ratio, has been shown to increase with heart rate (r2 = 0.15), resulting in an increased risk of incident idiopathic PAH (26). Our study demonstrated an inverse association between PA GLS and heart rate (r = −0.24), which is consistent with the literature.
Smoking and dyslipidemia are crucial atherogenic risk factors. Smokers with chronic obstructive pulmonary disease often exhibit extensive atherosclerosis, leading to arterial stiffness (34, 35). Studies have shown that cigarette smoke induces increased wall stiffness in the PA of rats (36). Additionally, arterial stiffness has been directly associated with a 7-year increase in high-density lipoprotein, low-density lipoprotein, and triglycerides (37). Our study found that smoking and dyslipidemia were negatively associated with PA GLS, despite a small number of participants being at risk for dyslipidemia due to smoking.
4.2 PA GLS and metabolites
We integrated advanced CMR imaging with serum metabolomic signals to evaluate clinical features of PA stiffness and metabolite patterns among this aging cohort. GLS assessed stiffness representing the PA trunk, while serum metabolomics distinguished older adults with high vs. low PA stiffness. In our study, we observed an association between PA GLS and circulating amino acids and acylcarnitines.
Both alanine and proline were negatively associated with PA GLS in both univariate analysis and multivariable analysis adjusted for clinical CVRF2. Alanine, a non-essential amino acid, is strongly linked to glucose metabolism. It plays a prominent role in the glucose-alanine shuttle between muscle and liver. A small percentage of individuals older than 85 years and the overall population have reported echocardiographic signs suggestive of pulmonary hypertension, indicating elevated PA stiffness (38, 39). Studies have shown that alanine is positively correlated with the pulmonary arterial medial thickness index—a histologic marker of PAH (16), with higher levels in blood samples from the PA of systemic sclerosis patients with PAH (17). Increased alanine levels in these studies were attributed to reduced tissue alanine aminotransferase levels (17). Proline contributes to pulmonary arterial remodeling in PAH rats (40). Rafikova et al. found high circulating levels of alanine and proline in early-stage PAH patients (41).
Long-chain acylcarnitines increase with age in healthy individuals (42). Our previous study showed that medium- and long-chain acylcarnitines were independently associated with arterial stiffness (20), similar to left atrial strain patterns obtained via CMR (23). This aligns with other studies in patients with pulmonary vascular disorders that observed higher levels of circulating long-chain acylcarnitines in patients with PAH, including idiopathic PAH, chronic thromboembolic pulmonary hypertension, and pulmonary hypertension associated with left heart disease (43, 44). By demonstrating an association between long-chain acylcarnitines and PA strain in otherwise healthy adults, our observations suggest that such a metabolic underpinning may be present upstream in the absence of disease. These findings may support broader validation in other non-disease cohorts, providing strategies for screening or charting pulmonary vascular diseases.
Overall, our integrated data provide a fresh approach to phenotyping human cohorts with omics (45), allowing more in-depth characterization of pulmonary arterial function. In addition to omics data, other strengths of our study include the prospective nature of participant recruitment, reducing biases related to recall while facilitating the simultaneous acquisition of clinical, CMR, and metabolomics data. Our study was prespecified to characterize older adults without clinical cardiovascular disease; therefore, circulating biomarkers represent the aging process rather than disease processes.
4.3 Limitations
This study has limitations. Firstly, we did not acquire velocity-encoded flow measurements at the pulmonary trunk in this study, and are thus neither able to determine pulmonary vascular resistance noninvasively nor perform computational hemodynamic modeling for estimation of PA pressure. Secondly, compared to the current targeted approach, an untargeted metabolomics approach employing more metabolites may broaden the metabolic maps associated with PA GLS. While our targeted approach allowed precise quantification of identified metabolites, untargeted approaches could uncover pathways represented by these metabolites. Thirdly, all measurements and clinical variables originated from a single cross-sectional evaluation. Future studies may find it interesting to associate metabolomic findings at baseline with prospective changes in PA GLS and outcome data. Fourthly, while we did not explicitly exclude participants with preexisting respiratory disease, the calculated VO2 measurements of participants enrolled into our current study were generally within normal ranges, which suggests that the impact of clinically significant respiratory disease is likely to be low in this community cohort. Fifthly, the effect of physical activity on cardiorespiratory status impacting PA GLS measurements is uncertain in the studied subjects, although aerobic capacity was generally satisfactory among the subjects. Lastly, non-fasting serum samples (used in our study) may introduce analytic differences compared to other cohorts that use fasting samples. However, our practice aligns with other cohort studies that found fasting did not contribute to variability in most metabolite measurements (46).
5 Conclusions
Using conventional CMR, PA GLS was associated with aging and vascular risk factors among a contemporary cohort of older adults. Metabolic pathways involved in PA stiffness may include glucogenesis, collagen synthesis, and fatty acid oxidation. Among asymptomatic older adults, PA GLS may serve as a novel risk stratification tool to identify early metabolic perturbations associated with pulmonary dysfunction.
Data availability statement
The original contributions presented in the study are included in the article/Supplementary Material, further inquiries can be directed to the corresponding authors
Ethics statement
The studies involving humans were approved by SingHealth Centralised Institutional Review Board. The studies were conducted in accordance with the local legislation and institutional requirements. The participants provided their written informed consent to participate in this study.
Author contributions
HZ: Formal Analysis, Investigation, Methodology, Writing – original draft. SL: Formal Analysis, Investigation, Methodology, Writing – review & editing. FG: Formal Analysis, Investigation, Methodology, Writing – review & editing. J-PK: Formal Analysis, Investigation, Writing – review & editing. HW: Formal Analysis, Investigation, Writing – review & editing. KC: Formal Analysis, Investigation, Writing – review & editing. JC: Formal Analysis, Investigation, Writing – review & editing. JA: Formal Analysis, Writing – review & editing. XZ: Formal Analysis, Writing – review & editing. R-ST: Investigation, Writing – review & editing. QW: Investigation, Supervision, Writing – review & editing. TL: Investigation, Writing – review & editing. AK: Conceptualization, Data curation, Formal Analysis, Funding acquisition, Investigation, Methodology, Project administration, Supervision, Writing – review & editing. LZ: Conceptualization, Data curation, Formal Analysis, Funding acquisition, Investigation, Methodology, Project administration, Supervision, Writing – review & editing.
Funding
The author(s) declare that financial support was received for the research, authorship, and/or publication of this article.
The Cardiac Aging Study has received funding support from the National Medical Research Council of Singapore (MOH-000153 and NMRC/OFIRG/0018/2016), Hong Leong Foundation, Duke-NUS Medical School, Estate of Tan Sri Khoo Teck Puat and SingHealth Foundation. HZ is supported by Graduate Research and Innovation Projects of Jiangxi Province, China (NO. YC2020-B055).
Conflict of interest
The authors declare that the research was conducted in the absence of any commercial or financial relationships that could be construed as a potential conflict of interest.
The author(s) declared that they were an editorial board member of Frontiers, at the time of submission. This had no impact on the peer review process and the final decision.
Publisher's note
All claims expressed in this article are solely those of the authors and do not necessarily represent those of their affiliated organizations, or those of the publisher, the editors and the reviewers. Any product that may be evaluated in this article, or claim that may be made by its manufacturer, is not guaranteed or endorsed by the publisher.
Supplementary material
The Supplementary Material for this article can be found online at: https://www.frontiersin.org/articles/10.3389/fcvm.2024.1346443/full#supplementary-material
References
1. Horvat D, Zlibut A, Orzan RI, Cionca C, Muresan ID, Mocan T, et al. Aging influences pulmonary artery flow and stiffness in healthy individuals: non-invasive assessment using cardiac MRI. Clin Radiol. (2021) 76:161.e19–e28. doi: 10.1016/j.crad.2020.09.021
2. Lam CS, Borlaug BA, Kane GC, Enders FT, Rodeheffer RJ, Redfield MM. Age-associated increases in pulmonary artery systolic pressure in the general population. Circulation. (2009) 119:2663–70. doi: 10.1161/CIRCULATIONAHA.108.838698
3. Mahfouz RA, Dewedar A, Abdelmoneim A, Hossien EM. Aortic and pulmonary artery stiffness and cardiac function in children at risk for obesity. Echocardiography. (2012) 29:984–90. doi: 10.1111/j.1540-8175.2012.01736.x
4. Kawut SM, Barr RG, Lima JA, Praestgaard A, Johnson WC, Chahal H, et al. Right ventricular structure is associated with the risk of heart failure and cardiovascular death: the multi-ethnic study of atherosclerosis (MESA)–right ventricle study. Circulation. (2012) 126:1681–8. doi: 10.1161/CIRCULATIONAHA.112.095216
5. van Empel VP, Kaye DM, Borlaug BA. Effects of healthy aging on the cardiopulmonary hemodynamic response to exercise. Am J Cardiol. (2014) 114:131–5. doi: 10.1016/j.amjcard.2014.04.011
6. Swift AJ, Rajaram S, Condliffe R, Capener D, Hurdman J, Elliot C, et al. Pulmonary artery relative area change detects mild elevations in pulmonary vascular resistance and predicts adverse outcome in pulmonary hypertension. Invest Radiol. (2012) 47:571–7. doi: 10.1097/RLI.0b013e31826c4341
7. Görgülü S, Eren M, Yildirim A, Ozer O, Uslu N, Celik S, et al. A new echocardiographic approach in assessing pulmonary vascular bed in patients with congenital heart disease: pulmonary artery stiffness. Anadolu Kardiyol Derg. (2003) 3:92–7.
8. Karacaglar E, Bal U, Eroglu S, Colak A, Bozbas S, Muderrisoglu H. Pulmonary artery distensibility is worsened in obstructive sleep apnea syndrome. Acta Cardiol Sin. (2019) 35:501–7. doi: 10.6515/ACS.201909_35(5).20190424A
9. Yenercag M, Arslan U, Dereli S, Coksevim M, Dogdus M, Kaya A. Effects of angiotensin receptor neprilysin inhibition on pulmonary arterial stiffness in heart failure with reduced ejection fraction. Int J Cardiovasc Imaging. (2021) 37:165–73. doi: 10.1007/s10554-020-01973-8
10. Altıparmak İH, Erkuş ME, Polat M, Yalçın F, Sak ZH, Sezen H, et al. Relation of elastic properties of pulmonary artery with left ventricular abnormalities and aortic stiffness in patients with moderate to severe obstructive sleep apnea: a cross-sectional echocardiographic study. Turk Kardiyol Dern Ars. (2016) 44:289–99. doi: 10.5543/tkda.2015.67862
11. Dawes TJ, Gandhi A, de Marvao A, Buzaco R, Tokarczuk P, Quinlan M, et al. Pulmonary artery stiffness is independently associated with right ventricular mass and function: a cardiac MR imaging study. Radiology. (2016) 280:398–404. doi: 10.1148/radiol.2016151527
12. Fourie PR, Coetzee AR, Bolliger CT. Pulmonary artery compliance: its role in right ventricular-arterial coupling. Cardiovasc Res. (1992) 26:839–44. doi: 10.1093/cvr/26.9.839
13. Singh I, Rahaghi FN, Naeije R, Oliveira RKF, Systrom DM, Waxman AB. Right ventricular-arterial uncoupling during exercise in heart failure with preserved ejection fraction: role of pulmonary vascular dysfunction. Chest. (2019) 156:933–43. doi: 10.1016/j.chest.2019.04.109
14. Anderson SG, Sanders TA, Cruickshank JK. Plasma fatty acid composition as a predictor of arterial stiffness and mortality. Hypertension. (2009) 53:839–45. doi: 10.1161/HYPERTENSIONAHA.108.123885
15. Menni C, Mangino M, Cecelja M, Psatha M, Brosnan MJ, Trimmer J, et al. Metabolomic study of carotid-femoral pulse-wave velocity in women. J Hypertens. (2015) 33:791–6. doi: 10.1097/HJH.0000000000000467
16. Izquierdo-Garcia JL, Arias T, Rojas Y, Garcia-Ruiz V, Santos A, Martin-Puig S, et al. Metabolic reprogramming in the heart and lung in a murine model of pulmonary arterial hypertension. Front Cardiovasc Med. (2018) 5:110. doi: 10.3389/fcvm.2018.00110
17. Deidda M, Piras C, Cadeddu Dessalvi C, Locci E, Barberini L, Orofino S, et al. Distinctive metabolomic fingerprint in scleroderma patients with pulmonary arterial hypertension. Int J Cardiol. (2017) 241:401–6. doi: 10.1016/j.ijcard.2017.04.024
18. Zhong L, Leng S, Alabed S, Chai P, Teo L, Ruan W, et al. Pulmonary artery strain predicts prognosis in pulmonary arterial hypertension. J Am Coll Cardiol Img. (2023) 16:1022–34. doi: 10.1016/j.jcmg.2023.02.007
19. Bell V, Mitchell WA, Sigurðsson S, Westenberg JJ, Gotal JD, Torjesen AA, et al. Longitudinal and circumferential strain of the proximal aorta. J Am Heart Assoc. (2014) 3:e001536. doi: 10.1161/JAHA.114.001536
20. Koh AS, Gao F, Liu J, Fridianto KT, Ching J, Tan RS, et al. Metabolomic profile of arterial stiffness in aged adults. Diab Vasc Dis Res. (2018) 15:74–80. doi: 10.1177/1479164117733627
21. Nes BM, Janszky I, Vatten LJ, Nilsen TI, Aspenes ST, Wisloff U. Estimating V.O 2peak from a nonexercise prediction model: the HUNT study, Norway. Med Sci Sports Exerc. (2011) 43:2024–30. doi: 10.1249/MSS.0b013e31821d3f6f
22. Koh AS, Gao F, Tan RS, Zhong L, Leng S, Zhao X, et al. Metabolomic correlates of aerobic capacity among elderly adults. Clin Cardiol. (2018) 41:1300–7. doi: 10.1002/clc.23016
23. Koh AS, Gao F, Leng S, Kovalik JP, Zhao X, Tan RS, et al. Dissecting clinical and metabolomics associations of left atrial phasic function by cardiac magnetic resonance feature tracking. Sci Rep. (2018) 8:8138. doi: 10.1038/s41598-018-26456-8
24. Kovalik JP, Zhao X, Gao F, Leng S, Chow V, Chew H, et al. Amino acid differences between diabetic older adults and non-diabetic older adults and their associations with cardiovascular function. J Mol Cell Cardiol. (2021) 158:63–71. doi: 10.1016/j.yjmcc.2021.05.009
25. Gao F, Kovalik JP, Zhao X, Chow VJ, Chew H, Teo LL, et al. Exacerbation of cardiovascular ageing by diabetes mellitus and its associations with acyl-carnitines. Aging. (2021) 13:14785–805. doi: 10.18632/aging.203144
26. Chemla D, Weatherald J, Lau EMT, Savale L, Boucly A, Attal P, et al. Clinical and hemodynamic correlates of pulmonary arterial stiffness in incident, untreated patients with idiopathic pulmonary arterial hypertension. Chest. (2018) 154:882–92. doi: 10.1016/j.chest.2018.06.015
27. Woodward M, Webster R, Murakami Y, Barzi F, Lam TH, Fang X, et al. The association between resting heart rate, cardiovascular disease and mortality: evidence from 112,680 men and women in 12 cohorts. Eur J Prev Cardiol. (2014) 21:719–26. doi: 10.1177/2047487312452501
28. Kornet L, Jansen JR, Nijenhuis FC, Langewouters GJ, Versprille A. The compliance of the porcine pulmonary artery depends on pressure and heart rate. J Physiol. (1998) 512:917–26. doi: 10.1111/j.1469-7793.1998.917bd.x
29. Chemla D, Castelain V, Hoette S, Creuzé N, Provencher S, Zhu K, et al. Strong linear relationship between heart rate and mean pulmonary artery pressure in exercising patients with severe precapillary pulmonary hypertension. Am J Physiol Heart Circ Physiol. (2013) 305:H769–77. doi: 10.1152/ajpheart.00258.2013
30. Spronck B, Tan I, Reesink KD, Georgevsky D, Delhaas T, Avolio AP, et al. Heart rate and blood pressure dependence of aortic distensibility in rats: comparison of measured and calculated pulse wave velocity. J Hypertens. (2021) 39:117–26. doi: 10.1097/HJH.0000000000002608
31. Mangoni AA, Mircoli L, Giannattasio C, Ferrari AU, Mancia G. Heart rate-dependence of arterial distensibility in vivo. J Hypertens. (1996) 14:897–901. doi: 10.1097/00004872-199607000-00013
32. Marcus RH, Korcarz C, McCray G, Neumann A, Murphy M, Borow K, et al. Noninvasive method for determination of arterial compliance using Doppler echocardiography and subclavian pulse tracings. Validation and clinical application of a physiological model of the circulation. Circulation. (1994) 89:2688–99. doi: 10.1161/01.cir.89.6.2688
33. Colin GC, Verlynde G, Pouleur AC, Gerber BL, Beauloye C, Kefer J, et al. Pulmonary hypertension due to left heart disease: diagnostic value of pulmonary artery distensibility. Eur Radiol. (2020) 30:6204–12. doi: 10.1007/s00330-020-06959-7
34. Iwamoto H, Yokoyama A, Kitahara Y, Ishikawa N, Haruta Y, Yamane K, et al. Airflow limitation in smokers is associated with subclinical atherosclerosis. Am J Respir Crit Care Med. (2009) 179:35–40. doi: 10.1164/rccm.200804-560OC
35. Sato S, Shimizu K, Ito T, Tsubono M, Ogawa A, Sasaki T, et al. Increased arterial stiffness in chronic thromboembolic pulmonary hypertension was improved with riociguat and balloon pulmonary angioplasty: a case report. Int Med Case Rep J. (2021) 14:191–7. doi: 10.2147/IMCRJ.S303997
36. Liu SQ, Fung YC. Material coefficients of the strain energy function of pulmonary arteries in normal and cigarette smoke-exposed rats. J Biomech. (1993) 26:1261–9. doi: 10.1016/0021-9290(93)90350-n
37. Agbaje AO, Barker AR, Mitchell GF, Tuomainen TP. Effect of arterial stiffness and carotid intima-media thickness progression on the risk of dysglycemia, insulin resistance, and dyslipidemia: a temporal causal longitudinal study. Hypertension. (2022) 79:667–78. doi: 10.1161/HYPERTENSIONAHA.121.18754
38. Hoeper MM, Humbert M, Souza R, Idrees M, Kawut SM, Sliwa-Hahnle K, et al. A global view of pulmonary hypertension. Lancet Respir Med. (2016) 4:306–22. doi: 10.1016/S2213-2600(15)00543-3
39. Moreira EM, Gall H, Leening MJ, Lahousse L, Loth DW, Krijthe BP, et al. Prevalence of pulmonary hypertension in the general population: the rotterdam study. PLoS One. (2015) 10:e0130072. doi: 10.1371/journal.pone.0130072
40. Zheng HK, Zhao JH, Yan Y, Lian TY, Ye J, Wang XJ, et al. Metabolic reprogramming of the urea cycle pathway in experimental pulmonary arterial hypertension rats induced by monocrotaline. Respir Res. (2018) 19:94. doi: 10.1186/s12931-018-0800-5
41. Rafikova O, Meadows ML, Kinchen JM, Mohney RP, Maltepe E, Desai AA, et al. Metabolic changes precede the development of pulmonary hypertension in the monocrotaline exposed rat lung. PLoS One. (2016) 11:e0150480. doi: 10.1371/journal.pone.0150480
42. Jarrell ZR, Smith MR, Hu X, Orr M, Liu KH, Quyyumi AA, et al. Plasma acylcarnitine levels increase with healthy aging. Aging. (2020) 12:13555–70. doi: 10.18632/aging.103462
43. Heresi GA, Mey JT, Bartholomew JR, Haddadin IS, Tonelli AR, Dweik RA, et al. Plasma metabolomic profile in chronic thromboembolic pulmonary hypertension. Pulm Circ. (2020) 10:1–11. doi: 10.1177/2045894019890553
44. Luo N, Craig D, Ilkayeva O, Muehlbauer M, Kraus WE, Newgard CB, et al. Plasma acylcarnitines are associated with pulmonary hypertension. Pulm Circ. (2017) 7:211–8. doi: 10.1086/690554
45. Koh AS, Kovalik JP. Metabolomics and cardiovascular imaging: a combined approach for cardiovascular ageing. ESC Heart Fail. (2021) 8:1738–50. doi: 10.1002/ehf2.13274
Keywords: pulmonary artery, metabolomics, ageing, cardiovascular magnetic resonance, strain
Citation: Zhang H, Leng S, Gao F, Kovalik J-P, Wee HN, Chua KV, Ching J, Allen JC, Zhao X, Tan R-S, Wu Q, Leiner T, Koh AS and Zhong L (2024) Characteristics of pulmonary artery strain assessed by cardiovascular magnetic resonance imaging and associations with metabolomic pathways in human ageing. Front. Cardiovasc. Med. 11:1346443. doi: 10.3389/fcvm.2024.1346443
Received: 29 November 2023; Accepted: 20 February 2024;
Published: 29 February 2024.
Edited by:
Ernst Wellnhofer, Berlin Technical University of Applied Sciences, GermanyReviewed by:
Vedant Arun Gupta, University of Kentucky, United StatesNilda Espinola-Zavaleta, National Institute of Cardiology Ignacio Chavez, Mexico
© 2024 Zhang, Leng, Gao, Kovalik, Wee, Chua, Ching, Allen, Zhao, Tan, Wu, Leiner, Koh and Zhong. This is an open-access article distributed under the terms of the Creative Commons Attribution License (CC BY). The use, distribution or reproduction in other forums is permitted, provided the original author(s) and the copyright owner(s) are credited and that the original publication in this journal is cited, in accordance with accepted academic practice. No use, distribution or reproduction is permitted which does not comply with these terms.
*Correspondence: Qinghua Wu ncwqh@163.com Angela S. Koh angela.koh.s.m@singhealth.com.sg Liang Zhong zhong.liang@nhcs.com.sg
†These authors have contributed equally to this work and share first authorship
‡These authors have contributed equally to this work and share senior authorship
Abbreviations CMR, cardiovascular magnetic resonance; CVRF2, cardiovascular risk factor ≥2 (hypertension, dyslipidemia, ever smoked); EDV, end-diastolic volume; EF, ejection fraction; ESV, end-systolic volume; GLS, global longitudinal strain; PA, pulmonary artery; PAH, pulmonary arterial hypertension; PWV, pulse wave velocity; RV, right ventricle; RVOT, RV outflow tract; SD, standard deviation; SV, stroke volume.