- 1CAG Center for Endotheliomics, Copenhagen University Hospital, Rigshospitalet, Copenhagen, Denmark
- 2Department of Cardiology, Copenhagen University Hospital, Rigshospitalet, Copenhagen, Denmark
- 3The Novo Nordisk Foundation Center for Biosustainability, Technical University of Denmark, Kgs. Lyngby, Denmark
- 4Department of Clinical Immunology, Copenhagen University Hospital, Rigshospitalet, Copenhagen, Denmark
Introduction: Pulmonary hypertension (PH) is a pathological condition that affects approximately 1% of the population. The prognosis for many patients is poor, even after treatment. Our knowledge about the pathophysiological mechanisms that cause or are involved in the progression of PH is incomplete. Additionally, the mechanism of action of many drugs used to treat pulmonary hypertension, including sotatercept, requires elucidation.
Methods: Using our graph-powered knowledge mining software Lifelike in combination with a very small patient metabolite data set, we demonstrate how we derive detailed mechanistic hypotheses on the mechanisms of PH pathophysiology and clinical drugs.
Results: In PH patients, the concentration of hypoxanthine, 12(S)-HETE, glutamic acid, and sphingosine 1 phosphate is significantly higher, while the concentration of L-arginine and L-histidine is lower than in healthy controls. Using the graph-based data analysis, gene ontology, and semantic association capabilities of Lifelike, led us to connect the differentially expressed metabolites with G-protein signaling and SRC. Then, we associated SRC with IL6 signaling. Subsequently, we found associations that connect SRC, and IL6 to activin and BMP signaling. Lastly, we analyzed the mechanisms of action of several existing and novel pharmacological treatments for PH. Lifelike elucidated the interplay between G-protein, IL6, activin, and BMP signaling. Those pathways regulate hallmark pathophysiological processes of PH, including vasoconstriction, endothelial barrier function, cell proliferation, and apoptosis.
Discussion: The results highlight the importance of SRC, ERK1, AKT, and MLC activity in PH. The molecular pathways affected by existing and novel treatments for PH also converge on these molecules. Importantly, sotatercept affects SRC, ERK1, AKT, and MLC simultaneously. The present study shows the power of mining knowledge graphs using Lifelike’s diverse set of data analytics functionalities for developing knowledge-driven hypotheses on PH pathophysiological and drug mechanisms and their interactions. We believe that Lifelike and our presented approach will be valuable for future mechanistic studies of PH, other diseases, and drugs.
1 Introduction
Pulmonary hypertension (PH) is a condition that is defined by a mean pulmonary arterial pressure of more than 20 mmHg at rest (1). The range of genetic, molecular, and humoral causes that can lead to PH is extensive. PH is classified into five different groups based on clinical and pathological findings as well as therapeutic interventions (2, 3), namely: Pulmonary arterial hypertension (PAH), PH associated with left heart disease (PH-LHD), lung disease (PH-LD), pulmonary arterial obstructions (CTEPH), and PH with unclear and/or multifactorial mechanisms (2, 4, 5). PH is a condition that affects people of all ages, with a global prevalence of 1%. However, it is more common in individuals over the age of 65. PH is characterized by the remodeling of distal pulmonary arteries, causing a progressive increase in vascular resistance. Vascular remodeling is associated with alterations in vasoconstriction, pulmonary artery-endothelial cell (PAEC), and -smooth muscle cell (PASMC) proliferation, inflammation, apoptosis, angiogenesis, and thrombosis. Some of the lesions found in PH are plexiform lesions, which are characterized by enhanced endothelial cell (EC) proliferation, thrombotic lesions, and the formation of a layer of myofibroblasts, and extracellular matrix between the endothelium and the external elastic lamina (6, 7). One of the first triggers for the development of PH is thought to be EC injury triggering the activation of cellular signaling pathways that are not completely understood (8). When activated, the endothelium secretes different growth factors and cytokines that affect EC and SMC proliferation, apoptosis, coagulation, attract inflammatory cells, and/or affect vasoactivity to restore homeostasis. Prolonged or chronic activation of the endothelium leads to EC dysfunction, and loss of homeostatic functions (9, 10).
The physiological changes associated with PH are inherently difficult to study in vivo. Limited studies have been carried out on PH patients undergoing lung transplantation, analyzing tissue available in biorepositories, or studying the lungs of deceased patients (11). Animal models and cultured cells are used extensively in the study of PH. A common approach is to use cultured human cells, including PAECs, PASMCs, and aortic ECs together with animal models. Human ECs are cultivated on plastic with a stiffness five orders of magnitude greater than living tissue (12), exposed to growth media with viscoelastic properties that are different from those of blood (13), and without hemodynamic shear stress (12). These conditions perturb mechanotransduction and cytoskeletal organization preventing the development of the EC phenotypes found in the human body. Recently, the use of lung-on-a-chip technologies has overcome many of the limitations of human EC cultures (14, 15). However, even when using these technologies, the results are general and the physiological conditions of a PH patient may differ from those used in a study.
We recently introduced a novel framework for studying the EC phenotypes of PH patients building on a genome-scale metabolic model (GEM) of the EC which was parameterized by the plasma metabolome from PH patients (16). We investigated 35 patients with iPAH (n=12), CTD-aPAH (n=12) and CTEPH (n=11) and found significant metabolic differences between the patients. These differences suggest that different disease mechanisms may be involved in each patient. Metabolite concentration measurements constitute a minimally invasive way to understand the physiological state of patients. However, interpreting these results is challenging due to the complex inter-connectivity of molecular networks within cells. Our goal is to identify the relevant biological mechanisms that are active in one patient, or a group of patients based on their metabolic profile. This is very difficult due to the abundance of data and information available in relevant databases and literature. Since Watson, Crick, Franklin, and Wilkins solved the three-dimensional structure of DNA in 1953 (17), advances in genomics (18–21), RNA sequencing (22), single-cell transcriptomics (23), proteomics (24), metabolomics (25), epigenetics (26), and electronic medical or health records (27) have lead to the creation of a vast amount of biological data. This vast ocean of biological information that grows faster every day, presents many opportunities for the advancement of biology and medicine (28). However, extracting valuable insights from diverse information sources is very challenging. The obstacles that must be overcome include finding the molecular mechanisms that help us explain the causes for the correlations that we can observe, and enriching the data obtained from a novel research effort with relevant preexisting data. Lifelike (29) was developed to help researchers integrate different sources of data, and to explore the molecular mechanisms that help explain experimental observations. In the current study, we use Lifelike to associate experimental data with existing knowledge about PH allowing us to propose a model of the molecular mechanisms involved in the pathophysiology of PH. This model allowed us to explore how the differentially expressed metabolites described in (16), might be connected to the physiological changes associated with PH.
2 Materials and methods
2.1 The data and knowledge mining platform Lifelike
Lifelike is a software platform for advanced analytics of biological data and knowledge management (29). Lifelike uses a knowledge graph (KG) which consolidates data from several public data sources into a unified single graph database comprising 97,855,162 nodes connected by 162,654,084 edges. The underlying database system is ArangoDB. We have integrated the ontologies Medical Subject Headings (MeSH; https://www.nlm.nih.gov/mesh/meshhome.html) (30) and Gene Ontology (GO) (31, 32), genes and associated taxonomies from NCBI (33, 34), proteins from UniProt (35) and protein-protein interactions from StringDB (36), enzymes from EXPASY (37), chemicals from ChEBI (38), as well as a biomedical data set comprising chemical-gene, chemical-disease, gene-disease and gene-gene relationships derived from text analysis of Medline abstracts (39). Several conceptually different analyses can be performed on Lifelike’s KG as detailed below. A subset of Lifelike’s capabilities is available via a public portal https://public.lifelike.bio/ upon self-registration. A guide on how to use Lifelike and its data analytics functionalities can be found in the user options panel of the software (bottom left button). Lifelike source code is available at https://github.com/SBRG/lifelike.
2.1.1 Annotation of biological entities in text data
Lifelike’s rule-based entity recognition system recognizes and highlights eight biological entity types plus user-specified custom annotations. The following entities are detected in text by default: gene, protein, disease, chemical/compound, phenotype/phenomena, anatomy, food, and species. It was utilized to annotate biological entities in text from portable document format (pdf) files and enrichment tables. The detected entities were used for further downstream analysis, e.g., statistical GO enrichment and semantic analysis.
2.1.2 Enrichment tables
Enrichment tables compile information from Lifelike’s knowledge graph into a single table based on a user’s input data set. Using a set of gene names (e.g., from a list of the genes that appear in an article) as an input, Lifelike retrieves information from its knowledge graph resources NCBI, UniProt, StringDB, and Gene Ontology, and combines the textual descriptions from each of the databases along with the respective URL in an enrichment table. Enrichment tables were inspected manually or automatically by semantic analysis using Lifelike’s word cloud functionality.
2.1.3 Statistical gene ontology enrichment
Statistical Gene Ontology (GO) enrichment analysis is used to associate a group of genes with their biological functions. GO enrichment was performed directly from Lifelike’s enrichment tables. GO terms were downloaded from https://www.geneontology.org/docs/downloads/ on 10/08/2020. Fisher’s exact test was used to identify GO terms that are statistically enriched in an input gene list. The False Discovery Rate was controlled by the Benjamini-Hochberg procedure (40) and an adjusted p-value (q-value) smaller than the significance level of 0.05 was considered statistically significant.
2.1.4 Semantic analysis
The semantic analysis makes use of Lifelike’s text entity recognition system. It can be used on annotated pdf files or enrichment tables. First, enrichment tables were generated using a list of genes as input (e.g., genes obtained from initial radiate analysis). Then, the textual descriptions from Lifelike’s KG are analyzed to detect and extract biological entities, which include genes, that are semantically associated with the input (see Section 2.1.1). The extracted genes were used for several downstream analyses, either for statistical GO enrichment or as an input for another iteration of enrichment table generation and semantic analysis.
2.1.5 Semantic entity clouds
Entity clouds are a facile way to grasp higher concepts from text quickly. They were generated in Lifelike from pdf files and enrichment tables. Biological entities (e.g., proteins, compounds, etc.) were automatically detected in text, counted (either in the entire table or row-wise), and visually displayed as word clouds. The higher the count of an entity, the larger its size is in the entity cloud. These are especially useful for identifying associated chemicals, phenotypes, proteins, and genes, which can be used to create enrichment tables.
2.1.6 Generation of knowledge maps
Knowledge maps provide a visual storyboard of results, incorporating elements such as images, links to related maps, PDFs, enrichment tables, graphical files, office documents, and biological entities. It is also possible to drag annotated entities from enrichment tables or annotated pdf files onto a map and search across the literature. Results from data analyses were summarized on knowledge maps to capture, interpret, and contextualize data analysis results and the knowledge gained. The elements on a knowledge map were linked to their origins, i.e., to URLs of enrichment tables, pdf, and other files in Lifelike, which were saved as attributes of a knowledge map element.
2.2 Graph data science
We conducted knowledge mining utilizing graph algorithms on a modified version of the graph database Reactome, which is a manually curated, peer-reviewed pathway database that is open-source, and open-access (41, 42). Reactome provides detailed information on biological pathways, which can also be downloaded as a graph database. Moreover, Reactome offers tools and APIs that facilitate the extraction and conversion of pathway data into formats compatible with graph databases and network analysis tools. The pathways in Reactome are manually curated and peer-reviewed by experts, ensuring the provision of high-quality and accurate information. It also boasts a user-friendly interface with tools that are easy to navigate for pathway visualization and data analysis, complementing the data analysis and visualization capabilities we have developed in Lifelike. The graph data science (GDS) code contains libraries that allow us to find molecules that affect and are affected by certain molecules and then analyze their connections in a graph database. Source code for graph data science is available at https://github.com/SBRG/GDS-Public/tree/main. Different graph algorithms were run (detailed below in Sections 2.2.3, 2.2.4), including radiate analysis (Radiate analysis notebook) with subsequent generation of Sankey visualizations (Radiate traces notebook) and all shortest paths (Shortest path notebook).
2.2.1 Construction of the Reactome graph database
The Reactome database (41, 42) was downloaded as a graph database (https://reactome.org/download-data version 75). A series of database queries was used to generate a database version suitable for graph data science which can be followed in detail in the provided Jupyter notebook (Reactome processing notebook). Briefly, nodes, labels, and relationships not required for graph algorithmic analyses were removed. For instance, this included nodes like person, affiliation, and taxa as well as all nodes representing entities of organisms other than Homo sapiens. Sub-cellular locations (compartments) of biological entities were set as node properties. To allow for improved graph traversal, selected relationships were reversed or added. Because currency metabolites, e.g., ATP, NAD(P)H and , can artificially connect metabolic reactions and pathways in network analyses (43, 44) we labeled such compounds plus the regulatory protein ubiquitin accordingly (Currency nodes) and thereby excluded them from all analyses. Finally, the database was transformed into an ArangoDB graph database consisting of 1,703,054 nodes and 3,368,926 edges, available at (29). Furthermore, we allowed only 12 selected edge types in the database to be traversed by the graph algorithms (Supplementary Table S1).
2.2.2 Finding the correct nodes for GDS analysis
Graph algorithms require finding specific molecules in the graph. Source and/or target node sets (e.g., 6 differential metabolites from pulmonary hypertension patients) were found in the graph using Reactome standard identifiers (stId; obtained from the Reactome website: https://reactome.org). The Reactome database models metabolites and other node types depending on their subcellular location (multiple cellular compartments are possible). Unless stated otherwise, metabolite nodes were found in the Reactome graph by selecting the extracellular compartment which allows the investigation of the effect of extracellular plasma metabolites on cellular entities like receptors and downstream effectors.
2.2.3 All shortest paths
To calculate all possible shortest paths between a source and a target node set, we developed a custom Python script that uses NetworkX’s all_shortest_paths method (https://networkx.org/documentation/stable/reference/algorithms/generated/networkx.algorithms.shortest_paths.generic.all_shortest_paths.html#all-shortest-paths). Shortest path calculations were performed either on an unweighted or a weighted network (for weighting details see Section 2.2.4). In unweighted networks, a shortest path between a source and a target node is defined as the path with the minimum number of edges connecting them. In weighted networks, a shortest path between a source and a target node is defined as the path with the minimum sum of edge weights along a path connecting them. This analysis yielded files that were manually uploaded to Lifelike for interactive visualization and detailed analysis.
2.2.4 Radiate analysis and tracing
To measure the importance of network nodes with respect to an input data set, we used a two-step approach which we termed “Radiate analysis and tracing.” This approach is an exploratory graph analysis method where only one input data set is used. First, ranking of network nodes with respect to an input data set is achieved using personalized Pagerank (PPR) (45, 46). PPR has been used previously for the analysis of biological networks including protein-protein interaction and metabolic (47) as well as gene-regulatory networks (48, 49). An input data set used as sources (e.g., differential metabolites) was mapped on the graph and PPRs of network nodes were calculated considering the edge directionality of the network. Forward radiate analysis uses the edge directionality in the network as is and answers the question “Which nodes in the network are highly influenced by the source input data set?.” In reverse radiate analysis, the directions of all edges in the graph were temporarily reversed before PPR calculation. It answers the question “Which nodes in the network have the highest influence over the source input data set?.” We used the NetworkX implementation of Pagerank (NetworkX documentation) which allows for personalization of the algorithm using a user-defined source data set. The obtained probability distribution was normalized such that the sum of all Pageranks in the network (including the source nodes) equals 1. This step returned a ranked list of network nodes for each forward and reverse radiate analysis where the Pagerank of a node measures its importance concerning the input data set. Second, trace graphs from source nodes to selected target nodes identified by radiate analysis were generated. This allowed us to visualize and understand the detailed connectivity of source nodes used as algorithmic input to target nodes identified by radiate analysis. Trace graphs were generated using either all shortest paths on the unweighted network (see above) or paths of highest influence on a weighted network. The weighting of each edge in the network was done using the inverse of the maximum PPR from one of the two nodes connected by the edge. Paths of highest influence were found by calculating all shortest paths on the weighted network. The shortest paths contain the smallest number of edges while the paths of highest influence have the minimum sum of inverse PPR values. This step yielded a Sankey file which is uploaded to Lifelike for detailed analysis and interpretation.
2.3 Experimental design
The individual data analytics functionalities of Lifelike can be combined in various ways to build mechanistic knowledge from input data sets as shown in Figure 1. In the present study, we followed an analysis workflow as depicted in Supplementary Figure S1 with the aim of generating deep knowledge from a comparatively small set of input data. Our analysis workflow started with a radiate analysis using the 6 differential metabolites as input. We performed both forward and reverse radiate analysis, to identify network nodes that are highly relevant with respect to the metabolite input data set. We mainly focused on highly relevant protein nodes identified using forward radiate analysis, unless stated otherwise. To understand and interpret the connectivity of metabolites to identified network nodes (e.g., proteins), we generated interactive Sankey diagrams, where each path can be inspected in a very detailed manner by the user. Lifelike enrichment tables were generated using lists of highly affected genes. Enrichment tables allow us to perform two downstream analysis tasks: semantic and statistical enrichment analysis. Semantic analysis yielded word clouds highlighting different biological entity types which are highly mentioned in the associated enrichment tables. Statistical enrichment analysis against gene ontology terms identified biological processes affected by the 6 plasma metabolites. To further expand the generated knowledge, semantic analysis was performed iteratively. All analysis results, including interpretations and knowledge gained, were summarized on Lifelike knowledge maps which represent an effective tool for data storytelling.
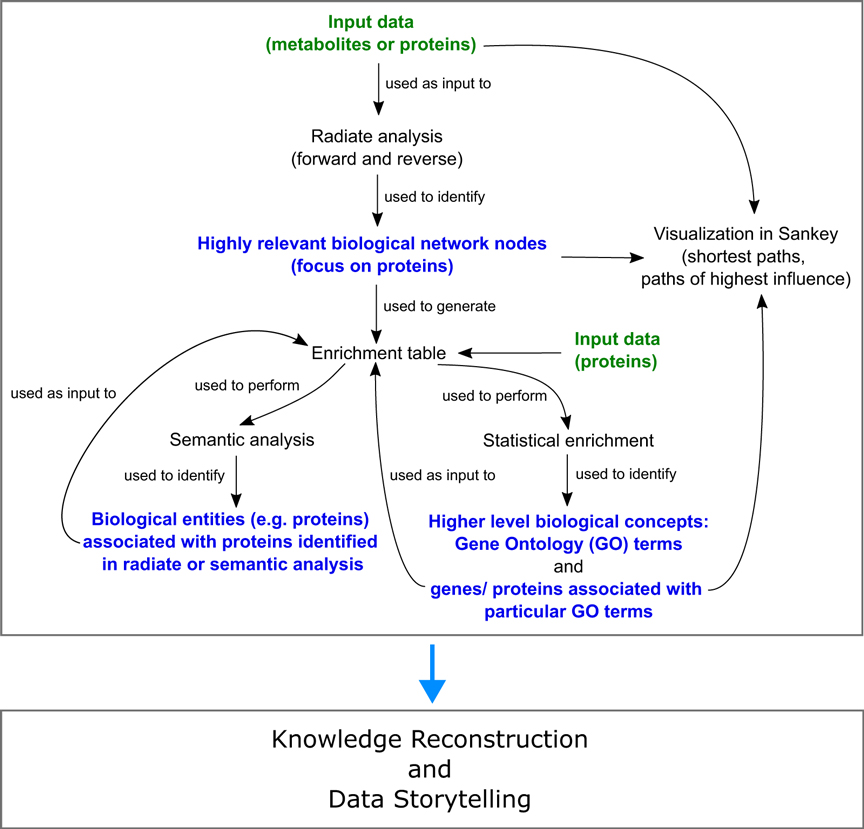
Figure 1. Schematic workflow employing Lifelike’s functionalities for the generation of deep knowledge from as little as 6 metabolites detected in the plasma of pulmonary hypertension patients.
3 Results
3.1 S1P, 12(S)-HETE, and L-Glu are more abundant in PH patients contributing to increased endothelial barrier permeability, vasoconstriction, and vascular cell proliferation
We found that the average concentrations of hypoxanthine (Hyp), 12(S)-Hydroxyeicosatetraenoic acid (12(S)-HETE), L-glutamic acid (L-Glu), and sphingosine 1 phosphate (S1P) increased more than two-fold in treated PH patients compared to the control group. Furthermore, the measured concentration of L-Arginine (L-Arg) and L-Histidine (L-His) are significantly lower in treated patients than in the control group (less than 71%) (16). We, therefore, searched for the molecules that are influenced by the metabolites as described in Section 2.2.4 using radiate analysis. The activation of Gi protein complexes by a G-protein-coupled receptor (GPCR) upon binding its corresponding ligand and the non-receptor tyrosine kinase SRC, were identified as crucial targets for S1P, 12(S)-HETE, and L-Glu (Table 1 rows 1, 2). GPCRs are important mediators of cellular signaling that are involved in the regulation of vascular cell proliferation (50), vascular tone (51–56) and endothelial barrier function (57, 58). Diverse G protein complexes, such as Gi/0, Gq, G12, and G13, enable GPCRs to elicit cell and tissue-specific responses to ligands.
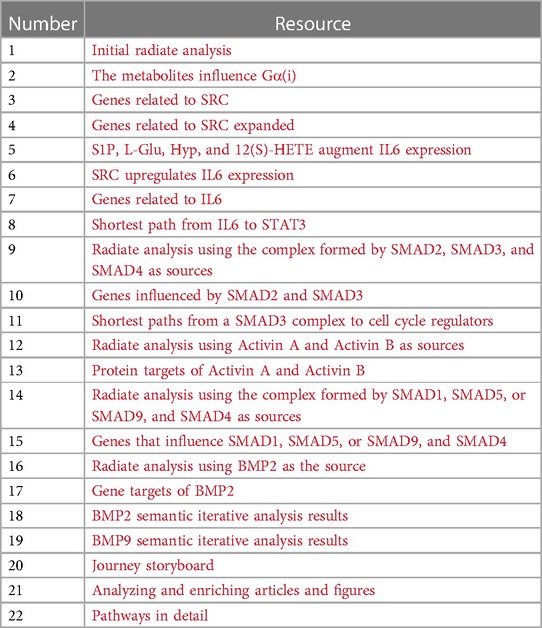
Table 1. Radiate analysis, enrichment tables, traces, and knowledge maps. Each entry in the resource column is a link.
3.1.1 Normally S1P preserves endothelial barrier function
In healthy individuals, the normal plasma concentration of S1P (0.1–0.8 M) is sufficient to activate the GPCR S1PR1 in PAECs (59). S1PR1 signals through Gi/0, PI3K and AKT1 to preserve endothelial barrier function. AKT1 causes cytoskeletal remodeling and EC spreading through RAC1 and Cortactin. Additionally, AKT1 activates eNOS, which catalyzes the production of NO, which causes the concentration of cGMP to increase. Then cGMP activates PKG I, which prevents the release of Ca2+ from the endoplasmic reticulum and activates MLCP. Subsequently MLCP deactivates MLC preventing cell contraction (52, 56, 60–62) (Figure 2A, and Supplementary Section S1.1.1).
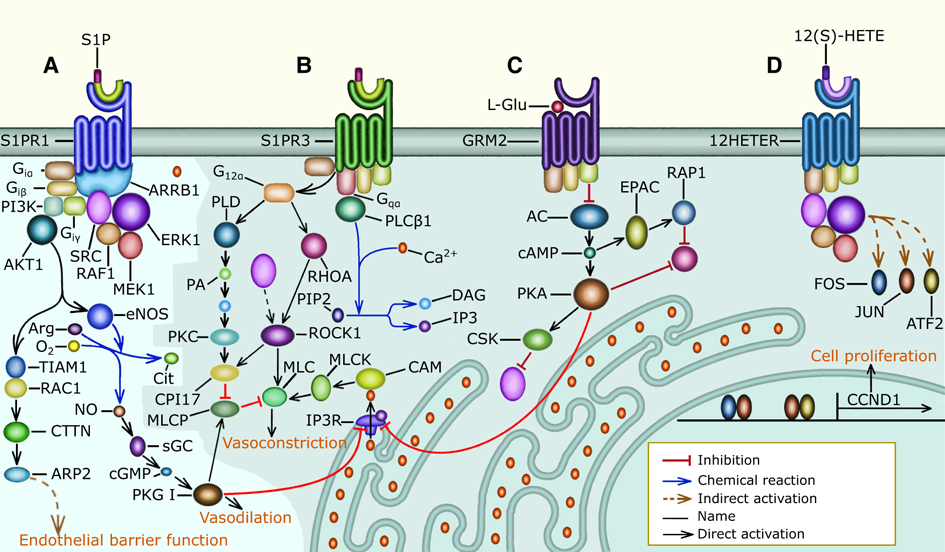
Figure 2. G protein signaling in pulmonary vascular cells: the shaded area of the figure includes signaling pathways that are overactivated in PH patients, (A) normally, S1P binds S1PR1 and preserves endothelial barrier function through the activation of the Gi, PI3K, AKT, and RAC1 signaling pathway that causes PAECs to increase the surface they cover through cytoskeletal remodeling. Gi, PI3K, and AKT signaling also activate eNOS to increase NO production and secretion. NO activates sGC increasing cGMP availability leading to PKG I activation. PKG I prevents MLC activation and cell contraction by decreasing the Ca2+ concentration in the cytosol and by activating MLCP. Lastly, S1P promotes normal PAEC proliferation through Gi, ARRB1, SRC, and ERK signaling, which promotes Cyclin D1 expression, (B) in PH patients, the concentration of S1P in the plasma is elevated and this activates S1PR2 (not shown), and S1PR3 signaling resulting in MLC activation and cell contraction. S1PR3 increases MLC activity through the G12, PKC, and MLCP signaling pathway, the G12, RHOA, and ROCK signaling pathway that requires SRC activity, and the Gq, PLC, Ca, and MLCK signaling pathway. In PAECs, MLC activation results in cell rounding and endothelial barrier permeability. Additionally, in PASMCs MLC activation leads to vasoconstriction, (C) the elevated concentration of L-Glu in the plasma of PH patients activates GRM3 (not shown) and GRM2-mediated Gi signaling that inhibits AC causing the cAMP concentration to decrease. The absence of cAMP inhibits PKA, allowing the release of Ca2+ from the sarcoplasmic reticulum. Also, the decrease in cAMP leads to an increase in SRC activity. Further, the reduction in cAMP increases RHOA activity, which causes MLC activation, loss of endothelial barrier function, and vasoconstriction, (D) the concentration of 12(S)-HETE is abnormally high in many PH patients, 12(S)-HETE binds 12HETER, leading to the activation of the Gi, ARRB1, SRC, and ERK signaling pathway, which causes excessive vascular cell proliferation. Additionally, 12HETER can signal through Gq and G12 causing PAEC contraction (not shown).
3.1.2 In PH patients S1PR2, S1PR3, 12HETER, GRM2, and GRM3 act in concert to promote vascular cell proliferation, inhibit apoptosis, and increase the activity of MLC resulting in vasoconstriction
The S1P receptors S1PR2, and S1PR3 (52, 56, 59, 62), and the 12(S)-HETE receptor 12HETER (50, 58, 63) are present in the cell membrane of PAECs and PASMCs. S1PR2, and S1PR3 are only activated when the concentration of S1P is abnormally elevated as observed in most PH patients. Similarly, the activity of 12HETER is abnormally elevated in PH patients. S1PR2, S1PR3 (Supplementary Section S1.1.2 and Figure 2B) and 12HETER (Supplementary Section S1.1.4 and Figure 2D) signal through Gq, PLC, IP3, and DAG. IP3 promotes the release of Ca2+ from the endoplasmic reticulum, and DAG inhibits MLCP, resulting in MLC activation (62, 64, 65). Additionally, S1PR2, S1PR3 and 12HETER also activate G12, and G13 signaling, which causes RHOA-mediated MLC activation (54–56) and preserves MLC activity through the inhibition of MLCP (53). Moreover, the elevated concentration of L-Glu found in PH patients suffices to activate GRM2, and GRM3, which are expressed by PAECs (66, 67). GRM2, and GRM3 signal through Gi/0 to decrease the concentration of cAMP, which inhibits the release of Ca2+ from the endoplasmic reticulum and is required for the RAP1-dependent inhibition of RHOA. Therefore, GRM2, and GRM3 augment MLC activity by decreasing the concentration of cAMP (68–70) (Figure 2C, Supplementary Section S1.1.3). In PAECs MLC activity increases endothelial barrier permeability. Furthermore, in PASMCs MLC activity leads to vasoconstriction. Additionally, S1PR2, S1PR3, 12HETER, GRM2, and GRM3 signal through Gi/0 and SRC resulting in the proliferation of PAECs and PASMCs (Figure 2D and Supplementary Section S1.1).
3.1.3 SRC is an important mediator of S1PR1, S1PR3, GRM2, GRM3, and 12HETER signaling in PH patients that stimulates excessive vascular cell proliferation, increases endothelial barrier permeability, and causes vasoconstriction
The non-receptor tyrosine kinase SRC was the first proto-oncogene to be described and is involved in the regulation of cell growth, differentiation, and survival (71). SRC plays an important role in the pathophysiology of PH and is a potential drug target for the treatment of PH (72). SRC is an important mediator of S1PR1, S1PR3, GRM2, GRM3, and 12HETER signaling because in PH patients, overactive Gi/0 signaling leads to excessive PAEC and PASMC proliferation by activating the SRC/ERK signaling pathway that induces the expression of Cyclin D1 (50, 73–75) (Figure 2D, Supplementary Section S1.1.4). Additionally, in a rat model SRC mediates hypoxic pulmonary vasoconstriction through the translocation of ROCK, which is necessary for MYPT-1 activation. MYPT-1 inhibits MLCP resulting in increased MLC phosphorylation and vasoconstriction (76). Furthermore, SRC phosphorylates VE-cadherin to regulate endothelial barrier permeability in response to flow-mediated mechanosensory stimuli, and in PH patients this leads to internalization of VE-cadherin and increased barrier permeability (77). The molecular mechanisms mentioned above imply that SRC is an essential component of many pathways that connect the differentially expressed metabolites with vasoconstriction, endothelial dysfunction, and vascular cell proliferation. We call molecules like SRC convergence nodes (Figures 2B–D).
3.2 IL6 signaling promotes pulmonary vascular cell proliferation and increases endothelial barrier permeability in PH patients
3.2.1 SRC activates the expression of IL6, leading to autocrine and paracrine IL6 signaling
Due to the importance of SRC as a convergence point for GPCR signaling, we performed a semantic analysis on textual descriptions derived from public databases such as NCBI, Uniprot, and StringDB, resulting in an enrichment table with related genes (Table 1 row 3), which we further analyzed utilizing the Gene Ontology (GO). This identified IL6 signaling, which is associated with STAT1, IL6, CBL, STAT3, and SRC. After that, we traced the shortest paths from SRC to IL6, which involve the AKT signaling pathway, which induces IL6 expression through CREB1 activation, and the RAS signaling pathway, which induces IL6 transcription through CEBPB phosphorylation (Table 1 rows 5, 6). This is in accordance with experimental observations that SRC activates the expression of IL6 (78, 79). The diverse mechanisms that allow SRC to increase IL6 expression likely contribute to the elevated concentration of IL6 found in the serum and lungs of PH patients (80, 81) (Figure 3J). In alignment with this, overexpression of IL6 triggers PH and right ventricle hypertrophy in mice by promoting pulmonary arterial muscularization and the formation of occlusive lesions composed of endothelial cells and T-lymphocytes in the distal pulmonary arterioles (82). Also, higher concentrations of IL6 are associated with a greater risk of death (81).
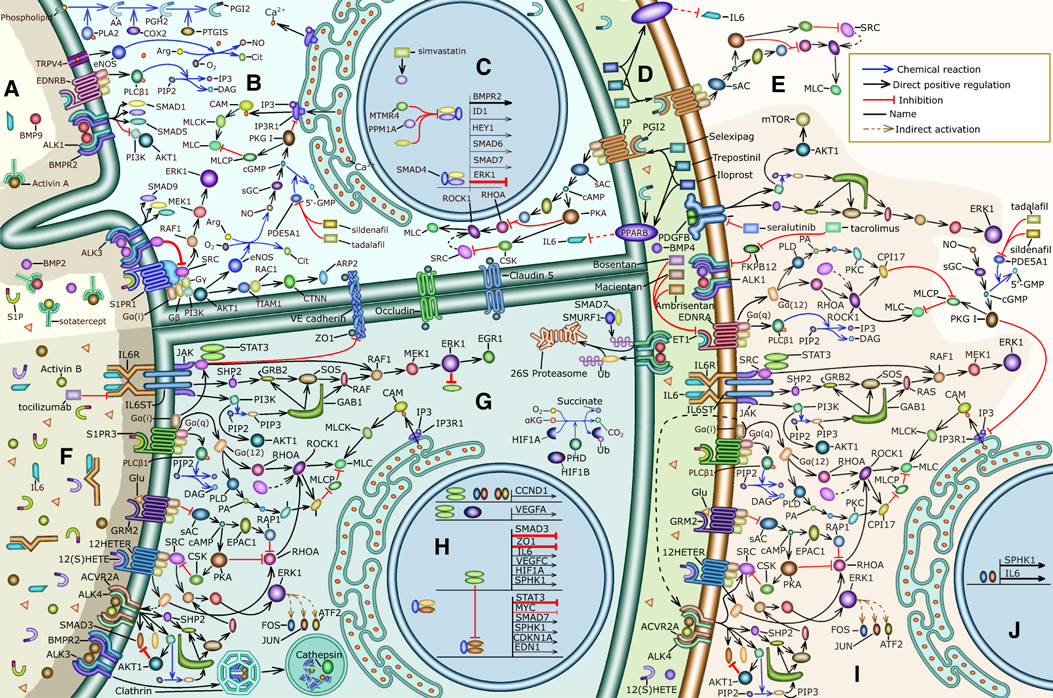
Figure 3. Summary of the signaling pathways involved in the pathophysiology of PH and the mechanisms of action of drugs that we explored: (A) the lumen of a pulmonary blood vessel of a treated PH patient, (B) the cytosol of a PAEC in a healthy person or treated PH patient, (C) the nucleus of a PAEC in a healthy person or treated PH patient, (D) the basement membrane separating PAECs from PASMCs, (E) mechanisms that cause vasodilation in healthy PASMCs, (F) the lumen of a pulmonary blood vessel from a PH patient, (G) the cytoplasm of a PAEC from a PH patient, (H) the nucleus of a PAEC from a PH patient, (I) the mechanisms that cause PASMC contraction and proliferation, which are overactive in PH patients, (J) the nucleus of a PASMC from a PH patient.
3.2.2 IL6 signaling increases endothelial barrier permeability, inhibits apoptosis, and increases cell proliferation through the activation of STAT, ERK and AKT signaling
The importance of IL6 in PH led us to perform a semantic analysis of STAT1, IL6, CBL, STAT3 and SRC that resulted in an enrichment table that contains genes related to IL6 (Table 1 row 7). Subsequently, we used GO enrichment to associate the genes related to IL6 with biological mechanisms that are important for the pathophysiology of PH, including positive regulation of ERK1 and ERK2 signaling, positive regulation of smooth muscle and endothelial cell proliferation, negative regulation of apoptotic process, and platelet activation. The IL6 receptor, IL6R is not expressed in PAECs. However, in the plasma of PH patients IL6 binds the soluble form of its receptor (sIL6R), and then both bind the coreceptor IL6ST (gp130) present in the cell membrane of PAECs forming a hetero-hexamer complex that initiates trans IL6 signaling (80, 81). IL6 signaling leads to the activation of STAT signaling, ERK signaling, and AKT signaling (Figures 4A–C) (83). IL6 signaling, through ERK1, ERK2, and STAT3 promotes PAEC and PASMC proliferation by augmenting the expression of Cyclin D1, MYC, and PIM1 (Figure 4A and Supplementary Section S1.2.2) (80, 84). Moreover, SRC and STAT3 are activated by IL6 signaling and increase endothelial barrier permeability by causing the internalization of adherence and tight junction components including VE-cadherin and ZO1 (85–87) (Figure 4D and Supplementary Section S1.2.3). Additionally, IL6 signaling integrates inflammatory and hypoxic signals to increase VEGFA (88) expression, which further increases endothelial barrier permeability (Figure 4F and Supplementary Section S1.2.4). Further, IL6-activated AKT signaling promotes PAEC survival by stimulating the expression of Survivin (89) and suppressing BAX-mediated apoptosis (90) (Figure 4C, and Supplementary Section S1.2.5).
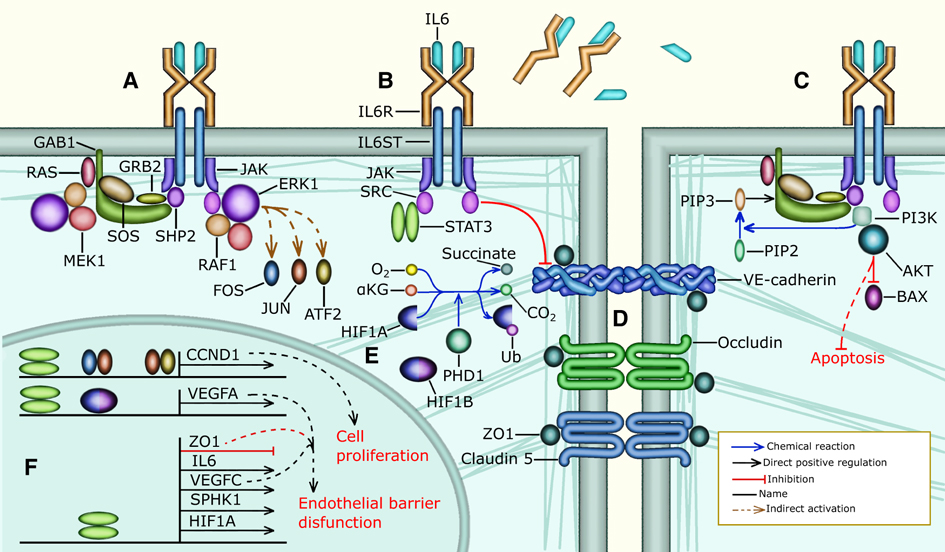
Figure 4. IL6 signaling in the endothelium of a PH patient: (A) PAECs do not express IL6R. However, sIL6R and IL6 are present in high concentrations in the blood plasma of PH patients. The complex formed by IL6, sIL6R and IL6ST activates JAK and then SHP2 or SRC to activate ERK signaling. SRC directly activates RAF1, leading to the activation of MEK1, and ERK1. SHP2 on the other hand requires GRB2, SOS, and RAS to activate RAF1, MEK1, and ERK1 signaling, (B) IL6 signaling can also activate STAT3 through SRC. Additionally, SRC phosphorylates VE-cadherin, (C) IL6 signaling can also activate PI3K through SHP2 and GAB1. PI3K then phosphorylates PIP2 to produce PIP3 which is needed to recruit GAB1 near the cell membrane. Additionally, PI3K activates AKT1, which inhibits BAX-mediated apoptosis, (D) STAT3 forms a dimer that inhibits the transcription of ZO1 resulting in the internalization of adherence and tight junctions increasing endothelial barrier permeability, (E) hypoxia prevents the ubiquitination and degradation of HIF1A. Then HIF1A binds HIF1B forming a complex that regulates the transcription of several genes, (F) STAT3, FOS, JUN, and ATF bind the promoter of CCND1 to activate the expression of Cyclin D1 allowing G1/S cell cycle progression, which leads to PAEC proliferation. IL6 is involved in some of the mechanisms through which hypoxia triggers PH, for instance HIF1A, HIF1B and STAT3 bind to the VEGFA promoter and activate the expression of VEGFA. Additionally, STAT3 activates the expression of IL6, VEGFC, SPHK1, and HIF1A. STAT3 also inhibits the transcription of ZO1.
3.3 In PH patients, activin signaling and cell proliferation are overactivated while BMP signaling and apoptosis are inhibited
In our SRC-centered iterative semantic analysis we encountered GDF2(BMP9), SMAD1, SMAD3, and SMAD4 (Table 1 row 4). Additionally, SMAD2 and SMAD3 are intermediary nodes in the shortest path trace from IL6 to STAT3 (Table 1 row 8). The TGF family of signaling pathways that include activin signaling and BMP signaling is involved in the regulation of EC identity, motility, and barrier function (91, 92). Further, BMPR2, ALK1, ENG, and SMAD9 mutations are associated with PH (93) and the activin and BMP signaling pathways are involved in the pathophysiology of PH (94, 95). Therefore, we decided to further explore the effect of activin and BMP signaling in PH.
3.3.1 In PH patients, SMAD2 and SMAD3-mediated activin signaling increases the production of S1P and ET1, inducing vascular cell proliferation and vasoconstriction. Additionally, SMAD-independent activin signaling stimulates ERK signaling and causes BMPR2 internalization and degradation, further increasing vascular cell proliferation
Activin A is overexpressed in the PAECs of PH patients (96). The canonical effect of activin signaling is the phosphorylation and activation of SMAD2 and SMAD3 (96–98). Therefore, we carried out a radiate analysis using the complex formed by SMAD2, SMAD3, and SMAD4 as the source (Table 1 row 9). Then, we took the genes targeted by SMAD2, SMAD3, and SMAD4 signaling, created an enrichment table (Table 1 row 10), searched for the GO biological processes that are significantly associated with the genes and found several GO terms associated with cell cycle regulation.
After that, we traced the shortest paths that connect the SMAD complex to important cell cycle regulators (Table 1 row 11). We found that SMAD3 signaling activates the expression of CDKN1A (p21) and downregulates MYC directly (Figure 5G) and cyclin D1 indirectly, therefore preventing cell proliferation. This is in accordance with what has been observed experimentally in many cell types including human umbilical vein ECs (99, 100) (Supplementary Section S1.3.1.1 and Figure 5G).
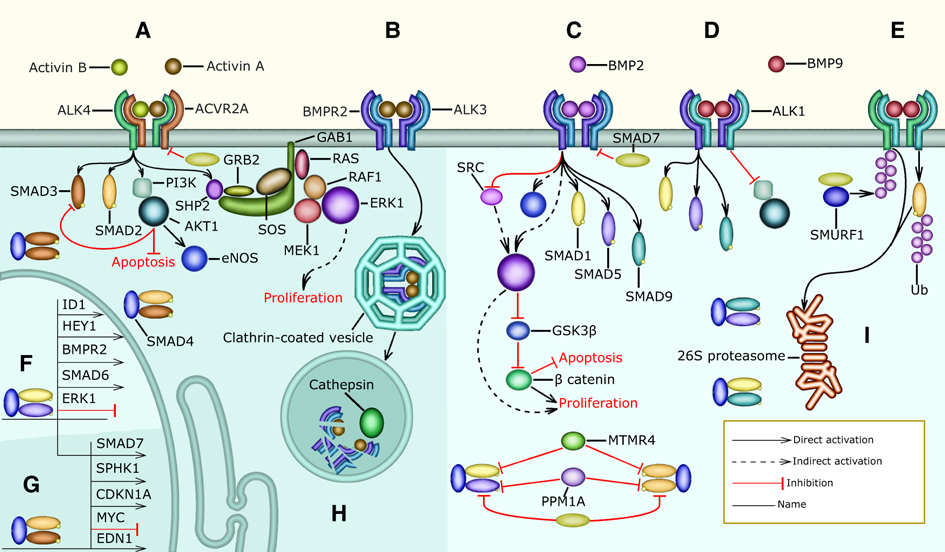
Figure 5. Activin and BMP signaling: the shaded areas highlight pathways that are overactivated in PH patients (A) the concentration of activin A and activin B in the serum of PH patients is higher than normal. Activin A and activin B bind ACVR2A with a higher affinity than BMP2 and other TGF-family ligands. When an activin dimer (AA, BB, or AB) forms a complex with ACVR2A and ALK4, it can activate SMAD2, SMAD3, AKT, and ERK signaling. AKT inhibits apoptosis and ERK allows vascular cell division leading to excessive vascular cell proliferation, (B) activin A also binds BMPR2 with high affinity, preventing BMP2 signaling through competitive inhibition, (C) in healthy subjects, BMP2 binds BMPR2 and ALK3 resulting in the activation of SMAD1, SMAD5 and SMAD9. Additionally, when BMP2 binds BPMR2 it inhibits SRC. Further, BMP2 signaling regulates apoptosis and promotes normal endothelial proliferation through the ERK-mediated activation of -catenin (D), BMP9 can form a complex with BMPR2 and ALK1 resulting in the activation of SMAD1, SMAD5, and SMAD9. Additionally, BMP9 inhibits PI3K and AKT signaling through BMPR2. (E) Further, BMP9 can bind ACVR2A and ALK1 forming a complex that leads to the activation of SMAD2. SMAD7 competes with SMAD2 for the receptor. Moreover, SMAD7 recruits and collaborates with the E3 ligase SMURF1 that ubiquitinates active R-SMADS and TBRIs targeting them for 26S proteosome-mediated degradation, (F) BMP-activated SMAD1, SMAD5, and SMAD9 can form complexes with SMAD4 that are transported into the nucleus where they inhibit the expression of ERK1 and activate the expression of HEY1, SMAD6, SMAD7, and ID1, (G) activin-induced SMAD2 and SMAD3 form complexes with SMAD4 that are transported to the nucleus where they inhibit the expression of MYC and activate the transcription of SMAD7, SPHK1, and CDKN1A, (H) actvin A binding leads to the internalization of BMPR2 and ALK3 in Clathrin-coated vesicles that fuse with lysosomes present in the cytoplasm. The lysosomes contain Cathepsin peptidases that are active due to the low pH and degrade BMPR2 and ALK3, (I) SMAD6, SMAD7, PPM1A, and MTMR4 downregulate the formation of SMAD transcription factor complexes.
In PASMCs, activin signaling stimulates excessive proliferation (98) and this may be explained by SMAD-independent pathways stimulated by activin signaling. Therefore, we performed a radiate analysis using activin A and B as the sources (Table 1 row 12). The GO terms significantly associated with the genes targeted by activin A and B (Table 1 row 13) include negative regulation of apoptotic process, positive regulation of cell population proliferation, and negative regulation of BMP signaling pathway supporting the assumption above.
Additionally, we used the entity cloud functionality of Lifelike on the enrichment table above to highlight frequently mentioned genes associated with activin signaling, including AKT1, BAD, BAX, SRC, ERK, RHO, and RAC1, which are involved in SMAD-independent activin signaling (Supplementary Section S1.3.1.2).
It has been reported in the literature that activin upregulates AKT (101) and ERK (102–104) signaling and induces the internalization and degradation of BMPR2 (Supplementary Section S1.3.1.2, Figures 5A,B,H). AKT inhibits apoptosis and prevents the phosphorylation and nuclear translocation of SMAD3 (105). Further, ERK signaling causes excessive proliferation in the PAECs of PH patients (106–108).
Literature curation identified two possible mechanisms through which SMAD-dependent activin signaling is detrimental for PH patients. In PASMCs SMAD2, SMAD3, and SMAD4 stimulate the expression of SPHK1, an enzyme that catalyzes S1P production (109) contributing to the excessive PASMC proliferation and vasoconstriction experienced by PH patients and it could be speculated that this also occurs in PAECs leading to an increase in endothelial barrier permeability. Furthermore, activin A augments the production of endothelin-1 (ET1) leading to vasoconstriction (98). The promoter of EDN1, which encodes a preproprotein that is proteolytically processed to generate ET1, contains a SMAD binding site, and phosphorylated SMAD3 activates the transcription of EDN1 in cultivated HUVECs (110).
3.3.2 Under normal conditions, BMP2 and BMP9 activate SMAD1, SMAD5 and SMAD9, which promote the transcription of BMPR2 and inhibit the transcription of ERK1 and ERK2. Additionally, BMP2-activated BMPR2 inhibits PAEC proliferation by inhibiting SRC activation
BMP signaling canonically leads to the phosphorylation of SMAD1, SMAD5, and SMAD9 (91, 111). We performed a radiate analysis with these R-SMADS as sources (Table 1 row 14). Then, we created an enrichment table with the most significant proteins that target canonical BMP signaling (very few proteins are targeted by these SMADs) (Table 1 row 15). After that, using GO we found that: negative regulation of cell population proliferation, regulation of smooth muscle cell proliferation, BMP signaling pathway, and activin receptor signaling pathway are significantly associated with the genes that regulate SMAD1, SMAD5, and SMAD9.
Similarly, to study the effect of both SMAD-dependent and SMAD-independent BMP signaling we performed a radiate analysis using BMP2 as the source (Table 1 row 16). Then we created an enrichment table containing the gene targets of BMP2 (Table 1 row 17). After that, using GO we found that the targets of BMP2 are significantly associated with the following terms: activation of cysteine-type endopeptidase activity involved in apoptotic process by cytochrome c, positive regulation of mitochondrial outer membrane permeabilization involved in apoptotic signaling pathway, and apoptotic process involved in blood vessel morphogenesis. It has also been observed experimentally that BMP2 signaling modulates hypoxia-induced apoptosis, and this reduces PH severity in rats (112).
However, perhaps the most beneficial effects of BMP signaling are stimulating the expression of BMPR2 forming a beneficial feedback mechanism that prevents excessive vascular cell proliferation. BMP2 inhibits PAEC proliferation by reducing SRC activity (113), inhibiting the transcription of ERK1 and ERK2 (114), and activating the transcription of ID1 and ID3 (115) (Supplementary Section S1.3.2 and Figures 5C,F).
Additionally, we performed a semantic iterative analysis with BMP2 as the source resulting in a significant association with bone mineralization and positive regulation of osteoblast proliferation (Table 1 row 18). Not all the effects of BMP2 signaling are beneficial for PH patients, as BMP2 signaling can induce vascular calcification greatly diminishing the therapeutic potential of BMP2 in the treatment of PH (116–118).
We also explored the function of BMP9 (GDF2) using a semantic iterative analysis (Table 1 row 19) and found that BMP9 is significantly associated with negative regulation of EC proliferation and negative regulation of vascular EC migration. This agrees with the experimentally observed function of BMP9, which is to preserve EC quiescence (95, 116, 119). However, BMPR2 loss in PAECs reverses the effect of BMP9 (118, 120).
3.4 The interaction between G protein, IL6, activin, and BMP signaling in PH
Collectively, our analyses point towards that S1P, L-Glu, 12(S)-HETE, IL6, and activin A stimulate the activity of signaling pathways that converge on SRC, ERK1, AKT, and MLC to cause many of the pathophysiological phenomena that characterize PH.
3.4.1 Normally S1P and STAT3 stimulate each other forming a positive feedback mechanism that preserves endothelial barrier function
IL6 signaling leads to the activation of the transcription factor STAT3 (Figure 3G) that stimulates the expression of SPHK1 (Figure 3H), SPHK2, and SPNS2. The enzymes SPHK1 and SPHK2 catalyze the phosphorylation of sphingosine to produce S1P. SPNS2 is a cell membrane protein that forms a channel and allows the transport of S1P from the cytoplasm to the extracellular space. S1P binds S1PR1 resulting in the activation of SRC, ERK1, and ERK2 (Figure 3B). SRC directly activates STAT3 and indirectly upregulates EGR1 through ERK1 (Figure 3G). STAT3 and EGR1 increase the expression of SPHK1 stimulating the production of S1P in human lung microvascular endothelial cells (Figure 3H). This positive feedback mechanism between S1P and STAT3 preserves endothelial barrier function (121). However, ERK1 can also phosphorylate STAT3 on serine 727, which inhibits STAT3 dimerization and activation by preventing the phosphorylation of tyrosine residues on STAT3 (122) (Figure 3G). This constitutes a negative feedback mechanism that likely prevents excessive S1P production. We surmise that this negative feedback mechanism is ineffective in PH patients due to the higher concentration of S1P present in their serum.
3.4.2 The interaction between G-protein signaling and IL6 signaling: S1P, L-Glu, 12(S)-HETE, and IL6 stimulate pulmonary vascular cell proliferation and increase PAEC barrier permeability
S1P also signals through S1PR3 resulting in SRC and ERK activation (123) (Figure 3G). Additionally, L-Glu signaling through GRM2 and GRM3 positively modulates SRC activity (Figure 3G, Supplementary Section S1.1.3). Further, 12(S)-HETE binds 12HETER and stimulates SRC activity (63) (Figure 3G). Therefore, we propose that in PH patients S1PR3, GRM2, GRM3, and 12HETER signaling causes the positive feedback mechanism that exists between STAT3 and S1P to be overactive (121) (Section 3.4.1). Notably, IL6, S1PR3, GRM2, GRM3, and 12HETER signal in concert to stimulate vascular cell proliferation, disrupt endothelial barrier function, and promote vasoconstriction (Supplementary Sections S1.1.2–S1.1.4, S1.2.2–S1.2.4).
3.4.3 In PH patients activin signaling and S1P signaling amplify each other through positive feedback mechanisms that lead to excessive PAEC and PASMC proliferation
GPCRs can transactivate receptor tyrosine kinases and serine/threonine kinase receptors, greatly amplifying their effect on cell signaling (124). For instance, S1PR3 can activate SMAD3 during the differentiation of cultured human foreskin fibroblasts into myofibroblasts (125). Vascular fibroblasts and myofibroblasts are very closely related to PAECs and PASMCs through the endothelial-to-mesenchymal transition and therefore we propose that this activation of SMAD3 also occurs in PAECs (Figure 3I). SMAD3 then stimulates the transcription of SPHK1 (Figure 3H) and SPHK2 increasing the concentration of S1P, forming a positive feedback mechanism that promotes excessive vascular cell proliferation in PH patients (109). Endothelin (ET1) binds to the GPCR endothelin receptor A (EDNRA) leading to SMAD2 phosphorylation in bovine aortic endothelial cells (126, 127). Further, SMAD3 stimulates the production of ET1 (98, 110) (Figure 3H). We propose the existence of a positive feedback mechanism between SMAD2 or SMAD3 and ET1 that promotes vasoconstriction. A molecular mechanism for the transactivation of TRIs by GPCRs (Figure 3I) involves the sequential activation of RHOA, ROCK, and integrin. Then, active integrin causes the large latent TGF complex to activate TRIs resulting in the phosphorylation of SMAD2 leading to increased PAEC and PASMC proliferation (128).
3.4.4 STAT3 and SMAD3 inhibit each other’s transcription and activation to stimulate PAEC and PASMC proliferation in PH patients
In PH patients activin A and IL6 are present at a higher concentration than in the healthy population (Figure 3G) (80, 81, 96). Activin A causes the activation of SMAD3 and IL6 signaling leads to the activation of STAT3 (Figure 3G). The crosstalk between STAT3 and SMAD3 depends on the specific biological context. We hypothesize that the following pathways might also be active in the PAECs and PASMCs of PH patients (129); In cultivated human lung adenocarcinoma cells STAT3 represses the transcription of SMAD3 in cooperation with SKI and SKIL preventing SMAD2, SMAD3, and SMAD4 from activating the transcription of SMAD3 (130) (Figure 3H). STAT3 (131) and SMAD3 (132) also inhibit the activity of SMAD3 by inducing the expression of SMAD7 (Figure 3H). Also, in an immortalized human keratinocyte cell line, STAT3 directly binds phosphorylated SMAD3 and prevents it from binding SMAD4 and regulating transcription (133) (Figure 3H). By inhibiting SMAD3, STAT3 might allow PAEC and PASMC proliferation in PH patients. Further, in cultured human hepatocellular carcinoma cells SMAD3 and SPTBN1 collaborate to inhibit the ATF3, and CREB2-mediated transcription of STAT3 (134) (Figure 3H). Interestingly SMAD2, SMAD3, and STAT3 collaborate to activate the expression of SNAI1, one of the main transcription factors involved in the endothelial-to-mesenchymal transition that causes EC to stop expressing VE-cadherin, PECAM1, and VEGFR2 and to detach from the intimal layer (92, 129, 135). This constitutes an additional molecular mechanism through which STAT3 erodes endothelial barrier function (Figures 3F–H).
3.5 The mechanism of action of commonly used and novel drugs for the treatment of PH
In this section, we aim to investigate how the mechanisms of action of currently used and novel pharmacological agents target the signaling pathways explored in the previous sections.
3.5.1 Prostacyclin agonists, originally introduced as vasodilators, also target inflammation and inhibit PASMC proliferation
Prostacyclin (PGI2) is a cardioprotective hormone produced and secreted by ECs. The synthesis of PGI2 begins with the liberation of arachidonic acid (AA) from membrane phospholipids, mainly by Ca2+ dependent phospholipase A2 (PLA2) isoforms. Then, AA is transformed into prostaglandin H2 by cyclo-oxygenases (COX1, COX2). After that prostacyclin synthase catalyzes the reaction that converts prostaglandin H2 into PGI2 (136) (Figure 3B).
PGI2 activates the Gs–coupled receptor IP and PPAR. IP stimulates adenylate cyclase leading to an increase in cAMP (136–138), which activates PKA and EPAC1 (66)—leading to the deactivation of MLC, preventing vasoconstriction, and enhancing endothelial barrier function (Supplementary Sections S1.1.2–S1.1.3). Further, PGI2 inhibits PASMC proliferation using a cAMP-dependent mechanism (139). Specifically, cAMP sequentially activates PKA and CSK to inhibit SRC and prevent excessive PASMC proliferation (69). PPAR mediates many of the anti-inflammatory effects of PGI2. For instance, PPAR activity inhibits TGF-mediated IL6 upregulation (140) (Figures 3B,E).
The PGI2 agonists epoprostenol, iloprost, and treprostinil activate both IP and PPAR-mediated pathways, selexipag only activates IP receptors (141) (Figure 3D). The therapeutic potential of PGI2-agonists for the treatment of PH is substantial due to their vasodilatory, antiproliferative, and anti-inflammatory effects (142), suggesting that they should be included more often as part of the initial treatment. The ability of PGI2-agonists to inhibit IL6 is particularly promising. The concentration of IL6 is elevated in the serum of PH patients and is correlated with disease severity and risk of death (80, 81). Additionally, IL6 overexpression causes PH (82). Therefore, pharmacological interventions that diminish IL6 signaling may have therapeutic potential in some PH patients (80). Although the IL6R inhibitor tocilizumab showed no benefit in a Phase 2 trial (143), other approaches including targeting IL6ST (144) and PGI2-agonists may result in more efficient PH treatment options that target IL6 signaling (145).
3.5.2 PDE5 and ET1 inhibitors inhibit vasoconstriction by preventing MLC activation
The PDE5 inhibitors sildenafil (146, 147) and tadalafil (148) were initially approved to treat erectile dysfunction. By inhibiting PDE5A1, sildenafil and tadalafil prevent the transformation of cGMP into 5’-GMP. Increased levels of cGMP then activate PKG I which prevents vasoconstriction by inhibiting PI3KR and averting the release of Ca2+ from the sarcoplasmic reticulum. Additionally, PKG I activates MLCP, which dephosphorylates MLC and hinders vasoconstriction (149, 150). Both drugs decrease pulmonary vascular resistance and improve exercise capacity (146, 148) (Figures 3B,E).
Another common approach to reduce vasoconstriction is to inhibit ET1 signaling. The expression of ET1 in the PAECs of PH patients is elevated compared to healthy individuals (151), possibly because SMAD3 upregulates the transcription of EDN1 which encodes ET1 (98, 110) (Figures 3H, 5G). Another relevant mechanism that could increase the expression of EDN1, involves the FOS and JUN that are upregulated by ERK signaling (74, 152) and stimulate the transcription of EDN1 (153, 154) (Figure 3J). All ET1 receptors belong to the GPCR superfamily of receptors.
PASMCs express two kinds of ET1 receptors; EDNRA is present at a higher concentration and mediates vasoconstriction, EDNRB is present at a lower concentration. In PASMCs, stimulation of both receptors by ET1 causes vasoconstriction (155, 156). ET1-stimulated EDNRA can activate G12, G13, and Gq signaling (157, 158) (Figure 3I) causing vasoconstriction (159) (Supplementary Section S1.1.2). Additionally, EDNRA signaling stimulates human PASMC proliferation (160) through ERK signaling (161). This pathway is likely mediated by Gi/0 signaling (157, 158). EDNRB also activates Gq increasing the concentration of Ca2+ in the cytosol through PLC signaling leading to MLC activation and PASMC contraction (Figure 3B).
In PAECs the EDNRB-mediated increase in Ca2+ activates TRPV4, which is a transient receptor potential channel permeable to Ca2+, activates eNOS stimulating the production of NO (162) (Figure 3B). Additionally, Ca2+ activates PLA2, which releases AA from membrane phospholipids resulting in increased PGI2 production (136) (Figure 3B). The increase in NO and PGI2 causes vasodilation. EDNRB also functions as an ET1 trap and decreases its availability for EDNRA (163). Several PH drugs target endothelin signaling (Figure 3D): bosentan (164–166) and macientan (167) are inhibitors of EDNRA and ENDRB that improve exercise endurance, hemodynamics, and survival in PH patients (167). Other drugs like ambrisentan (168) selectively target EDNRA aiming to preserve ENDRB-mediated production of NO and PGI2 and allowing EDNRB to clear ET1 from circulation. However, this effect is very dependent on specific pathophysiological conditions that are largely unknown (169). Selectively targeting EDNRA decreases the risk of increased liver aminotansferases and increases the risk of edema (169).
3.5.3 Pharmacological approaches to restore the balance between activin and BMP signaling
In PH patients activin signaling is upregulated and BMP2 signaling is downregulated (94–96, 98). In PH patients the concentration of activin A and activin B is elevated (96, 98). These ligands bind BMPR2 with a higher affinity than BMP2 and BMP4. Therefore, activins A and B downregulate BMP2 and BMP4 signaling through competitive inhibition (170) (Figure 3F). Additionally, activin signaling promotes the internalization and the proteasomal degradation of BMPR2 (96) (Figure 3G). Further, the expression of BMPR2 and ALK3 is markedly reduced in the pulmonary vasculature of PH patients (171). This disbalance promotes excessive PASMC proliferation, vasoconstriction, and loss of PAEC identity resulting in perturbed endothelial barrier function. There have been three main approaches to restoring this balance.
The canonical effectors of activin signaling are SMAD2 and SMAD3. Simvastatin activates PP2A and PPM1A that dephosphorylate SMAD2 and SMAD3 (172). However, in a phase 1 trial, simvastatin only produced a small and transient reduction in right ventricle mass (173). Based on our analysis the inefficacy of simvastatin as a PH treatment might be because SMAD-independent activin signaling promotes vasoconstriction and PASMC proliferation (Section 3.4.1), also because PPM1A inhibits SMAD1, SMAD5, and SMAD9 activity (Figure 3C), which can prevent excessive vascular cell proliferation (Section 3.4.2).
Tacrolimus (FK506), which inhibits calcineurin and is used to prevent transplanted organ rejection, released FKBP12 from ALK1, ALK2, and ALK3 allowing BMP4 to activate SMAD1 and SMAD5 leading to enhanced BMPR2 signaling in cultured PAECs from PH patients (174) (Figure 3I). A phase IIa trial of tacrolimus as a PH treatment concluded that it had beneficial effects on three end-stage patients (175). However, another phase IIa trial found that the beneficial effects of tacrolimus were not significant (176). Increasing BMP4 signaling in PASMCs leads to their proliferation and upregulates vasoconstriction (112). Further, calcineurin is involved in a signaling pathway that also involves S1P, PLC, NFATC3, and OPN that leads to PASMCs proliferation (177). By upregulating BMP4 signaling and inhibiting calcineurin, we surmise that tacrolimus has an unpredictable effect on PASMC proliferation that depends on the pathophysiological context of each patient.
Sotatercept, which was originally developed for the treatment of anemia and bone loss (178), is under trial as a promising treatment for PH (97, 179). Sotatercept is a soluble ACVR2A IgG-Fc fusion protein that functions as a trap for activin A, activin B, BMP9, GDF8, GDF11 and other ligands from the TGF family that have a high binding affinity for ACVR2A (170) (Figure 3A). Sotatercept increases BMPR2 availability by preventing activin binding and BMPR2 degradation (Figures 3F,G. This allows BMP2 to activate SMAD1, SMAD5, and SMAD9 inhibiting the transcription of ERK1 and ERK2 (114), and activating the transcription of BMPR2 (116), ID1 and ID3 (115) (Figure 3C). Additionally, when BMP2 binds BMPR2 it decreases SRC phosphorylation in PASMCs (113) (Figure 3B). The effect of these BMP2-associated pathways is to prevent excessive vascular cell proliferation, preserve PAEC barrier function and prevent vasoconstriction. Downregulating activin signaling decreases SMAD2 and SMAD3 activity, and this prevents excessive S1P and ET1 production (Figure 3H). Additionally, downregulating activin signaling prevents SMAD-independent activation of SRC and ERK1 (Figure 3G) inhibiting GPCR-mediated vasoconstriction, endothelial barrier disruption and PASMC proliferation. In summary, sotatercept prevents the excessive activation of SRC, ERK1, AKT, and MLC, the molecules we identified as convergence nodes in the interplay between different PH signaling pathways.
3.5.4 Seralutinib prevents excessive PASMC proliferation by inhibiting PDGF signaling
PDGF signaling is one of the main molecular mechanisms that induce PASMC proliferation. PAECs, pulmonary vascular pericytes, and PASMCs express PDGFA, PDGFB, PDGFR and PDGFR and these genes are overexpressed in the lung arteries of PH patients (180, 181). In PASMCs, PDGFB signaling induces ERK1-mediated cell proliferation and stimulates cell survival and growth through AKT1 and mTOR (180) (Figure 3I). In rat PASMCs, PDGFB-stimulated ERK1 and AKT signaling upregulates the expression of SPHK1 and the production of S1P promoting PASMC proliferation (182). In mesangial cells that are contractile and perivascular like PASMCs, JUN and FOS that are upregulated by ERK signaling, activate the transcription of SPHK1 (183) (Figure 3J). Additionally, S1P signaling increases the expression of miR-21, which decreases the expression of BMPR2 and ID1 allowing excessive PASMC proliferation (115, 182). Also, -catenin (BC), which promotes PASMC proliferation, is elevated in PH patients. Moreover, PDGFB signaling prevents GSK3-mediated BC degradation and promotes BC nuclear localization and transcriptional activity in PASMCs. Further, BC knockdown reduces PDGFB-stimulated PASMC proliferation (184). Lastly, from a metabolic perspective, it is interesting to note that in PASMCs PDGFB, AKT1, mTOR, and HIF1A signaling is associated with the Warburg effect (185), defined as an increase in glucose breakdown and lactate production even when sufficient oxygen is available. The Warburg effect may cause excessive PAEC and PASMC proliferation in PAH patients (186).
Inhibiting PDGFR, PDGFR, and other tyrosine kinases systemically using imatinib leads to reduced PASMC proliferation. However, it is associated with very serious side effects including an increased risk of subdural hematoma preventing its use in the treatment of PH (187). Seralutinib is a novel tyrosine kinase inhibitor delivered via inhalation that downregulates PDGFR, and PDGFR signaling specifically in the lungs (Figure 3I). Seralutinib is currently under phase 2 clinical trial (188) and its effect on rats and PH lung samples was promising and restored the pulmonary expression of BMPR2 (189). All the hypotheses generated by our analysis are summarized in Tables 2, 3.
4 Discussion
Despite substantial progress in the understanding of PH pathophysiology over the last years, the detailed mechanisms underlying PH remain elusive due to the complex interplay of dysregulated biological processes. Also, although the mechanisms of action of some drugs that have been successfully used for the treatment of PH have been proposed, certain drugs may show additional mechanisms of action that are yet to be identified. Understanding these additional mechanisms of action is crucial for the personalized and thus more effective treatment of PH patients. In this study, we aimed to generate detailed mechanistic hypotheses of pathophysiological processes in PH using our in-house developed software platform Lifelike. Importantly, we have focused our analyses on the potential effects of blood plasma metabolites from PH patients on pulmonary endothelial and smooth muscle cells. Moreover, we used Lifelike to investigate the mechanisms of action of existing and new drugs used for the treatment of PH in more detail.
4.1 Integrating information from several sources using Lifelike enabled us to generate novel hypotheses about potential molecular mechanisms involved in the pathophysiology of PH
Using only the four differentially abundant blood plasma metabolites S1P, 12(S)-HETE, L-Glu, and L-Arg of PH patients as a starting point, this study demonstrates how knowledge mining with Lifelike enabled us to:
1. generate detailed mechanistic hypotheses about PH pathophysiology,
2. gain insights into the complex interplay of signaling pathways relevant to PH,
3. suggest mechanisms of drug actions in addition to those previously reported (e.g., sotatercept) that might impact personalized treatment of PH patients in the future, and
4. capture new knowledge effectively by creating Lifelike knowledge maps with links to references.
We have achieved this by analyzing metabolite data and receptor ligands on the curated database Reactome using graph algorithms, and by combining our software’s additional data analytics functionalities, including statistical enrichment and semantic analysis of textual information from Lifelike’s knowledge graph. Additionally, we validated, interpreted, and extended our results based on relevant existing literature. This entire process allowed us to explore the pathophysiology of PH and to propose the model shown in Figure 3 and summarized in Figure 6. Further, Lifelike allowed us to generate the hypotheses discussed in the following sections and shown in Tables 2, 3.
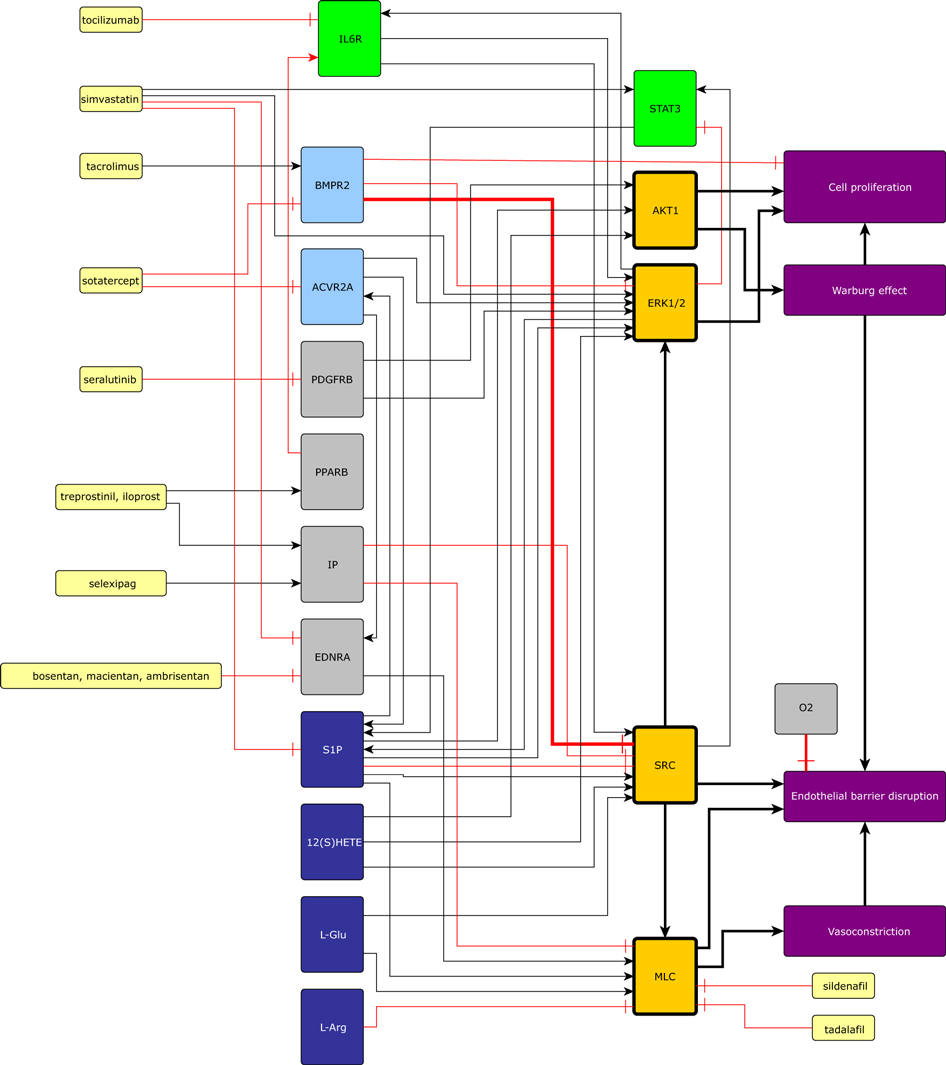
Figure 6. Currently available pharmaceutical treatments, GPCRs, IL6, actvin, BMP, PPARB, and PDGFB converge on SRC, MLC, ERK1, and AKT. The four convergence nodes then cause to PAEC and PASMC proliferation, endothelial barrier dysfunction, vasoconstriction, and the Warburg effect. Black arrows represent activation, and red arrows represent inhibitions. Yellow nodes are medicines, green nodes are part of the IL6 signaling pathway, light blue nodes are part of the activin or BMP signaling pathways, convergence nodes are shown in orange, dark blue nodes are differentially expressed metabolites, and purple nodes represent physiological effects. This figure also contains potential mechanisms of drug action proposed in this study.
Lifelike also enables the reader of a biology article to quickly find the molecules, diseases, and phenotypes that are discussed in the manuscript or included in the figures through entity recognition. For instance, genes detected in articles by Lifelike’s entity recognition system can be used to create an enrichment table where the genes can be associated with biological processes, molecular functions, and cell structures by GO enrichment. This allows the reader to find context-specific information quickly, and to extend the knowledge beyond what is described in the article with other sources of information (Table 1 row 21). We encourage the reader to upload this manuscript in PDF format into Lifelike for improved reading and knowledge extraction capabilities. Lifelike knowledge maps aid in the development of custom high-quality knowledge reconstructions with links to relevant literature through manual curation (Table 1 row 22).
Using Lifelike, we assembled a model of the molecular network involved in PH by connecting experimental observations (patient metabolite measurement data) with existing curated knowledge about pathways from Reactome, PAEC and PASMC gene expression and hundreds of articles describing relevant experimental results constituting a guided review of relevant pathophysiological mechanisms. We have put together a lot of experimentally validated knowledge. Specifically, we manually reviewed the evidence supporting our model increasing the robustness and reliability of the identified molecular mechanisms and hypotheses. This methodology substantially reduces the risk of overinterpretation. Overfitting is not a problem because our model is not based on adjusting parameters to fit the available data. Nevertheless, experimental validation is essential especially for the convergence of signaling pathways and the feedback mechanisms we describe in our hypotheses. Any model of a system is a logical structure that represents some of the characteristics of the system, while others are left out due to unavoidable simplification. However, models can be adapted or extended to explore other questions or integrate novel experimental insights. Just as the hypotheses generated by analyzing a model require experimental validation, any experimental research effort needs to be guided by the correct observations, hypotheses, and interpretation that only sound theory and models can provide. This is especially true when using a systemic approach to study a complex physiological system. We envision developing the computational tools that will enable us to transform these curated model networks into graph databases that can be then integrated with relevant existing databases or used on their own for graph analysis.
4.2 Interaction between different signaling pathways
The prevailing reductionist research paradigm focuses on one molecule, or at most one pathway at a time (190). Currently, the presence and activity of many molecules can be experimentally measured simultaneously. Additionally, many molecules and pathways have been carefully analysed and deposited in curated databases. This allows us to explore how molecular pathways influence each other, forming complex dynamic systems characterized by emergent properties that cannot be explained by a single molecule, pathway, or cell (190–192). Finding these emergent properties to develop a holistic understanding of a disease for the improvement of current treatments and future drug development is the goal of many research efforts in medical systems biology (193) including the present study. The convergence of several pathways on certain molecules is an important emergent property of the network model presented in Figure 3. This convergence allowed us to generate several hypotheses (Tables 2B–F). Notably, the elevated concentration of S1P, L-Glu, 12(S)-HETE, IL6, activin A, and ET1 present in the microenvironment that surrounds the PAECs and PASMCs of PH patients, stimulates the activity of signaling pathways that additively converge on SRC, ERK1, AKT1, and MLC to increase vascular cell proliferation, disrupt endothelial barrier function, and promote vasoconstriction (Figure 6). AKT1 is associated with cell proliferation and the Warburg effect which promotes cell proliferation and endothelial barrier dysfunction. SRC activates MLC and ERK1 and causes endothelial barrier dysfunction. ERK1 induces cell proliferation. MLC activity leads to vasoconstriction and endothelial barrier dysfunction (Figure 6).
4.3 Potentially important feedback mechanisms
In dynamic systems, positive feedback mechanisms that contain an even number of inhibitory edges, are associated with multistability, which can be interpreted as phenotypic diversity in biological systems (194–196). Alternatively, positive feedback mechanisms can amplify signals (197). Based on evidence from other cell types that share many important characteristics with PAECs and PASMCs, we propose that SMAD3 is part of two positive feedback mechanisms that involve S1P (Figure 7B), and ET1 (Figure 7A). These mechanisms promote vasoconstriction, endothelial barrier dysfunction, and excessive cell proliferation in PH patients (Tables 2G–I).
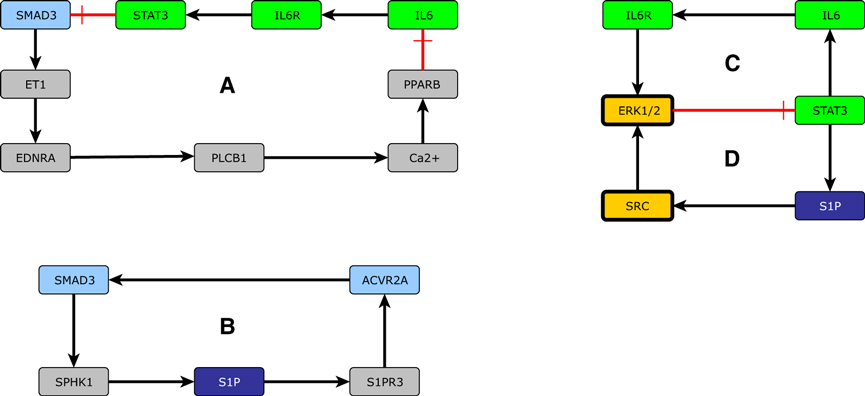
Figure 7. Feedback mechanisms: (A) a positive feedback mechanism between SMAD3 and ET1, (B) a positive feedback mechanism that involves S1P and SMAD3, (C) a negative feedback mechanism that involves IL6, (D) a negative feedback mechanism that involves S1P. Black arrows represent activation, and red arrows represent inhibitions. Green nodes are part of the IL6 signaling pathway, light blue nodes are part of the activin or BMP signaling pathways, convergence nodes are shown in orange, and dark blue nodes are differentially expressed metabolites.
Negative feedback mechanisms, characterized by the presence of an odd number of inhibitory edges, may cause or allow cyclic behavior, like the cell cycle and the circadian rhythm (194, 196, 198, 199). Alternatively, negative feedback circuits can balance the activity of two molecules or pathways. In biological systems this can contribute to homeostasis and robustness (200). The intrinsic robustness against perturbations of biological systems regulated by molecular regulatory networks and morphogen gradients might be one of the main causes that prevent pharmacological compounds from having the desired therapeutic effect (201). The role of robustness in the effectiveness of PH treatment remains largely unknown. Normally, S1P is present at a concentration that is only sufficient to activate S1PR1 signaling to preserve PAEC barrier function, and the regular activity of IL6 signaling prevents PAEC apoptosis and hypoxia-induced PH. Two negative feedback mechanisms might prevent the excessive IL6 and S1P activity observed in PH patients and maintain homeostasis. S1P signaling induces SRC that activates ERK1, which inhibits STAT3 activation preventing an increase in the production of S1P (Figure 7D). Similarly, IL6 signaling also activates ERK1, inhibiting the activation of STAT3 and the subsequent transcription of IL6 (Figure 7C).
4.4 Hypotheses concerning the mechanism of action of existing and novel pharmacological treatments of PH and their clinical implications
PH is a complex disease process involving multiple molecular mechanisms and chronic environmental, metabolic, genetic, and hypoxic insults resulting in pathologic remodeling of primarily medium- and small-sized pulmonary arterioles (202). The progression of pulmonary vascular remodeling in PH is driven by dynamic and local dysfunctions of both the pulmonary endothelium and vascular smooth muscle. In addition, abnormalities that affect the inflammatory and immune compartment is essential to produce the disease phenotype (203). Conventional therapies of currently approved PH drugs, all target vasoconstriction (203). Available PH medications include endothelin receptor antagonists (ERAs), phosphodiesterase type-5 inhibitors (PDE-5i), soluble guanylate cyclase stimulators, prostacyclin analogs, and oral prostacyclin receptor agonists none of which are curative (1). Using Lifelike’s ability to find novel potentially important drug mechanisms of action, enables pharmacological repurposing by finding additional mechanisms of action for existing drugs or proposing the potential mechanism of action of novel drugs. We were able to associate existing and novel drugs with molecular mechanisms of action that affect endothelial barrier dysfunction and PASMC proliferation (Table 3).
The quest for specific molecules that can influence signal transduction has become an essential part of exploring potential new drugs for the treatment of PH. In PH, signaling pathways are stimulated that converge on SRC, ERK, AKT, and MLC leading to excessive vasoconstriction, PASMC proliferation, and endothelial barrier dysfunction. The mechanisms of action of the existing and novel treatments for PH also converge on these four molecules (Figure 6). ERAs and PGI2 agonists are used mostly due to their known vasodilatory effect; our work emphasizes that they also prevent excessive cell proliferation through the inhibition of SRC. The PDGFRB antagonist seralutinib prevents excessive PASMC proliferation by downregulating -catenin and S1P signaling. Lifelike allowed us to understand that sotatercept is exceptional because it simultaneously targets SRC, ERK, AKT, and MLC by modulating SMAD-independent activin and BMP signaling, not mentioned in previous descriptions of the mechanism of action of sotatercept (97, 178).
4.5 Limitations of our methodology and future perspectives
When Arturo Rosenblueth and Norbert Wiener discussed the role of models in biology, they proposed that “The best material model for a cat is another or preferably the same cat.” However, for practical reasons, it is necessary to reduce the complexity of models until it is possible to analyze and understand them (204). PH is a complex disease that involves vasoconstriction, endothelial barrier function, vascular cell proliferation, and other important biological mechanisms including the molecules that regulate oxidative stress, coagulation, inflammation, mechanosensory mechanisms, and EC cytoskeleton remodeling, many of which are not included in our model. Moreover, the genetic mutations and the comorbidities that afflict each specific PH patient can affect the molecular mechanisms involved in PH. Due to the retrospective observational design of the present and the previous study from which we obtained metabolite data (16), we will discuss potential confounding factors of metabolite data analysis. Metabolite analysis can be influenced by several confounding factors as reviewed by (205). Such confounders include—among others—genetic background, disease subtype, comorbidities, background medication, nutrition, lifestyle factors, and combinations thereof. We have selected metabolite data from a 35-patient cohort that consists of an almost identical distribution of the three clinical PH groups IPAH, CTD-aPAH and CTEPH (16). Since each group is treated differently and specific pharmacological treatments may influence metabolite profiles, we chose to select differential metabolites across all rather than from within individual clinical groups. While this data selection might mitigate the potential effects of administered PH drugs, a potential confounding effect of PH and further medication on metabolite profiles cannot be excluded. Because data on the genetic background, nutrition, and lifestyle of individual patients was not available, we cannot exclude the possibility that such confounders have influenced the metabolic profiles. Larger multicenter prospective studies with metabolite measurements at several timepoints are needed to appropriately estimate the influence of individual confounders or combinations thereof on metabolomic profiles in PH patients. Additionally, from a cellular perspective, we focused on PAECs and PASMCs. Macrophages, platelets, fibroblasts, and other cell types are also important in PH. Further, PH leads to right ventricle hypertrophy and other pathological processes that occur in other organs. It would be interesting to include these additional biological mechanisms, cell types, and organs in a future version of our model. The effectiveness of graph algorithms is highly dependent on the accuracy, completeness, and quality of the knowledge graph. Incomplete or inaccurate graphs can lead to misleading results. Path-finding algorithms often prioritize the shortest path, which might not always represent the most meaningful or relevant connection between two nodes. Centrality measures might sometimes overly prioritize nodes that are merely popular or have more connections. Incorporating additional contextual data, like gene expression datasets or gene mutations, can guide path-finding algorithms more effectively. If the knowledge graph is not regularly updated with fresh information, it will become outdated, leading to analyses that no longer reflect the current state of knowledge or understanding in a particular field. Moving forward, we aim to employ graph embeddings to anticipate new connections between nodes, fostering the generation of novel hypotheses. Another important limitation of our methodology is that certain interactions between molecules and their effect have been observed only in other cell types, model organisms, or cells cultivated under conditions that differ from those encountered in vivo. Also, we assembled a topological model of the network of molecules involved in PH. These molecules constitute a dynamic system. Using a mathematical formalism like a Boolean network, a Petri net, or a set of ordinary differential equations would allow us to simulate and explore the behavior of this dynamic system including the effect of the different existing and novel pharmacological treatments. A mathematical formalism would also allow us to find important missing connections in the network (206). Lastly, modeling in general, including this study only allows us to summarize, analyze, and propose novel hypotheses. Importantly, all the hypotheses we generated require experimental validation.
5 Conclusions
Pulmonary hypertension (PH) constitutes a complex disease process affecting 1% of the global population. Despite major advances in the treatment, PH remains a devastating disease involving multiple pathophysiological mechanisms including genetic, molecular, and environmental factors. There is a pressing need for more potent, disease-modifying clinical therapies to enhance long-term outcomes for PH patients. A comprehensive review of the PH signaling pathways involved in cell proliferation, endothelial barrier disruption, vasoconstriction, and the Warburg effect is presented using the software platform Lifelike. The molecular mechanisms we have elucidated using Lifelike open a window for pharmacological repurposing. By identifying new actions for existing drugs and suggesting potential mechanisms for new drugs, we highlight new promising therapeutic targets for this devastating group of diseases.
Data availability statement
All the programs we used are open source and accessible to the reader. The modified version of the Reactome database that is suitable for graph data science research and the Lifelike source code is available at (29). The Python module for graph data science, including radiate analysis and tracing is stored in the repository https://github.com/SBRG/GDS-Public. A publicly accessible version of the Lifelike platform is available through the public portal https://public.lifelike.bio/ upon self-registration.
Ethics statement
Ethical approval was not required for the study involving humans in accordance with the local legislation and institutional requirements. Written informed consent to participate in this study was not required from the participants or the participants’ legal guardians/next of kin in accordance with the national legislation and the institutional requirements.
Author contributions
NW: Conceptualization, Data curation, Formal Analysis, Investigation, Methodology, Software, Validation, Visualization, Writing – original draft, Writing – review & editing. JC: Conceptualization, Formal Analysis, Funding acquisition, Investigation, Resources, Writing – review & editing. SS: Conceptualization, Data curation, Formal Analysis, Investigation, Methodology, Software, Visualization, Writing – original draft, Writing – review & editing. TS: Conceptualization, Data curation, Methodology, Software, Writing – review & editing. HHH: Conceptualization, Data curation, Formal Analysis, Validation, Writing – review & editing. ET: Conceptualization, Data curation, Formal Analysis, Funding acquisition, Investigation, Methodology, Resources, Software, Writing – review & editing. PIJ: Conceptualization, Data curation, Formal Analysis, Funding acquisition, Investigation, Methodology, Resources, Supervision, Validation, Writing – original draft, Writing – review & editing.
Funding
The authors declare financial support was received for the research, authorship, and/or publication of this article.
This work was funded by the Novo Nordisk Foundation, grant reference number NNF20OC0064556 to JC, and grant reference number NNF20CC0035580 to ET.
Acknowledgments
We thank Ethan Daniel Sanchez, Michael Siu, Dominik Mariusz Maszczyk, Robin Cai, Ewa Lis, David Regla Demaree, Sharon Wiback, Maciej Tomasz Grzelczak, and Christian Degnbol Madsen for the development and upkeep of Lifelike. We greatly appreciate the help of Rikke Stine Friis Fléron.
Conflict of interest
The authors declare that the research was conducted in the absence of any commercial or financial relationships that could be construed as a potential conflict of interest.
Publisher's note
All claims expressed in this article are solely those of the authors and do not necessarily represent those of their affiliated organizations, or those of the publisher, the editors and the reviewers. Any product that may be evaluated in this article, or claim that may be made by its manufacturer, is not guaranteed or endorsed by the publisher.
Supplementary material
The Supplementary Material for this article can be found online at: https://www.frontiersin.org/articles/10.3389/fcvm.2024.1341145/full#supplementary-material
References
1. Humbert M, Kovacs G, Hoeper MM, Badagliacca R, Berger RM, Brida M, et al., 2022 ESC/ERS guidelines for the diagnosis and treatment of pulmonary hypertension: developed by the task force for the diagnosis and treatment of pulmonary hypertension of the European society of cardiology (ESC) and the European respiratory society (ERS). Endorsed by the International Society for Heart and Lung Transplantation (ISHLT) and the European reference network on rare respiratory diseases (ERN-LUNG). Eur Heart J. (2022) 43:3618–731. doi: 10.1093/eurheartj/ehac237
2. Kovacs G, Dumitrescu D, Barner A, Greiner S, Grünig E, Hager A, et al., Definition, clinical classification and initial diagnosis of pulmonary hypertension: updated recommendations from the Cologne Consensus Conference 2018. Int J Cardiol. (2018) 272:11–9. doi: 10.1016/j.ijcard.2018.08.083
3. Simonneau G, Hoeper MM. The revised definition of pulmonary hypertension: exploring the impact on patient management. Eur Heart J Suppl. (2019) 21:K4–8. doi: 10.1093/eurheartj/suz211
4. Noordegraaf AV, Groeneveldt JA, Bogaard HJ. Pulmonary hypertension. Eur Respir Rev. (2016) 25:4–11. doi: 10.1183/16000617.0096-2015
5. Simonneau G, Montani D, Celermajer DS, Denton CP, Gatzoulis MA, Krowka M, et al., Haemodynamic definitions and updated clinical classification of pulmonary hypertension. Eur Respir J. (2019) 53:13. doi: 10.1183/13993003.01913-2018
6. Humbert M, Morrell NW, Archer SL, Stenmark KR, MacLean MR, Lang IM, et al., Cellular and molecular pathobiology of pulmonary arterial hypertension. J Am Coll Cardiol. (2004) 43:S13–24. doi: 10.1016/j.jacc.2004.02.029
7. Tuder RM, Groves B, Badesch DB, Voelkel NF. Exuberant endothelial cell growth and elements of inflammation are present in plexiform lesions of pulmonary hypertension. Am J Pathol. (1994) 144:275. 1887146; 7508683.7508683
8. Kurakula K, Smolders VF, Tura-Ceide O, Jukema JW, Quax PH, Goumans M-J. Endothelial dysfunction in pulmonary hypertension: cause or consequence? Biomedicines. (2021) 9:57. doi: 10.3390/biomedicines9010057
9. Budhiraja R, Tuder RM, Hassoun PM. Endothelial dysfunction in pulmonary hypertension. Circulation. (2004) 109:159–65. doi: 10.1161/01.CIR.0000102381.57477.50
10. Hadi HA, Carr CS, Al Suwaidi J. Endothelial dysfunction: cardiovascular risk factors, therapy, and outcome. Vasc Health Risk Manag. (2005) 1:183. 17319104; 1993955.17319104
11. Nakamura J, Tsujino I, Ohira H, Nakaya T, Sugimoto A, Sato T, et al., Various factors contribute to death in patients with different types of pulmonary hypertension: a retrospective pilot study from a single tertiary center. Respir Investig. (2022) 60:647–57. doi: 10.1016/j.resinv.2022.05.001
12. Kvietys PR, Granger DN. Endothelial cell monolayers as a tool for studying microvascular pathophysiology. Am J Physiol Gastrointest Liver Physiol. (1997) 273:G1189–99. doi: 10.1152/ajpgi.1997.273.6.G1189
13. Haymet AB, Bartnikowski N, Wood ES, Vallely MP, McBride A, Yacoub S, et al., Studying the endothelial glycocalyx in vitro: what is missing?. Front Cardiovasc Med. (2021) 8:647086. doi: 10.3389/fcvm.2021.647086
14. Bai H, Ingber DE. What can an organ-on-a-chip teach us about human lung pathophysiology? Physiology. (2022) 37(5):242–52. doi: 10.1152/physiol.00012.2022
15. Haensel M, Wojciak-Stothard B. The role of endothelial cells in pulmonary hypertension: old concepts and new science. Curr Opin Physiol. (2023) 34:100667. doi: 10.1016/j.cophys.2023.100667
16. Carlsen J, Henriksen H, Marin de Mas I, Johansson P. An explorative metabolomic analysis of the endothelium in pulmonary hypertension. Sci Rep. (2022) 12:1–9. doi: 10.1038/s41598-022-17374-x
17. Watson JD, Crick FH. Molecular structure of nucleic acids: a structure for deoxyribose nucleic acid. Nature. (1953) 171:737–8. doi: 10.1038/171737a0
18. Fiers W, Contreras R, Duerinck F, Haegeman G, Iserentant D, Merregaert J, et al., Complete nucleotide sequence of bacteriophage MS2 RNA: primary and secondary structure of the replicase gene. Nature. (1976) 260:500–7. doi: 10.1038/260500a0
19. Gao B, Chi L, Zhu Y, Shi X, Tu P, Li B, et al., An introduction to next generation sequencing bioinformatic analysis in gut microbiome studies. Biomolecules. (2021) 11:530. doi: 10.3390/biom11040530
20. Heather JM, Chain B. The sequence of sequencers: the history of sequencing DNA. Genomics. (2016) 107:1–8. doi: 10.1016/j.ygeno.2015.11.003
21. Holley RW, Apgar J, Everett GA, Madison JT, Marquisee M, Merrill SH, et al., Structure of a ribonucleic acid. Science. (1965) 147:1462–5. doi: 10.1126/science.147.3664.1462
22. Kukurba KR, Montgomery SB. RNA sequencing and analysis. Cold Spring Harb Protoc. (2015) 2015:951–69. doi: 10.1101/pdb.top084970
23. Aldridge S, Teichmann SA. Single cell transcriptomics comes of age. Nat Commun. (2020) 11:1–4. doi: 10.1038/s41467-020-18158-5
24. Aslam B, Basit M, Nisar MA, Khurshid M, Rasool MH. Proteomics: technologies and their applications. J Chromatogr Sci. (2017) 55:182–96. doi: 10.1093/chromsci/bmw167
25. Wishart DS. Metabolomics for investigating physiological and pathophysiological processes. Physiol Rev. (2019) 99:1819–75. doi: 10.1152/physrev.00035.2018
26. Arora I, Tollefsbol TO. Computational methods and next-generation sequencing approaches to analyze epigenetics data: profiling of methods and applications. Methods. (2021) 187:92–103. doi: 10.1016/j.ymeth.2020.09.008
27. Sudlow C, Gallacher J, Allen N, Beral V, Burton P, Danesh J, et al., UK biobank: an open access resource for identifying the causes of a wide range of complex diseases of middle and old age. PLoS Med. (2015) 12:e1001779. doi: 10.1371/journal.pmed.1001779
28. Hatzopoulos AK. Data from: Disease models & mechanisms in the age of big data. (2019). doi: 10.1242/dmm.041699
29. Schulz S, Sanchez ED, Siu M, Maszczyk DM, Weinstein N, Cai R, et al., Lifelike v0.98.1. (2023). doi: 10.5281/zenodo.6109727
30. Dhammi IK, Kumar S. Medical subject headings (MeSH) terms. Indian J Orthop. (2014) 48:443–4. doi: 10.4103/0019-5413.139827
31. Ashburner M, Ball CA, Blake JA, Botstein D, Butler H, Cherry JM, et al., Gene ontology: tool for the unification of biology. Nat Genet. (2000) 25:25–9. doi: 10.1038/75556
32. Gene Ontology Consortium, Aleksander SA, Balhoff J, Carbon S, Cherry JM, Drabkin HJ, et al. The gene ontology knowledgebase in 2023. Genetics. (2023) 224:iyad031. doi: 10.1093/genetics/iyad031
33. Federhen S. The NCBI taxonomy database. Nucleic Acids Res. (2012) 40:D136–43. doi: 10.1093/nar/gkr1178
34. Schoch CL, Ciufo S, Domrachev M, Hotton CL, Kannan S, Khovanskaya R, et al., NCBI taxonomy: a comprehensive update on curation, resources and tools. Database. (2020) 2020:baaa062. doi: 10.1093/database/baaa062
35. The UniProt Consortium. UniProt: the universal protein knowledgebase in 2023. Nucleic Acids Res. (2023) 51:D523–31. doi: 10.1093/nar/gkac1052
36. Szklarczyk D, Kirsch R, Koutrouli M, Nastou K, Mehryary F, Hachilif R, et al., The STRING database in 2023: protein–protein association networks and functional enrichment analyses for any sequenced genome of interest. Nucleic Acids Res. (2023) 51:D638–46. doi: 10.1093/nar/gkac1000
37. Duvaud S, Gabella C, Lisacek F, Stockinger H, Ioannidis V, Durinx C. Expasy, the Swiss bioinformatics resource portal, as designed by its users. Nucleic Acids Res. (2021) 49:W216–27. doi: 10.1093/nar/gkab225
38. Hastings J, Owen G, Dekker A, Ennis M, Kale N, Muthukrishnan V, et al., ChEBI in 2016: improved services and an expanding collection of metabolites. Nucleic Acids Res. (2016) 44:D1214–9. doi: 10.1093/nar/gkv1031
39. Percha B, Altman RB. A global network of biomedical relationships derived from text. Bioinformatics. (2018) 34:2614–24. doi: 10.1093/bioinformatics/bty114
40. Benjamini Y, Hochberg Y. Controlling the false discovery rate: a practical and powerful approach to multiple testing. J R Stat Soc Ser B. (1995) 57:289–300. doi: 10.1111/j.2517-6161.1995.tb02031.x
41. Fabregat A, Korninger F, Viteri G, Sidiropoulos K, Marin-Garcia P, Ping P, et al., Reactome graph database: efficient access to complex pathway data. PLoS Comput Biol. (2018) 14:e1005968. doi: 10.1371/journal.pcbi.1005968
42. Gillespie M, Jassal B, Stephan R, Milacic M, Rothfels K, Senff-Ribeiro A, et al., The reactome pathway knowledgebase 2022. Nucleic Acids Res. (2022) 50:D687–92. doi: 10.1093/nar/gkab1028
43. Ma H, Zeng A-P. Reconstruction of metabolic networks from genome data and analysis of their global structure for various organisms. Bioinformatics. (2003) 19:270–7. doi: 10.1093/bioinformatics/19.2.270
44. Martínez VS, Saa PA, Jooste J, Tiwari K, Quek L-E, Nielsen LK. The topology of genome-scale metabolic reconstructions unravels independent modules and high network flexibility. PLoS Comput Biol. (2022) 18:e1010203. doi: 10.1371/journal.pcbi.1010203
45. Bianchini M, Gori M, Scarselli F. Inside pagerank. ACM Transactions on Internet Technology. (2005) 5(1):92–128. doi: 10.1145/1052934.1052938
46. Brin S, Page L. The anatomy of a large-scale hypertextual web search engine. Comput Netw ISDN Syst. (1998) 30:107–17. doi: 10.1016/S0169-7552(98)00110-X
47. Iván G, Grolmusz V. When the web meets the cell: using personalized pagerank for analyzing protein interaction networks. Bioinformatics. (2011) 27:405–7. doi: 10.1093/bioinformatics/btq680
48. Yu B, Zhang K, Milner JJ, Toma C, Chen R, Scott-Browne JP, et al., Epigenetic landscapes reveal transcription factors that regulate CD8+ T cell differentiation. Nat Immunol. (2017) 18:573–82. doi: 10.1038/ni.3706
49. Zhang K, Wang M, Zhao Y, Wang W. Taiji: system-level identification of key transcription factors reveals transcriptional waves in mouse embryonic development. Sci Adv. (2019) 5:eaav3262. doi: 10.1126/sciadv.aav3262
50. Preston IR, Hill NS, Warburton RR, Fanburg BL. Role of 12-lipoxygenase in hypoxia-induced rat pulmonary artery smooth muscle cell proliferation. Am J Physiol Lung Cell Mol Physiol. (2006) 290:L367–74. doi: 10.1152/ajplung.00114.2005
51. Edwards A, Cao C, Pallone TL. Cellular mechanisms underlying nitric oxide-induced vasodilation of descending vasa recta. Am J Physiol Ren Physiol. (2011) 300:F441–56. doi: 10.1152/ajprenal.00499.2010
52. Murakami A, Takasugi H, Ohnuma S, Koide Y, Sakurai A, Takeda S, et al., Sphingosine 1-phosphate (S1P) regulates vascular contraction via S1P3 receptor: investigation based on a new s1p3 receptor antagonist. Mol Pharmacol. (2010) 77:704–13. doi: 10.1124/mol.109.061481
53. Natarajan V, Jayaram HN, Scribner WM, Garcia J. Activation of endothelial cell phospholipase d by sphingosine and sphingosine-1-phosphate. Am J Respir Cell Mol Biol. (1994) 11:221–9. doi: 10.1165/ajrcmb.11.2.8049083
54. Somlyo AP, Somlyo AV. Signal transduction by G-proteins, rho-kinase and protein phosphatase to smooth muscle and non-muscle myosin II. J Physiol (Lond). (2000) 522:177–85. doi: 10.1111/j.1469-7793.2000.t01-2-00177.x
55. Somlyo AP, Somlyo AV. Ca2+ sensitivity of smooth muscle and nonmuscle myosin II: modulated by G proteins, kinases, and myosin phosphatase. Physiol Rev. (2003) 83:1325–58. doi: 10.1152/physrev.00023.2003
56. Watterson KR, Ratz PH, Spiegel S. The role of sphingosine-1-phosphate in smooth muscle contraction. Cell Signal. (2005) 17:289–98. doi: 10.1016/j.cellsig.2004.09.013
57. Nguyen CH, Stadler S, Brenner S, Huttary N, Krieger S, Jäger W, et al., Cancer cell-derived 12 (S)-HETE signals via 12-HETE receptor, RHO, ROCK and MLC2 to induce lymph endothelial barrier breaching. Br J Cancer. (2016) 115:364–70. doi: 10.1038/bjc.2016.201
58. Tang DG, Timar J, Grossi IM, Renaud C, Kimler VA, Diglio CA, et al., The lipoxygenase metabolite, 12 (S)-HETE, induces a protein kinase c-dependent cytoskeletal rearrangement and retraction of microvascular endothelial cells. Exp Cell Res. (1993) 207:361–75. doi: 10.1006/excr.1993.1203
59. Li Q, Li Y, Lei C, Tan Y, Yi G. Sphingosine-1-phosphate receptor 3 signaling. Clin Chim Acta. (2021) 519:32–9. doi: 10.1016/j.cca.2021.03.025
60. Cantalupo A, Gargiulo A, Dautaj E, Liu C, Zhang Y, Hla T, et al., S1PR1 (sphingosine-1-phosphate receptor 1) signaling regulates blood flow and pressure. Hypertension. (2017) 70:426–34. doi: 10.1161/HYPERTENSIONAHA.117.09088
61. Pyne NJ, Pyne S. Sphingosine 1-phosphate receptor 1 signaling in mammalian cells. Molecules. (2017) 22:344. doi: 10.3390/molecules22030344
62. Taha TA, Argraves KM, Obeid LM. Sphingosine-1-phosphate receptors: receptor specificity versus functional redundancy. Biochim Biophys Acta. (2004) 1682:48–55. doi: 10.1016/j.bbalip.2004.01.006
63. Guo Y, Zhang W, Giroux C, Cai Y, Ekambaram P, Dilly A-K, et al., Identification of the orphan G protein-coupled receptor gpr31 as a receptor for 12-(S)-hydroxyeicosatetraenoic acid. J Biol Chem. (2011) 286:33832–40. doi: 10.1074/jbc.M110.216564
64. Amberg GC, Navedo MF. Calcium dynamics in vascular smooth muscle. Microcirculation. (2013) 20:281–9. doi: 10.1111/micc.12046
65. Caricasole A, Sala C, Roncarati R, Formenti E, Terstappen GC. Cloning and characterization of the human phosphoinositide-specific phospholipase C-beta 1 (PLC1). Biochim Biophys Acta. (2000) 1517:63–72. doi: 10.1016/S0167-4781(00)00260-8
66. Cullere X, Shaw SK, Andersson L, Hirahashi J, Luscinskas FW, Mayadas TN. Regulation of vascular endothelial barrier function by Epac, a cAMP-activated exchange factor for Rap GTPase. Blood. (2005) 105:1950–5. doi: 10.1182/blood-2004-05-1987
67. Julio-Pieper M, Flor PJ, Dinan TG, Cryan JF. Exciting times beyond the brain: metabotropic glutamate receptors in peripheral and non-neural tissues. Pharmacol Rev. (2011) 63:35–58. doi: 10.1124/pr.110.004036
68. Gill SS, Mueller RW, Mcguire PF, Pulido OM. Potential target sites in peripheral tissues for excitatory neurotransmission and excitotoxicity. Toxicol Pathol. (2000) 28:277–84. doi: 10.1177/01926233000280
69. Trepanier C, Lei G, Xie Y-F, MacDonald JF. Group II metabotropic glutamate receptors modify N-methyl-D-aspartate receptors via Src kinase. Sci Rep. (2013) 3:926. doi: 10.1038/srep00926
70. Verin AD, Gilbert-McClain LI, Patterson CE, Garcia JG. Biochemical regulation of the nonmuscle myosin light chain kinase isoform in bovine endothelium. Am J Respir Cell Mol Biol. (1998) 19:767–76. doi: 10.1165/ajrcmb.19.5.3126
71. Roskoski R Jr. Src protein-tyrosine kinase structure, mechanism, and small molecule inhibitors. Pharmacol Res. (2015) 94:9–25. doi: 10.1016/j.phrs.2015.01.003
72. Liu P, Gu Y, Luo J, Ye P, Zheng Y, Yu W, et al., Inhibition of src activation reverses pulmonary vascular remodeling in experimental pulmonary arterial hypertension via Akt/mTOR/HIF-1¡ alpha¿ signaling pathway. Exp Cell Res. (2019) 380:36–46. doi: 10.1016/j.yexcr.2019.02.022
73. Jain R, Watson U, Vasudevan L, Saini DK. ERK activation pathways downstream of GPCRs. Int Rev Cell Mol Biol. (2018) 338:79–109. doi: 10.1016/bs.ircmb.2018.02.003
74. Klein EA, Assoian RK. Transcriptional regulation of the cyclin D1 gene at a glance. J Cell Sci. (2008) 121:3853–7. doi: 10.1242/jcs.039131
75. Lavoie H, Gagnon J, Therrien M. ERK signalling: a master regulator of cell behaviour, life and fate. Nat Rev Mol Cell Biol. (2020) 21:607–32. doi: 10.1038/s41580-020-0255-7
76. Knock GA, Snetkov VA, Shaifta Y, Drndarski S, Ward JP, Aaronson PI. Role of src-family kinases in hypoxic vasoconstriction of rat pulmonary artery. Cardiovasc Res. (2008) 80:453–62. doi: 10.1093/cvr/cvn209
77. Orsenigo F, Giampietro C, Ferrari A, Corada M, Galaup A, Sigismund S, et al., Phosphorylation of VE-cadherin is modulated by haemodynamic forces and contributes to the regulation of vascular permeability in vivo. Nat Commun. (2012) 3:1208. doi: 10.1038/ncomms2199
78. Singer CA, Lontay B, Unruh H, Halayko AJ, Gerthoffer WT. Src mediates cytokine-stimulated gene expression in airway myocytes through ERK MAPK. Cell Commun Signal. (2011) 9:1–5. doi: 10.1186/1478-811X-9-14
79. Zhang R-G, Niu Y, Pan K-W, Pang H, Chen C-L, Yip C-Y, et al., 2-Adrenoceptor activation stimulates IL-6 production via PKA, ERK1/2, Src, and beta-arrestin2 signaling pathways in human bronchial epithelia. Lung. (2021) 199:619–27. doi: 10.1007/s00408-021-00484-0
80. Pullamsetti SS, Seeger W, Savai R. Classical IL-6 signaling: a promising therapeutic target for pulmonary arterial hypertension. J Clin Invest. (2018) 128:1720–3. doi: 10.1172/JCI120415
81. Simpson CE, Chen JY, Damico RL, Hassoun PM, Martin LJ, Yang J, et al., Cellular sources of interleukin-6 and associations with clinical phenotypes and outcomes in pulmonary arterial hypertension. Eur Respir J. (2020) 55:1901761. doi: 10.1183/13993003.01761-2019
82. Steiner MK, Syrkina OL, Kolliputi N, Mark EJ, Hales CA, Waxman AB. Interleukin-6 overexpression induces pulmonary hypertension. Circ Res. (2009) 104:236–44. doi: 10.1161/CIRCRESAHA.108.182014
83. Eulenfeld R, Dittrich A, Khouri C, Müller PJ, Mütze B, Wolf A, et al., Interleukin-6 signalling: more than jaks and STATs. Eur J Cell Biol. (2012) 91:486–95. doi: 10.1016/j.ejcb.2011.09.010
84. Hirano T, Ishihara K, Hibi M. Roles of STAT3 in mediating the cell growth, differentiation and survival signals relayed through the il-6 family of cytokine receptors. Oncogene. (2000) 19:2548–56. doi: 10.1038/sj.onc.1203551
85. Alsaffar H, Martino N, Garrett JP, Adam AP. Interleukin-6 promotes a sustained loss of endothelial barrier function via Janus kinase-mediated STAT3 phosphorylation and de novo protein synthesis. Am J Physiol Cell Physiol. (2018) 314:C589–602. doi: 10.1152/ajpcell.00235.2017
86. Wallez Y, Huber P. Endothelial adherens and tight junctions in vascular homeostasis, inflammation and angiogenesis. Biochim Biophys Acta. (2008) 1778:794–809. doi: 10.1016/j.bbamem.2007.09.003
87. Yun J-H, Park SW, Kim K-J, Bae J-S, Lee EH, Paek SH, et al., Endothelial STAT3 activation increases vascular leakage through downregulating tight junction proteins: implications for diabetic retinopathy. J Cell Physiol. (2017) 232:1123–34. doi: 10.1002/jcp.25575
88. Jung JE, Lee H-G, Cho I-H, Chung DH, Yoon S-H, Yang YM, et al., STAT3 is a potential modulator of HIF-1-mediated VEGF expression in human renal carcinoma cells. FASEB J. (2005) 19:1296–8. doi: 10.1096/fj.04-3099fje
89. Papapetropoulos A, Fulton D, Mahboubi K, Kalb RG, O’Connor DS, Li F, et al., Angiopoietin-1 inhibits endothelial cell apoptosis via the Akt/survivin pathway. J Biol Chem. (2000) 275:9102–5. doi: 10.1074/jbc.275.13.9102
90. Kolliputi N, Waxman AB. IL-6 cytoprotection in hyperoxic acute lung injury occurs via PI3K/Akt-mediated Bax phosphorylation. Am J Physiol Lung Cell Mol Physiol. (2009) 297:6–16. doi: 10.1152/ajplung.90381.2008
91. Kulikauskas MR, Bautch VL. The versatility and paradox of BMP signaling in endothelial cell behaviors and blood vessel function. Cell Mol Life Sci. (2022) 79:77. doi: 10.1007/s00018-021-04033-z
92. van Meeteren LA, Ten Dijke P. Regulation of endothelial cell plasticity by TGF-. Cell Tissue Res. (2012) 347:177–86. doi: 10.1007/s00441-011-1222-6
93. Austin ED, Loyd JE. The genetics of pulmonary arterial hypertension. Circ Res. (2014) 115:189–202. doi: 10.1161/CIRCRESAHA.115.303404
94. Tielemans B, Delcroix M, Belge C, Quarck R. TGF and BMPRII signalling pathways in the pathogenesis of pulmonary arterial hypertension. Drug Discov Today. (2019) 24:703–16. doi: 10.1016/j.drudis.2018.12.001
95. Upton PD, Davies RJ, Trembath RC, Morrell NW. Bone morphogenetic protein (BMP) and activin type II receptors balance BMP9 signals mediated by activin receptor-like kinase-1 in human pulmonary artery endothelial cells. J Biol Chem. (2009) 284:15794–804. doi: 10.1074/jbc.M109.002881
96. Ryanto GR, Ikeda K, Miyagawa K, Tu L, Guignabert C, Humbert M, et al., An endothelial activin A-bone morphogenetic protein receptor type 2 link is overdriven in pulmonary hypertension. Nat Commun. (2021) 12:1720. doi: 10.1038/s41467-021-21961-3
97. Humbert M, McLaughlin V, Gibbs JSR, Gomberg-Maitland M, Hoeper MM, Preston IR, et al., Sotatercept for the treatment of pulmonary arterial hypertension. New Engl J Med. (2021) 384:1204–15. doi: 10.1056/NEJMoa2024277
98. Yndestad A, Larsen K-O, Øie E, Ueland T, Smith C, Halvorsen B, et al., Elevated levels of activin A in clinical and experimental pulmonary hypertension. J Appl Physiol Respir Environ Exerc Physiol. (2009) 106:1356–64. doi: 10.1152/japplphysiol.90719.2008
99. Kaneda H, Arao T, Matsumoto K, De Velasco M, Tamura D, Aomatsu K, et al., Activin A inhibits vascular endothelial cell growth and suppresses tumour angiogenesis in gastric cancer. Br J Cancer. (2011) 105:1210–7. doi: 10.1038/bjc.2011.348
100. Yagi K, Furuhashi M, Aoki H, Goto D, Kuwano H, Sugamura K, et al., c-myc Is a downstream target of the smad pathway* 210. J Biol Chem. (2002) 277:854–61. doi: 10.1074/jbc.M104170200
101. Chang AC, Fu Y, Garside VC, Niessen K, Chang L, Fuller M, et al., Notch initiates the endothelial-to-mesenchymal transition in the atrioventricular canal through autocrine activation of soluble guanylyl cyclase. Dev Cell. (2011) 21:288–300. doi: 10.1016/j.devcel.2011.06.022
102. Jiang L, Qi Y, Kong X, Wang R, Qi J, Lin F, et al., Activin A as a novel chemokine induces migration of L929 fibroblasts by ERK signaling in microfluidic devices. Front Cell Dev Biol. (2021) 9:660316. doi: 10.3389/fcell.2021.660316
103. Zhang L, Xu P, Wang X, Zhang M, Yan Y, Chen Y, et al., Activin B regulates adipose-derived mesenchymal stem cells to promote skin wound healing via activation of the MAPK signaling pathway. Int J Biochem Cell Biol. (2017) 87:69–76. doi: 10.1016/j.biocel.2017.04.004
104. Zhang M, Sun L, Wang X, Chen S, Kong Y, Liu N, et al., Activin B promotes BMSC-mediated cutaneous wound healing by regulating cell migration via the JNK—ERK signaling pathway. Cell Transplant. (2014) 23:1061–73. doi: 10.3727/096368913X666
105. Conery AR, Cao Y, Thompson EA, Townsend CM Jr, Ko TC, Luo K. Akt interacts directly with Smad3 to regulate the sensitivity to TGF--induced apoptosis. Nat Cell Biol. (2004) 6:366–72. doi: 10.1038/ncb1117
106. Sysol JR, Natarajan V, Machado RF. PDGF induces Sphk1 expression via Egr-1 to promote pulmonary artery smooth muscle cell proliferation. Am J Physiol Cell Physiol. (2016) 310:C983–92. doi: 10.1152/ajpcell.00059.2016
107. Wang D, Uhrin P, Mocan A, Waltenberger B, Breuss JM, Tewari D, et al., Vascular smooth muscle cell proliferation as a therapeutic target. Part 1: molecular targets and pathways. Biotechnol Adv. (2018) 36:1586–607. doi: 10.1016/j.biotechadv.2018.04.006
108. Xing J, Wang M, Hong J, Gao Y, Liu Y, Gu H, et al., TRPM7 channel inhibition exacerbates pulmonary arterial hypertension through MEK/ERK pathway. Aging. (2019) 11:4050. doi: 10.18632/aging.102036
109. Wang J, Feng W, Li F, Shi W, Zhai C, Li S, et al., Sphk1/S1P mediates TGF-1-induced proliferation of pulmonary artery smooth muscle cells and its potential mechanisms. Pulm Circ. (2018) 9:2045894018816977. doi: 10.1177/2045894018816977
110. Rodríguez-Pascual F, Reimunde FM, Redondo-Horcajo M, Lamas S. Transforming growth factor- induces endothelin-1 expression through activation of the Smad signaling pathway. J Cardiovasc Pharmacol. (2004) 44:S39–42. doi: 10.1097/01.fjc.0000166216.27544.22
111. de Vinuesa AG, Abdelilah-Seyfried S, Knaus P, Zwijsen A, Bailly S. BMP signaling in vascular biology and dysfunction. Cytokine Growth Factor Rev. (2016) 27:65–79. doi: 10.1016/j.cytogfr.2015.12.005
112. Anderson L, Lowery JW, Frank DB, Novitskaya T, Jones M, Mortlock DP, et al., Bmp2 and Bmp4 exert opposing effects in hypoxic pulmonary hypertension. Am J Physiol Regul Integr Comp Physiol. (2010) 298:R833–42. doi: 10.1152/ajpregu.00534.2009
113. Wong WK, Knowles JA, Morse JH. Bone morphogenetic protein receptor type II C-terminus interacts with c-Src: implication for a role in pulmonary arterial hypertension. Am J Respir Cell Mol Biol. (2005) 33:438–46. doi: 10.1165/rcmb.2005-0103OC
114. Zhang C, Lv J, He Q, Wang S, Gao Y, Meng A, et al., Inhibition of endothelial ERK signalling by Smad1/5 is essential for haematopoietic stem cell emergence. Nat Commun. (2014) 5:3431. doi: 10.1038/ncomms443
115. Lowery JW, Frump AL, Anderson L, DiCarlo GE, Jones MT, de Caestecker MP. ID family protein expression and regulation in hypoxic pulmonary hypertension. Am J Physiol Regul Integr Comp Physiol. (2010) 299:R1463–77. doi: 10.1152/ajpregu.00866.2009
116. Long L, Ormiston ML, Yang X, Southwood M, Gräf S, Machado RD, et al., Selective enhancement of endothelial BMPR-II with BMP9 reverses pulmonary arterial hypertension. Nat Med. (2015) 21:777–85. doi: 10.1038/nm.3877
117. Yang P, Troncone L, Augur ZM, Kim SS, McNeil ME, Paul BY. The role of bone morphogenetic protein signaling in vascular calcification. Bone. (2020) 141:115542. doi: 10.1016/j.bone.2020.115542
118. Ye D, Liu Y, Pan H, Feng Y, Lu X, Gan L, et al., Insights into bone morphogenetic proteins in cardiovascular diseases. Front Pharmacol. (2023) 14:1125642. doi: 10.3389/fphar.2023.1125642
119. Hodgson J, Swietlik EM, Salmon RM, Hadinnapola C, Nikolic I, Wharton J, et al., Characterization of GDF2 mutations and levels of BMP9 and BMP10 in pulmonary arterial hypertension. Am J Respir Crit Care Med. (2020) 201:575–85. doi: 10.1164/rccm.201906-1141OC
120. Theilmann AL, Hawke LG, Hilton LR, Whitford MK, Cole DV, Mackeil JL, et al., Endothelial BMPR2 loss drives a proliferative response to BMP (bone morphogenetic protein) 9 via prolonged canonical signaling. Arterioscler Thromb Vasc Biol. (2020) 40:2605–18. doi: 10.1161/ATVBAHA.119.313357
121. Akhter MZ, Chandra Joshi J, Balaji Ragunathrao VA, Maienschein-Cline M, Proia RL, Malik AB, et al., Programming to S1PR1+ endothelial cells promotes restoration of vascular integrity. Circ Res. (2021) 129:221–36. doi: 10.1161/CIRCRESAHA.120.318412
122. Chung J, Uchida E, Grammer TC, Blenis J. STAT3 serine phosphorylation by ERK-dependent and-independent pathways negatively modulates its tyrosine phosphorylation. Mol Cell Biol. (1997) 17:6508–16. doi: 10.1128/MCB.17.11.6508
123. Duru EA, Fu Y, Davies MG. SRC regulates sphingosine-1-phosphate mediated smooth muscle cell migration. J Surg Res. (2012) 175:30–4. doi: 10.1016/j.jss.2011.07.021
124. Burch ML, Getachew R, Osman N, Febbraio MA, Little PJ. Thrombin-mediated proteoglycan synthesis utilizes both protein-tyrosine kinase and serine/threonine kinase receptor transactivation in vascular smooth muscle cells. J Biol Chem. (2013) 288:7410–9. doi: 10.1074/jbc.M112.400259
125. Keller CD, Gil PR, Tölle M, van der Giet M, Chun J, Radeke HH, et al., Immunomodulator FTY720 induces myofibroblast differentiation via the lysophospholipid receptor S1P3 and Smad3 signaling. Am J Pathol. (2007) 170:281–92. doi: 10.2353/ajpath.2007.060485
126. Little PJ, Burch ML, Getachew R, Al-Aryahi S, Osman N. Endothelin-1 stimulation of proteoglycan synthesis in vascular smooth muscle is mediated by endothelin receptor transactivation of the transforming growth factor- type I receptor. J Cardiovasc Pharmacol. (2010) 56:360–8. doi: 10.1097/FJC.0b013e3181ee6811
127. Sharifat N, Mohammad Zadeh G, Ghaffari M-A, Dayati P, Kamato D, Little PJ, et al., Endothelin-1 (ET-1) stimulates carboxy terminal Smad2 phosphorylation in vascular endothelial cells by a mechanism dependent on ET receptors and de novo protein synthesis. J Pharm Pharmacol. (2017) 69:66–72. doi: 10.1111/jphp.12654
128. Mohamed R, Janke R, Guo W, Cao Y, Zhou Y, Zheng W, et al., GPCR transactivation signalling in vascular smooth muscle cells: role of NADPH oxidases and reactive oxygen species. Vasc Biol. (2019) 1:1–11. doi: 10.1530/VB-18-0004
129. Itoh Y, Saitoh M, Miyazawa K. Smad3–STAT3 crosstalk in pathophysiological contexts. Acta Biochim Biophys Sin (Shanghai). (2018) 50:82–90. doi: 10.1093/abbs/gmx118
130. Makino Y, Yoon J-H, Bae E, Kato M, Miyazawa K, Ohira T, et al., Repression of Smad3 by Stat3 and c-Ski/SnoN induces gefitinib resistance in lung adenocarcinoma. Biochem Biophys Res Commun. (2017) 484:269–77. doi: 10.1016/j.bbrc.2017.01.093
131. Luwor R, Baradaran B, Taylor L, Iaria J, Nheu T, Amiry N, et al., Targeting Stat3 and Smad7 to restore TGF- cytostatic regulation of tumor cells in vitro and in vivo. Oncogene. (2013) 32:2433–41. doi: 10.1038/onc.2012.260
132. von Gersdorff G, Susztak K, Rezvani F, Bitzer M, Liang D, Böttinger EP. Smad3 and Smad4 mediate transcriptional activation of the human Smad7 promoter by transforming growth factor . J Biol Chem. (2000) 275:11320–6. doi: 10.1074/jbc.275.15.11320
133. Wang G, Yu Y, Sun C, Liu T, Liang T, Zhan L, et al., STAT3 selectively interacts with Smad3 to antagonize tgf-. Oncogene. (2016) 35:4388–98. doi: 10.1038/onc.2015.446
134. Lin L, Yao Z, Bhuvaneshwar K, Gusev Y, Kallakury B, Yang S, et al., Transcriptional regulation of STAT3 by SPTBN1 and SMAD3 in HCC through cAMP-response element-binding proteins ATF3 and CREB2. Carcinogenesis. (2014) 35:2393–403. doi: 10.1093/carcin/bgu163
135. Weinstein N, Mendoza L, Álvarez-Buylla ER. A computational model of the endothelial to mesenchymal transition. Front Genet. (2020) 11:40. doi: 10.3389/fgene.2020.00040
136. Mitchell JA, Kirkby NS. Eicosanoids, prostacyclin and cyclooxygenase in the cardiovascular system. Br J Pharmacol. (2019) 176:1038–50. doi: 10.1111/bph.14167
137. Dorris SL, Peebles RS. PGI2 as a regulator of inflammatory diseases. Mediat Inflamm. (2012) 2012:926968. doi: 10.1155/2012/926968
138. Katsuyama M, Sugimoto Y, Namba T, Irie A, Negishi M, Narumiya S, et al., Cloning and expression of a cDNA for the human prostacyclin receptor. FEBS Lett. (1994) 344:74–8. doi: 10.1016/0014-5793(94)00355-6
139. Clapp LH, Finney P, Turcato S, Tran S, Rubin LJ, Tinker A. Differential effects of stable prostacyclin analogs on smooth muscle proliferation and cyclic AMP generation in human pulmonary artery. Am J Respir Cell Mol Biol. (2002) 26:194–201. doi: 10.1165/ajrcmb.26.2.4695
140. Stockert J, Adhikary T, Kaddatz K, Finkernagel F, Meissner W, Müller-Brüsselbach S, et al., Reverse crosstalk of TGF and PPAR/ signaling identified by transcriptional profiling. Nucleic Acids Res. (2011) 39:119–31. doi: 10.1093/nar/gkq773
141. Mitchell JA, Ahmetaj-Shala B, Kirkby NS, Wright WR, Mackenzie LS, Reed DM, et al., Role of prostacyclin in pulmonary hypertension. Glob Cardiol Sci Pract. (2015) 2014:53. doi: 10.5339/gcsp.2014.53
142. Stitham J, Midgett C, Martin KA, Hwa J. Prostacyclin: an inflammatory paradox. Front Pharmacol. (2011) 2:24. doi: 10.3389/fphar.2011.00024
143. Toshner M, Rothman AM. Data from: IL-6 in pulmonary hypertension: why novel is not always best. (2020). doi: 10.1183/13993003.00314-2020
144. Prisco SZ, Hartweck LM, Rose L, Lima PD, Thenappan T, Archer SL, et al., Inflammatory glycoprotein 130 signaling links changes in microtubules and junctophilin-2 to altered mitochondrial metabolism and right ventricular contractility. Circ Heart Fail. (2022) 15:e008574. doi: 10.1161/CIRCHEARTFAILURE.121.008574
145. Xu W-J, Wu Q, He W-N, Wang S, Zhao Y-L, Huang J-X, et al., Interleukin-6 and pulmonary hypertension: from physiopathology to therapy. Front Immunol. (2023) 14:1181987. doi: 10.3389/fimmu.2023.1181987
146. Ghofrani HA, Osterloh IH, Grimminger F. Sildenafil: from angina to erectile dysfunction to pulmonary hypertension and beyond. Nat Rev Drug Discov. (2006) 5:689–702. doi: 10.1038/nrd2030
147. Prasad S, Wilkinson J, Gatzoulis MA. Sildenafil in primary pulmonary hypertension. New Engl J Med. (2000) 343:1342. doi: 10.1056/NEJM200011023431814
148. Falk JA, Philip KJ, Schwarz ER. The emergence of oral tadalafil as a once-daily treatment for pulmonary arterial hypertension. Vasc Health Risk Manag. (2010) 6:273–80. doi: 10.2147/vhrm.s6392
149. Lincoln TM, Dey N, Sellak H. Invited review: cGMP-dependent protein kinase signaling mechanisms in smooth muscle: from the regulation of tone to gene expression. J Appl Physiol Respir Environ Exerc Physiol. (2001) 91:1421–30. doi: 10.1152/jappl.2001.91.3.1421
150. Toxvig AK, Wehland M, Grimm D, Infanger M, Krüger M. A focus on riociguat in the treatment of pulmonary arterial hypertension. Basic Clin Pharmacol Toxicol. (2019) 125:202–14. doi: 10.1111/bcpt.13272
151. Giaid A, Yanagisawa M, Langleben D, Michel RP, Levy R, Shennib H, et al., Expression of endothelin-1 in the lungs of patients with pulmonary hypertension. New Engl J Med. (1993) 328:1732–9. doi: 10.1056/NEJM199306173282402
152. Watanabe D, Takagi H, Suzuma K, Suzuma I, Oh H, Ohashi H, et al., Transcription factor Ets-1 mediates ischemia-and vascular endothelial growth factor-dependent retinal neovascularization. Am J Pathol. (2004) 164:1827–35. doi: 10.1016/S0002-9440(10)63741-8
153. Luscher TF, Barton M. Endothelins and endothelin receptor antagonists: therapeutic considerations for a novel class of cardiovascular drugs. Circulation. (2000) 102:2434–40. doi: 10.1161/01.CIR.102.19.2434
154. Zhang Y, Xu C-B. The roles of endothelin and its receptors in cigarette smoke-associated pulmonary hypertension with chronic lung disease. Pathol Res Pract. (2020) 216:153083. doi: 10.1016/j.prp.2020.153083
155. D’Orleans-Juste P, Labonte J, Bkaily G, Choufani S, Plante M, Honore J. Function of the endothelinb receptor in cardiovascular physiology and pathophysiology. Pharmacol Ther. (2002) 95:221–38. doi: 10.1016/S0163-7258(02)00235-8
156. Sauvageau S, Thorin E, Caron A, Dupuis J. Endothelin-1-induced pulmonary vasoreactivity is regulated by ETA and ETB receptor interactions. J Vasc Res. (2007) 44:375–81. doi: 10.1159/000102534
157. Doi T, Sugimoto H, Arimoto I, Hiroaki Y, Fujiyoshi Y. Interactions of endothelin receptor subtypes A and B with Gi, Go, and Gq in reconstituted phospholipid vesicles. Biochemistry. (1999) 38:3090–9. doi: 10.1021/bi981919m
158. Gohla A, Schultz G, Offermanns S. Role for G12/G13 in agonist-induced vascular smooth muscle cell contraction. Circ Res. (2000) 87:221–7. doi: 10.1161/01.RES.87.3.221
159. Horinouchi T, Terada K, Higashi T, Miwa S. Endothelin receptor signaling: new insight into its regulatory mechanisms. J Pharmacol Sci. (2013) 123:85–101. doi: 10.1254/jphs.13R02CR
160. Zamora MA, Dempsey EC, Walchak SJ, Stelzner TJ. BQ-123, an ETA receptor antagonist, inhibits endothelin-l-mediated proliferation of human pulmonary artery smooth muscle cells. Am J Respir Cell Mol Biol. (1993) 9:429–33. doi: 10.1165/ajrcmb/9.4.429
161. Yahiaoui L, Villeneuve A, Valderrama-Carvajal H, Burke F, Fixman ED. Endothelin-1 regulates proliferative responses, both alone and synergistically with PDGF, in rat tracheal smooth muscle cells. Cell Physiol Biochem. (2006) 17:37–46. doi: 10.1159/000091462
162. Marziano C, Hong K, Cope EL, Kotlikoff MI, Isakson BE, Sonkusare SK. Nitric oxide–dependent feedback loop regulates transient receptor potential vanilloid 4 (trpv 4) channel cooperativity and endothelial function in small pulmonary arteries. J Am Heart Assoc. (2017) 6:e007157. doi: 10.1161/JAHA.117.007157
163. Mazzuca MQ, Khalil RA. Vascular endothelin receptor type B: structure, function and dysregulation in vascular disease. Biochem Pharmacol. (2012) 84:147–62. doi: 10.1016/j.bcp.2012.03.020
164. Clozel M, Breu V, Gray G, Kalina B, Löffler B, Burri K, et al., Pharmacological characterization of bosentan, a new potent orally active nonpeptide endothelin receptor antagonist. J Pharmacol Exp Ther. (1994) 270:228–35. 8035319.8035319
165. McLaughlin V, Sitbon O, Badesch D, Barst R, Black C, Galiè N, et al., Survival with first-line bosentan in patients with primary pulmonary hypertension. Eur Respir J. (2005) 25:244–9. doi: 10.1183/09031936.05.00054804
166. Rubin LJ, Badesch DB, Barst RJ, Galie N, Black CM, Keogh A, et al., Bosentan therapy for pulmonary arterial hypertension. New Engl J Med. (2002) 346:896–903. doi: 10.1056/NEJMoa012212
167. Kholdani CA, Fares WH, Trow TK. Macitentan for the treatment of pulmonary arterial hypertension. Vasc Health Risk Manag. (2014) 10:665–73. doi: 10.2147/VHRM.S33904
168. Galiè N, Olschewski H, Oudiz RJ, Torres F, Frost A, Ghofrani HA, et al., Ambrisentan for the treatment of pulmonary arterial hypertension: results of the ambrisentan in pulmonary arterial hypertension, randomized, double-blind, placebo-controlled, multicenter, efficacy (ARIES) study 1 and 2. Circulation. (2008) 117:3010–9. doi: 10.1161/CIRCULATIONAHA.107.742510
169. Trow TK, Taichman DB. Endothelin receptor blockade in the management of pulmonary arterial hypertension: selective and dual antagonism. Respir Med. (2009) 103:951–62. doi: 10.1016/j.rmed.2009.02.016
170. Aykul S, Martinez-Hackert E. Transforming growth factor- family ligands can function as antagonists by competing for type II receptor binding. J Biol Chem. (2016) 291:10792–804. doi: 10.1074/jbc.M115.713487
171. El-Bizri N, Wang L, Merklinger SL, Guignabert C, Desai T, Urashima T, et al. Smooth muscle protein 22–mediated patchy deletion of Bmpr1a impairs cardiac contractility but protects against pulmonary vascular remodeling. Circ Res. (2008) 102:380–8. doi: 10.1161/CIRCRESAHA.107.161059
172. Rizvi F, Siddiqui R, DeFranco A, Homar P, Emelyanova L, Holmuhamedov E, et al., Simvastatin reduces TGF-1-induced SMAD2/3-dependent human ventricular fibroblasts differentiation: role of protein phosphatase activation. Int J Cardiol. (2018) 270:228–36. doi: 10.1016/j.ijcard.2018.06.061
173. Wilkins MR, Ali O, Bradlow W, Wharton J, Taegtmeyer A, Rhodes CJ, et al., Simvastatin as a treatment for pulmonary hypertension trial. Am J Respir Crit Care Med. (2010) 181:1106–13. doi: 10.1164/rccm.200911-1699OC
174. Spiekerkoetter E, Tian X, Cai J, Hopper RK, Sudheendra D, Li CG, et al., FK506 activates BMPR2, rescues endothelial dysfunction, and reverses pulmonary hypertension. J Clin Invest. (2013) 123:3600–13. doi: 10.1172/JCI65592
175. Spiekerkoetter E, Sung YK, Sudheendra D, Bill M, Aldred MA, van de Veerdonk MC, et al., Low-dose FK506 (tacrolimus) in end-stage pulmonary arterial hypertension. Am J Respir Crit Care Med. (2015) 192:254–7. doi: 10.1164/rccm.201411-2061LE
176. Spiekerkoetter E, Sung YK, Sudheendra D, Scott V, Del Rosario P, Bill M, et al., Randomised placebo-controlled safety and tolerability trial of FK506 (tacrolimus) for pulmonary arterial hypertension. Eur Respir J. (2017) 50:1602449. doi: 10.1183/13993003.02449-2016
177. Yan X, Wang J, Zhu Y, Feng W, Zhai C, Liu L, et al., S1P induces pulmonary artery smooth muscle cell proliferation by activating calcineurin/NFAT/OPN signaling pathway. Biochem Biophys Res Commun. (2019) 516:921–7. doi: 10.1016/j.bbrc.2019.06.160
178. Raje N, Vallet S. Sotatercept, a soluble activin receptor type 2A IgG-Fc fusion protein for the treatment of anemia and bone loss. Curr Opin Mol Ther. (2010) 12:586–97. 20886391.20886391
179. Hoeper MM, Badesch DB, Ghofrani HA, Gibbs JSR, Gomberg-Maitland M, McLaughlin VV, et al., Phase 3 trial of sotatercept for treatment of pulmonary arterial hypertension. New Engl J Med. (2023) 388:1478–90. doi: 10.1056/NEJMoa2213558
180. Noskovičová N, Petřek M, Eickelberg O, Heinzelmann K. Platelet-derived growth factor signaling in the lung. from lung development and disease to clinical studies. Am J Respir Cell Mol Biol. (2015) 52:263–84. doi: 10.1165/rcmb.2014-0294TR
181. Schupp JC, Adams TS, Cosme C Jr, Raredon MSB, Yuan Y, Omote N, et al., Integrated single-cell atlas of endothelial cells of the human lung. Circulation. (2021) 144:286–302. doi: 10.1161/CIRCULATIONAHA.120.052318
182. Li F, Wang J, Zhu Y, Liu L, Feng W, Shi W, et al., Sphk1/S1P mediates PDGF-induced pulmonary arterial smooth muscle cell proliferation via mir-21/bmprii/id1 signaling pathway. Cell Physiol Biochem. (2018) 51:487–500. doi: 10.1159/000495243
183. Huang K, Huang J, Chen C, Hao J, Wang S, Huang J, et al., AP-1 regulates sphingosine kinase 1 expression in a positive feedback manner in glomerular mesangial cells exposed to high glucose. Cell Signal. (2014) 26:629–38. doi: 10.1016/j.cellsig.2013.12.002
184. Takahashi J, Orcholski M, Yuan K, de Jesus Perez V. PDGF-dependent -catenin activation is associated with abnormal pulmonary artery smooth muscle cell proliferation in pulmonary arterial hypertension. FEBS Lett. (2016) 590:101–9. doi: 10.1002/1873-3468.12038
185. Xiao Y, Peng H, Hong C, Chen Z, Deng X, Wang A, et al., PDGF promotes the warburg effect in pulmonary arterial smooth muscle cells via activation of the PI3K/AKT/mTOR/HIF-1 signaling pathway. Cell Physiol Biochem. (2017) 42:1603–13. doi: 10.1159/000479401
186. Archer SL. Pyruvate kinase and warburg metabolism in pulmonary arterial hypertension: uncoupled glycolysis and the cancer-like phenotype of pulmonary arterial hypertension. Circulation. (2017) 136:2486–90. doi: 10.1161/CIRCULATIONAHA.117.031655
187. Frost AE, Barst RJ, Hoeper MM, Chang H-J, Frantz RP, Fukumoto Y, et al., Long-term safety and efficacy of imatinib in pulmonary arterial hypertension. J Heart Lung Transplant. (2015) 34:1366–75. doi: 10.1016/j.healun.2015.05.025
188. Frantz RP, Benza RL, Channick RN, Chin K, Howard LS, McLaughlin VV, et al., TORREY, a Phase 2 study to evaluate the efficacy and safety of inhaled seralutinib for the treatment of pulmonary arterial hypertension. Pulm Circ. (2021) 11:1–7. doi: 10.1177/20458940211057071
189. Galkin A, Sitapara R, Clemons B, Garcia E, Kennedy M, Guimond D, et al., Inhaled seralutinib exhibits potent efficacy in models of pulmonary arterial hypertension. Eur Respir J. (2022) 60:2102356. doi: 10.1183/13993003.02356-2021
190. Duval MX. The inadequacy of the reductionist approach in discovering new therapeutic agents against complex diseases. Exp Biol Med. (2018) 243:1004–13. doi: 10.1177/1535370218794365
191. Joshi A, Rienks M, Theofilatos K, Mayr M. Systems biology in cardiovascular disease: a multiomics approach. Nat Rev Cardiol. (2021) 18:313–30. doi: 10.1038/s41569-020-00477-1
192. Mazzocchi F. Complementarity in biology: a reassessment in relation to molecular-reductionist and systemic approaches. EMBO Rep. (2010) 11:339–44. doi: 10.1038/embor.2010.56
193. Wang R-S, Maron BA, Loscalzo J. Systems medicine: evolution of systems biology from bench to bedside. Wiley Interdiscip Rev Syst Biol Med. (2015) 7:141–61. doi: 10.1002/wsbm.1297
194. Azpeitia E, Muñoz S, González-Tokman D, Martínez-Sánchez ME, Weinstein N, Naldi A, et al., The combination of the functionalities of feedback circuits is determinant for the attractors’ number and size in pathway-like boolean networks. Sci Rep. (2017) 7:42023. doi: 10.1038/srep42023
195. Gouzé J-L. Positive and negative circuits in dynamical systems. J Biol Syst. (1998) 6:11–5. doi: 10.1142/S0218339098000054
196. Snoussi EH. Necessary conditions for multistationarity and stable periodicity. J Biol Syst. (1998) 6:3–9. doi: 10.1142/S0218339098000042
197. Nistala GJ, Wu K, Rao CV, Bhalerao KD. A modular positive feedback-based gene amplifier. J Biol Eng. (2010) 4:1–8. doi: 10.1186/1754-1611-4-4
198. Pigolotti S, Krishna S, Jensen MH. Oscillation patterns in negative feedback loops. Proc Natl Acad Sci. (2007) 104:6533–7. doi: 10.1073/pnas.061075910
199. Richard A, Comet J-P. Stable periodicity and negative circuits in differential systems. J Math Biol. (2011) 63:593–600. doi: 10.1007/s00285-010-0388-y
200. El-Samad H. Biological feedback control—respect the loops. Cell Syst. (2021) 12:477–87. doi: 10.1016/j.cels.2021.05.004
201. Kitano H. A robustness-based approach to systems-oriented drug design. Nat. Rev Drug Discov. (2007) 6:202–10. doi: 10.1038/nrd2195
202. Shah AJ, Beckmann T, Vorla M, Kalra DK. New drugs and therapies in pulmonary arterial hypertension. Int J Mol Sci. (2023) 24:5850. doi: 10.3390/ijms24065850
203. Humbert M, Sitbon O, Guignabert C, Savale L, Boucly A, Gallant-Dewavrin M, et al., Treatment of pulmonary arterial hypertension: recent progress and a look to the future. Lancet Respir Med. (2023) 11:804–19. doi: 10.1016/S2213-2600(23)00264-3
204. Gunawardena J. Models in biology: “accurate descriptions of our pathetic thinking”. BMC Biol. (2014) 12:1–11. doi: 10.1186/1741-7007-12-29
205. Tokarz J, Adamski J. Confounders in metabolomics. In: Adamski J, editor. Metabolomics for Biomedical Research. Cambridge, MA: Academic Press (2020). p. 17–32. doi: 10.1016/B978-0-12-812784-1.00002-5
Keywords: pulmonary hypertension, GPCR, IL6, activin, BMP, SRC, sotatercept, Lifelike
Citation: Weinstein N, Carlsen J, Schulz S, Stapleton T, Henriksen HH, Travnik E and Johansson PI (2024) A Lifelike guided journey through the pathophysiology of pulmonary hypertension—from measured metabolites to the mechanism of action of drugs. Front. Cardiovasc. Med. 11:1341145. doi: 10.3389/fcvm.2024.1341145
Received: 19 November 2023; Accepted: 12 April 2024;
Published: 23 May 2024.
Edited by:
Samuel Calvert Dudley, University of Minnesota Twin Cities, United StatesReviewed by:
Haiyang Tang, University of Arizona, United StatesPer-Arne Lönnqvist, Karolinska Institutet (KI), Sweden
© 2024 Weinstein, Carlsen, Schulz, Stapleton, Henriksen, Travnik and Johansson. This is an open-access article distributed under the terms of the Creative Commons Attribution License (CC BY). The use, distribution or reproduction in other forums is permitted, provided the original author(s) and the copyright owner(s) are credited and that the original publication in this journal is cited, in accordance with accepted academic practice. No use, distribution or reproduction is permitted which does not comply with these terms.
*Correspondence: Pär Ingemar Johansson, per.johansson@regionh.dk
Evelyn Travnik, evelyn.travnik@gmail.com