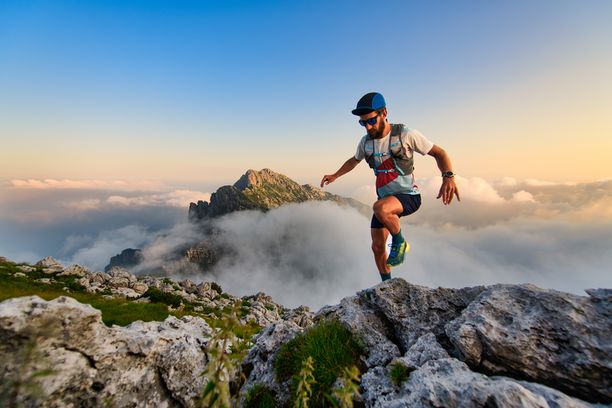
94% of researchers rate our articles as excellent or good
Learn more about the work of our research integrity team to safeguard the quality of each article we publish.
Find out more
ORIGINAL RESEARCH article
Front. Cardiovasc. Med., 05 April 2024
Sec. Coronary Artery Disease
Volume 11 - 2024 | https://doi.org/10.3389/fcvm.2024.1340022
Several regression-based models for predicting outcomes after acute myocardial infarction (AMI) have been developed. However, prediction models that encompass diverse patient-related factors over time are limited. This study aimed to develop a machine learning-based model to predict longitudinal outcomes after AMI. This study was based on a nationwide prospective registry of AMI in Korea (n = 13,104). Seventy-seven predictor candidates from prehospitalization to 1 year of follow-up were included, and six machine learning approaches were analyzed. Primary outcome was defined as 1-year all-cause death. Secondary outcomes included all-cause deaths, cardiovascular deaths, and major adverse cardiovascular event (MACE) at the 1-year and 3-year follow-ups. Random forest resulted best performance in predicting the primary outcome, exhibiting a 99.6% accuracy along with an area under the receiver-operating characteristic curve of 0.874. Top 10 predictors for the primary outcome included peak troponin-I (variable importance value = 0.048), in-hospital duration (0.047), total cholesterol (0.047), maintenance of antiplatelet at 1 year (0.045), coronary lesion classification (0.043), N-terminal pro-brain natriuretic peptide levels (0.039), body mass index (BMI) (0.037), door-to-balloon time (0.035), vascular approach (0.033), and use of glycoprotein IIb/IIIa inhibitor (0.032). Notably, BMI was identified as one of the most important predictors of major outcomes after AMI. BMI revealed distinct effects on each outcome, highlighting a U-shaped influence on 1-year and 3-year MACE and 3-year all-cause death. Diverse time-dependent variables from prehospitalization to the postdischarge period influenced the major outcomes after AMI. Understanding the complexity and dynamic associations of risk factors may facilitate clinical interventions in patients with AMI.
Cardiovascular disease is a major health burden globally, resulting in approximately 17 million deaths annually. It also contributes to 10% of the global disease burden (1, 2). Ischemic heart disease is the single most common cause of cardiovascular death and overall mortality worldwide (3). Interventional strategies for ischemic heart disease have advanced in the past three decades, and the associated clinical outcomes and mortality rates have improved. However, the prognosis of ischemic heart disease is variable, encompassing a variety of diverse risk factors (4–6). The appropriate prediction of prognosis in acute myocardial infarction (AMI) is crucial for proper clinical decision-making at an individual patient level. Appropriate prediction is important for the alleviation of expenditures and effective distribution of resources. Over the last two decades, several prognosis predicting models for AMI patients have been introduced, which reflect numerous patient-level risk factors (7, 8). However, a systematic predictive model that comprehends various risk factors across different time periods—namely prehospitalization variables, in-hospital events, procedural results, and postdischarge variables—is lacking. This is especially true in the prediction of long-term outcomes following AMI events.
Machine learning identifies the underlying patterns from large volumes of datasets and can facilitate effective predictions (9–11). Machine learning-based algorithms have led to the development of prediction models that integrate multiple patient-related variables. However, conflicting results have been reported regarding the efficacy of machine learning-based algorithms in predicting clinical outcomes after cardiovascular events (12, 13). Therefore, this study aimed to develop a machine learning-based prediction model that estimates longitudinal clinical outcomes, including mortality and major adverse cardiovascular event (MACE), after AMI. The study also investigated the performance of various machine learning-based algorithms.
This study was based on a nationwide, prospective, observational registry of AMI in South Korea (KAMIR-NIH). The KAMIR-NIH registry was established across 20 tertiary centers equipped for coronary interventions, spanning from November 2011–December 2015 (14). The KAMIR-NIH registry consists of 13,104 patients with AMI and their 3-year follow-up clinical outcomes. The protocols of the KAMIR-NIH conformed to the principles of the Declaration of Helsinki and were approved by the institutional review boards of each center (CNUH-2011-172). Written informed consent was obtained from each patient or legal representative. All data were collected through electronic case report forms in a data management system established by the Centers for Disease Control and Prevention, Ministry of Health and Welfare, Republic of Korea (iCReaT Study No. C110016; KCT-0000863). A detailed protocol and additional information about data collection for the KAMIR-NIH registry have been previously published (14, 15).
The primary outcome was defined as all-cause death at 1 year of follow-up. The secondary outcomes included five dependent variables: (1) cardiovascular death at the 1-year follow-up, (2) MACE at the 1-year follow-up, (3) all-cause death at the 3-year follow-up, (4) cardiovascular death at the 3-year follow-up, and (5) MACE at the 3-year follow-up. The 3-year outcomes were confined to any events that occurred by the 3-year follow-up without occurring at the 1-year follow-up. Death was classified as cardiovascular in origin if no other non-cardiac causes were identified. MACE was defined as a composite outcome requiring clinical intervention, including myocardial infarction, repeat percutaneous coronary intervention (PCI) of target or non-target vessel revascularization, coronary artery bypass graft, stent thrombosis, cerebrovascular accident (ischemic stroke or hemorrhagic stroke), and re-hospitalization due to heart failure aggravation. Seventy-seven independent variables were included to predict outcomes derived from various time periods: prehospitalization, in-hospitalization, during coronary intervention, and postdischarge (Supplementary Table S1). Data for predicting outcomes in the candidates comprised demographic, health, and treatment information, including procedural factors such as coronary angiography and PCI.
Six machine learning approaches (artificial neural network, decision tree, logistic regression, naïve Bayes, random forest, and support vector machine) were used to predict all-cause death, cardiovascular death, and MACE at the 1-year and 3-year follow-ups (16–19). The limited-memory Broyden–Fletcher–Goldfarb–Shanno algorithm was used for the optimization of the artificial neural network, and the number of neurons was 10 for each of its two hidden layers. The random forest employed 1,000 trees without imposing a maximum depth.
A decision tree comprises three elements: a test on an independent variable (intermediate note), an outcome of the test (branch), and the value of the dependent variable (terminal node). A naïve Bayesian classifier performs classification based on Bayes' theorem. In contrast, a random forest is a collection of many decision trees, employing majority votes on the dependent variable (bootstrap aggregation) (16). Meanwhile, a support vector machine estimates a group of support vectors, forming a line or space known as a hyperplane. This hyperplane effectively separates data by maximizing the gap between various subgroups. Lastly, an artificial neural network consists of neurons, which are information units interconnected through weights (16).
Data on 9,661 observations with complete information were randomly divided into training and validation sets using a 75:25 ratio (7,246 vs. 2,415). Accuracy, which quantifies the ratio of correct predictions among the 2,415 observations, was employed as a standard for validating the models. The area under the receiver-operating-characteristic curve (AUC) was the other performance indicator in this study. Sensitivity, specificity, and the F1 score were taken into consideration to enhance the understanding of accuracy. Random forest variable importance, defined as the contribution of a certain variable to the performance of the random forest, was used to examine the major predictors of the six dependent variables.
Further analysis of SHapley Additive exPlanations (SHAP) values was adopted to illustrate the relative attribution of independent variables to each outcome. The SHAP value of a predictor for a participant measures the difference between what the random forest predicts for the probability of the death/event with and without the predictor. These are described as SHAP summary plots. The SHAP dependence plot for a predictor registers a relationship between the value of the predictor and its SHAP value.
For rigorous analysis, the random split and examination were iterated 50 times. The ensuing average was then employed for external validation (18, 19). Python (CreateSpace: Scotts Valley, 2009) was employed for the analysis between December 15, 2021, and May 15, 2022.
A total of 13,104 patients were enrolled in the KAMIR-NIH registry, and from this cohort, 9,661 patients with data on dependent and independent variables were further selected (Figure 1). Among them, 8,806 patients with 3-year follow-up data were analyzed for the 3-year outcome prediction. For the 9,661 patients included in the 1-year outcome analysis, the mean age of onset was 62.6 ± 12.2 years and 2,297 (23.8%) were female (Table 1). ST-elevation myocardial infarction was diagnosed in 4,975 patients (51.5%). Cardiogenic shock was diagnosed in 576 (6.0%) patients, and extracorporeal membrane oxygenation was applied in 24 patients (0.2%). With respect to procedures, 9,016 patients (93.3%) underwent PCI, whereas 612 patients (6.3%) received balloon angioplasty only (patients without coronary angiography data were excluded from the analysis). Similar clinical characteristics were observed in the study population included in the 3-year outcome analysis (Supplementary Table S2). During the 1-year follow-up, 35 patients (0.4%) died, with 20 (0.2%) deaths attributed to cardiovascular causes. Moreover, MACE occurred in 314 patients (3.3%). During the 3-year follow-up, 144 patients (1.6%) died, with 79 (0.9%) classified as cardiovascular deaths, and MACE occurred in 254 patients (2.9%, Supplementary Table S2).
Figure 1. Flowchart of the study process. KAMIR-NIH, Korean Acute Myocardial Infarction-National Institutes of Health; ANN, artificial neural network; DT, decision tree; LR, logistic regression; NB, naïve Bayes; RF, random forest; SVM, support vector machine; CAG, coronary angiography; MACE, major adverse cardiovascular event; SHAP, shapley additive explanations.
For the primary outcome, the random forest algorithm showed best performance, achieving an AUC of 0.874 and an accuracy of 99.6% (Figure 2, Table 2, Supplementary Figure S1). The consistent excellence of the random forest algorithm carried over to the secondary outcomes, surpassing the performance of logistic regression.
Figure 2. Model performance of machine learning algorithms and logistic regression. (A) Model performance for 1-year follow-up data. (B) Model performance for 3-year follow-up data. AUC, area under the receiver-operating characteristic curve; LR, logistic regression; DT, decision tree; NB, naïve Bayes; RF, random forest; SVM, support vector machine; ANN, artificial neural network; MACE, major adverse cardiovascular event.
Identified predictors of 1-year all-cause death included peak troponin-I (variable importance value = 0.048), in-hospital duration (0.047), total cholesterol (0.047), maintenance of antiplatelet at 1 year (0.045), coronary lesion classification (0.043), N-terminal pro-brain natriuretic peptide levels (0.039), body mass index (BMI) (0.037), door-to-balloon time (0.035), vascular approach (0.033), and use of glycoprotein IIb/IIIa inhibitor (0.032, Figure 3A). For the 3-year follow-up, predictors of all-cause death included statin use at discharge (0.051), sex (0.043), BMI (0.040), use of glycoprotein IIb/IIIa inhibitor (0.038), in-hospital duration (0.038), NT-proBNP (0.036), coronary lesion classification (0.036), total cholesterol (0.036), door-to-balloon time (0.035), and peak troponin-I (0.034, Figure 3B). The importance ranking of BMI stayed within the top seven predictors for all outcomes, except for the prediction of 3-year MACE, where it dropped to the 14th position. Further details based on the random forest algorithm are provided in Supplementary Tables 3, 4.
Figure 3. Predictors for 1-year and 3-year all-cause death as determined by random forest analysis. (A) Top 30 predictors for 1-year all-cause death based on random forest variable importance. (B) Top 30 predictors for 3-year all-cause death based on random forest variable importance. NT-proBNP, N-terminal pro-brain natriuretic peptide; MACE, major adverse cardiovascular event; PCI, percutaneous coronary intervention; VI, variable importance.
The SHAP summary plots depicting the random forest's attributions are represented in Supplementary Figure S2. To illustrate, when examining 1-year cardiovascular death, the SHAP values for BMI range from −0.0009 (minimum) to 0.0287 (maximum), with a median value of 0.0139. In addition, including the predictor BMI in the random forest model resulted in a 1.39% increase in the probability of experiencing cardiovascular death during the 1-year follow-up, particularly for a participant at the median level. Interestingly, the impact of BMI exhibited a varied pattern across different outcomes, elucidated through the SHAP dependence plots showcased in Figure 4. For instance, in the context of 3-year MACE (Figure 4F), as the BMI value increases, its corresponding SHAP value traces a U-shaped trajectory, signifying a sequence of decline followed by elevation. This distinctive pattern is exemplified by the interplay between blue and red dots representing low and high C-reactive protein levels, respectively. Additionally, a noticeable correlation emerges between BMI and C-reactive protein levels in terms of their SHAP values for MACE at the 3-year follow-up.
Figure 4. Random forest SHAP dependence plots: body mass index. (A) All-cause death at 1 year. (B) Cardiovascular death at 1 year. (C) MACE at 1 year. (D) All-cause death at 3 years. (E) Cardiovascular death at 3 years. (F) MACE at 3 years. MACE, major adverse cardiovascular event; PCI, percutaneous coronary intervention; CABG, coronary artery bypass graft, SHAP, shapley additive explanations.
Based on a nationwide prospective registry of AMI patients, we developed a machine learning-based prediction model that estimates major long-term outcomes after AMI. The main findings from this study could be summarized as follows (Figure 5). First, the machine learning-based algorithms, especially the random forest approach, outperformed the conventional logistic regression-based algorithms in predicting the outcomes after AMI. In particular, the random forest model's prediction of the primary and secondary outcomes of cardiovascular death at 1-year resulted in outstanding performance (with AUC values of 0.874 and 0.881, respectively). Second, diverse patient-related factors from different time periods were included to predict outcomes. The factors used were (1) prehospital variables (demographic factors and comorbidities), (2) in-hospital variables (laboratory markers and clinical events during index admission), (3) procedure-related variables (coronary lesion severity and revascularization strategies), and (4) postdischarge variables (medications and patient compliance). Lastly, the identified predictors did not influence the outcomes in a uniform, linear manner, as typically seen with conventional regression-based algorithms. The results were nonlinear, exerting variable influences as indicated by the SHAP values. These values effectively captured the amalgamation of factors in the real-world scenario, reflecting the inherent outcome variability.
Figure 5. Overview of machine learning analysis of clinical outcomes after AMI. Based on a nationwide AMI registry from Korea, machine learning analysis was performed to predict clinical outcomes after AMI. The analysis encompassed a wide range of patient-related factors, spanning from prehospitalization to postdischarge variables (Left). The random forest algorithm demonstrated the best predictive performance (Right, top). The identified predictors demonstrated nonlinear, variable influences (SHAP analysis) that reflected the complex interplay of multiple factors in real-world scenarios (Right, bottom). AMI, acute myocardial infarction; KAMIR-NIH, Korean acute myocardial infarction-national institutes of health; ANN, artificial neural network; DT, decision tree; LR, logistic regression; NB, naïve Bayes; RF, random forest; SVM, support vector machine; MACE, major adverse cardiovascular event; CAG, coronary angiography; PCI, percutaneous coronary intervention; SHAP, shapley additive explanations; NT-proBNP, N-terminal pro-brain natriuretic peptide.
The predictors of the 1-year and 3-year outcomes did not reflect significant differences in their characteristics. Specifically, the prehospital, in-hospital, postdischarge, and procedural factors demonstrated close associations with both the 1-year and 3-year outcomes following AMI. Among these risk factors spanning different time periods, the in-hospital risk factors and procedure-related factors accounted for approximately two-thirds of the top 30 predictors for all outcomes. This trend was even more pronounced in the context of the 3-year outcomes. Notably, maintenance of statin therapy at discharge emerged as the most pivotal predictor of the 3-year outcome. This finding implies that after acute revascularization of coronary lesions, the maintenance of potent lipid-lowering therapies becomes a crucial therapeutic strategy that significantly impacts long-term outcomes after AMI. Furthermore, among the various predictors, BMI was identified as one of the most powerful predictors of outcomes after AMI. However, BMI did not exert a uniform influence on the outcomes. This phenomenon is further discussed in detail in the following.
Several predictive models to assess mortality after AMI have been introduced in the past two decades, and most have been based on conventional regression-based algorithms (7, 8, 20). Among these, the thrombolysis in the myocardial infarction (TIMI) risk score and global registry of acute coronary events (GRACE) risk score have emerged as robust prediction scores (7, 20). These scores were formulated during the early phase of development of PCI and are tailored to predict short-term mortality (30 days to 6 months) after AMI. They encompass simple predictors of in-hospital variables, such as age, blood pressure, and electrocardiographic findings. Both of these scores were constructed using substantial populations of AMI cases during an earlier period and subsequently validated successfully with validation cohorts. However, the predictive performances of the TIMI and GRACE risk scores are relatively low (with AUC values of 0.746 and 0.75, respectively), and their applicability may be limited due to the evolution of therapeutic strategies for coronary revascularization and medical therapy.
Recently, several prediction models employing machine learning algorithms have been developed to predict mortality in AMI patients. One machine learning-based model, utilizing data from the previous KAMIR registry (2006–2013), exhibited a fair discriminative power (AUC 0.915) in predicting 1-year mortality after AMI (8). This model incorporated both in-hospital and procedural variables as predictor candidates. In addition, it also reflected unmodifiable risk factors or initial clinical factors as important predictors of 1-year mortality (such as age, left ventricular ejection fraction, initial Killip class, BMI, initial creatinine, and low-density lipoprotein levels). Similarly, a more recent machine learning-based risk stratification model (the PRAISE score) also demonstrated remarkable performance in predicting 1-year all-cause death after acute coronary syndrome (AUC 0.82 for internal validation, 0.92 for external validation) (21). The PRAISE score encompasses eight predictors of 1-year all-cause death, including left ventricular ejection fraction, age, hemoglobin level, statin use at discharge, estimated glomerular filtration rate, use of angiotensin-converting enzyme inhibitor or angiotensin-II receptor blockers at discharge, previous bleeding, and malignancy. While the specific variables investigated may differ from those used in our study, our prediction model aligns with the PRAISE score in two key aspects. Both models incorporate (i) initial in-hospital variables that reflect hypoperfusion (left ventricular ejection fraction and creatinine and hemoglobin levels) and (ii) postdischarge medications (statin use at discharge) as important predictors of 1-year all-cause death.
We investigated the relative influence of BMI on different outcomes using SHAP dependence plots. In this study, we demonstrated that BMI was an important predictor of all outcomes, with this factor consistently ranking within the top seven predictors, except for 3-year MACE. However, the SHAP dependence plot has revealed varying effects of BMI on each outcome, contingent on its value. For example, the degree of obesity represented by BMI did not significantly impact 1-year all-cause mortality or 1-year cardiovascular mortality. However, it did result in a U-shaped influence on 1-year and 3-year MACE and all-cause mortality. Additionally, we explored the interplay between BMI and outcomes in the presence of other independent variables. For example, the absence of new-onset heart failure during index admission exhibited a strong association with BMI in the context of 1-year all-cause mortality, while a low level of C-reactive protein showed a similar association with BMI in the context of 3-year MACE.
The relative influence described by the SHAP dependence plots partly aligns with the obesity paradox observed in coronary heart disease and heart failure, However, it enhances the existing paradigm by highlighting several key points: (i) obesity assessed through BMI does not affect various cardiovascular outcomes in a uniform manner; (ii) the degree of obesity exerts varying degrees of influence on outcomes; and (iii) obesity is also associated with other independent variables (22–25). The nonlinear and dynamic impacts of BMI on different cardiovascular outcomes complement the observed obesity paradox in cardiovascular contexts (24, 26). Thus, this implies the emergence of a new paradigm that reflects the uncertainties inherent in the real world that cannot be fully explained by conventional statistics.
In this study, we investigated a validated, multi-center registry of AMI that includes more than 9,000 patients and developed machine learning-based algorithms that could predict various long-term outcomes. Large volumes of clinical variables that rise from different times were also included. We included extensive clinical variables spanning various time points and scrutinized their correlations with distinct clinical outcomes. In comparison to conventional regression-based methods, our machine learning-based prediction algorithms showcased superior discriminatory capabilities across all outcomes. To the best of our knowledge, this is the first study to investigate the dynamic interplay of individual predictors across varying degrees, influencing diverse outcomes. Moreover, we delved into the associated covariates that mirrored the intricacies, variations, and uncertainties of the real-world scenario among patients with AMI.
This study has several limitations. First, while the original registry reported a 1-year mortality of 4.3%, our cohort exhibited a considerably lower incidence of 1-year mortality (0.4%). This discrepancy could be attributed to the exclusion of variables with missing values. Notably, individuals who did not survive across various time intervals may have been associated with a higher likelihood of missing data. Consequently, this approach might have introduced a selection bias, shifting the patient cohort towards those with milder conditions. Second, the current prediction model has not been externally validated. Although the training set included more than 7,000 patients that was internally validated, validation with independent datasets may improve the generalizability of our prediction model. Third, investigating the variety of mechanisms that could exist between the six dependent variables and their major predictors was beyond the scope of this study. Limited attention has been devoted to this aspect, underscoring the need for further exploration in this direction. Fourth, regarding the rapidly evolving interventional strategy and change of the clinical guidelines, prediction model based on relatively remote data may need to be updated with recent data. Nonetheless, KAMIR-NIH registry is a validated, nationwide registry of AMI, and independent variables included in our database are risk factors that still matters in clinical field. Lastly, synthesizing diverse modes of machine learning-based methods for different types of cardiovascular data could pioneer a new approach in this field.
In AMI patients, numerous patient-related risk factors spanning the prehospitalization, in-hospitalization, and postdischarge periods exert an impact on both 1-year and 3-year outcomes. Dynamic associations among risk factors should be understood, and appropriate clinical interventions are needed after AMI. Our study suggests potential role of machine learning-based algorithm to predict adverse outcomes in patients with AMI. Further validation based on an external cohort may be needed to generalize the prediction model.
The original contributions presented in the study are included in the article/Supplementary Material, further inquiries can be directed to the corresponding authors.
The studies involving humans were approved by Chonnam national university hospital, CNUH-2011-172. The studies were conducted in accordance with the local legislation and institutional requirements. The participants provided their written informed consent to participate in this study.
JJ: Writing – original draft, Writing – review & editing, Visualization. KL: Writing – original draft, Writing – review & editing, Formal Analysis, Methodology. SP: Writing – original draft, Writing – review & editing, Conceptualization, Data curation, Investigation, Supervision. SK: Writing – review & editing, Data curation, Investigation. MK: Writing – review & editing, Data curation, Investigation. SC: Writing – review & editing, Data curation, Investigation. SH: Writing – review & editing, Data curation, Investigation. IS: Writing – review & editing, Data curation, Investigation. SO: Writing – review & editing, Data curation, Investigation. TA: Writing – review & editing, Data curation, Investigation. MJ: Writing – review & editing, Data curation, Investigation, Funding acquisition, Supervision.
The author(s) declare that financial support was received for the research, authorship, and/or publication of this article.
This work was supported by the Ministry of Health and Welfare of South Korea under Korea Health Industry Development Institute grants [No. HI21C156001; HI22C1302 (Korea Health Technology R&D Project)] and the Research of Korea Centers for Disease Control and Prevention (2013-E63005-02). The funding source did not contribute to the study's design, data collection, analysis, interpretation, or manuscript preparation.
We extend our gratitude to all the investigators involved in the KAMIR-NIH registry for their invaluable contribution in establishing the essential database of AMI patients in Korea.
The authors declare that the research was conducted in the absence of any commercial or financial relationships that could be construed as a potential conflict of interest.
All claims expressed in this article are solely those of the authors and do not necessarily represent those of their affiliated organizations, or those of the publisher, the editors and the reviewers. Any product that may be evaluated in this article, or claim that may be made by its manufacturer, is not guaranteed or endorsed by the publisher.
The Supplementary Material for this article can be found online at: https://www.frontiersin.org/articles/10.3389/fcvm.2024.1340022/full#supplementary-material
1. Mendis S, Puska P, Norrving B, World Health Organization. Global Atlas on Cardiovascular Disease Prevention and Control. Geneva: World Health Organization in collaboration with the World Heart Federation and the World Stroke Organization (2011).
2. Visseren FLJ, Mach F, Smulders YM, Carballo D, Koskinas KC, Bäck M, et al. 2021 ESC guidelines on cardiovascular disease prevention in clinical practice. Eur Heart J. (2021) 42:3227–337. doi: 10.1093/eurheartj/ehab484
3. Ibanez B, James S, Agewall S, Antunes MJ, Bucciarelli-Ducci C, Bueno H, et al. 2017 ESC guidelines for the management of acute myocardial infarction in patients presenting with ST-segment elevation: the task force for the management of acute myocardial infarction in patients presenting with ST-segment elevation of the European Society of Cardiology (ESC). Eur Heart J. (2018) 39:119–77. doi: 10.1093/eurheartj/ehx393
4. Kim S, Cho DH, Kim MN, Hong SJ, Yu CW, Lim DS, et al. Transmural difference in myocardial damage assessed by layer-specific strain analysis in patients with ST elevation myocardial infarction. Sci Rep. (2020) 10:11104. doi: 10.1038/s41598-020-68043-w
5. Kang DO, Park Y, Seo JH, Jeong MH, Chae SC, Ahn TH, et al. Time-dependent prognostic effect of high sensitivity C-reactive protein with statin therapy in acute myocardial infarction. J Cardiol. (2019) 74:74–83. doi: 10.1016/j.jjcc.2018.12.022
6. Cha JJ, Bae S, Park DW, Park JH, Hong SJ, Park SM, et al. Clinical outcomes in patients with delayed hospitalization for non-ST-segment elevation myocardial infarction. J Am Coll Cardiol. (2022) 79:311–23. doi: 10.1016/j.jacc.2021.11.019
7. Morrow DA, Antman EM, Charlesworth A, Cairns R, Murphy SA, de Lemos JA, et al. TIMI risk score for ST-elevation myocardial infarction: a convenient, bedside, clinical score for risk assessment at presentation: an intravenous nPA for treatment of infarcting myocardium early II trial substudy. Circulation. (2000) 102:2031–7. doi: 10.1161/01.cir.102.17.2031
8. Lee HC, Park JS, Choe JC, Ahn JH, Lee HW, Oh JH, et al. Prediction of 1-year mortality from acute myocardial infarction using machine learning. Am J Cardiol. (2020) 133:23–31. doi: 10.1016/j.amjcard.2020.07.048
9. Deo RC. Machine learning in medicine. Circulation. (2015) 132:1920–30. doi: 10.1161/CIRCULATIONAHA.115.001593
10. Shouval R, Bondi O, Mishan H, Shimoni A, Unger R, Nagler A. Application of machine learning algorithms for clinical predictive modeling: a data-mining approach in SCT. Bone Marrow Transplant. (2014) 49:332–7. doi: 10.1038/bmt.2013.146
11. Kim TW, Ahn J, Ryu JA. Machine learning-based predictor for neurologic outcomes in patients undergoing extracorporeal cardiopulmonary resuscitation. Front Cardiovasc Med. (2023) 10:1278374. doi: 10.3389/fcvm.2023.1278374
12. Gupta S, Ko DT, Azizi P, Bouadjenek MR, Koh M, Chong A, et al. Evaluation of machine learning algorithms for predicting readmission after acute myocardial infarction using routinely collected clinical data. Can J Cardiol. (2020) 36:878–85. doi: 10.1016/j.cjca.2019.10.023
13. Kasim S, Malek S, Ibrahim KS, Amir PNF, Aziz MF. Investigating performance of deep learning and machine learning risk stratification of Asian in-hospital patients after ST-elevation myocardial infarction. Eur Heart J Digit Health. (2021) 42:3068. doi: 10.1093/ehjdh/ztab104.3068
14. Kim JH, Chae SC, Oh DJ, Kim HS, Kim YJ, Ahn Y, et al. Multicenter cohort study of acute myocardial infarction in Korea: interim analysis of the Korea acute myocardial infarction registry-national institutes of health registry. Circ J. (2016) 80:1427–36. doi: 10.1253/circj.CJ-16-0061
15. Park CS, Yang HM, Kang J, Han JK, Park KW, Kang HJ, et al. Long-term use of renin-angiotensin-system inhibitors after acute myocardial infarction is not associated with survival benefits: analysis of data from the Korean acute myocardial infarction registry-national institutes of health registry. Front Cardiovasc Med. (2022) 9:994419. doi: 10.3389/fcvm.2022.994419
16. Lee KS, Park H. Machine learning on thyroid disease: a review. Front Biosci (Landmark Ed). (2022) 27:101. doi: 10.31083/j.fbl2703101
17. Lee KS, Kim ES, Song IS, Kim HI, Ahn KH. Association of preterm birth with inflammatory bowel disease and salivary gland disease: machine learning analysis using national health insurance data. Int J Environ Res Public Health. (2022) 19:3056. doi: 10.3390/ijerph19053056
18. Park HS, Lee KS, Seo BK, Kim ES, Cho KR, Woo OH, et al. Machine learning models that integrate tumor texture and perfusion characteristics using low-dose breast computed tomography are promising for predicting histological biomarkers and treatment failure in breast cancer patients. Cancers (Basel). (2021) 13:2013. doi: 10.3390/cancers13236013
19. Lee JY, Lee KS, Seo BK, Cho KR, Woo OH, Song SE, et al. Radiomic machine learning for predicting prognostic biomarkers and molecular subtypes of breast cancer using tumor heterogeneity and angiogenesis properties on MRI. Eur Radiol. (2022) 32:650–60. doi: 10.1007/s00330-021-08146-8
20. Eagle KA, Lim MJ, Dabbous OH, Pieper KS, Goldberg RJ, Van de Werf F, et al. A validated prediction model for all forms of acute coronary syndrome - estimating the risk of 6-month postdischarge death in an international registry. JAMA. (2004) 291:2727–33. doi: 10.1001/jama.291.22.2727
21. D'Ascenzo F, De Filippo O, Gallone G, Mittone G, Deriu MA, Iannaccone M, et al. Machine learning-based prediction of adverse events following an acute coronary syndrome (PRAISE): a modelling study of pooled datasets. Lancet. (2021) 397:199–207. doi: 10.1016/S0140-6736(20)32519-8
22. Uretsky S, Messerli FH, Bangalore S, Champion A, Cooper-Dehoff RM, Zhou Q, et al. Obesity paradox in patients with hypertension and coronary artery disease. Am J Med. (2007) 120:863–70. doi: 10.1016/j.amjmed.2007.05.011
23. Zeller M, Steg PG, Ravisy J, Lorgis L, Laurent Y, Sicard P, et al. Relation between body mass index, waist circumference, and death after acute myocardial infarction. Circulation. (2008) 118:482–90. doi: 10.1161/circulationaha.107.753483
24. Lavie CJ, McAuley PA, Church TS, Milani RV, Blair SN. Obesity and cardiovascular diseases: implications regarding fitness, fatness, and severity in the obesity paradox. J Am Coll Cardiol. (2014) 63:1345–54. doi: 10.1016/j.jacc.2014.01.022
25. Hwang IC, Choi HM, Yoon YE, Park JJ, Park JB, Park JH, et al. Body mass index, muscle mass, and all-cause mortality in patients with acute heart failure: the obesity paradox revisited. Int J Heart Fail. (2022) 4:95–109. doi: 10.36628/ijhf.2022.0007
Keywords: body mass index, machine learning analysis, myocardial infarction, artificial intelligence, prediction model
Citation: Jeong JH, Lee K-S, Park S-M, Kim SR, Kim M-N, Chae SC, Hur S-H, Seong IW, Oh SK, Ahn TH and Jeong MH (2024) Prediction of longitudinal clinical outcomes after acute myocardial infarction using a dynamic machine learning algorithm. Front. Cardiovasc. Med. 11:1340022. doi: 10.3389/fcvm.2024.1340022
Received: 17 November 2023; Accepted: 27 March 2024;
Published: 5 April 2024.
Edited by:
Yohei Sotomi, Osaka University, JapanReviewed by:
Devesh Rai, Rochester General Hospital, United States© 2024 Jeong, Lee, Park, Kim, Kim, Chae, Hur, Seong, Oh, Ahn and Jeong. This is an open-access article distributed under the terms of the Creative Commons Attribution License (CC BY). The use, distribution or reproduction in other forums is permitted, provided the original author(s) and the copyright owner(s) are credited and that the original publication in this journal is cited, in accordance with accepted academic practice. No use, distribution or reproduction is permitted which does not comply with these terms.
*Correspondence: Seong-Mi Park c21wYXJrbWRAa29yZWEuYWMua3I= Myung Ho Jeong bXl1bmdob0BjaG9sbGlhbi5uZXQ=
†These authors have contributed equally to this work
‡ORCID Seong-Mi Park orcid.org/0000-0002-6710-685X Myung Ho Jeong orcid.org/0000-0003-2424-810X
Disclaimer: All claims expressed in this article are solely those of the authors and do not necessarily represent those of their affiliated organizations, or those of the publisher, the editors and the reviewers. Any product that may be evaluated in this article or claim that may be made by its manufacturer is not guaranteed or endorsed by the publisher.
Research integrity at Frontiers
Learn more about the work of our research integrity team to safeguard the quality of each article we publish.