- Department of Cardiology, Dongfang Hospital, Beijing University of Chinese Medicine, Beijing, China
Background: Observational studies have indicated a potential association between education and cardiovascular diseases (CVDs). However, uncertainties regarding the causal relationship persist. Therefore, this study aimed to investigate whether higher levels of education causally reduce the risks of CVDs.
Methods: Employing a two-sample Mendelian randomization (MR) design, our study examined the relationship between education and ten different CVDs. Utilizing data from the IEU Open GWAS database, relevant single nucleotide polymorphisms (SNPs) were identified through stringent screening criteria. Causality was assessed using the inverse-variance weighted (IVW), ME-Egger regression, and weighted median methods. Sensitivity analyses, including heterogeneity and pleiotropy tests, were conducted to ensure the robustness of our findings.
Results: Our study identified a genetic predisposition associated with an additional 3.6 years of education, which significantly reduced the risk of various CVDs. Specifically, this genetic factor was found to lower the risk of type 2 diabetes by 46.5%, coronary heart disease by 37.5%, ischemic stroke by 35.4%, cardiac-related mortality by 28.6%, heart failure by 28.2%, transient ischemic attack by 24%, atrial fibrillation by 15.2%, peripheral artery disease by 0.3%, and hypertension by 0.3%. However, no significant evidence revealed a causal relationship between education and pulmonary embolism.
Conclusion: Our study provides robust evidence supporting the role of higher educational attainment in reducing the incidence of various cardiovascular diseases, including type 2 diabetes, coronary heart disease, ischemic stroke, cardiac-related mortality, heart failure, transient ischemic attack, atrial fibrillation, peripheral artery disease, and hypertension. However, the impact of education on pulmonary embolism remains inconclusive.
1 Introduction
Cardiovascular diseases (CVDs) encompass a wide range of conditions that have a significant impact on quality of life and contribute to global mortality (1, 2). Ischemic heart disease and stroke are major causes of disability among individuals aged 50 and above (3). The prevalence of CVDs has been steadily increasing worldwide for several decades (4). Various factors, including cardiometabolic, environmental, and social risks, contribute to the development of CVDs. While causal relationships have been established for certain risk factors like hypertension and diabetes, the impact of other factors, such as education, on the causality of CVDs remains uncertain. Further research is warranted to comprehensively understand these complex interactions.
Those with higher levels of education tend to exhibit better health, experience fewer illnesses, and have longer lifespans than those with lower levels of education (5, 6). Research has demonstrated the significant impact of socioeconomic risk factors, including education, on the development of CVDs (7–10). People with lower levels of education generally have worse cardiovascular health, more comorbidities, and a higher overall risk for developing cardiovascular disease (8). However, it is important to note that while traditional observational studies have established an association between education and CVDs, they do not prove causality due to various limitations. To gain deeper insights into the causes of CVDs and develop effective preventive measures, it is crucial to investigate the potential causal relationship between education and CVDs. Although randomized controlled trials (RCTs) would be the ideal approach, practical challenges such as the long latency period between exposure and outcomes, as well as ethical concerns related to limiting children's education, render such trials unfeasible. Therefore, alternative research methods should be implemented to effectively evaluate causality. Improving our understanding in this area will aid in developing targeted interventions and strategies to alleviate the burden of CVDs across different educational backgrounds.
Mendelian randomization (MR) research utilizes single nucleotide polymorphisms (SNPs) as instrumental variables (IVs) to establish models and infer causal effects (11). By randomly assigning exposed IVs during conception, unobserved confounders can be eliminated, allowing for the assessment of causal relationships between exposure and disease (12). Recent advances in genome-wide association studies (GWAS) have yielded abundant data on genetic variants and their associations with phenotypes. Of which the IEU Open GWAS database is a manually curated collection of complete GWAS summary datasets. It is developed at the MRC Integrative Epidemiology Unit (IEU) at the University of Bristol and made available as open source files for download or by querying a database of the complete data. Leveraging this data, MR studies enable the evaluation of the impact of risk factors on outcomes without requiring additional patient recruitment. MR studies have been widely employed in various diseases for causal inference. In this particular study, two-sample MR analyses were conducted to investigate the potential causal association between education and ten cardiovascular diseases. The objective of this research is to provide valuable insights that can contribute to the prevention and treatment of CVDs.
2 Methods
2.1 Study design
In our study, we conducted multiple MR analyses to investigate the relationship between education and CVDs (11). We comprehensively analyzed various types of CVDs, including coronary heart disease, hypertension, atrial fibrillation, cardiac death, pulmonary embolism, transient ischemic attack, peripheral artery disease, heart failure, type 2 diabetes, and ischemic stroke. By utilizing data from GWAS, we obtained valuable insights into the genetic basis of these diseases. We employed the two-sample MR method to explore potential causal effects between education and the development of CVDs (Figure 1). It is important to note that MR studies rely on three fundamental assumptions: establishing a strong association between genetic variation and the exposure variable, ensuring no association between the genetic variation and potential confounding factors, and confirming no association between genetic variation (apart from its effect on the exposure variable) and the outcome of interest (13). Furthermore, our study exclusively utilized publicly available summary data, which precluded the necessity for ethical approval.
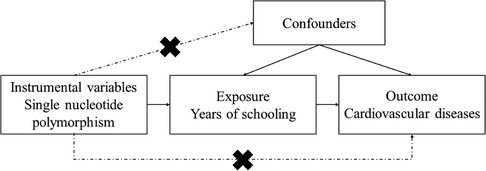
Figure 1. Description of the study design: three core hypotheses of the Mendelian randomization study.
2.2 Data sources
The analysis conducted in our study utilized summary-level data obtained from GWAS, which were predominantly conducted on individuals of European ancestry. To explore the genetic variants associated with education, we relied upon a large-scale genetic association analysis published in Nature Genetics in 2018. This analysis included approximately 1.1 million individuals and analyzed a vast dataset of 10,101,242 SNPs, reported educational attainment in people of European descent (14). We defined educational attainment in the same manner as that in this study in which educational attainment was defined by whether the participant attained a given level of schooling based on the International Standard Classification of Education 1997 classification scale. The results provided an estimate of relative risk caused by each standard deviation, which is 3.6 years, increase in the years of schooling. For coronary heart disease, our study included 60,801 cases and 123,504 controls of European ancestry, with 9,455,779 SNPs analyzed. In the investigation of hypertension, we analyzed 1,237 cases and 359,957 controls of European ancestry, examining 9,646,741 SNPs. More information about the studies on other cardiovascular diseases can be found in Table 1. Notably, all the analysis data were aggregated and accessible through the IEU Open GWAS database (https://gwas.mrcieu.ac.uk/).
2.3 Selection and validation of SNPs
In the selection process of suitable SNPs, we employed three key criteria. Firstly, SNPs were chosen based on the significant threshold of genome-wide association (P < 5 × 10−8) (15). Secondly, we conducted pairwise-linkage disequilibrium analysis to evaluate the independence among selected SNPs. SNPs with a correlation coefficient (r2) greater than 0.001, within a clumping window of 10,000 kb, were removed if they exhibited a higher P-value or were correlated with a larger number of SNPs (16, 17). Lastly, we validated the strength of individual SNPs using the F-statistic. SNPs with an F-statistic exceeding ten were considered powerful enough to mitigate potential bias. Prior to conducting the Mendelian Randomization (MR) analysis, we implemented data-harmonization steps to ensure that the effects of an SNP on exposure and outcome corresponded to the same allele.
2.4 MR analysis
In the analyzed study, we conducted an inverse-variance weighted (IVW) meta-analysis as the primary analysis method, employing a random-effects model (18). Additionally, we performed two sensitivity analyses using different methods. The first sensitivity analysis involved the weighted median method, which provides valid estimates if at least 50% of the information came from valid instrumental variables (19, 20). The second sensitivity analysis employed the MR-Egger method to evaluate the presence of horizontal pleiotropy in the selected instrumental variables (21, 22). To assess heterogeneity among the instrumental variables, we used Cochran's Q-value. Furthermore, we performed a leave-one-out sensitivity analysis to examine the potential impact of individual single nucleotide polymorphisms. The analytical procedures were carried out using R (version 4.3.1) and R Studio (version 2022.06.1 + 524). We considered statistical significance to be defined as P < 0.05.
3 Results
The included studies, published between 2015 and 2021, primarily focused on the European population. We identified 317 IVs that reached genome-wide significance levels, with all of them having F-statistics greater than ten.
The IVW analysis demonstrated a significant inverse association between higher educational attainment and nine cardiovascular diseases (Figure 2). These diseases, ranked by decreasing magnitude of association, included type 2 diabetes (odds ratio (OR) = 0.535; 95% confidence interval (CI), 0.473–0.605; P < 0.001), coronary heart disease (OR = 0.625; 95% CI, 0.563–0.692; P < 0.001), ischemic stroke (OR = 0.646; 95% CI, 0.585–0.713; P < 0.001), death due to cardiac causes (OR = 0.714; 95% CI, 0.596–0.856; P < 0.001), heart failure (OR = 0.718; 95% CI, 0.653–0.789; P < 0.001), transient ischemic attack (OR = 0.760; 95% CI, 0.645–0.895; P = 0.001), atrial fibrillation (OR = 0.848; 95% CI, 0.775–0.928; P < 0.001), peripheral artery disease (OR = 0.997; 95% CI, 0.995–0.998; P < 0.001), and hypertension (OR = 0.997; 95% CI, 0.995–0.998; P < 0.001). However, there was no evidence to suggest a potential causal relationship between education and pulmonary embolism (OR = 0.990; 95% CI, 0.789–1.244; P = 0.935). Most cardiovascular diseases showed consistent estimates but with low precision in the weighted-median and MR-Egger analyses (Table 2). No directional pleiotropy was detected, although heterogeneity was observed to be higher in certain CVDs. Therefore, an IVW meta-analysis under a random-effects model was employed to mitigate the influence of heterogeneity.
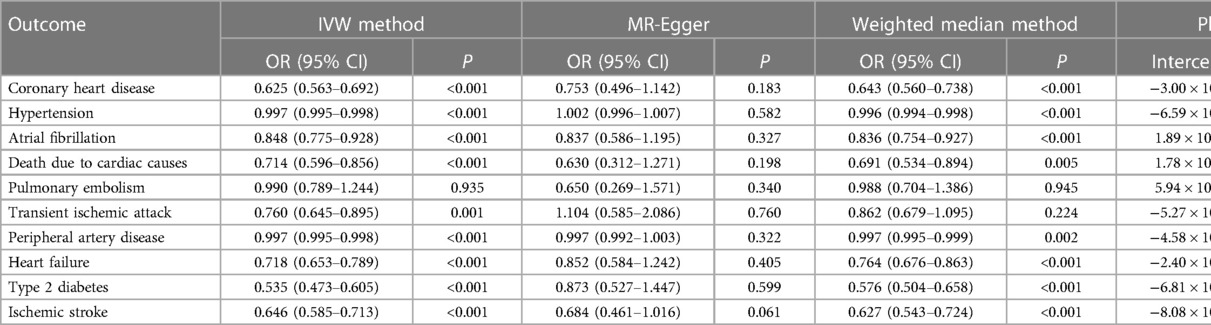
Table 2. Mendelian randomization estimates of the associations between education attainment and cardiovascular diseases.
Scatterplots depicting the relationship between education and CVDs can be found in Figure 3, while the forest plots displaying the association are provided in Figure 4. The leave-one-out sensitivity analyses, presented in Figure 5, indicate that the overall estimates were not significantly affected by any individual SNP. Additionally, the funnel plot in Figure 6 demonstrates no signs of horizontal pleiotropy.
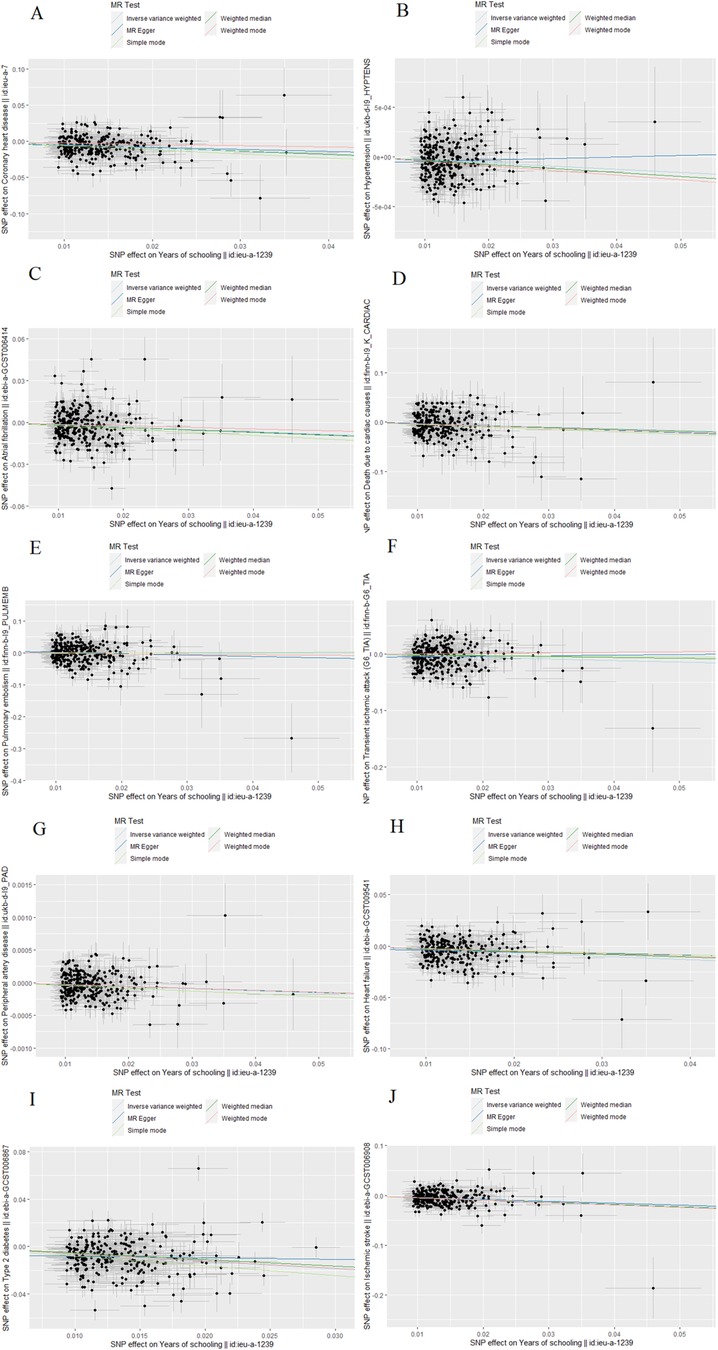
Figure 3. The scatter plot of Mendelian randomization analyses. (A) Coronary heart disease; (B) Hypertension; (C) Atrial fibrillation; (D) Death due to cardiac causes; (E) Pulmonary embolism; (F) Transient ischemic attack; (G) Peripheral artery disease; (H) Heart failure; (I) Type 2 diabetes; (J) Ischemic stroke.
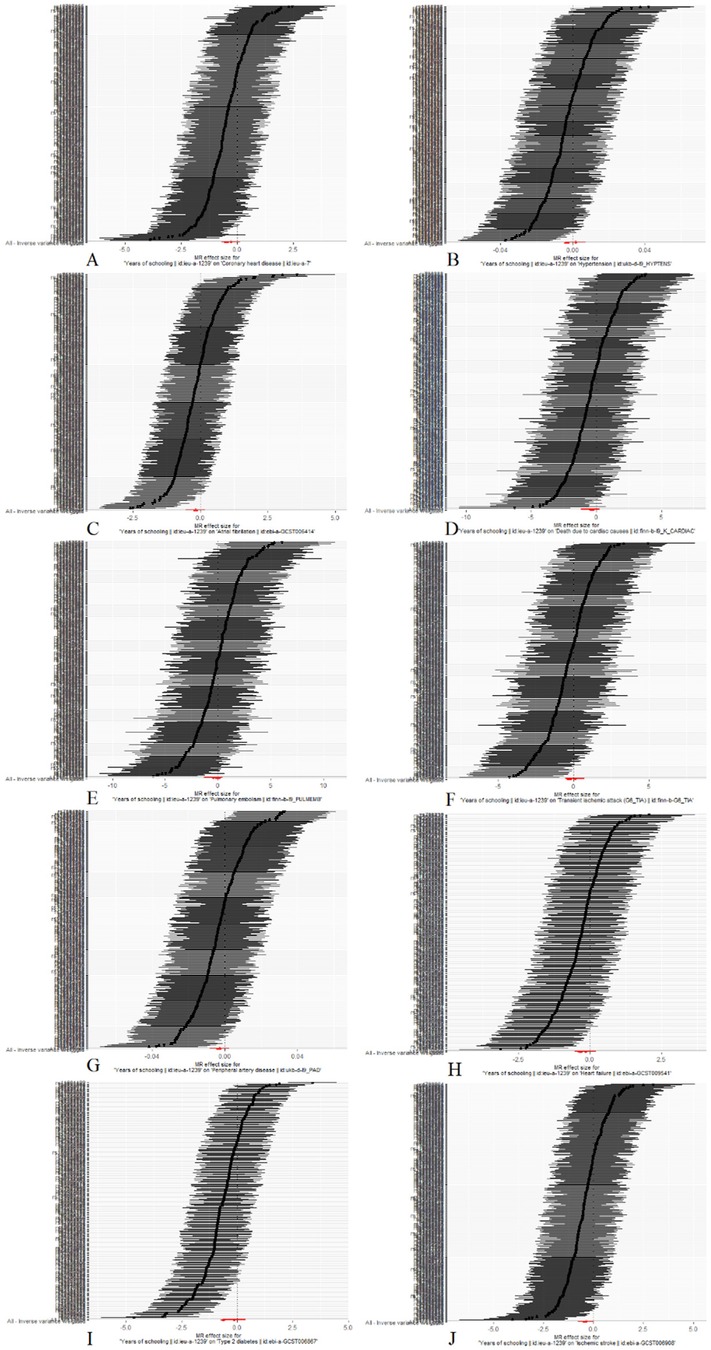
Figure 4. The forest plot of Mendelian randomization analyses. (A) Coronary heart disease; (B) Hypertension; (C) Atrial fibrillation; (D) Death due to cardiac causes; (E) Pulmonary embolism; (F) Transient ischemic attack; (G) Peripheral artery disease; (H) Heart failure; (I) Type 2 diabetes; (J) Ischemic stroke.
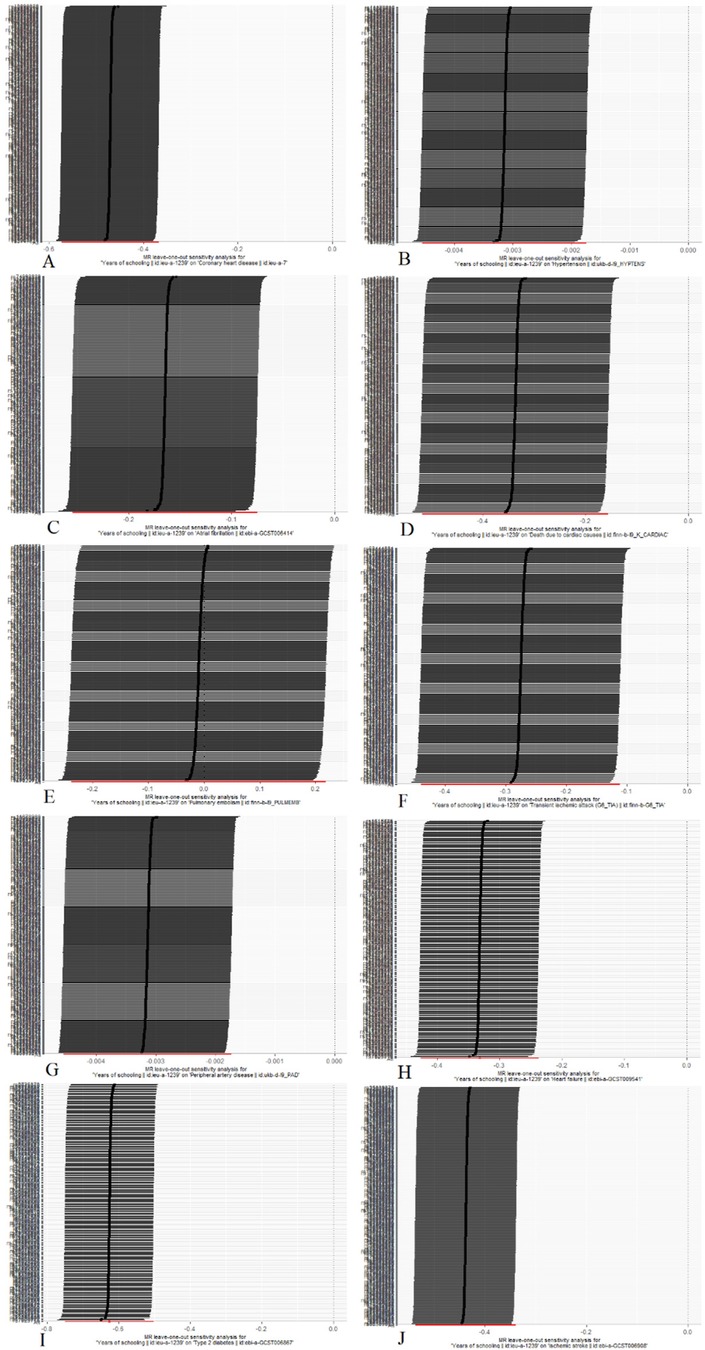
Figure 5. Mendelian randomization leave-one-out analyses. (A) Coronary heart disease; (B) Hypertension; (C) Atrial fibrillation; (D) Death due to cardiac causes; (E) Pulmonary embolism; (F) Transient ischemic attack; (G) Peripheral artery disease; (H) Heart failure; (I) Type 2 diabetes; (J) Ischemic stroke.
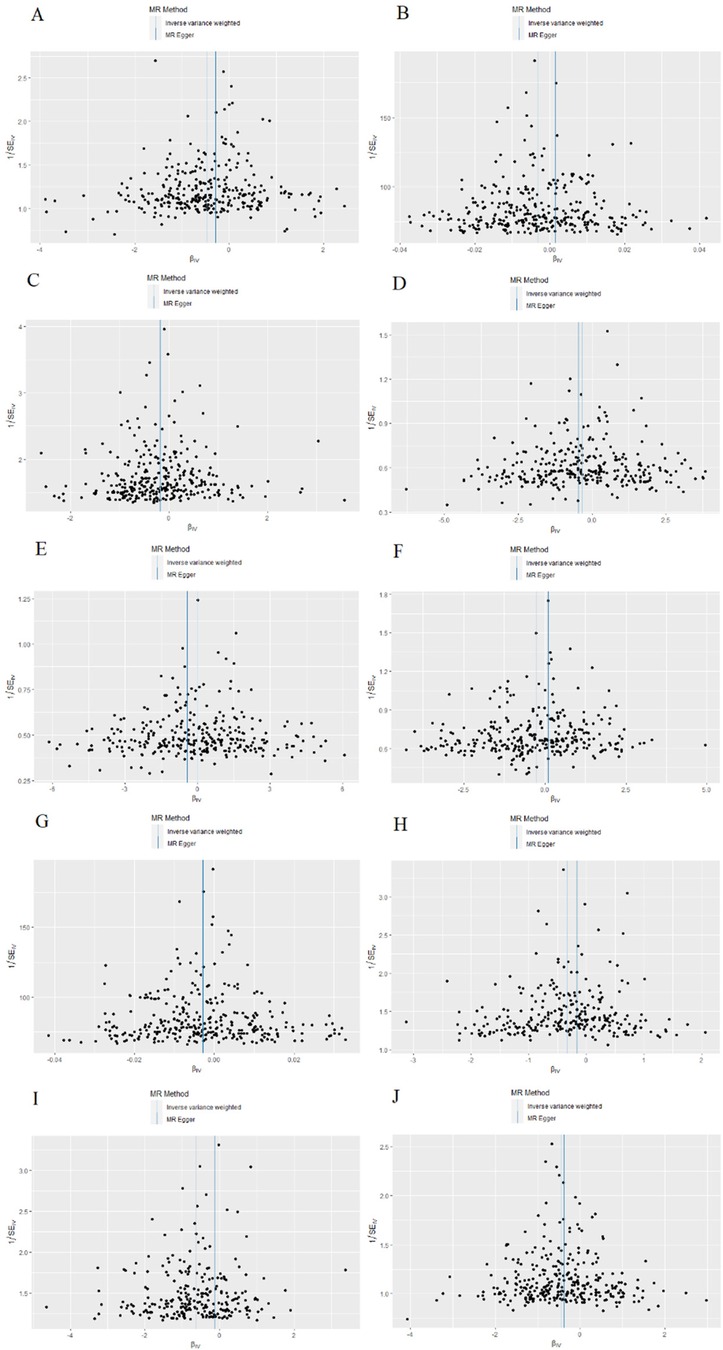
Figure 6. The funnel plot of Mendelian randomization analyses. (A) Coronary heart disease; (B) Hypertension; (C) Atrial fibrillation; (D) Death due to cardiac causes; (E) Pulmonary embolism; (F) Transient ischemic attack; (G) Peripheral artery disease; (H) Heart failure; (I) Type 2 diabetes; (J) Ischemic stroke.
4 Discussion
In our comprehensive two-sample MR analysis, we thoroughly examined the causal relationship between education and CVDs. Our findings demonstrated a significant association between higher educational attainment and a decreased likelihood of developing type 2 diabetes, coronary heart disease, ischemic stroke, cardiac-related mortality, heart failure, transient ischemic attack, atrial fibrillation, peripheral artery disease, and hypertension. Specifically, each additional 3.6 years of education resulted in a risk reduction of 46.5%, 37.5%, 35.4%, 28.6%, 28.2%, 24%, 15.2%, 0.3%, and 0.3% for the aforementioned CVDs, respectively. While education did impact peripheral artery disease and hypertension, the effect sizes were minimal. However, no statistically significant effect of education on pulmonary embolism was observed.
Education plays a pivotal role in determining health outcomes, particularly in the realm of cardiovascular diseases. A study encompassing 1.2 million individuals in Sweden from 1943 to 2007 discovered that compulsory education was associated with reduced mortality rates (6). Similarly, an investigation utilizing the UK Biobank dataset revealed that prolonged schooling mitigated the risk of diabetes and mortality (7). Moreover, consistent evidence suggests that higher educational attainment is correlated with a decreased lifetime risk of cardiovascular diseases (23). Regarding specific cardiovascular conditions, research demonstrated an inverse relationship between educational attainment and the likelihood of heart failure in patients with coronary heart disease (24). This highlights the potential benefit of implementing preventive measures for individuals with lower education levels to address survival disparities. Furthermore, a separate analysis uncovered an independent association between educational attainment and overall cardiovascular risk, underscoring the significance of education in maintaining cardiovascular health (8). Importantly, education emerged as the most reliable predictor of overall cardiovascular risk among hypertensive patients (9). Recent advancements in genetic research have provided fresh insights into the intricate interplay between education and cardiovascular diseases. Mendelian randomization studies identified robust causal links between genetic variations related to education and specific cardiovascular conditions, such as coronary heart disease and heart failure (25, 26). However, to the best of our knowledge, our study represents the first comprehensive exploration of education in the context of CVDs employing MR methods.
Education exerts a significant influence on the development of CVDs, with various intermediate phenotypes contributing to this association. Previous studies have emphasized the predictive nature of education in terms of income, occupation, and health-related behaviors (27). Individuals with higher education levels tend to adopt healthier lifestyles compared to those with lower education levels (28). For instance, lower educational attainment has been causally linked to an increased risk of smoking (29), which subsequently contributes to unfavorable serum lipid levels, central obesity, higher resting heart rate, and poor fat distribution (30). Additionally, higher education levels have been associated with lower body mass index (BMI), suggesting a potential protective effect against obesity (31). The well-established relationship between BMI and CVD risk highlights the elevated risks of coronary heart disease, hypertension, and type 2 diabetes associated with increased BMI (32). Furthermore, studies have demonstrated that individuals with lower education levels have a significantly higher risk of coronary heart disease compared to those with higher education, with smoking and obesity identified as influential risk factors contributing to this disparity (33). Moreover, evidence suggests that the negative association between educational attainment and CVD incidence is mediated by factors such as diabetes, hypertension, and BMI (34). A recent Mendelian randomization study confirmed that the impact of education on CVD risk primarily operates through modifiable factors like BMI, systolic blood pressure, or smoking (35). It is crucial to consider potential confounding factors associated with education, including diverse socio-economic statuses and the cost of education. Higher socio-economic status generally correlates with better academic performance, healthier lifestyles, and improved access to healthcare, thereby influencing overall cardiovascular risk (36).
This study demonstrates several notable strengths. Firstly, the MR analysis conducted in this study yields compelling evidence supporting a causal link between education and CVDs. Secondly, the MR analysis effectively accounts for potential confounding factors and reverse causality, thereby providing accurate estimates of the causal effects. However, it is essential to acknowledge and address the limitations inherent in this study. The GWAS data employed primarily represent individuals of European descent, necessitating the validation of these findings within other ethnic groups to ensure their applicability outside of European populations. Furthermore, researchers must exercise caution when interpreting our study's outcomes. Education is influenced by a combination of social, environmental, and genetic factors, with genetic effects accounting for only approximately 20% of the variance. Consequently, genetic effects should not be construed as independent of environmental factors (37). Why education has such a small effect on peripheral arterial disease and hypertension, and why it has no effect on pulmonary embolism, still needs to be studied and explained in relation to a variety of other factors.
5 Conclusion
Our MR study yields compelling findings suggesting a causal relationship between higher educational attainment and a decreased risk of various CVDs, such as type 2 diabetes, coronary heart disease, ischemic stroke, cardiac-related mortality, heart failure, transient ischemic attack, atrial fibrillation, peripheral artery disease, and hypertension. However, the available evidence regarding the influence of education on pulmonary embolism remains limited. Consequently, it is imperative to acknowledge the significant impact of education in preventing CVDs, emphasizing the inclusion of education in public health initiatives and government policy-making, especially in educationally disadvantaged areas.
Data availability statement
The original contributions presented in the study are included in the article/Supplementary Material, further inquiries can be directed to the corresponding author.
Author contributions
WL: Conceptualization, Formal Analysis, Project administration, Writing – original draft, Writing – review & editing. QL: Formal Analysis, Writing – original draft, Writing – review & editing. ZF: Project administration, Writing – review & editing. JC: Project administration, Writing – review & editing. YW: Conceptualization, Writing – review & editing.
Funding
The authors declare financial support was received for the research, authorship, and/or publication of this article.
The work was supported by grants from the National Natural Science Foundation of China (grant no. 81573900).
Conflict of interest
The authors declare that the research was conducted in the absence of any commercial or financial relationships that could be construed as a potential conflict of interest.
The reviewer XL declared a shared affiliation with the authors to the handling editor at the time of review.
Publisher's note
All claims expressed in this article are solely those of the authors and do not necessarily represent those of their affiliated organizations, or those of the publisher, the editors and the reviewers. Any product that may be evaluated in this article, or claim that may be made by its manufacturer, is not guaranteed or endorsed by the publisher.
References
1. GBD 2017 Causes of Death Collaborators. Global, regional, and national age-sex-specific mortality for 282 causes of death in 195 countries and territories, 1980–2017: a systematic analysis for the global burden of disease study 2017. Lancet. (2018) 392:1736–88. doi: 10.1016/S0140-6736(18)32203-7
2. GBD 2017 DALYs and HALE Collaborators. Global, regional, and national disability-adjusted life-years (DALYs) for 359 diseases and injuries and healthy life expectancy (HALE) for 195 countries and territories, 1990–2017: a systematic analysis for the global burden of disease study 2017. Lancet. (2018) 392:1859–922. doi: 10.1016/S0140-6736(18)32335-3
3. GBD 2019 Diseases and Injuries Collaborators. Global burden of 369 diseases and injuries in 204 countries and territories, 1990–2019: a systematic analysis for the global burden of disease study 2019. Lancet. (2020) 396:1204–22. doi: 10.1016/S0140-6736(20)30925-9
4. Roth GA, Mensah GA, Johnson CO, Addolorato G, Ammirati E, Baddour LM, et al. Global burden of cardiovascular diseases and risk factors, 1990–2019: update from the GBD 2019 study. J Am Coll Cardiol. (2020) 76:2982–3021. doi: 10.1016/j.jacc.2020.11.010
5. Clark D, Royer H. The effect of education on adult mortality and health: evidence from Britain. Am Econ Rev. (2013) 103:2087–120. doi: 10.1257/aer.103.6.2087
6. Lager AC, Torssander J. Causal effect of education on mortality in a quasi-experiment on 1.2 million Swedes. Proc Natl Acad Sci U S A. (2012) 109:8461–6. doi: 10.1073/pnas.1105839109
7. Davies NM, Dickson M, Davey Smith G, van den Berg GJ, Windmeijer F. The causal effects of education on health outcomes in the UK biobank. Nat Hum Behav. (2018) 2:117–25. doi: 10.1038/s41562-017-0279-y
8. Di Chiara T, Scaglione A, Corrao S, Argano C, Pinto A, Scaglione R. Association between low education and higher global cardiovascular risk. J Clin Hypertens. (2015) 17:332–7. doi: 10.1111/jch.12506
9. Di Chiara T, Scaglione A, Corrao S, Argano C, Pinto A, Scaglione R. Education and hypertension: impact on global cardiovascular risk. Acta Cardiol. (2017) 72:507–13. doi: 10.1080/00015385.2017.1297626
10. Veronesi G, Ferrario MM, Kuulasmaa K, Bobak M, Chambless LE, Salomaa V, et al. Educational class inequalities in the incidence of coronary heart disease in Europe. Heart. (2016) 102:958–65. doi: 10.1136/heartjnl-2015-308909
11. Skrivankova VW, Richmond RC, Woolf B, Yarmolinsky J, Davies NM, Swanson SA, et al. Strengthening the reporting of observational studies in epidemiology using Mendelian randomization: the STROBE-MR statement. JAMA. (2021) 326:1614–21. doi: 10.1001/jama.2021.18236
12. Burgess S, Davey Smith G, Davies NM, Dudbridge F, Gill D, Glymour MM, et al. Guidelines for performing Mendelian randomization investigations: update for summer 2023. Wellcome Open Res. (2019) 4:186. doi: 10.12688/wellcomeopenres.15555.3
13. Emdin CA, Khera AV, Kathiresan S. Mendelian randomization. JAMA. (2017) 318:1925–6. doi: 10.1001/jama.2017.17219
14. Lee JJ, Wedow R, Okbay A, Kong E, Maghzian O, Zacher M, et al. Gene discovery and polygenic prediction from a genome-wide association study of educational attainment in 1.1 million individuals. Nat Genet. (2018) 50:1112–21. doi: 10.1038/s41588-018-0147-3
15. Machiela MJ, Chanock SJ. LDlink: a web-based application for exploring population-specific haplotype structure and linking correlated alleles of possible functional variants. Bioinformatics. (2015) 31:3555–7. doi: 10.1093/bioinformatics/btv402
16. Burgess S, Davies NM, Thompson SG. Bias due to participant overlap in two-sample Mendelian randomization. Genet Epidemiol. (2016) 40:597–608. doi: 10.1002/gepi.21998
17. Burgess S, Thompson SG. Avoiding bias from weak instruments in Mendelian randomization studies. Int J Epidemiol. (2011) 40:755–64. doi: 10.1093/ije/dyr036
18. Zhu Z, Zhang F, Hu H, Bakshi A, Robinson MR, Powell JE, et al. Integration of summary data from GWAS and eQTL studies predicts complex trait gene targets. Nat Genet. (2016) 48:481–7. doi: 10.1038/ng.3538
19. Burgess S, Bowden J, Fall T, Ingelsson E, Thompson SG. Sensitivity analyses for robust causal inference from Mendelian randomization analyses with multiple genetic variants. Epidemiology. (2017) 28:30–42. doi: 10.1097/EDE.0000000000000559
20. Bowden J, Davey Smith G, Haycock PC, Burgess S. Consistent estimation in Mendelian randomization with some invalid instruments using a weighted median estimator. Genet Epidemiol. (2016) 40:304–14. doi: 10.1002/gepi.21965
21. Bowden J, Davey Smith G, Burgess S. Mendelian randomization with invalid instruments: effect estimation and bias detection through egger regression. Int J Epidemiol. (2015) 44:512–25. doi: 10.1093/ije/dyv080
22. Verbanck M, Chen CY, Neale B, Do R. Detection of widespread horizontal pleiotropy in causal relationships inferred from Mendelian randomization between complex traits and diseases. Nat Genet. (2018) 50:693–8. doi: 10.1038/s41588-018-0099-7
23. Kubota Y, Heiss G, MacLehose RF, Roetker NS, Folsom AR. Association of educational attainment with lifetime risk of cardiovascular disease: the atherosclerosis risk in communities study. JAMA Intern Med. (2017) 177:1165–72. doi: 10.1001/jamainternmed.2017.1877
24. Sulo G, Nygård O, Vollset SE, Igland J, Ebbing M, Sulo E, et al. Higher education is associated with reduced risk of heart failure among patients with acute myocardial infarction: a nationwide analysis using data from the CVDNOR project. Eur J Prev Cardiol. (2016) 23:1743–50. doi: 10.1177/2047487316655910
25. Tillmann T, Vaucher J, Okbay A, Pikhart H, Peasey A, Kubinova R, et al. Education and coronary heart disease: Mendelian randomisation study. Br Med J. (2017) 358:j3542. doi: 10.1136/bmj.j3542
26. Liao LZ, Zhuang XD, Zhang SZ, Liao XX, Li WD. Education and heart failure: new insights from the atherosclerosis risk in communities study and Mendelian randomization study. Int J Cardiol. (2021) 324:115–21. doi: 10.1016/j.ijcard.2020.09.068
27. Liberatos P, Link BG, Kelsey JL. The measurement of social class in epidemiology. Epidemiol Rev. (1988) 10:87–121. doi: 10.1093/oxfordjournals.epirev.a036030
28. Lawrence EM. Why do college graduates behave more healthfully than those who are less educated. J Health Soc Behav. (2017) 58:291–306. doi: 10.1177/0022146517715671
29. Gage SH, Bowden J, Davey Smith G, Munafò MR. Investigating causality in associations between education and smoking: a two-sample Mendelian randomization study. Int J Epidemiol. (2018) 47:1131–40. doi: 10.1093/ije/dyy131
30. Åsvold BO, Bjørngaard JH, Carslake D, Gabrielsen ME, Skorpen F, Smith GD, et al. Causal associations of tobacco smoking with cardiovascular risk factors: a Mendelian randomization analysis of the HUNT study in Norway. Int J Epidemiol. (2014) 43:1458–70. doi: 10.1093/ije/dyu113
31. Böckerman P, Viinikainen J, Pulkki-Råback L, Hakulinen C, Pitkänen N, Lehtimäki T, et al. Does higher education protect against obesity? Evidence using Mendelian randomization. Prev Med. (2017) 101:195–8. doi: 10.1016/j.ypmed.2017.06.015
32. Lyall DM, Celis-Morales C, Ward J, Iliodromiti S, Anderson JJ, Gill J, et al. Association of body mass index with cardiometabolic disease in the UK biobank: a Mendelian randomization study. JAMA Cardiol. (2017) 2:882–9. doi: 10.1001/jamacardio.2016.5804
33. Kershaw KN, Droomers M, Robinson WR, Carnethon MR, Daviglus ML, Monique Verschuren WM. Quantifying the contributions of behavioral and biological risk factors to socioeconomic disparities in coronary heart disease incidence: the MORGEN study. Eur J Epidemiol. (2013) 28:807–14. doi: 10.1007/s10654-013-9847-2
34. Dégano IR, Marrugat J, Grau M, Salvador-González B, Ramos R, Zamora A, et al. The association between education and cardiovascular disease incidence is mediated by hypertension, diabetes, and body mass index. Sci Rep. (2017) 7:12370. doi: 10.1038/s41598-017-10775-3
35. Carter AR, Gill D, Davies NM, Taylor AE, Tillmann T, Vaucher J, et al. Understanding the consequences of education inequality on cardiovascular disease: Mendelian randomisation study. Br Med J. (2019) 365:11855. doi: 10.1136/bmj.l1855
36. Stringhini S, Sabia S, Shipley M, Brunner E, Nabi H, Kivimaki M, et al. Association of socioeconomic position with health behaviors and mortality. JAMA. (2010) 303:1159–66. doi: 10.1001/jama.2010.297
Keywords: education, years of schooling, cardiovascular diseases, Mendelian randomization, causality
Citation: Liu W, Lin Q, Fan Z, Cui J and Wu Y (2024) Education and cardiovascular diseases: a Mendelian randomization study. Front. Cardiovasc. Med. 11:1320205. doi: 10.3389/fcvm.2024.1320205
Received: 12 October 2023; Accepted: 5 February 2024;
Published: 15 February 2024.
Edited by:
János Nemcsik, Semmelweis University, HungaryReviewed by:
Csaba Móczár, Semmelweis University, HungarySzilveszter Dolgos, St. Margaret Hospital, Hungary
Xingxing Li, The Hospital Affiliated to Shaanxi University of Chinese Medicine, China
© 2024 Liu, Lin, Fan, Cui and Wu. This is an open-access article distributed under the terms of the Creative Commons Attribution License (CC BY). The use, distribution or reproduction in other forums is permitted, provided the original author(s) and the copyright owner(s) are credited and that the original publication in this journal is cited, in accordance with accepted academic practice. No use, distribution or reproduction is permitted which does not comply with these terms.
*Correspondence: Yang Wu ZHJ3dXlhbmdAMTYzLmNvbQ==
Abbreviations BMI, body mass index; CVDs, cardiovascular diseases; GWAS, genome-wide association studies; IEU, integrative epidemiology unit; IVs, instrumental variables; IVW, inverse-variance weighted; MR, Mendelian randomization; RCTs, randomized controlled trials; SNPs, single nucleotide polymorphisms.