- 1Department of Hypertension, Henan Provincial People’s Hospital & Zhengzhou University People’s Hospital, Zhengzhou, China
- 2Department of Clinical Laboratory, The Third Affifiliated Hospital, Southern Medical University, Guangzhou, China
- 3Department of Medical Statistics, School of Public Health, Sun Yat-sen University, Guangzhou, China
- 4Department of Medical Imaging, Henan Provincial People’s Hospital & Zhengzhou University People’s Hospital, Zhengzhou, China
- 5The School of Electrical and Information Engineering, Zhengzhou University of Light Industry, Zhengzhou, China
- 6Department of Infectious Disease, the Third Affiliated Hospital of Sun Yat-sen University, Guangzhou, China
Background: The risk factors of cardiovascular disease (CVD) in end-stage renal disease (ESRD) with hemodialysis remain not fully understood. In this study, we developed and validated a clinical-longitudinal model for predicting CVD in patients with hemodialysis, and employed Mendelian randomization to evaluate the causal 6study included 468 hemodialysis patients, and biochemical parameters were evaluated every three months. A generalized linear mixed (GLM) predictive model was applied to longitudinal clinical data. Calibration curves and area under the receiver operating characteristic curves (AUCs) were used to evaluate the performance of the model. Kaplan-Meier curves were applied to verify the effect of selected risk factors on the probability of CVD. Genome-wide association study (GWAS) data for CVD (n = 218,792,101,866 cases), end-stage renal disease (ESRD, n = 16,405, 326 cases), diabetes (n = 202,046, 9,889 cases), creatinine (n = 7,810), and uric acid (UA, n = 109,029) were obtained from the large-open GWAS project. The inverse-variance weighted MR was used as the main analysis to estimate the causal associations, and several sensitivity analyses were performed to assess pleiotropy and exclude variants with potential pleiotropic effects.
Results: The AUCs of the GLM model was 0.93 (with accuracy rates of 93.9% and 93.1% for the training set and validation set, sensitivity of 0.95 and 0.94, specificity of 0.87 and 0.86). The final clinical-longitudinal model consisted of 5 risk factors, including age, diabetes, ipth, creatinine, and UA. Furthermore, the predicted CVD response also allowed for significant (p < 0.05) discrimination between the Kaplan-Meier curves of each age, diabetes, ipth, and creatinine subclassification. MR analysis indicated that diabetes had a causal role in risk of CVD (β = 0.088, p < 0.0001) and ESRD (β = 0.26, p = 0.007). In turn, ESRD was found to have a causal role in risk of diabetes (β = 0.027, p = 0.013). Additionally, creatinine exhibited a causal role in the risk of ESRD (β = 4.42, p = 0.01).
Conclusions: The results showed that old age, diabetes, and low level of ipth, creatinine, and UA were important risk factors for CVD in hemodialysis patients, and diabetes played an important bridging role in the link between ESRD and CVD.
1 Introduction
The end-stage renal disease (ESRD) is the leading cause of kidney-related mortality (1), accounting of over 1.2 million associated deaths annually worldwide (2). Patients with ESRD are candidates for kidney transplants (3), and have an overall 5-year survival rate reaching 80%−90% after transplants (4). However, maximizing access to transplants and reducing access disparities poses a challenge for patients receiving prompt kidney transplants (5). According to both the European and American ESRD management guidelines, hemodialysis (HD) is recommended as a method of renal replacement therapy (6). ESRD patients underlying HD enjoy a survival benefit (7), but nearly 20% of these patients would die from cardiovascular disease (CVD) within three years (8–10). For ESRD patients at high risk of CVD, timely clinical intervention holds the potential to prevent further deterioration of the viscera system and prolong survival time, particularly if targeted therapy can be initiated before ESRD progresses to the advanced stage (11, 12).
Several clinical factors are potential risk for ESRD who are likely to have CVD. Aging, hypertension, and diabetes can cause cardiovascular dysfunction in both the general population and HD patients (13–16). In addition, certain HD-specific risk factors, such as anemia, chronic inflammation, and metabolic disturbance, may be involved in the pathogenesis of CVD in HD patients (17). Conversely, adopting suitable lifestyle habits, such as a plant-based diet rich in fiber and low in protein, can reduce the CVD risk in HD patients (18, 19). Several predictive studies have been conducted to identify risk factors for CVD in HD patients and have shown promising results (20–23). However, these studies have only analyzed data from a single time point, which limits their ability to capture the developmental changes in risk factors over time and establish causal relationships among risk factors, CVD, and ESRD (24). Furthermore, the cross-sectional datasets used in these studies relied on simple regression techniques, focusing on population-averaged inference among variables. These reduce the performance of models and can lead to inconsistent results (25, 26). Thus, longitudinal data analysis is needed to identify clinical risk factors and improve the accuracy of CVD prediction in HD patients. This will ultimately contribute to more precise and effective clinical decision-making.
Mendelian randomization (MR) is a novel causality analysis method that utilizes the random allocation of genetic variants as instrumental variables to estimate the causal association between exposure and clinical outcomes (27, 28). MR provides robust causal inferences and is minimally affected by residual confounding. This is because genetic variants are randomly assigned from parents and normally fixed, making them unaffected by the outcomes and confounders. This random distribution of confounders is comparable to the randomization process in randomized controlled trials (29).
Therefore, in this research, longitudinal clinical data of HD patients would be analyzed to estimate and validate a prediction model. This model aims to serve as a useful tool for predicting CVD. Additionally, Mendelian randomization analysis was applied to evaluate the causal inferences between the risk factors and CVD in HD patients.
2 Materials and methods
2.1 The longitudinal population
In this retrospective study, a total of 609 HD patients were included from the hemodialysis centers of the Third Affiliated Hospital of Southern Medical University, Third Affiliated Hospital of Sun Yat-sen University, and Henan Provincial People's Hospital according between January 2015 to September 2022 with the following inclusion criteria: (1) regular hemodialysis for more than 12 months; (2) agegreater than 18 years old; (3) no prior history of CVD events before starting hemodialysis; (4) availability of clinical data matrix. Participants who met any of the following exclusion criteria were not included in this study: (1) pregnant; (2) currently suffering from ongoing infection; (3) diagnosed with malignancy. The study protocol was approved by the ethics committee in the Third Affiliated Hospital of Southern Medical University, the Third Affiliated Hospital of Sun Yat-sen University, and Henan Provincial People's Hospital.
2.2 The longitudinal data collection
At the start of the first hemodialysis therapy, demographic characteristics, medical history, and dialysis-related information, including dialysis frequency, dialysis volume, and vascular access was recorded by trained nurses. Before each hemodialysis session, patients underwent an assessment to establish the corresponding dialysis plan. This assessment primarily involved setting the blood flow rate, dialysis rate, and ultrafiltration rate based on factors such as body weight, heart rate, and blood pressure. During the hemodialysis process, real-time monitoring was conducted for the patient's blood pressure, heart rate, dialysate temperature, pre-pump arterial pressure, and post-pump arterial pressure. Additionally, nurses regularly monitored various indicators throughout the dialysis session and adjusted the dialysate flow rate according to the patient's specific condition. These measures ensure the safety and effectiveness of the hemodialysis procedure. Every approximately 4 months, overnight fasting blood samples were collected from each participant. Nineteen biochemical parameters, including blood routine (white blood cells (WBC), hemoglobin (HB), platelets (PLT), neutrophile granulocyte (NEU)), glucose, lipids (high density lipoprotein (HDL), low density lipoprotein (LDL), total cholesterol (CHOL), triglyceride (TG)), infectious disease antigen (HBsAg, hepatitis C virus (HCV), human immunodeficiency virus (HIV)), as well as liver and kidney function indicators (intact parathyroid hormone (ipth), neutrophile granulocyte (BUN), creatinine (CRE), uric acid (UA), calcium (Ca), phosphorus (P)), were measured at the laboratory and radiology of the three centers. Additionally, radiological examinations, including computed tomography, magnetic resonance angiography, and color ultrasound, were conducted annually for the diagnosis of CVD.
First non-fatal and fatal CVD events, including valvular heart disease, myocardial disease, arrhythmia extracorporeal defibrillation, cerebrovascular disease, and peripheral vascular disease after hemodialysis, were recorded (30, 31). CVD time was recorded from the first hemodialysis to the first CVD event. The data were collected from the three centers until September 30, 2022.
2.3 The longitudinal data analysis
The data was preprocessed using R software (version 4.2.2), including the following steps. Despite a standardized data collection form being applied, the presence of missing data in our data matrix was unavoidable due to measurements not being taken, failure to send or retrieve questionnaires, and errors in manual data entry. Firstly, the missing data rate was calculated for each subject at the identified time points. If a subject's missing data rate exceeded 30%, they were removed from the data matrix (32). Then, the missing values in the cleared data matrix were imputed using the “MissForest” R package. This package allows for the imputation of both continuous and categorical data, taking into account complex interactions and nonlinear relations (33).
To account for population-averaged inference and within-subject correlation between the repeated measures, we implemented a longitudinal model called generalized linear mixed model (GLMM) using IBM SPSS Statistics (version 26). The GLMM integrates both the fixed effects and random effects of predictors, which enhances the validity and reproducibility of the experimental findings (34). The fixed effects were used to measure whether candidate factors had an impact on the risk of CVD. However, considering that these factors were measured multiple times, there might be correlations introduced due to multiple measurements of the same observed variable. To account for this correlation and estimate the results more accurately, random effects was incorporated in our model to estimate whether the effect of risk factors varying at different time points. In the model, we used the logit link function to model the natural logarithm of the odds of CVD. The model could be expressed as the following form: log (p/1 - p) = Xβ + Zγ + ɛ, where p represented the p value of CVD; X was the design matrix for fixed effects; β represented the parameters vector for fixed effects; Z was the design matrix for random effects; γ represented the parameter vector for random effects; ɛ represented the vector of residual errors.
To compare the results with those of a traditional prediction model, we conducted a logistic regression model (LRM). The performance of both models was evaluated using a calibration curve and the area under the receiver operating characteristic curve (AUC). The calibration plot for the probability of objective response showed agreement between the predicted probabilities computed by the models and actual observations. The discrimination ability of the two models was measured by bootstrap-corrected AUCs.
The primary endpoint of interest was the probability of CVD, and the second endpoint of interest was the survival time. The former could be predicted by the GLMM. The variables which were significant (p < 0.05) in the GLMM were chosen as risk factors. To assess the survival time across different risk factors, the continuous variables such as age, ipth, CRE, and UA were binarization by the “survminer” package, an R package to determine the optimal cut point (35). The survival curves of each risk factor were constructed using the Kaplan-Meier method and compared with the log-rank test.
2.4 Mendelian randomization
The GWAS summary statistics of ERSD, CVD, diabetes, creatinine, and uric acid were searched and downloaded from the IEU Open GWAS project (https://gwas.mrcieu.ac.uk/), which includes large amounts of standard format of human GWAS summary statistics. There was no overlap between the participants in the GWAS study and the HD patients used for longitudinal analysis mentioned above. Some basic information of the population in the five GWAS statistics was shown in Supplementary Table S1.
Risk genetic variants [single-nucleotide polymorphisms (SNPs)] associated ( for CVD, diabetes, creatinine and uric acid and for ESRD) with exposures were selected as instruments. The threshold for ESRD was relaxed because no SNP reached for reference to the strategy applied in previous MR studies (36). SNPs were excluded in moderate linkage disequilibrium (r2 > 0.001) to reduce bias due to genetic correlation. The linkage disequilibrium across these SNPs were calculated based on the European 1000-Genomers reference panel. SNPs who had association () with outcomes were excluded to obtain the assumption of MR that instrumental variables are strongly associated with exposure and have no direct association with the outcome. We also excluded SNPs that were palindromic and had an intermediate allele frequency to further reduce potential bias and uncertainty, as previously described (37). Outliers were determined with respect to their contribution to global heterogeneity, quantified by Cochran's Q-statistic, using a significance threshold of 0.05 for p-value, 1 for the inverse variance weights, and 0.0001 for the tolerance threshold for performing the iterative inverse-variance weighted (IVW) approach (38).
The following bidirectional univariate MR analyses were conducted separately to determine the causal effects of each of genetically predicted risk factors (diabetes, creatinine, and uric acid) phenotype on CVD and ERSD risk, and then vice versa.
The IVW approach was applied as the main MR model, which provides most accurate estimate under the assumption that the instrumental variables were all valid (39). In order to account for potential heterogeneity and horizontal pleiotropy, three additional approaches for MR analyses including MR-Egger regression, weighted median and weighted mode methods, were applied. Cochran's Q test and MR-Egger intercept test were used to detect the heterogeneity and horizontal pleiotropy, respectively.
The odds ratios (ORs) and corresponding 95% confidence intervals (CSs) were used to assess the strength of causal associations. All p values were two-sided and this study used conventional significance level (p < 0.05). Genetic instrument selection and MR analyses were carried out using “TwoSampleMR” and “RadialMR” package in R.
3 Results
Four hundred sixty-eight subjects were included after data cleaning, 221 from the Third Affiliated Hospital of Southern Medical University, 179 from the Third Affiliated Hospital of Sun Yat-sen University, and 68 from the Henan Provincial People's Hospital. Demographic information and biochemical parameters at baseline were shown in Table 1. 36.75% of the HD patients developed CVD within the following 4.7 years. Chi-square test showed that subjects with diabetes were more likely to have CVD (44.71% vs. 27.18%, p < 0.001). Two sample t-test showed that the CVD group had higher age (59.84 ± 14.13 vs. 52.07 ± 15.39, p < 0.001), WBC (7.67 ± 3.09 vs. 6.96 ± 2.99, p = 0.02), PLT (218.64 ± 72.74 vs. 198.86 ± 71.99, p = 0.005), lymph (2.12 ± 2.81 vs. 1.43 ± 1.16, p < 0.001), as well as lower ipth (361.67 ± 274.27 vs. 507.75 ± 430.19, p < 0.001), BUN (22.69 ± 11.16 vs. 25.07 ± 9.90, p = 0.02), CRE (709.31 ± 422.84 vs. 943.21 ± 340.93, p < 0.001), and P (1.76 ± 0.62 vs. 1.97 ± 0.60, p < 0.001), compared to the non-CVD group. No other group differences were found at baseline. The change over time of continuous biochemical parameters was shown in Figure 1.
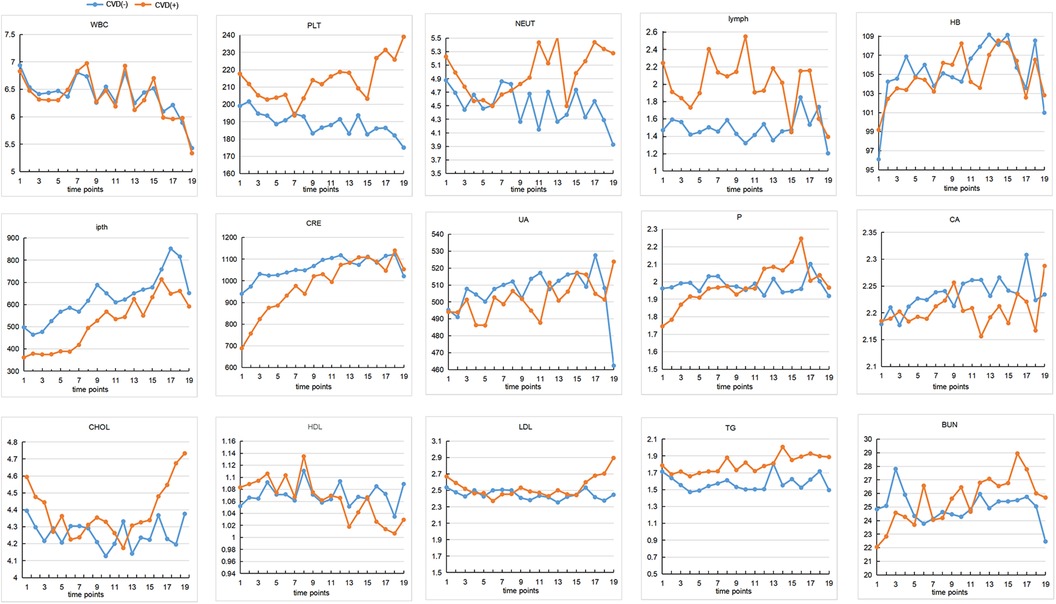
Figure 1 The changes of continuous biochemical variables. The interval between each time point on the horizontal coordinate is 4 months. The vertical axis represents the content of each variable. CVD, cardiovascular disease; WBC, white blood cells; HB, hemoglobin; PLT, platelets; NEU, neutrophile granulocyte; ipth, intact parathyroid hormone; BUN, blood urea nitrogen; CRE, creatinine; UA, uric acid; CA, calcium; P, phosphorus; CHOL, total cholesterol; TG, triglyceride; HDL, high density lipoprotein; LDL, low density lipoprotein.
The total classification accuracy for the LRM and GLMM models was 81.4% and 93.9%, respectively. The coefficient of age, diabetes, combined disease, WBC, PLT, NEUT, lymph, P, HBsAg, and HIV was significant (p < 0.05) in the LRM. In the GLMM model, the fixed effects of age, diabetes, ipth, CRE, and UA were statistically significant (p < 0.05); the random effect of ipth and P was significant (p < 0.05). The detail of the two models was shown in Table 2. The calibration plot for the GLMM was closer to the ideal curve than the calibration plot for the LRM (Figure 1A). The AUCs of the LRM and GLMM were 0.833 and 0.93, respectively (Figure 2B).
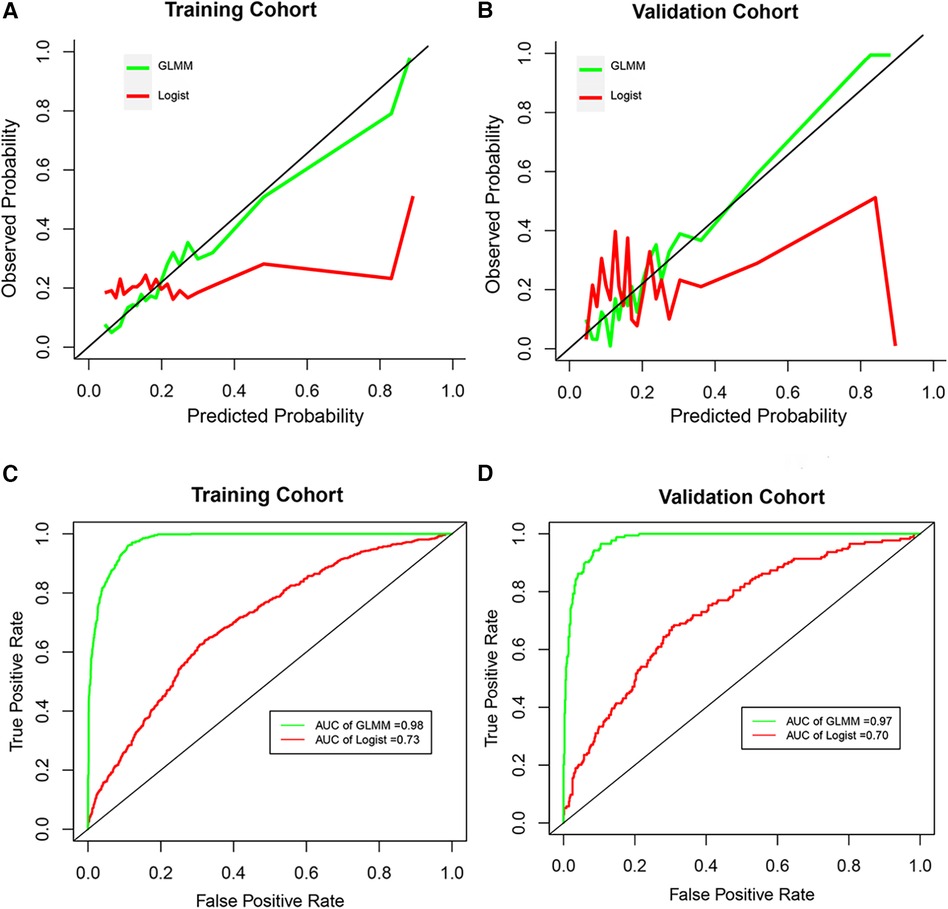
Figure 2 The calibration curve and receiver operation characteristic curve. (A and B) Represented the calibration curve in the training cohort and validation cohort, respectively. (C and D) Represented the receiver operation characteristic curve in the training cohort and validation cohort, respectively. GLMM, generalized linear mixed model; Logist, logistic regression model; AUC, area under the receiver operating characteristic curves.
The cut points for age, ipth, CRE, and UA were 56 years old, 489 pg/ml, 7.53 umol/L, and 1.42 umol/L. The cut points divided these continuum factors into two hierarchical levels. The Kaplan-Meier curves for CVD outcome between two hierarchical levels satisfied statistically significant differences (p < 0.05) in the actual CVD response and predicted response by the GLMM model, except risk factor of UA (Figure 3).
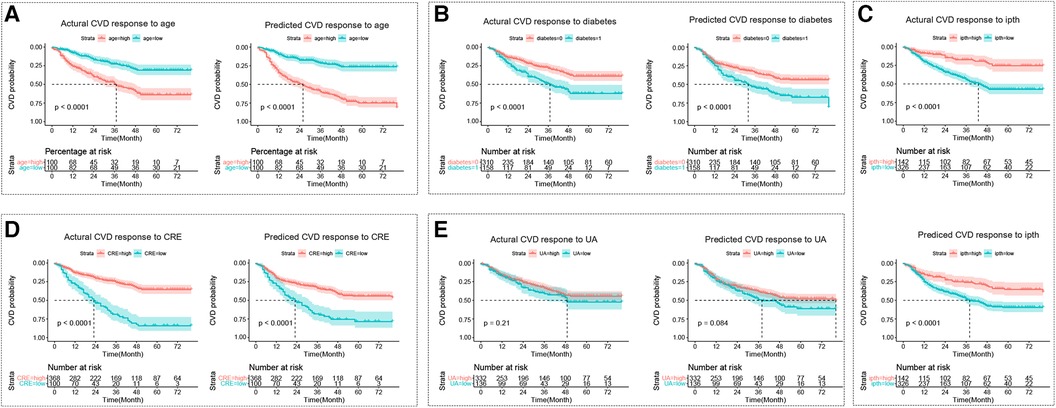
Figure 3 The kaplan-meier curve for the cardiovascular disease response. (A–E) Represent Kaplan-Meier curves for each sub-classification of age, diabetes, ipth, CRE, and UA, respectively. The cut points for age, ipth, CRE, and UA were 56 years old, 489 pg/ml, 7.53 umol/L, and 1.42 umol/L. In sub-figure b, diabetes = 0 represented no diabetes group, and diabetes = 1 represented diabetes group. CVD, cardiovascular disease; HD, hemodialysis patients; ipth, intact parathyroid hormone; CRE, creatinine; UA, uric acid (UA).
3.1 Causal-relationships among risk factors, ESRD, and CVD
The possible causal relationships among risk factors, ESRD, and CVD was shown in Figure 4. The two-sample IVW MR provided evidence for a causal role of diabetes in risk of CVD (β = 0.088, p < 0.0001) and ERSD (β = 0.26, p = 0.007). In turn, ERSD had a causal role of diabetes (β = 0.027, p = 0.013). In addition, diabetes could decrease the level of uric acid (β = −0.033, p = 0.045). The increase of creatinine level had a causal role in risk of ESRD (β = 4.42, p = 0.01). There was no evidence of horizontal pleiotropy (p of EI > 0.05) in these causal inferences except the causal inference from diabetes to CVD (p of EI = 0.045) (Supplementary Tables S2–S6). There was no evidence of heterogeneity except in the causal inference from diabetes to CVD, uric acid, and ESRD (p of Q < 0.05). No other causal relationship was found in this study (Supplementary Table S2–S6). The F statistics of SNPs were shown in Supplementary Table S7. The effect size of individual SNP and all SNPs was shown in Supplementary Figure S1.
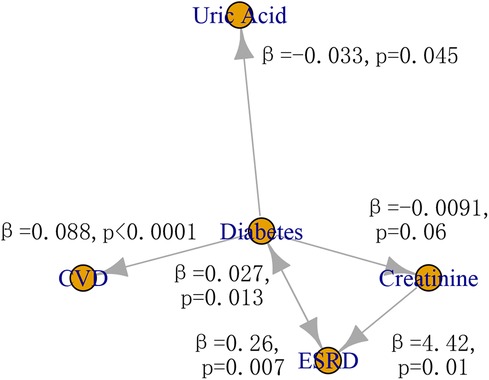
Figure 4 The causal relationship among risk factors, CVD, and ESRD. CVD, cardiovascular disease; ESRD, end-stage renal disease.
4 Discussion
To the best of our knowledge, this was the first longitudinal cohort study to investigate the causal relationships among risk factors of CVD in patients with ESRD. We developed and validated a clinical GLMM model for selecting risk factors of CVD in patients with hemodialysis based on longitudinal data. Additionally, MR analyses were performed to evaluate the causal relationships among risk factors, CVD, and ESRD. The GLMM model revealed that diabetes, creatinine, UA, and ipth were risk factors for CVD. The MR analyses provided robust genetic evidence supporting the notion that diabetes played a causal role in the relationship between ESRD and CVD, which raised the importance of blood sugar control.
The predictors used in our prediction model integrated various clinical variables and were found to be one of the most important components in estimating the status of the body system. Notably, most of the significant predictors, such as diabetes, ipth, CRE, and UA, were related to metabolism. This finding in consistent with previous studies that have shown metabolic dysfunctions, including diabetes, obesity, and uarthritis, to be involved in aberrations preceding clinically overt CVD (40–43). Furthermore, a recent meta-analysis corroborated these results, revealing association between metabolic dysfunction and CVD (44).
Previous studies have primarily focused on the effects of predictors at a single time point, neglecting the longitudinal effectsin the analysis. In this study, we aimed to examine the potential risk factors for both independence and relevance by using the GLMM model with longitudinal clinical data. Recent studies on degenerative joint disease, COVID-19, and pulmonary parenchymal lesions have revealed that associations that are not apparent in other frameworks can be elucidated using GLMMs (45–47). In our study, compared to the traditional logistic regression model, the GLMM model exhibited higher classification accuracy, indicating a better ability to explain variability. Hence, we selected and identified the results from the GLMM for further analysis in this study. Notably, a significant difference between the two models lies in the predictors. The traditional logistic regression model indicated that predictors such as WBC, PLT, NEUT, and lymph were primarily associated with the immune system. While, in the GLMM, predictors like ipth, CRE, and UA, were predominantly linked to the metabolism system and were not significant in the logistic regression model. Although inflammation has long been considered to be associated with CVD in hemodialysis patients, recent evidence has demonstrated a close relationship between ipth, CRE, UA and CVD (48–51). It is important to note that these predictors had considerable standard deviations, implying substantial interindividual variability, which poses a challenge to the consistency of the results. This variability might explain why the effects of ipth and CRE on CVD in HD patients show inconsistent directions, as both negative (26, 52, 53) and positive (54–56) associations have been reported. On the other hand, clinical data inherently exhibits universal and multidimensional variability due to factors such as exercise, diet, psychology, and some random or non-random noise. However, longitudinal analysis can help “minimized” this variability by capturing diverse patterns of intra- and/or inter-personal variability (57). Therefore, even though no significant differences were observed in UA levels at the baseline, the GLMM revealed UA as a significant predictor (p < 0.05). This finding is consistent with a recent meta study that a robust and independent association between elevated UA levels and lower risk of cardiovascular mortality in maintenance hemodialysis patients (58).
In addition to assessing CVD occurrence, the performance of our GLMM was evaluated by the Kaplan-Meier curves. It was observed that the Kaplan-Meier curve of the CVD occurrence or no occurrence predicted by our GLMM was similar to the curve of the CVD status observed by a physician. In addition, the utility of the GLMM was convincingly supported by the significant differences in Kaplan-Meier curves across different levels of age, diabetes, ipth, CRE, and UA, all of which were identified as significant predictors by the GLMM. Our results showed that patients with older age, diabetes, lower ipth, lower CRE, or lower UA were at a higher risk of CVD. The causal role of old age and diabetes have long been recognized, and the possible mechanisms have been extensively studies (59, 60). There is ample evidence to suggest that lower ipth, CRE, or UA is associated with a loss of autonomy or inflammation (61–63). However, the relationships among these risk factors, CVD and ESRD remain unclear.
Our MR analyses showed that diabetes played an important bridging role among them, suggesting the importance of blood glucose control in preventing both CVD and ESRD. Consistent with previous MR results, diabetes has causal effect on cardiovascular injury and kidney disease (64, 65). Recent MR studies from different cohorts have reported no causal effect of uric acid type 2 diabetes, although a significant association have been widely reported, which is in line with our result (66–68). Unlike previous MR studies, bidirectional causal effect was considered to identify some new causal effects in this study. Through bidirectional MR analysis, diabetes was also found to have a causal role in the decrease of uric acid and creatinine, which were risk factors identified by the longitudinal analysis. Our findings may help explain the associations between diabetes and clinical factors such as uric acid and creatinine observed in previous observational studies (69, 70).
Despite the causal relationships between the risk factors and CVD in HD, this study has several limitations. Firstly, this is a retrospective study, and several clinical variables could not be measured. For example, the absence of various proinflammatory cytokines, such as interleukin-1 beta, interleukin 6, and transforming growth factor beta (71), makes it impossible to further explore the relationship between the inflammatory response and the risk factors identified in this research. In addition, the nutritional status is also an important indicator, as dialysis patients often face the problem of inadequate nutrition intake, and nutrition can potentially affect patients' quality of life and treatment outcomes (72). Secondly, although subjects were selected based on the inclusion criteria, the heterogeneity across patients due to factors like medication, lifestyle habits, and uncounted confounding factors, might yeild a null hypothesis. Thirdly, we did not classify the type of CVD, but the impact of risk factors might differ across CVD subtypes. Future studies with sufficiently large sample sizes can be carried out to explore the difference among CVD subtypes. Currently, our model assists clinicians in selecting targeted agents in advance to enable appropriate treatment choices for HD patients. Finally, horizontal pleiotropy and heterogeneity resulting from population variances in GWAS statistics can affect the MR results. In order to provide consistent and robust causal estimation, multiple sensitivity analyses, such as simple median, weighted median, and MR-Egger methods, were employed in this study. In future, larger sample size studies are needed to validate these issues.
In conclusion, our longitudinal model revealed that old age, diabetes, depressed ipth, CRE, and UA were risk factors for CVD in HD patients, with diabetes playing an important bridging role among them. Effective blood glucose control is crucial for preventing CVD in HD patients.
Data availability statement
The original contributions presented in the study are included in the article/Supplementary Material, further inquiries can be directed to the corresponding authors.
Ethics statement
The studies involving humans were approved by Ethics Committee of Henan Provincial People's Hospital. The studies were conducted in accordance with the local legislation and institutional requirements. The participants provided their written informed consent to participate in this study.
Author contributions
DT: Conceptualization, Formal Analysis, Methodology, Writing – original draft. YX: Conceptualization, Data curation, Investigation, Methodology, Writing – review & editing. YW: Formal Analysis, Software, Writing – review & editing. XZ: Data curation, Writing – review & editing. CH: Data curation, Methodology, Writing – review & editing. ML: Conceptualization, Funding acquisition, Writing – review & editing. PL: Conceptualization, Formal Analysis, Funding acquisition, Writing – review & editing. XL: Conceptualization, Funding acquisition, Writing – review & editing.
Funding
The author(s) declare financial support was received for the research, authorship, and/or publication of this article.
This work was supported by the Third Affifiliated Hospital of Sun Yat-Sen University, Clinical Research Program (P000-277), the Natural Science Foundation of Guangdong (2022A1515012597), the Meizhou Science and Technology Project (2019B0203003), the National Natural Science Foundation of China (82270463), the National Natural Science Foundation of Henan (222300420376) and the Doctoral Research Start-up Foundation of Zhengzhou University of light Industry (2020BSJJ007).
Acknowledgments
The authors thank all participants for their contribution to our study.
Conflict of interest
The authors declare that the research was conducted in the absence of any commercial or financial relationships that could be construed as a potential conflict of interest.
Publisher's note
All claims expressed in this article are solely those of the authors and do not necessarily represent those of their affiliated organizations, or those of the publisher, the editors and the reviewers. Any product that may be evaluated in this article, or claim that may be made by its manufacturer, is not guaranteed or endorsed by the publisher.
Supplementary material
The Supplementary Material for this article can be found online at: https://www.frontiersin.org/articles/10.3389/fcvm.2024.1306159/full#supplementary-material
References
1. Ruiz-Ortega M, Rayego-Mateos S, Lamas S, Ortiz A, Rodrigues-Diez RR. Targeting the progression of chronic kidney disease. Nat Rev Nephrol. (2020) 16(5):269–88. doi: 10.1038/s41581-019-0248-y
2. Cheng HT, Xu X, Lim PS, Hung KY. Worldwide epidemiology of diabetes-related end-stage renal disease, 2000–2015. Diabetes Care. (2021) 44(1):89–97. doi: 10.2337/dc20-1913
3. Fanouriakis A, Kostopoulou M, Cheema K, Anders HJ, Aringer M, Bajema I, et al. 2019 update of the Joint European League Against Rheumatism and European Renal Association-European Dialysis and Transplant Association (EULAR/ERA-EDTA) recommendations for the management of lupus nephritis. Ann Rheum Dis. (2020) 79(6):713–23. doi: 10.1136/annrheumdis-2020-216924
4. Kramer A, Pippias M, Noordzij M, Stel VS, Andrusev AM, Aparicio-Madre MI, et al. The European Renal Association - European dialysis and transplant association (ERA-EDTA) registry annual report 2016: a summary. Clin Kidney J. (2019) 12(5):702–20. doi: 10.1093/ckj/sfz011
5. Hart A, Lentine KL, Smith JM, Miller JM, Skeans MA, Prentice M, et al. OPTN/SRTR 2019 annual data report: kidney. Am J Transplant. (2021) 21:21–137. doi: 10.1111/ajt.16502
6. Ikizler TA, Burrowes JD, Byham-Gray LD, Campbell KL, Carrero J-J, Chan W, et al. KDOQI clinical practice guideline for nutrition in CKD: 2020 update. Am J Kidney Dis. (2020) 76(3):S1–S107. doi: 10.1053/j.ajkd.2020.05.006
7. Zhang Y, Gerdtham UG, Rydell H, Jarl J. Quantifying the treatment effect of kidney transplantation relative to dialysis on survival time: new results based on propensity score weighting and longitudinal observational data from Sweden. Int J Environ Res Public Health. (2020) 17(19):7318. doi: 10.3390/ijerph17197318
8. Murashima M, Hamano T, Abe M, Masakane I. Combination of once-weekly haemodialysis with peritoneal dialysis is associated with lower mortality compared with peritoneal dialysis alone: a longitudinal study. Clin Kidney J. (2021) 14(6):1610–7. doi: 10.1093/ckj/sfaa173
9. Genovesi S, Boriani G, Covic A, Vernooij RWM, Combe C, Burlacu A, et al. Sudden cardiac death in dialysis patients: different causes and management strategies. Nephrol Dial Transplant. (2021) 36(3):396–405. doi: 10.1093/ndt/gfz182
10. Jankowski J, Floege J, Fliser D, Bohm M, Marx N. Cardiovascular disease in chronic kidney disease: pathophysiological insights and therapeutic options. Circulation. (2021) 143(11):1157–72. doi: 10.1161/CIRCULATIONAHA.120.050686
11. Moe SM, Chertow GM, Parfrey PS, Kubo Y, Block GA, Correa-Rotter R, et al. Cinacalcet, fibroblast growth factor-23, and cardiovascular disease in hemodialysis the evaluation of cinacalcet HCl therapy to lower cardiovascular events (EVOLVE) trial. Circulation. (2015) 132(1):27–39. doi: 10.1161/CIRCULATIONAHA.114.013876
12. Ursi R, Pesce F, Albanese M, Pavone V, Grande D, Ciccone MM, et al. Reverse cardiac remodeling after fluid balance optimization in patients with end-stage renal disease. Hemodial Int. (2022) 26(3):345–50. doi: 10.1111/hdi.13019
13. Li J, Huang N, Zhong Z, Joe P, Wang D, Ai Z, et al. Risk factors and outcomes of cardiovascular disease readmission within the first year after dialysis in peritoneal dialysis patients. Ren Fail. (2021) 43(1):159–67. doi: 10.1080/0886022X.2020.1866009
14. Wang Y, Miao X, Xiao G, Huang C, Sun J, Wang Y, et al. Clinical prediction of heart failure in hemodialysis patients: based on the extreme gradient boosting method. Front Genet. (2022) 13:889378. doi: 10.3389/fgene.2022.889378
15. Sivanantham P, Kar SS, Lakshminarayanan S, Sahoo JP, Bobby Z, Varghese C. Performance of WHO updated cardiovascular disease risk prediction charts in a low-resource setting-findings from a community-based survey in puducherry, India. Nutr Metab Cardiovasc Dis. (2022) 32(9):2129–36. doi: 10.1016/j.numecd.2022.05.024
16. Mohamud MFY, Adan FN, Jeele MOO, Ahmed MAM. Major cardiovascular events and associated factors among routine hemodialysis patients with end-stage renal disease at tertiary care hospital in Somalia. Front Med (Lausanne). (2023) 10:1086359. doi: 10.3389/fmed.2023.1086359
17. Cozzolino M, Mangano M, Stucchi A, Ciceri P, Conte F, Galassi A. Cardiovascular disease in dialysis patients. Nephrol Dial Transplant. (2018) 33(suppl_3):iii28–34. doi: 10.1093/ndt/gfy174
18. Kalantar-Zadeh K, Joshi S, Schlueter R, Cooke J, Brown-Tortorici A, Donnelly M, et al. Plant-dominant low-protein diet for conservative management of chronic kidney disease. Nutrients. (2020) 12(7):1931. doi: 10.3390/nu12071931
19. Teymoori F, Mokhtari E, Farhadnejad H, Mirmiran P, Rad HA, Azizi F. The dietary and lifestyle indices of insulin resistance are associated with increased risk of cardiovascular diseases: a prospective study among an Iranian adult population. Nutr Metab Cardiovasc Dis. (2022) 32(9):2216–26. doi: 10.1016/j.numecd.2022.05.022
20. You X, Huang YY, Wang Y, Yu MX, Li XY, Xu L, et al. Prediction model for cardiovascular disease risk in hemodialysis patients. Int Urol Nephrol. (2022) 54(5):1127–34. doi: 10.1007/s11255-021-02984-7
21. Braunisch MC, Mayer CC, Bauer A, Lorenz G, Haller B, Rizas KD, et al. Cardiovascular mortality can be predicted by heart rate turbulence in hemodialysis patients. Front Physiol. (2020) 11:77. doi: 10.3389/fphys.2020.00077
22. Ouyang H, Shi QH, Zhu J, Shen HY, Jiang S, Song K. Nomogram for predicting 1-, 5-, and 10-year survival in hemodialysis (HD) patients: a single center retrospective study. Renal Fail. (2021) 43(1):1508–19. doi: 10.1080/0886022X.2021.1997762
23. Lee YH, Kim JS, Jung SW, Hwang HS, Moon JY, Jeong KH, et al. Gait speed and handgrip strength as predictors of all-cause mortality and cardiovascular events in hemodialysis patients. BMC Nephrol. (2020) 21(1):11. doi: 10.1186/s12882-020-1684-4
24. Burlacu A, Iftene A, Popa IV, Crisan-Dabija R, Brinza C, Covic A. Computational models used to predict cardiovascular complications in chronic kidney disease patients: a systematic review. Medicina-Lithuania. (2021) 57(6):11. doi: 10.3390/medicina57060538
25. Coyne SM, Rogers AA, Zurcher JD, Stockdale L, Booth M. Does time spent using social media impact mental health?: an eight year longitudinal study. Comput Human Behav. (2020) 104:106160. doi: 10.1016/j.chb.2019.106160
26. Jia F, Wang S, Jing Y, Zhao H, Rong P, Zhang H, et al. Osteocalcin and abdominal aortic calcification in hemodialysis patients: an observational cross-sectional study. Front Endocrinol (Lausanne). (2021) 12:620350. doi: 10.3389/fendo.2021.620350
27. Venkatesh SS, Ferreira T, Benonisdottir S, Rahmioglu N, Becker CM, Granne I, et al. Obesity and risk of female reproductive conditions: a Mendelian randomisation study. PLoS Med. (2022) 19(2):30. doi: 10.1371/journal.pmed.1003679
28. Liu XM, Tong X, Zou YQ, Lin XQ, Zhao H, Tian L, et al. Mendelian randomization analyses support causal relationships between blood metabolites and the gut microbiome. Nat Genet. (2022) 54(1):52–61. doi: 10.1038/s41588-021-00968-y
29. Taschler B, Smith SM, Nichols TE. Causal inference on neuroimaging data with Mendelian randomisation. Neuroimage. (2022) 258:20. doi: 10.1016/j.neuroimage.2022.119385
30. You X, Gu B, Chen T, Li X, Xie G, Sang C, et al. Development of long-term cardiovascular disease risk prediction model for hemodialysis patients with end-stage renal disease based on nomogram. Ann Palliat Med. (2021) 10(3):3142–53. doi: 10.21037/apm-21-286
31. Kasiske BL, Wheeler DC. KDIGO clinical practice guideline for the evaluation and management of chronic kidney disease foreword. Kidney Int Suppl (2011). (2013) 3(1):2. doi: 10.1038/kisup.2012.74
32. Pedersen AB, Mikkelsen EM, Cronin-Fenton D, Kristensen NR, Pham TM, Pedersen L, et al. Missing data and multiple imputation in clinical epidemiological research. Clin Epidemiol. (2017) 9:157–66. doi: 10.2147/CLEP.S129785
33. Stekhoven DJ, Buhlmann P. MissForest-non-parametric missing value imputation for mixed-type data. Bioinformatics. (2012) 28(1):112–8. doi: 10.1093/bioinformatics/btr597
34. Yu ZX, Guindani M, Grieco SF, Chen LJ, Holmes TC, Xu XM. Beyond t-test and ANOVA: applications of mixed-effects models for more rigorous statistical analysis in neuroscience research. Neuron. (2022) 110(1):21–35. doi: 10.1016/j.neuron.2021.10.030
35. Li SY, Chen S, Wang BX, Zhang L, Su YN, Zhang XP. A robust 6-lncRNA prognostic signature for predicting the prognosis of patients with colorectal cancer metastasis. Front Med (Lausanne). (2020) 7:10. doi: 10.3389/fmed.2020.00010
36. He LJ, Yu TT, Zhang W, Wang BJ, Ma YF, Li S. Causal associations of obesity with Achilles tendinopathy: a two-sample Mendelian randomization study. Front Endocrinol (Lausanne). (2022) 13:902412. doi: 10.3389/fendo.2022.902142
37. Hemani G, Zhengn J, Elsworth B, Wade KH, Haberland V, Baird D, et al. The MR-base platform supports systematic causal inference across the human phenome. Elife. (2018) 7. doi: 10.7554/eLife.34408
38. Bowden J, Spiller W, Del Greco MF, Sheehan N, Thompson J, Minelli C, et al. Improving the visualization, interpretation and analysis of two-sample summary data Mendelian randomization via the radial plot and radial regression. Int J Epidemiol. (2018) 47(4):1264–78. doi: 10.1093/ije/dyy101
39. Sha ZM, Hou TZC, Zhou TJ, Dai Y, Bao YY, Jin Q, et al. Causal relationship between atrial fibrillation and leukocyte telomere length: a two sample, bidirectional Mendelian randomization study. Front Cardiovasc Med. (2023) 10:1093255. doi: 10.3389/fcvm.2023.1093255
40. Asnicar F, Berry SE, Valdes AM, Nguyen LH, Piccinno G, Drew DA, et al. Microbiome connections with host metabolism and habitual diet from 1,098 deeply phenotyped individuals. Nat Med. (2021) 27(2):321–32. doi: 10.1038/s41591-020-01183-8
41. Talmor-Barkan Y, Bar N, Shaul AA, Shahaf N, Godneva A, Bussi Y, et al. Metabolomic and microbiome profiling reveals personalized risk factors for coronary artery disease. Nat Med. (2022) 28(2):259–302. doi: 10.1038/s41591-022-01686-6
42. Drosos GC, Vedder D, Houben E, Boekel L, Atzeni F, Badreh S, et al. EULAR recommendations for cardiovascular risk management in rheumatic and musculoskeletal diseases, including systemic lupus erythematosus and antiphospholipid syndrome. Ann Rheum Dis. (2022) 81(6):768–79. doi: 10.1136/annrheumdis-2021-221733
43. Ren Z, Sun W, Wang S, Ying J, Liu W, Fan L, et al. Status and transition of normal-weight central obesity and the risk of cardiovascular diseases: a population-based cohort study in China. Nutr Metab Cardiovasc Dis. (2022) 32(12):2794–802. doi: 10.1016/j.numecd.2022.07.023
44. Zhang Y-B, Pan X-F, Chen J, Cao A, Xia L, Zhang Y, et al. Combined lifestyle factors, all-cause mortality and cardiovascular disease: a systematic review and meta-analysis of prospective cohort studies. J Epidemiol Community Health. (2021) 75(1):92–9. doi: 10.1136/jech-2020-214050
45. Alonso-Llamazares C, Blanco Marquez B, Lopez B, Pardinas AF. Assessing individual and population variability in degenerative joint disease prevalence using generalized linear mixed models. Am J Phys Anthropol. (2021) 175(3):611–25. doi: 10.1002/ajpa.24195
46. Saez M, Tobias A, Varga D, Antonia Barcelo M. Effectiveness of the measures to flatten the epidemic curve of COVID-19. The case of Spain. Sci Total Environ. (2020) 727:138761. doi: 10.1016/j.scitotenv.2020.138761
47. Kalchiem-Dekel O, Connolly JG, Lin IH, Husta BC, Adusumilli PS, Beattie JA, et al. Shape-sensing robotic-assisted bronchoscopy in the diagnosis of pulmonary parenchymal lesions. Chest. (2022) 161(2):572–82. doi: 10.1016/j.chest.2021.07.2169
48. Galan Carrillo I, Vega A, Goicoechea M, Shabaka A, Gatius S, Abad S, et al. Impact of serum magnesium levels on kidney and cardiovascular prognosis and mortality in CKD patients. J Ren Nutr. (2021) 31(5):494–502. doi: 10.1053/j.jrn.2020.09.004
49. Fan HJ, Wang CH, Hsu BG, Tsai JP. Association between serum adipocyte fatty acid binding protein level and endothelial dysfunction in chronic hemodialysis patients. Life (Basel). (2022) 12(2):316. doi: 10.3390/life12020316
50. Liu Z-q, Huang Z-w, Kang S-l, Hu C-c, Chen F, He F, et al. Serum uric acid and cardiovascular or all-cause mortality in peritoneal dialysis patients: a systematic review and meta-analysis. Front Cardiovasc Med. (2021) 8:751182. doi: 10.3389/fcvm.2021.751182
51. Li M, Ye Z-C, Li C-M, Zhao W-B, Tang H, Liu X, et al. Low serum uric acid levels increase the risk of all-cause death and cardiovascular death in hemodialysis patients. Renal Fail. (2020) 42(1):315–22. doi: 10.1080/0886022X.2020.1745234
52. He J, Sun X, Nie R, Zhao L. Relationship between serum parathyroid hormone levels and abdominal aortic calcification in patients starting hemodialysis who have never taken calcium tablets, calcitriol, or vitamin D analogs. Renal Fail. (2022) 44(1):1409–16. doi: 10.1080/0886022X.2022.2114369
53. Yajima T, Arao M, Yajima K, Takahashi H. Usefulness of computed tomography-measured psoas muscle thickness per height for predicting mortality in patients undergoing hemodialysis. Sci Rep. (2021) 11(1):19070. doi: 10.1038/s41598-021-98613-5
54. Xu H, Peng W, Yang Z, Zhang Y, Xia C, Li Z, et al. The association of secondary hyperparathyroidism and myocardial damages in hemodialysis end-stage renal disease patients: assessed by cardiovascular magnetic resonance native T1 mapping. J Cardiovasc Magn Reson. (2021) 23(1):23. doi: 10.1186/s12968-021-00713-8
55. Liu F, Cui B, Zhao X, Wu Y, Qin H, Guo Y, et al. Correlation of serum adropin levels with risk factors of cardiovascular disease in hemodialysis patients. Metab Syndr Relat Disord. (2021) 19(7):401–8. doi: 10.1089/met.2021.0015
56. Yamamoto S, Matsuzawa R, Hoshi K, Suzuki Y, Harada M, Watanabe T, et al. Modified creatinine Index and clinical outcomes of hemodialysis patients: an indicator of sarcopenia? J Ren Nutr. (2021) 31(4):370–9. doi: 10.1053/j.jrn.2020.08.006
57. Zhou W, Sailani MR, Contrepois K, Zhou Y, Ahadi S, Leopold SR, et al. Longitudinal multi-omics of host-microbe dynamics in prediabetes. Nature. (2019) 569(7758):663–71. doi: 10.1038/s41586-019-1236-x
58. Wang H, Liu J, Xie D, Liu H, Zhen L, Guo D, et al. Elevated serum uric acid and risk of cardiovascular or all-cause mortality in maintenance hemodialysis patients: a meta-analysis. Nutr Metab Cardiovasc Dis. (2021) 31(2):372–81. doi: 10.1016/j.numecd.2020.11.017
59. Jaiswal S, Libby P. Clonal haematopoiesis: connecting ageing and inflammation in cardiovascular disease. Nat Rev Cardiol. (2020) 17(3):137–44. doi: 10.1038/s41569-019-0247-5
60. Nona P, Russell C. Cardio- rheumatology prevention of cardiovascular disease in inflammatory disorders. Med Clin N Am. (2022) 106(2):349–63. doi: 10.1016/j.mcna.2021.11.010
61. Villain C, Ecochard R, Bouchet JL, Daugas E, Drueke TB, Hannedouche T, et al. Relative prognostic impact of nutrition, anaemia, bone metabolism and cardiovascular comorbidities in elderly haemodialysis patients. Nephrol Dial Transplant. (2019) 34(5):848–58. doi: 10.1093/ndt/gfy272
62. Liu F, Zhang X, Qi H, Wang J, Wang M, Zhang Y, et al. Correlation of serum magnesium with cardiovascular risk factors in maintenance hemodialysis patients–a cross-sectional study. Magnes Res. (2013) 26(3):100–8. doi: 10.1684/mrh.2013.0344
63. Arase H, Yamada S, Yotsueda R, Taniguchi M, Yoshida H, Tokumoto M, et al. Modified creatinine index and risk for cardiovascular events and all-cause mortality in patients undergoing hemodialysis: the Q-cohort study. Atherosclerosis. (2018) 275:115–23. doi: 10.1016/j.atherosclerosis.2018.06.001
64. Xu M, Huang Y, Xie L, Peng K, Ding L, Lin L, et al. Diabetes and risk of arterial stiffness: a Mendelian randomization analysis. Diabetes. (2016) 65(6):1731–40. doi: 10.2337/db15-1533
65. Drake I, Fryk E, Strindberg L, Lundqvist A, Rosengren AH, Groop L, et al. The role of circulating galectin-1 in type 2 diabetes and chronic kidney disease: evidence from cross-sectional, longitudinal and Mendelian randomisation analyses. Diabetologia. (2022) 65(1):128–39. doi: 10.1007/s00125-021-05594-1
66. Hu XT, Rong S, Wang Q, Sun TP, Bao W, Chen LK, et al. Association between plasma uric acid and insulin resistance in type 2 diabetes: a Mendelian randomization analysis. Diabetes Res Clin Pract. (2021) 171:9. doi: 10.1016/j.diabres.2020.108542
67. Keerman M, Yang F, Hu H, Wang J, Wang F, Li ZY, et al. Mendelian randomization study of serum uric acid levels and diabetes risk: evidence from the Dongfeng-Tongji cohort. BMJ Open Diabetes Res Care. (2020) 8(1):10. doi: 10.1136/bmjdrc-2019-000834
68. Sluijs I, Holmes MV, van der Schouw YT, Beulens JWJ, Asselbergs FW, Huerta JM, et al. A Mendelian randomization study of circulating uric acid and type 2 diabetes. Diabetes. (2015) 64(8):3028–36. doi: 10.2337/db14-0742
69. Norris KC, Smoyer KE, Rolland C, Van der Vaart J, Grubb EB. Albuminuria, serum creatinine, and estimated glomerular filtration rate as predictors of cardio-renal outcomes in patients with type 2 diabetes mellitus and kidney disease: a systematic literature review. BMC Nephrol. (2018) 19:13. doi: 10.1186/s12882-018-0821-9
70. Katsiki N, Dimitriadis GD, Mikhailidis DP. Serum uric acid and diabetes: from pathophysiology to cardiovascular disease. Curr Pharm Des. (2021) 27(16):1941–51. doi: 10.2174/1381612827666210104124320
71. Karumanchi P, Fareed J, Bansal V, Siddiqui F, Hoppensteadt D, Kantarcioglu B. Thrombo-inflammatory biomarkers of cardiorenal syndrome in patients undergoing maintenance hemodialysis in end stage renal disease. Am J Hematol. (2023) 98:E54–5. doi: 10.1093/ndt/gfad063c_2724
Keywords: end-stage renal disease, hemodialysis, cardiovascular disease, causal factors, diabetes
Citation: Tian D, Xu Y, Wang Y, Zhu X, Huang C, Liu M, Li P and Li X (2024) Causal factors of cardiovascular disease in end-stage renal disease with maintenance hemodialysis: a longitudinal and Mendelian randomization study. Front. Cardiovasc. Med. 11: 1306159. doi: 10.3389/fcvm.2024.1306159
Received: 3 October 2023; Accepted: 8 July 2024;
Published: 18 July 2024.
Edited by:
Pietro Enea Lazzerini, University of Siena, ItalyReviewed by:
Valdo Jose Dias Da Silva, Universidade Federal do Triângulo Mineiro, BrazilLingyun Zu, Peking University Third Hospital, China
© 2024 Tian, Xu, Wang, Zhu, Huang, Liu, Li and Li. This is an open-access article distributed under the terms of the Creative Commons Attribution License (CC BY). The use, distribution or reproduction in other forums is permitted, provided the original author(s) and the copyright owner(s) are credited and that the original publication in this journal is cited, in accordance with accepted academic practice. No use, distribution or reproduction is permitted which does not comply with these terms.
*Correspondence: Min Liu, bGl1bWluMTM2QDEyNi5jb20=; Panlong Li, MTMwMTE4NTQwOTBAMTYzLmNvbQ==; Xiangyong Li, bHh5MjAwNTEyM0AxMjYuY29t
†These authors have contributed equally to this work and share first authorship