- 1Department of Cardiology, Shigatse People’s Hospital, Tibet, China
- 2Department of Cardiology, Shanghai Tenth People’s Hospital, School of Medicine, Tongji University, Shanghai, China
Background: High-altitude pulmonary hypertension (HAPH) is a common disease in regions of high altitude where performing right heart catheterization (RHC) is challenging. The development of a diagnostic scoring system is crucial for effective disease screening.
Methods: A total of 148 individuals were included in a retrospective analysis, and an additional 42 residents were prospectively enrolled. We conducted a multivariable analysis to identify independent predictors of HAPH. Subsequently, we devised a prediction score based on the retrospective training set to anticipate the occurrence and severity of HAPH. This scoring system was further subjected to validation in the prospective cohort, in which all participants underwent RHC.
Results: This scoring system, referred to as the GENTH score model (Glycated hemoglobin [OR = 4.5], Echocardiography sign [OR = 9.1], New York Heart Association-functional class [OR = 12.5], Total bilirubin [OR = 3.3], and Hematocrit [OR = 3.6]), incorporated five independent risk factors and demonstrated strong predictive accuracy. In the training set, the area under the curve (AUC) values for predicting the occurrence and severity of HAPH were 0.851 and 0.832, respectively, while in the validation set, they were 0.841 and 0.893. In the validation set, GENTH score model cutoff values of ≤18 or >18 points were established for excluding or confirming HAPH, and a threshold of >30 points indicated severe HAPH.
Conclusions: The GENTH score model, combining laboratory and echocardiography indicators, represents an effective tool for distinguishing potential HAPH patients and identifying those with severe HAPH. This scoring system improves the clinical screening of HAPH diseases and offers valuable insights into disease diagnosis and management.
Introduction
Pulmonary hypertension (PH) is a multifaceted pathophysiological disorder associated with a spectrum of clinical conditions. It is defined as mean pulmonary arterial pressure (mPAP) >20 mmHg at rest, as determined through right heart catheterization (RHC) (1). The primary underlying pathophysiological process of PH is pulmonary vascular remodeling (2), a phenomenon that can ultimately result in right heart failure (3).
High-altitude pulmonary hypertension (HAPH) is classified as group 3 PH, primarily attributed to prolonged exposure to high-altitude environments (4, 5). These high-altitude regions are typically 2,500 m above sea level (6), inhabited by over 140 million permanent residents, with an additional 40 million temporary visitors (7). The living conditions between low-altitude plains (<1,000 m) and high-altitude areas (>2,500 m) (8) differ significantly, giving rise to various health challenges. The notable factors affecting the health of individuals include hypoxia and hypobaric conditions, resulting in hypoxemia, symptomatic excessive erythrocytosis, and elevated mPAP (9). The diagnostic criteria for HAPH typically involve mPAP measurements obtained through right heart catheterization (mPAPRHC), with mPAPRHC >30 mmHg, as defined by the Qinghai criteria established by the International Society for Mountain Medicine (ISMM) in 2005 (5, 10). However, owing to limited equipment and technological resources, RHC, the gold standard for HAPH diagnosis, is rarely conducted in high-altitude regions (5). Consequently, the diagnosis of HAPH often relies on imaging techniques, particularly echocardiography, which may be imprecise in predicting mPAP compared with RHC due to the high variability inherent in the spectrum of right heart dysfunction (11) and the potential impact of observer-dependent factors (12).
Previous research has confirmed that HAPH patients also often have polycythemia and hypoxemia, as well as structural and functional alterations in the right heart (13). However, the precise independent risk factors remain undetermined, and a comprehensive scoring system to aid in diagnosis has yet to be devised. Therefore, there is an urgent requirement to develop a dedicated scoring system that amalgamates clinical characteristics with echocardiography data to improve the early diagnosis and management of HAPH in high-altitude regions.
Methods
Study design and population
The study received ethical approval from the Shigatse People's Hospital (altitude 4,040 m) (14) institutional review board, and informed consent was obtained from all enrolled patients. As ethnicity influences the responses to chronic hypoxia exposure, the study was limited to Tibetans and excluded other ethnicities and residents who had migrated to the region. Blood samples were consistently collected at 7 am in a fasting state. In the retrospective training set, the inclusion criteria were as follows: (1) male and females aged 18–85; (2) admission to Shigatse People's Hospital between August 2020 and August 2022; (3) a documented history of residing in high-altitude areas for more than 20 years; (4) an initial diagnosis suggesting PH; and (5) performance of CTA during hospitalization. The exclusion criteria for the retrospective training set included: (1) individuals with contraindications for undergoing CTA or indications of chronic thromboembolic disease in CTA; (2) PaO2 of ≥83 mmHg; (3) a documented history of left heart disease or echocardiography signs thereof, which include diastolic dysfunction, systolic dysfunction, and valvular diseases; (4) excessive erythrocytosis (defined as Hb of ≥19 g/dl for females and ≥21 g/dl for males); (5) the presence of severe hepatic or renal insufficiency; (6) pregnancy (for a detailed process of patient selection, please refer to Supplementary Figure 1).
For the prospective cohort, the inclusion criteria were further expanded as follows: (1) individuals admitted to Shigatse People's Hospital between August 2022 and August 2023; (2) no contraindications for undergoing RHC; and (3) provision of informed consent. Furthermore, echocardiography, CTA, and RHC (prospective cohort) were performed before medical therapy within 1 week after the first fasting blood. The chronological sequence of echocardiography, CTA, and RHC, along with the detailed process of patient selection, is presented in Supplementary Figure 2. Body surface area (BSA) and oxygen consumption (VO2) were estimated using established formulas (15). As per the Qinghai criteria, individuals were diagnosed with HAPH when mPAP was >30 mmHg (10). As patients with an mPAP >60 mmHg had a higher 30-day mortality rate (16), moderate and severe HAPH were defined as an mPAP of 30–60 mmHg and >60 mmHg, respectively (17, 18).
Echocardiography
Transthoracic echocardiography was conducted in accordance with the guidelines provided by the American Society of Echocardiography (19). The following parameters were measured: vertical and transverse diameters of the left and right atrium and ventricle, main pulmonary artery diameter, and left ventricle ejection fraction (LVEF) (19). It is worth mentioning that the parameters of the right atrium and ventricle were measured using a right ventricle-focused apical four-chamber view (A4C), which was based on the A4C by adjusting the transducer to maximize the right ventricle visualized area. The main pulmonary artery diameter was measured using a pulmonic valve (PV)-focused parasternal short-axis (PSAX) view (20). To estimate the pressure gradient between the right atrium and right ventricle, the modified Bernoulli equation was applied, relying on the tricuspid regurgitation pressure gradient (TRPG) (21). Additionally, the right atrial pressure was evaluated and, in combination with TRPG, used to estimate pulmonary arterial systolic pressure (PASP) (22).
Computed tomography angiography (CTA) acquisition
CTA scans were acquired using United Imaging scanners, with patients in a supine position and instructed to hold their breath during inspiration. The imaging parameters were set as follows: a tube voltage of 120 kVp, tube current of 300 mA, tube rotation time of 0.3 s, and collimator width of 64 mm × 0.625 mm. An iodinated contrast agent (Lomeprol, Bracco Sine, China, 400 mg/ml) was intravenously administered via a double-syringe power injector through the median cubital vein. The intelligent tracking mode was employed, and a 60 ml contrast agent was infused at a rate of 4 ml/s. When the density of the main pulmonary artery reached the predefined peak of 80 Hounsfield Units (HU), the scanning was triggered (23). On the four-chambered transverse view, measurements were taken for the atrial diameter, left lower bronchus, and arterial diameter. The left lower artery-bronchus ratio (ABR) was computed at the junction of the left main bronchus. The left lower ABR and the ratio of right to left atrial diameter (rRLA) were integrated into the prediction model described in previous publications. The model for calculating mPAPPredicted was as follows: mPAPpredicted = −34 + 40 × left lower ABR + 7 × rRLA (r = 0.907, R2 = 0.823, p < 0.0001). This model demonstrated a strong fit and served as a reliable alternative to RHC for assessing mPAP (24, 25).
Right heart catheterization and pulmonary angiography
In the prospective validation cohort, RHC was conducted following standard procedures by an interventional cardiologist with over 10 years of experience (1). Using local anesthesia, an introducer sheath was positioned in the right femoral vein, and a JR 4.0 catheter was advanced into the pulmonary artery to measure mPAP (mPAPRHC). mPAPRHC was recorded in the main pulmonary artery, just above the pulmonary valve. Subsequently, systolic and diastolic pressures of the right ventricle, right atrium, and superior vena cava were measured through standard image analysis techniques (26). To enhance the visualization of the pulmonary vessels. a contrast medium was injected into the openings of the left and right upper and lower pulmonary arteries (26).
Risk factor screening and transformation
Following difference and correlation analyses, the parameters transformed dichotomous variables and then incorporated them into a multivariate logistics regression. The upper and lower limits for these dichotomous variables were determined based on the calibration range of the laboratory instruments at Shigatse People's Hospital (27, 28), as well as the criteria outlined in the guidelines for hyperbilirubinemia (29), hyperuricemia (30), and diabetes (31). In line with recent updates to guidelines, in which TRPG and PASP from echocardiography have been replaced by other relevant echocardiographic signs that contribute to the assessment of the probability of PH (1, 32, 33). We defined echocardiographic signs indicative of right heart dysfunction in this study as follows: (1) vertical and transverse enlargement of the right atrium; (2) vertical and transverse enlargement of the right ventricle; and (3) dilation of the main pulmonary artery. These three indicators were collectively referred to as “Echocardiography signs” within the GENTH score model. A prediction score system, termed the GENTH score model, was devised to predict the occurrence and severity of HAPH. The GENTH score model comprises five critical risk factors: Glycated hemoglobin, Echocardiography sign, New York Heart Association-functional class (NYHA-FC), Total bilirubin, and Hematocrit.
Statistical analysis
Characteristics of the training and validation patients were described by mPAPPredicted and mPAPRHC, respectively. The normal distribution of measurements was assessed using Kolmogorov–Smirnov's test and Shapiro–Wilk's test. Normally distributed data, non-normally distributed data, and categorical data are presented as mean ± standard deviation, median (the first quantile and the third quantile), and frequency, respectively. Group differences were analyzed as appropriate using one-way ANOVA, a Kruskal–Wallis H-test, or chi-square test. The trends in continuous variables or rates of dichotomous variables were assessed through linear regression analysis and Cochran-Armitage, respectively. Lasso regression was used to screen for the risk factors. Spearman's correlation was employed to examine the relationship between parameters and mPAPPredicted. Univariate and multivariate logistic regression analyses were conducted to calculate odds ratios (ORs) and 95% confidence intervals (CIs). Variables that remained statistically significant after the multivariate logistic analysis were considered candidates for the development of a novel scoring model, and the relevant points were determined based on the OR values, which reflect their impact on disease diagnosis. The receiver operating characteristic (ROC) curve was generated, and the area under the ROC curve (AUC) was calculated to evaluate the predictive performances of the scoring model. A nomogram was used to visualize the scoring model based on multivariate logistic regression analysis. The C-index was calculated to assess the predictive performances of the scoring models. Cutoff values were calculated to achieve a specificity of at least 95%. All statistical analyses were performed using R v.4.1.2 (R Foundation for Statistical Computing, Vienna, Austria) and SPSS v.26.0 (SPSS Inc. Chicago, Illinois, USA). A two-sided p < 0.05 was considered statistically significant.
Results
Baseline characteristics of individuals in the training set
In the training group, all participants underwent pulmonary CTA to gather data on rRLA and left lower ABR, enabling the calculation of mPAPPredicted. The average mPAPPredicted was 47 mmHg, ranging from 14 to 113 mmHg. There were no significant differences among the groups with respect to gender, age, or living altitude. However, patients with HAPH were more likely to experience cardiac dysfunction and had higher NYHA-FC grades. Laboratory tests revealed that HAPH patients often exhibited polycythemia, as indicated by elevated levels of erythrocytes, hemoglobin, and hematocrit (p for trend <0.05). In comparison with healthy highlanders (HH), HAPH patients showed elevated transaminase, uric acid, and total bilirubin, and a prolonged prothrombin time (p for trend <0.05). Blood gas analysis unveiled that HAPH patients frequently had metabolic alkalosis, characterized by increased PaCO2 and base excess. Moreover, HAPH patients had lower total cholesterol and triglyceride levels, and higher glycated hemoglobin levels, implying the influence of hypoxia and the low atmospheric pressure environment on lipid and glucose metabolism in high-altitude regions (Table 1). Notably, there were no significant differences among the groups in terms of vital signs, height, weight, and metabolic indicators such as BMI, BSA, and VO2 as well as myocardial enzymes (Supplementary Table 1). Additional information regarding the between-group differences and 95% CIs for clinical characteristics is provided in Supplementary Table 2.
Echocardiography characteristics in the training set
The representative imaging for parameters of the right atrium and ventricle is shown in Figure 1. Patients with abnormal mPAPPredicted were more likely to exhibit several echocardiographic abnormalities, including an enlarged right atrium and ventricle in both transverse and vertical dimensions (p for trend <0.01), a dilated main pulmonary artery (p for trend <0.01), and an elevated TRPG and PASP (p for trend <0.05). However, there were no significant differences in left heart diameter and LVEF among the three groups with varying mPAPPredicted. These findings indicated that HAPH patients are more likely to display signs of right heart dysfunction, as evidenced by echocardiography (p for trend <0.05; illustrated in Table 2). Further details regarding the between-group differences and 95% CIs for echocardiography characteristics are provided in Supplementary Table 3.
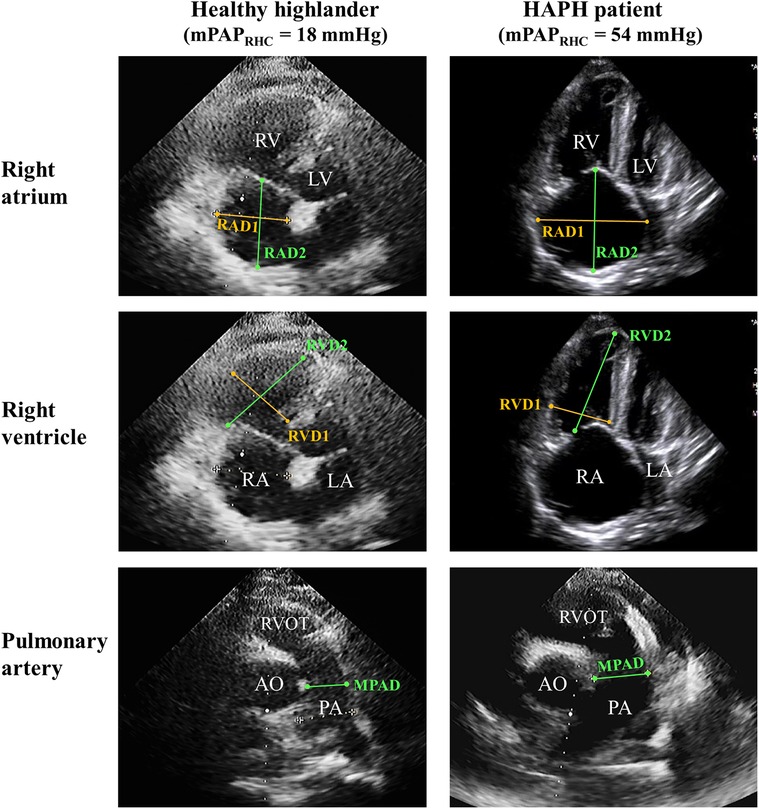
Figure 1. Representative imaging of the echocardiographic sign of right heart dysfunction. Right ventricle-focused apical four-chamber view and pulmonic valve-focused parasternal short-axis view of HH and HAPH patients. PA, pulmonary artery; RAD1, transverse diameter of right atrium; RAD2, vertical diameter of right atrium; RVD1, transverse diameter of right ventricle; RVD2, vertical diameter of right ventricle.
Independent risk factors associated with HAPH
The parameters, including basic characteristics and echocardiography signs, were considered for potential predictive variables. Lasso regression was used to screen parameters, and the 10-fold cross-validation method was applied to the iterative analysis (Figure 2). The screened variables included age, gender, NYHA-FC, hemoglobin, hematocrit, total bilirubin, uric acid, prothrombin time, echocardiography sign, glycated hemoglobin, PaCO2, and BE. Correlation analyses revealed positive correlations of mPAPPredicted with NYHA-FC (r = 0.391, p < 0.001), hematocrit (r = 0.322, p < 0.001), uric acid (r = 0.323, p < 0.001), glycated hemoglobin (r = 0.500, p < 0.001), echocardiography sign (r = 0.411, p < 0.001), total bilirubin (r = 0.285, p < 0.001), and prothrombin time (r = 0.285, p = 0.001) (Table 3). Variables with a p ≤ 0.001 were transformed into dichotomous variables and subsequently compared between HH and HAPH, as well as between moderate and severe HAPH, respectively (Supplementary Table 4). These variables were then included in the subsequent logistic regression analysis.
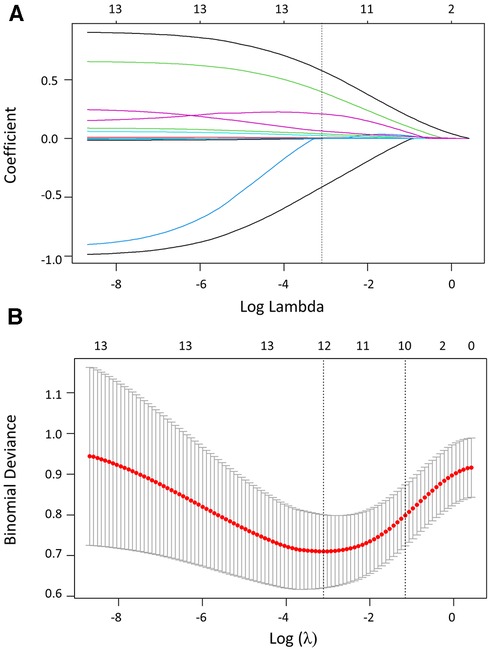
Figure 2. Variable screening based on lasso regression. (A) The variation characteristics of the coefficient of variables. (B) The selection process of the optimum value of the parameter log (λ) in the Lasso regression model using the cross-validation method.
Construction of a novel prediction model of HAPH and severe HAPH
The results of the multivariate logistic regression analysis unveiled a set of independent risk factors associated with the diagnosis of HAPH compared with HH (p < 0.05). These factors included an NYHA-FC ≥ Grade III (OR: 4.1, p = 0.021), hematocrit of >45% (OR: 3.6, p = 0.045), glycated hemoglobin of >6% (OR: 4.5, p = 0.039), and an echocardiography sign of ≥2 points (OR: 9.1, p = 0.001). Further stratification of HAPH patients into moderate HAPH (30 mmHg < mPAP ≤ 60 mmHg) and severe HAPH (mPAP > 60 mmHg) identified additional independent risk factors for severe HAPH through multivariate logistic regression analysis, including an NYHA-FC ≥ Grade III (OR: 12.5, p = 0.004), hematocrit of >45% (OR: 3.2, p = 0.028), total bilirubin of >34 μmol/L (OR: 3.3, p = 0.037), and glycated hemoglobin of >6% (OR: 3.8, p = 0.012) (Table 4). The results of the univariate logistic regression are shown in Supplementary Table 5.
To facilitate clinical application and assessment, a novel prediction model for HAPH diagnosis and severity classification, named the GENTH score model, was developed based on the OR values from multivariate logistic analysis. The GENTH scoring system incorporated the results of OR values in both multivariate logistics regressions, which could predict HAPH and its severity at the same time to improve convenience. Based on the independent predictors of HH vs. HAPH, the score of the highest proportional predictor (NYHA-FC) of moderate vs. severe HAPH was adjusted according to the OR = 12.5. Then, total bilirubin, the new predictor of moderate vs. severe HAPH was also added to the scoring system with its OR = 3.3. Each independent predictor of HAPH was assigned a weighted point value, with glycated hemoglobin receiving 5 points, echocardiography signs 9 points, NYHA-FC 13 points, total bilirubin 3 points, and hematocrit 4 points. This scoring system yielded a total score ranging from 0 to 34. A detailed explanation of the GENTH score model calculation is presented in Table 5.
The predictive and cutoff value of the GENTH score model
The baseline characteristics and echocardiography sign of the validation set are presented in Supplementary Tables 6, 7. There was a high correlation between mPAPRHC and mPAPPredicted (r = 0.876, p < 0.001) (Supplementary Table 8). To facilitate the clinical service, the nomogram was used to visualize the GENTH scoring system, and the parameters of the model were derived from the results of multivariate logistic regression analysis as mentioned above (Figures 3A–B). The C-index of the GENTH score model for predicting HAPH was 0.878 (95% CI: 0.793–0.881) in internal validation and 0.959 (95% CI: 0.884–0.963) in external validation. As for predicting severe HAPH, the C-index was 0.853 (95% CI: 0.767–0.56) in internal validation and 0.914 (95% CI: 0.802–0.918) in external validation. To assess the predictive performance of the GENTH scoring model, ROC curve analysis was conducted, and AUC values were calculated to evaluate the consistency of mPAP and the GENTH score model when compared with echocardiography-derived PASP (Figures 3C–D). In the validation cohort, the AUC values for the GENTH score model in diagnosing HAPH and severe HAPH were 0.835 and 0.848, respectively. These results were higher than the AUCs obtained for echocardiography PASP, which were 0.771 for HAPH and 0.724 for severe HAPH. The GENTH score model cutoff values for diagnosing HAPH (mPAP of >30 mmHg) and severe HAPH (mPAP of >60 mmHg) with a specificity of ≥95% were determined to be >18 and >30 points, respectively. The corresponding sensitivity, specificity, positive predictive value (PPV), and negative predictive value (NPV) are presented in Table 6.
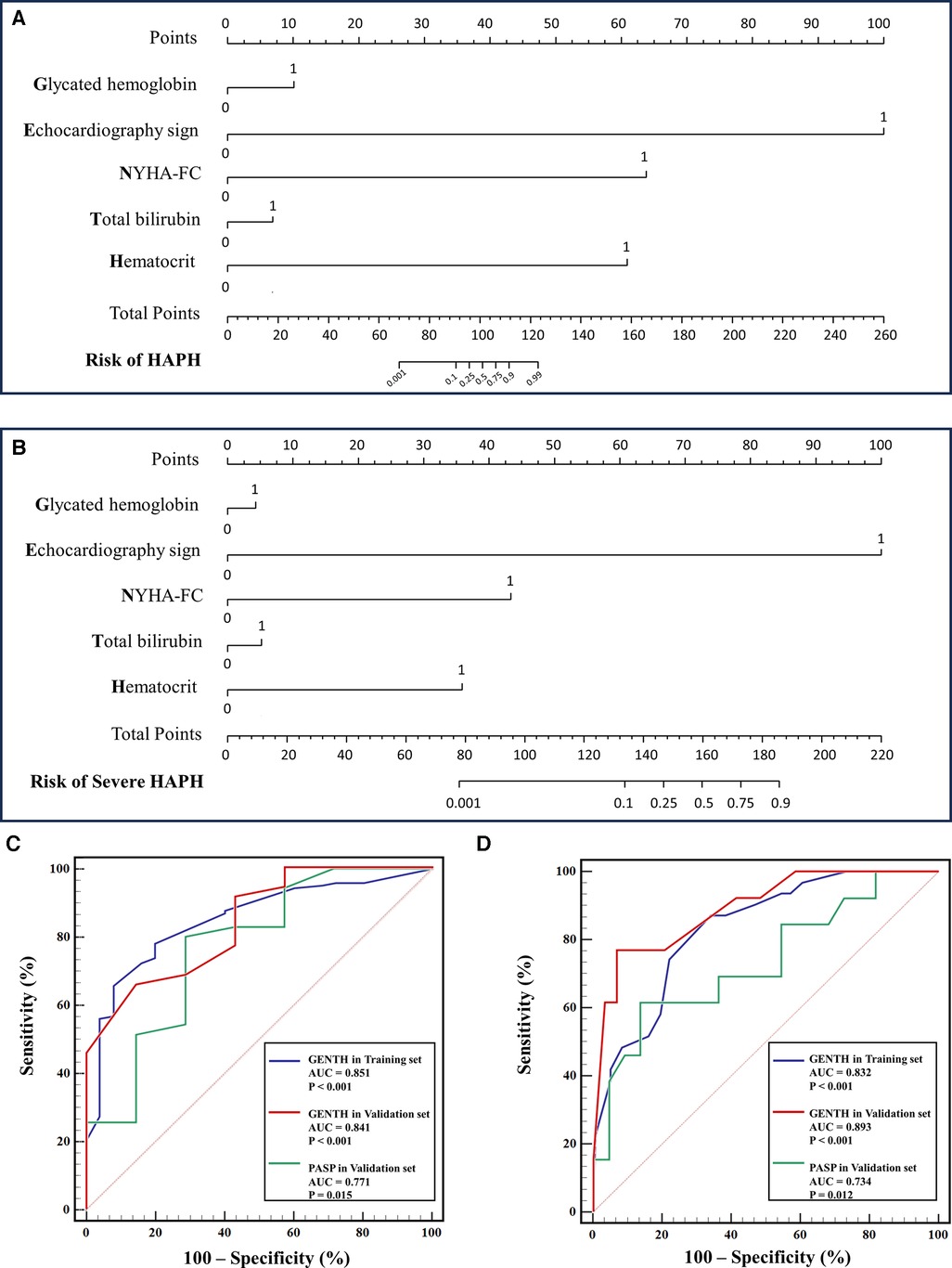
Figure 3. Nomogram and ROC curve of the GENTH score in the diagnosis and severity of HAPH. (A) Nomogram for the GENTH scoring system predicting HAPH in the validation set. (B) Nomogram for the GENTH scoring system predicting severe HAPH in the validation set. (C) ROC of the GENTH scoring system and PASP predicting HAPH in the training and validation sets. (D) ROC of the GENTH scoring system and PASP predicting severe HAPH in the training and validation sets.
Figure 4A illustrates the parameters from CTA in patients with different GENTH scores in the validation set. When compared with HH, HAPH patients were more likely to exhibit an enlarged right atrium, main pulmonary artery, and left pulmonary artery. Additionally, the ratios based on these parameters, such as rRLA, rPA, and left lower ABR, were increased. Subsequent RHC and pulmonary angiography were performed to confirm the diagnosis. In comparison with HH, HAPH patients with a GENTH score of >18 demonstrated enlarged, increased, and distorted arterial branches with rough intima, particularly noticeable in the lower pulmonary artery. This phenomenon was more pronounced in patients with a GENTH score of >30, which corresponds to higher mPAP values measured via RHC (Figure 4B).
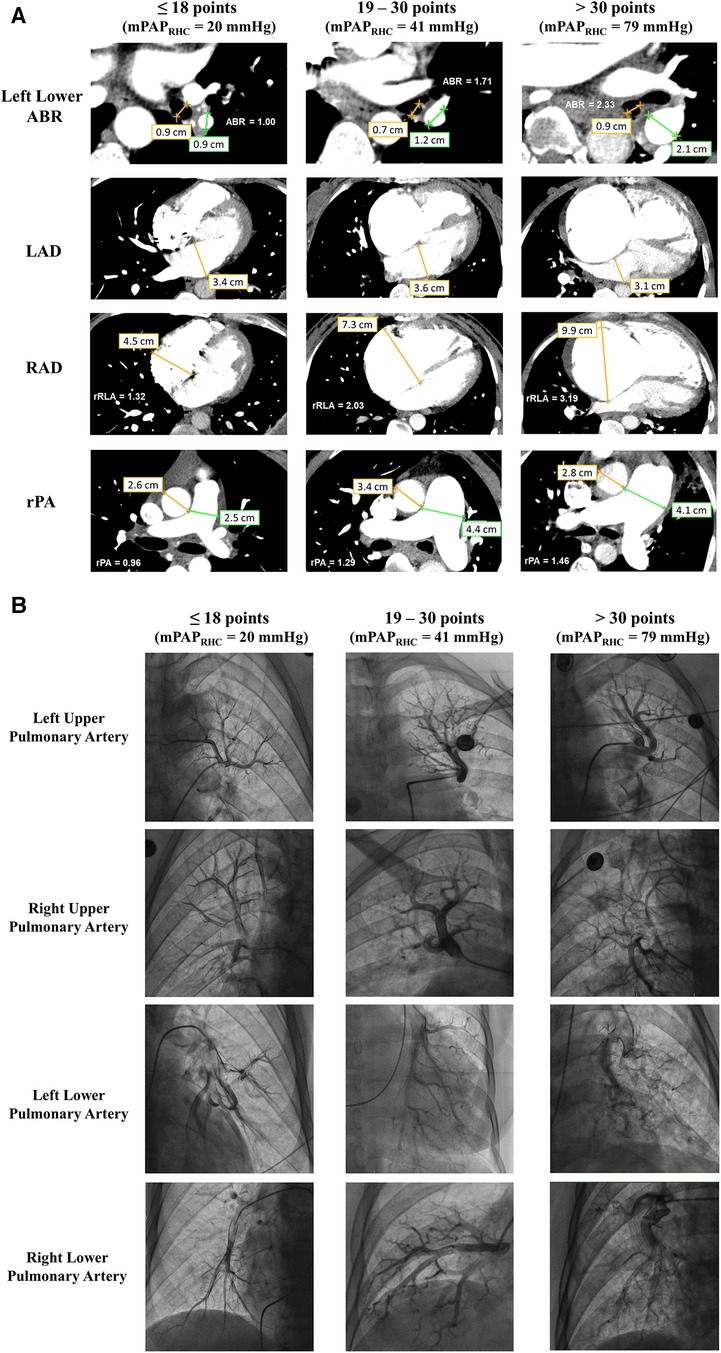
Figure 4. Imaging features of CTA and pulmonary arteriography in the GENTH score. (A) The diameter of the left lower pulmonary artery and bronchus, right and left atria, aorta, and main pulmonary artery in patients with different GENTH scores in the validation set. (B) Imaging features of pulmonary arteriography in patients with different GENTH scores in the validation set. ABR, pulmonary artery-bronchus ratio; LAD, left atrium diameter; RAD, right atrium diameter; rPA, ratio of the main pulmonary artery to aorta diameter; rRLA, ratio of the right to left atrial diameter.
Discussion
Our study places particular emphasis on HAPH, a subset of PH that has generated relatively less global attention and faces challenges in terms of diagnosis and treatment, especially in high-altitude regions (5). We have introduced the GENTH score model, which is the first clinical prediction scoring system designed for both diagnosing HAPH and categorizing its severity. This scoring system has undergone validation in a prospective cohort, marking a significant step toward improved management of this condition.
The study commenced with a baseline analysis aimed at the early screening of risk factors in HAPH. Alterations in laboratory markers among HAPH patients include erythrocytosis and CO2 accumulation, which are likely linked to compensatory physiological responses developed because of prolonged chronic hypoxia (17). Moreover, compared with HH, HAPH patients exhibit variations in prothrombin time and metabolic indicators, reflecting disruptions in lipid and cholesterol homeostasis, macronutrient metabolism, and the coagulation function of the liver (34). These findings suggest that the metabolic changes observed in HAPH patients may influence liver function through three potential mechanisms. First, the hypoxia-inducible factor (HIF) family is activated during cellular adaptation to hypoxic stress (35). HIF-1 and HIF-2α are upregulated in hepatocytes under hypoxic conditions, impacting glucose metabolism (36) and leading to lipid accumulation within hepatocytes (37), respectively. Second, the liver plays a critical role in blood volume regulation (38), and it is not uncommon to observe hyperbilirubinemia in long-standing PH, possibly arising from subsequent liver congestion associated with polycythemia (39). Moreover, HAPH patients often develop right ventricular failure, which can lead to liver congestion (40) and subsequently elevate myocardial markers and transaminases (41). The metabolic changes in HAPH patients may be attributed to chronic hypoxia, an increase in blood volume, and right ventricular dysfunction. It is noteworthy that compared with HH, HAPH patients typically have lower total cholesterol and triglyceride levels. This difference can be linked to hepatocyte lipid accumulation (42) and alterations in peripheral lipid metabolism under hypoxic conditions (43). These factors may potentially contribute to the decreased incidence of obesity in high-altitude regions (4, 44). Moreover, the prevalence of pulmonary embolism in the retrospective cohort was high at 21% (52/248). Previous studies reported that high altitude was the risk factor for pulmonary embolism, and the potential mechanisms may be related to activated coagulation and increased inflammation under hypoxic stimulation (45). In addition, the prevalence of pulmonary embolism was higher in patients with polycythemia (39%) than in those without polycythemia (11%) (46). Therefore, this phenomenon may result from secondary polycythemia and hypoxia-activated coagulation and inflammation. Furthermore, as we excluded patients without CTA in this research, which was more commonly performed in patients with suspected pulmonary embolism, this ratio may be biased.
The selected predictors of the GENTH score model were closely associated with various aspects, including blood volume, cardiac function, cardiac structural changes, and metabolism. The treatment and prognosis of PH are closely related to the recovery of left or right heart function (47). Notably, right ventricular functional recovery (RVFnRec) has emerged as a novel endpoint in the successful treatment of pulmonary hypertension (48). This underscores the pivotal role played by echocardiography and cardiac function classification in the predictive accuracy of the GENTH score model. The echocardiography sign of right heart dysfunction was assigned with the highest points for HAPH, highlighting the importance of detecting right heart structural changes (13) as a potential early indicator for HAPH. In the case of severe HAPH, we often observe pulmonary vascular remodeling (49) and cardiac dysfunction (3), manifested through worsening clinical symptoms and elevated NYHA-FC grades. Therefore, echocardiography signs were excluded from the independent predictors, reflecting the compensatory and potentially reversible dilatation of the right heart and pulmonary artery in the early stages (50). During this phase, the proportion of NYHA-FC elevated. Moreover, previous studies have reported that the initial mPAP of HAPH patients tends to be higher than that of PH patients in low-altitude regions (17), despite the absence of pronounced clinical symptoms in the early stages (51). Therefore, it is important for clinicians to prioritize the early detection of echocardiographic structural changes in the right heart, particularly when assessing patients with poor cardiac function at the initial diagnosis.
In addition to echocardiography and NYHA-FC, the other variables incorporated into the GENTH score model have also been extensively reported. Hematocrit, in particular, has been found to hold more clinical significance than hemoglobin (5) and serves as a valuable indicator for evaluating pulmonary vascular resistance (PVR) in high-altitude regions (52). Moreover, erythrocytosis and hepatic congestion, which reflect elevated blood volume and right heart dysfunction, often result in increased bilirubin levels (39). Additionally, previous studies have suggested that long-term residence in high-altitude regions contributes to increased glycated hemoglobin, which may be linked to hypoxia-inducible factor activation (36) and alterations in gluconeogenesis and glycogenesis influenced by activated neutrophils under altitude-induced hypoxia (53). Therefore, the GENTH score model effectively encapsulates the diverse changes in HAPH patients and proves to be a valuable tool for screening.
In our previous study, we used CTA to predict mPAPRHC, which improved the convenience of evaluating HAPH. However, CTA involves the use of a contrast agent, which belongs to large-scale examination (24). The current study refines the process for diagnosing and staging HAPH, improving screening effectiveness. We selected the cutoff value from the validation set with RHC as the standard for stratification, and further evaluation was guided by the GENTH score model. Specifically, we recommended more comprehensive echocardiography for potential HAPH patients with a GENTH score ranging from 18 to 30 points. Subsequently, the decision for RHC or follow-up should be based on the detailed echocardiographic report. RHC and intervention were strongly recommended for patients with a high suspicion of severe HAPH, indicated by a GENTH score of >30 points (Central Illustration). It is worthing mention that as the average altitude of the Shigatse area in Tibet is above 4,000 m, there was no influence of altitude variation and low altitude exposure. However, it has been reported that lower altitude exposure impacted highlanders and high-altitude hypoxia, thus the application of the GENTH scoring system may be limited in hospitals at lower altitudes (54).
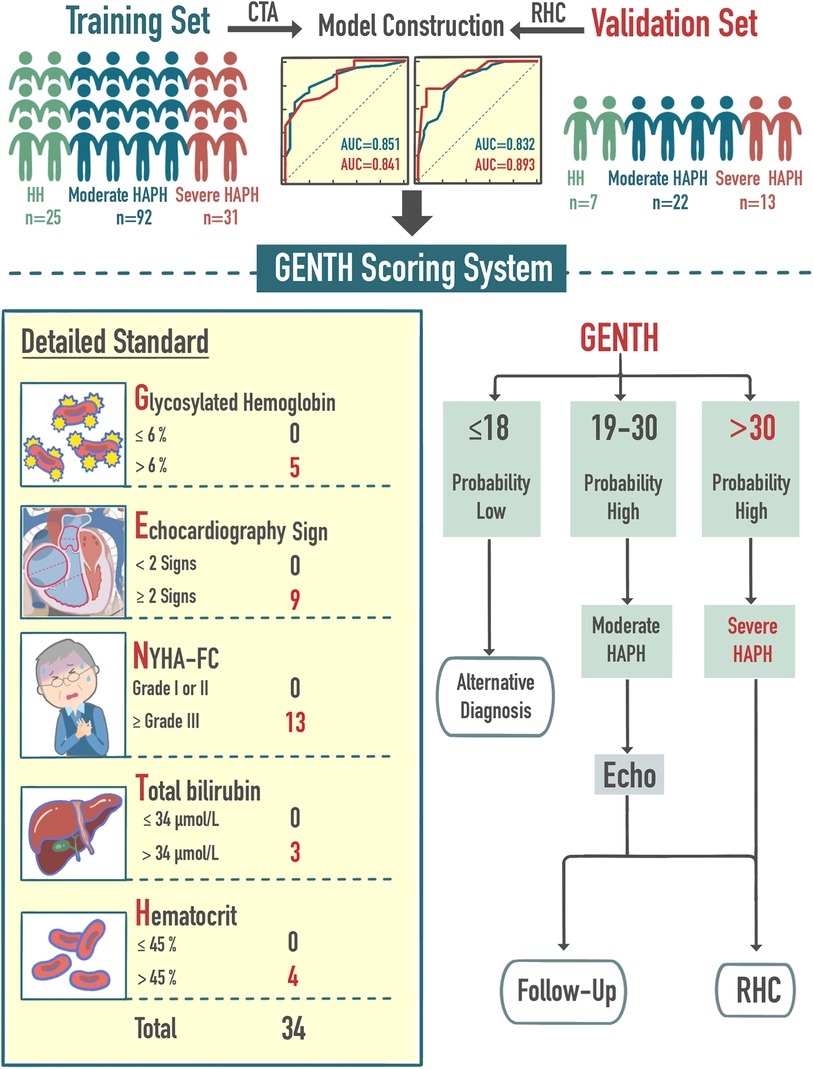
Central Illustration. Brief model construction process and clinical application. The GENTH score was based on five risk factors: NYHA-FC, hematocrit, glycated hemoglobin, echocardiography sign, and total bilirubin. The GENTH score assisted the initial diagnosis and severity of HAPH and further guided the subsequent diagnosis and follow-up.
This study also had limitations. The development of clinical scoring standards requires an adequate sample size, which was challenging to achieve in our case. RHC data was lacking in the training group due to the difficulties associated with performing this procedure in high-altitude regions with limited medical resources. In addition, the presence of right heart dysfunction in HAPH patients (3) significantly impacts the accuracy of mPAP evaluation via echocardiography (11). Therefore, we employed a combined approach using limited RHC data and CTA to construct a predictive model for HAPH patients in the Shigatse People's Hospital (24), which was further applied to this retrospective large sample dataset for calculation and grouping. To further validate the accuracy of the GENTH scoring criteria, we conducted a prospective validation cohort that included RHC and pulmonary angiography, partially compensating for the limited sample size. Furthermore, lung function was not routinely performed in this research, so the exclusion of chronic obstructive pulmonary disease (COPD) may have been partially missed. The echocardiography only reported the parameters of the heart chamber and valvular regurgitation and did not assess the complete right heart function. More complete echocardiography parameters may be included to improve the accuracy of the model in the future. It is important to recognize that this study focused on an Asian population, and it is unclear whether the GENTH scoring model can be applied to other populations. However, previous studies in the Aksay and Andean highlanders have reported similar risk factors (4, 18). Thus, the GENTH scoring model may have predictive value in other high-altitude regions, but the relative weighted point value of these risk factors may differ. We look forward to its prediction results in other high-altitude regions.
Conclusion
In this study, we introduced the GENTH score model, the first clinical prediction scoring system designed for both HAPH diagnosis and severity classification. The reliability and effectiveness of this scoring system were rigorously validated in a prospective cohort that employed RHC as the gold standard for diagnosing HAPH. The scoring system provided essential insights into the clinical characteristics associated with various disease stages, effectively addressing the limitations of echocardiography and ultimately improving the accuracy and efficiency of both HAPH diagnosis and severity classification in high-altitude regions.
Data availability statement
The raw data supporting the conclusions of this article will be made available by the authors, without undue reservation.
Ethics statement
The studies involving humans were approved by Shigatse People's Hospital institutional review board. The studies were conducted in accordance with the local legislation and institutional requirements. The participants provided their written informed consent to participate in this study.
Author contributions
YZ: Data curation, Investigation, Methodology, Project administration, Validation, Conceptualization, Formal Analysis, Software, Supervision, Visualization, Writing – original draft. GZ: Investigation, Methodology, Project administration, Software, Validation, Visualization, Writing – original draft. BL: Software, Writing – review & editing, Investigation, Resources. QY: Project administration, Funding acquisition, Software, Validation, Writing – review & editing. MN: Data curation, Methodology, Project administration, Writing – original draft, Resources. NM: Writing – original draft, Data curation, Methodology, Project administration, Supervision. MM: Investigation, Resources, Validation, Writing – original draft. DZ: Investigation, Resources, Validation, Writing – original draft. JZ: Funding acquisition, Project administration, Resources, Validation, Writing – review & editing, Data curation, Investigation, Methodology. WP: Conceptualization, Funding acquisition, Project administration, Resources, Validation, Writing – review & editing.
Funding
The author(s) declare financial support was received for the research, authorship, and/or publication of this article.
This study was funded by the Natural Science Foundation of Shigatse (RKZ2023ZR-015(Z)), Cardiovascular Multidisciplinary Integrated Research Fund of China International Medical Foundation (2022-N-01-7), and Clinical Research Plan of SHDC (SHDC22023223).
Acknowledgments
We thank Qiang Li (Department of Liver Diseases, Shanghai Public Health Clinical Center, Fudan University, Shanghai 201508, China) for assistance in model construction and revision and the patients who participated in this study.
Conflict of interest
The authors declare that the research was conducted in the absence of any commercial or financial relationships that could be construed as a potential conflict of interest.
Publisher's note
All claims expressed in this article are solely those of the authors and do not necessarily represent those of their affiliated organizations, or those of the publisher, the editors and the reviewers. Any product that may be evaluated in this article, or claim that may be made by its manufacturer, is not guaranteed or endorsed by the publisher.
Supplementary material
The Supplementary Material for this article can be found online at: https://www.frontiersin.org/articles/10.3389/fcvm.2023.1290895/full#supplementary-material
References
1. Humbert M, Kovacs G, Hoeper MM, Badagliacca R, Berger RMF, Brida M, et al. 2022 ESC/ERS guidelines for the diagnosis and treatment of pulmonary hypertension developed by the task force for the diagnosis and treatment of (ESC) and the European respiratory society (ERS). Eur Heart J. (2022):1–114. doi: 10.1093/eurheartj/ehac237
2. Hoeper MM, Badesch DB, Ghofrani HA, Gibbs JSR, Gomberg-Maitland M, McLaughlin VV, et al. Phase 3 trial of sotatercept for treatment of pulmonary arterial hypertension. N Engl J Med. (2023) 388:1478–90. doi: 10.1056/nejmoa2213558
3. Brener MI, Grayburn P, Lindenfeld JA, Burkhoff D, Liu M, Zhou Z, et al. Right ventricular–pulmonary arterial coupling in patients with HF secondary MR: analysis from the COAPT trial. JACC Cardiovasc Interv. (2021) 14:2231–42. doi: 10.1016/j.jcin.2021.07.047
4. Furian M, Latshang TD, Aeschbacher SS, Sheraliev U, Marazhapov NH, Mirrakhimov E, et al. Markers of cardiovascular risk and their reversibility with acute oxygen therapy in Kyrgyz highlanders with high altitude pulmonary hypertension. Pulmonology. (2021) 27:394–402. doi: 10.1016/j.pulmoe.2021.02.001
5. Naeije R. Pulmonary hypertension at high altitude. Eur Respir J. (2019) 53:3–5. doi: 10.1183/13993003.00985-2019
6. Bärtsch P, Swenson ER. Acute high-altitude illnesses. N Engl J Med. (2013) 368:2294–302. doi: 10.1056/NEJMCP1214870
7. Luks AM, Hackett PH. Medical conditions and high-altitude travel. N Engl J Med. (2022) 386:364–73. doi: 10.1056/nejmra2104829
8. Lei S, Sun Z, He X, Li C, Zhang Y, Luo X, et al. Clinical characteristics of pulmonary hypertension patients living in plain and high-altitude regions. Clin Respir J. (2019) 13:485–92. doi: 10.1111/CRJ.13049
9. Shah AM, Cikes M, Prasad N, Li G, Getchevski S, Claggett B, et al. Echocardiographic features of patients with heart failure and preserved left ventricular ejection fraction. J Am Coll Cardiol. (2019) 74:2858–73. doi: 10.1016/j.jacc.2019.09.063
10. León-Velarde F, Maggiorini M, Reeves JT, Aldashev A, Asmus I, Bernardi L, et al. Consensus statement on chronic and subacute high altitude diseases. High Alt Med Biol. (2005) 6:147–57. doi: 10.1089/HAM.2005.6.147
11. Finkelhor RS, Lewis SA, Pillai D. Limitations and strengths of Doppler/echo pulmonary artery systolic pressure-right heart catheterization correlations: a systematic literature review. Echocardiography. (2015) 32:10–8. doi: 10.1111/echo.12594
12. Fisher MR, Forfia PR, Chamera E, Housten-Harris T, Champion HC, Girgis RE, et al. Accuracy of Doppler echocardiography in the hemodynamic assessment of pulmonary hypertension. Am J Respir Crit Care Med. (2009) 179:615–21. doi: 10.1164/rccm.200811-1691OC
13. Brito J, Siques P, López R, Romero R, León-Velarde F, Flores K, et al. Long-term intermittent work at high altitude: right heart functional and morphological status and associated cardiometabolic factors. Front Physiol. (2018) 9:1–13. doi: 10.3389/fphys.2018.00248
14. Jiang T, Zhao L, Yin Y, Yu H, Li Q. Evaluation of middle ear function by tympanometry and the influence of lower barometric pressure at high altitude. High Alt Med Biol. (2021) 22:70–6. doi: 10.1089/ham.2020.0042
15. Tonelli AR, Wang XF, Abbay A, Zhang Q, Ramos J, McCarthy K. Can we better estimate resting oxygen consumption by incorporating arterial blood gases and spirometric determinations? Respir Care. (2015) 60:517–25. doi: 10.4187/RESPCARE.03555
16. Ahmad M, Del Cid Fratti J, Henien M, Pant K, Wattelet MP, Whorf D, et al. Association between pulmonary hypertension and its effect on 30-day mortality, readmission, and cost after transcatheter aortic valve replacement: a multicenter study. Cureus. (2023) 15:1–10. doi: 10.7759/cureus.40976
17. Villafuerte FC, Corante N. Chronic mountain sickness: clinical aspects, etiology, management, and treatment. High Alt Med Biol. (2016) 17:61–9. doi: 10.1089/HAM.2016.0031/ASSET/IMAGES/LARGE/FIGURE2.JPEG
18. León-Velarde F, Villafuerte FC, Richalet JP. Chronic mountain sickness and the heart. Prog Cardiovasc Dis. (2010) 52:540–9. doi: 10.1016/j.pcad.2010.02.012
19. Mitchell C, Rahko PS, Blauwet LA, Canaday B, Finstuen JA, Foster MC, et al. Guidelines for performing a comprehensive transthoracic echocardiographic examination in adults: recommendations from the American society of echocardiography. J Am Soc Echocardiogr. (2019) 32:1–64. doi: 10.1016/J.ECHO.2018.06.004
20. Xiao H, Li H, Wang JJ, Zhang JS, Shen J, An XB, et al. IL-18 cleavage triggers cardiac inflammation and fibrosis upon β-adrenergic insult. Eur Heart J. (2018) 39:60–9. doi: 10.1093/eurheartj/ehx261
21. Zoghbi WA, Adams D, Bonow RO, Enriquez-Sarano M, Foster E, Grayburn PA, et al. ASE guidelines and standards recommendations for noninvasive evaluation of native valvular regurgitation a report from the American society of echocardiography developed in collaboration with the society for cardiovascular magnetic resonance. J Am Soc Echocardiogr. (2017) 30:303–71. doi: 10.1016/j.echo.2017.01.007
22. Augustine DX, Coates-Bradshaw LD, Willis J, Harkness A, Ring L, Grapsa J, et al. Echocardiographic assessment of pulmonary hypertension: a guideline protocol from the British society of echocardiography. Echo Res Pract. (2018) 5:G11–24. doi: 10.1530/ERP-17-0071
23. Zhu Y, Tang X, Wang Z, Wei Y, Zhu X, Liu W, et al. Pulmonary hypertension parameters assessment by electrocardiographically gated computed tomography: normal limits by age, sex, and body surface area in a Chinese population. J Thorac Imaging. (2019) 34:329–37. doi: 10.1097/RTI.0000000000000359
24. Zeng Y, Yu Q, Maimaitiaili N, Li B, Liu P, Hou Y, et al. Clinical and predictive value of computed tomography angiography in high-altitude pulmonary hypertension. JACC Asia. (2022) 2:803–15. doi: 10.1016/j.jacasi.2022.09.014
25. Cao Y, Zhang Y, Song J. Multi-imaging modality facilitates screening of pulmonary hypertension at high altitude. JACC Asia. (2022) 2:816–8. doi: 10.1016/J.JACASI.2022.10.001
26. Rosenkranz S, Preston IR. Right heart catheterisation: best practice and pitfalls in pulmonary hypertension. Eur Respir Rev. (2015) 24:642–52. doi: 10.1183/16000617.0062-2015
27. Barbui T, Thiele J, Gisslinger H, Kvasnicka HM, Vannucchi AM, Guglielmelli P, et al. The 2016 WHO classification and diagnostic criteria for myeloproliferative neoplasms: document summary and in-depth discussion. Blood Cancer J. (2018) 8:15. doi: 10.1038/s41408-018-0054-y
28. Ingram GI, Hills M. Reference method for the one-stage prothrombin time test on hlman blood. International committee for standardization in hematology. Thromb Haemost. (1976) 36:237–8. doi: 10.1055/s-0038-1648029
29. Bhutani VK, Srinivas S, Castillo Cuadrado ME, Aby JL, Wong RJ, Stevenson DK. Identification of neonatal haemolysis: an approach to predischarge management of neonatal hyperbilirubinemia. Acta Paediatr Int J Paediatr. (2016) 105:e189–94. doi: 10.1111/apa.13341
30. FitzGerald JD, Dalbeth N, Mikuls T, Brignardello-Petersen R, Guyatt G, Abeles AM, et al. 2020 American college of rheumatology guideline for the management of gout. Arthritis Rheumatol. (2020) 72:879–95. doi: 10.1002/art.41247
31. Elsayed NA, Aleppo G, Aroda VR, Bannuru RR, Brown FM, Bruemmer D, et al. 2. Classification and diagnosis of diabetes: standards of care in diabetes—2023. Diabetes Care. (2023) 46:S19–40. doi: 10.2337/dc23-S002
32. Gali N, Hoeper MM, Humbert M, Torbicki A, Vachiery JL, Barbera JA, et al. Guidelines for the diagnosis and treatment of pulmonary hypertension. Eur Heart J. (2009) 30:2493–537. doi: 10.1093/eurheartj/ehp297
33. Galiè N, Humbert M, Vachiery JL, Gibbs S, Lang I, Torbicki A, et al. 2015 ESC/ERS guidelines for the diagnosis and treatment of pulmonary hypertension. Eur Respir J. (2015) 46:903–75. doi: 10.1183/13993003.01032-2015
34. Alves-Bezerra M, Cohen DE. Triglyceride metabolism in the liver. Compr Physiol. (2018) 8:1–22. doi: 10.1002/cphy.c170012
35. Malekan M, Ebrahimzadeh MA, Sheida F. The role of hypoxia-inducible factor-1alpha and its signaling in melanoma. Biomed Pharmacother. (2021) 141:111873. doi: 10.1016/j.biopha.2021.111873
36. Mesarwi OA, Moya EA, Zhen X, Gautane M, Zhao H, Giro PW, et al. Hepatocyte HIF-1 and intermittent hypoxia independently impact liver fibrosis in murine nonalcoholic fatty liver disease. Am J Respir Cell Mol Biol. (2021) 65:390–402. doi: 10.1165/rcmb.2020-0492OC
37. Chen J, Chen J, Fu H, Li Y, Wang L, Luo S, et al. Hypoxia exacerbates nonalcoholic fatty liver disease via the HIF-2α/PPARα pathway. Am J Physiol - Endocrinol Metab. (2019) 317:E710–22. doi: 10.1152/ajpendo.00052.2019
38. Trefts E, Gannon M, Wasserman DH. The liver. Curr Biol. (2017) 27:R1147–51. doi: 10.1016/j.cub.2017.09.019
39. Kramer MR, Marshall SE, Tiroke A, Lewiston NJ, Starnes VA, Theodore J. Clinical significance of hyperbilirubinemia in patients with pulmonary hypertension undergoing heart-lung transplantation. J Hear Lung Transplant. (1991) 10:317–21.
40. Adamson C, Cowan LM, de Boer RA, Diez M, Drożdż J, Dukát A, et al. Liver tests and outcomes in heart failure with reduced ejection fraction: findings from DAPA-HF. Eur J Heart Fail. (2022) 24:1856–68. doi: 10.1002/ejhf.2649
41. Bogaert J, Claessen G, Dresselaers T, Masci PG, Belge C, Delcroix M, et al. Magnetic resonance relaxometry of the liver—a new imaging biomarker to assess right heart failure in pulmonary hypertension. J Hear Lung Transplant. (2022) 41:86–94. doi: 10.1016/j.healun.2021.09.005
42. Rodriguez MA d, Deng L, Gemmink A, van Weeghel M, Aoun ML, Warnecke C, et al. Hypoxia-inducible lipid droplet-associated induces DGAT1 and promotes lipid storage in hepatocytes. Mol Metab. (2021) 47:101168. doi: 10.1016/j.molmet.2021.101168
43. Wang F, Zou J, Xu H, Huang W, Zhang X, Wei Z, et al. Effects of chronic intermittent hypoxia and chronic sleep fragmentation on gut microbiome, Serum metabolome. Liver and adipose tissue morphology. Front Endocrinol (Lausanne). (2022) 13:1–19. doi: 10.3389/fendo.2022.820939
44. Díaz-Gutiérrez J, Martínez-González MÁ, Izquierdo JJP, González-Muniesa P, Martínez JA, Bes-Rastrollo M. Living at higher altitude and incidence of overweight/obesity: prospective analysis of the SUN cohort. PLoS One. (2016) 11:1–13. doi: 10.1371/journal.pone.0164483
45. Yang H, Yang G, Xu M, Zhao Y, He S, Wang Q, et al. Impact of high altitude on the incidence of postoperative venous thromboembolism and its genetic susceptibility: a meta-analysis and systematic review. Sci Total Environ. (2022) 838:156632. doi: 10.1016/j.scitotenv.2022.156632
46. Ristić L, Rančić M, Radović M, Ćirić Z, Kurtović DK. Pulmonary embolism in chronic hypoxemic patients with and without secondary polycythemia—analysis of risk factors in prospective clinical study. Med Glas. (2013) 10:258–65.
47. Huston J, Shah SJ. Understanding the pathobiology of pulmonary hypertension due to left heart disease. Circ Res. (2023) 130:1382–403. doi: 10.1161/CIRCRESAHA.122.319967
48. Rischard FP, Bernardo RJ, Vanderpool RR, Kwon DH, Acharya T, Park MM, et al. Classification and predictors of right ventricular functional recovery in pulmonary arterial hypertension. Circ Heart Fail. (2023) 16:e010555. doi: 10.1161/CIRCHEARTFAILURE.123.010555
49. Humbert M, Guignabert C, Bonnet S, Dorfmüller P, Klinger JR, Nicolls MR, et al. Pathology and pathobiology of pulmonary hypertension: state of the art and research perspectives. Eur Respir J. (2019) 53:1801887. doi: 10.1183/13993003.01887-2018
50. Lichtblau M, Saxer S, Furian M, Mayer L, Bader PR, Scheiwiller PM, et al. Cardiac function and pulmonary hypertension in central Asian highlanders at 3250 m. Eur Respir J. (2020) 56:1902474. doi: 10.1183/13993003.02474-2019
51. McGoon MD, Benza RL, Escribano-Subias P, Jiang X, Miller DP, Peacock AJ, et al. Pulmonary arterial hypertension: epidemiology and registries. J Am Coll Cardiol. (2013) 34:D51–9. doi: 10.1016/j.jacc.2013.10.023
52. Vanderpool RR, Naeije R. Hematocrit-corrected pulmonary vascular resistance. Am J Respir Crit Care Med. (2018) 198:305–9. doi: 10.1164/rccm.201801-0081PP
53. Sadiku P, Willson JA, Ryan EM, Carmeliet P, Whyte MKB, Walmsley SR. Neutrophils fuel effective immune responses through gluconeogenesis and glycogenesis. Cell Metab. (2021) 33:1–13. doi: 10.1016/j.cmet.2020.11.016
Keywords: high-altitude pulmonary hypertension, high-altitude, GENTH score model, prediction model, pulmonary hypertension
Citation: Zeng Y, Zhakeer G, Li B, Yu Q, Niu M, Maimaitiaili N, Mi M, Deji Z, Zhuang J and Peng W (2024) A novel clinical prediction scoring system of high-altitude pulmonary hypertension. Front. Cardiovasc. Med. 10:1290895. doi: 10.3389/fcvm.2023.1290895
Received: 23 October 2023; Accepted: 15 December 2023;
Published: 8 January 2024.
Edited by:
Gino Seravalle, Italian Auxological Institute (IRCCS), ItalyReviewed by:
Argen Mamazhakypov, University of Freiburg, GermanyYunshan Cao, Gansu Provincial Hospital, China
Yi Yan, Shanghai Jiaotong University School of Medicine, China
© 2024 Zeng, Zhakeer, Li, Yu, Niu, Maimaitiaili, Mi, Deji, Zhuang and Peng W. This is an open-access article distributed under the terms of the Creative Commons Attribution License (CC BY). The use, distribution or reproduction in other forums is permitted, provided the original author(s) and the copyright owner(s) are credited and that the original publication in this journal is cited, in accordance with accepted academic practice. No use, distribution or reproduction is permitted which does not comply with these terms.
*Correspondence: Jianhui Zhuang amhfemh1YW5nQHRvbmdqaS5lZHUuY24= Wenhui Peng cHdlbmh1aUB0b25namkuZWR1LmNu
†These authors have contributed equally to this work and share first authorship
Abbreviations PH, pulmonary hypertension; HH, healthy highlanders; HAPH, high-altitude pulmonary hypertension; RHC, right heart catheterization; mPAPPredicted, mean pulmonary arterial pressure predicted with CTA models; mPAPRHC, mean pulmonary arterial pressure measured by right heart catheterization; rRLA, the ratio of right to left atrial diameter; ABR, artery-bronchus ratio; PASP, pulmonary arterial systolic pressure; TRPG, tricuspid regurgitation pressure gradient.