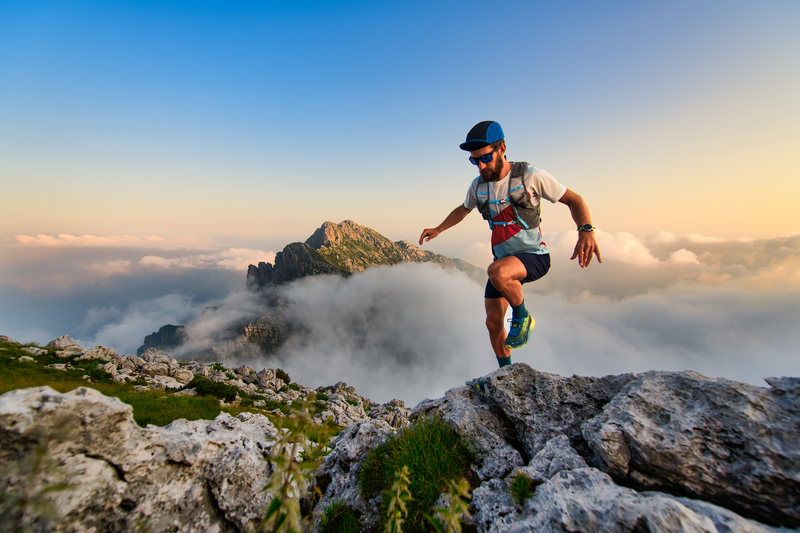
94% of researchers rate our articles as excellent or good
Learn more about the work of our research integrity team to safeguard the quality of each article we publish.
Find out more
ORIGINAL RESEARCH article
Front. Cardiovasc. Med. , 21 November 2023
Sec. Cardiovascular Epidemiology and Prevention
Volume 10 - 2023 | https://doi.org/10.3389/fcvm.2023.1269358
This article is part of the Research Topic Obesity and Cardiovascular Disease View all 4 articles
Objective: To assess the association between dynamic patterns of change in body mass or fat mass and stroke.
Methods: A population-based cohort of participants was selected from the China Health and Retirement Longitudinal Study (CHARLS). Body mass and fat mass were measured using obesity-related indices, including weight, body mass index (BMI), waist circumference (WC), waist-to-height ratio (WHtR), lipid accumulation product (LAP), and visceral adiposity index (VAI). Five changed patterns were defined: low-stable, decreasing, moderate, increasing, and persistent-high. Logistic regression analysis was performed to evaluate the association between obesity-related indices and stroke.
Results: A total of 5,834 participants were included, and the median age was 58.0 years. During a 7-years follow-up period, 354 (6.1%) participants developed stroke. The baseline levels of obesity-related indices were significantly associated with incident stroke. Regarding the dynamic change patterns, the low-stable pattern carried the lowest odds for stroke and the persistent-high pattern had the highest odds for stroke, with odds ratios of all the indices ranging from 1.73 to 3.37 (all P < 0.05). The increasing pattern was also associated with a higher odds of stroke, whereas the moderate pattern of weight, BMI, and WHtR was comparable to the low-stable pattern in terms of stroke.
Conclusion: Current status and dynamic changes in body mass and fat mass were significantly associated with incident stroke. Maintaining the low-stable pattern of body mass and fat mass as measured by weight, WC, BMI, WHtR, LAP, and VAI may be an alternative strategy for primary stroke prevention.
Stroke is the second leading cause of premature death and disability and its incidence has been increased over the past three decades (1, 2). The burden of stroke has risen steadily since 1990, with 143 million loss of disability-adjusted life years, 6.55 million deaths due to stroke, and 101 million prevalent stroke cases in 2019 (3). Globally, 90.5% of the stroke burden is attributable to modifiable risk factors, including behavioral (such as poor diet and low physical activity) and metabolic (such as high body-mass index) factors (4). It suggests that stroke can be prevented to some extent by improving the lifestyle. Given the stroke's impact on quality of life and financial burden, the idea that prevention is always better than cure is desirable.
Among the risk factors for stroke, obesity has been the focus of attention, given the possibility of an obesity paradox. Several studies have reported an association between obesity and stroke; however, the results have been inconsistent. A Mendelian randomization study revealed that abdominal adiposity may lead to cerebrovascular diseases independent of blood pressure and glucose levels (5), whereas general obesity was not significantly associated with stroke (6). Previous studies were limited by methodological concerns, including the retrospective nature of most studies, the assessment of obesity using only body mass index (BMI), and potential confounding factors (7). In addition, most studies only used parameters from a single measurement, and few studies have assessed the effect of dynamic changes in obesity status on stroke. It has been shown that stable obesity and weight gain were associated with an increased mortality risk (8). Moreover, waist circumference (WC) increase is associated with higher mortality risk in middle-aged and older Chinese adults (9).
Given that the obesity status could change over time, the present study evaluated the association between dynamic change patterns of body mass or fat mass and the odds of stroke. Body mass or fat mass was measured using several commonly used obesity-related indices, including weight, WC, BMI, waist-to-height ratio (WHtR), lipid accumulation product (LAP), and visceral adiposity index (VAI).
Study participants were selected from the China Health and Retirement Longitudinal Study (CHARLS), which is a nationally representative cohort. This project was supported by Peking University, and the details are available on its website (http://charls.pku.edu.cn/en). The CHARLS aims to collect a high-quality, nationally representative sample of Chinese residents aged 45 years and older to serve the needs of scientific research on middle-aged and older people. The baseline national wave of CHARLS was conducted in 2011, and 17,708 individuals from 28 provinces (150 counties/districts and 450 villages/residential committees) in China participated in the investigation. Three subsequent follow-ups were performed in 2013–2014 (wave 2), 2015–2016 (wave 3), and 2017–2018 (wave 4) and two blood samples were collected in waves 1 and 3.
The participants selection process is illustrated in Figure 1. Briefly, 9,546 individuals with complete data were screened for eligibility. For the present study, to evaluate the association between the dynamic change patterns of obesity status and the odds of stroke, those without a blood test at wave 3 were excluded. We also excluded participants who had experienced stroke events before/at wave 3 to minimize potential reverse causality. Finally, 5,834 participants were included (Figure 1). The CHARLS study received approval from the institutional review board of Peking University, and all participants provided written informed consent.
Information on demographics, socioeconomics, and health status was collected by trained investigators using computer-assisted personal interviews as described previously (10). The variables included in this study were age, sex, BMI, smoking and drinking consumption status, educational levels, systolic blood pressure (SBP), diastolic blood pressure (DBP), fasting plasma glucose (FPG), total cholesterol (TC), triglycerides (TG), low-density lipoprotein cholesterol (LDL-C), high-density lipoprotein cholesterol (HDL-C), serum creatinine (Scr), uric acid (UA), and high-sensitivity C reaction protein (hs-CRP). The chronic diseases included hypertension, diabetes mellitus (DM), and heart disease. Hypertension was defined as SBP ≥140 mmHg and/or DBP ≥90 mmHg or being under anti-hypertensive therapy. DM was defined as FPG ≥7.0 mmol/L or a history of DM diagnosed by a physician. Heart diseases included coronary heart disease, congestive heart failure and heart attacks.
The primary exposure was body mass or fat mass and its dynamic change patterns, measured using obesity-related indices including weight, WC, BMI, WHtR, LAP, and VAI. We first divided the study population into four groups according to the values of each index, with the first (Q1) and last (Q4) groups being those with values in lowest and highest quartiles, respectively. Five patterns were identified, based on the longitudinal trends of each index from the wave 1 to wave 3 surveys to evaluate dynamic changes in obesity status, including the low stable, decreasing, moderate, increasing, and persistent-high. Individuals with an index remaining in the lowest (Q1) or highest (Q4) quartiles in both surveys were identified as having low-stable or persistent-high patterns, respectively. Those remaining in Q2 and Q3 in both surveys were considered to have a moderate pattern. The decreasing group was defined as a decrease from a higher to a lower quartile, whereas the reverse was true for the definition of the increasing group. The outcome of the present study was an incident stroke during the wave 3 and wave 4 surveys, data on which was collected by asking a participant, “Have you been told by a doctor that you have been diagnosed with a stroke?” (11).
Data were presented as median (interquartile range) and number (percentage) where appropriate and were compared between the stroke and non-stroke groups using Mann–Whitney U-test or Chi-square test.
Given the same follow-up interval (from wave 3 to wave 4), logistic regression analysis was used to evaluate the association of obesity status and its dynamic change patterns with incident stroke. We first constructed an adjustment model (model 1) derived from directed acyclic graphs (DAGs) which generated minimal sufficient adjustment sets based on causal assumptions (Supplementary Figure S1). In addition, another two models were generated based on previous literature reports, clinical knowledge and collinearity testing. Model 2 was adjusted for demographic factors, physical and laboratory examinations, including age, sex, smoking and drinking consumption status, educational level, SBP, FPG, TC, LDL-C, Scr, UA, and hs-CRP levels. Model 3 further considered the disease status, including hypertension, DM, and heart disease. There was no significant collinearity among variables in the model 3. Odds ratios (OR) were reported for per SD increase of each index, quartile group (with Q1 serving as a reference), and change pattern (with the low-stable pattern serving as a reference). To response to reviewer's comments, we performed a sensitivity analysis by excluded those who developed hypertension or DM during the follow-up period. Statistical analyses were performed using SPSS 23.0 for Windows. A two-sided P < 0.05 was considered statistically significant.
A total of 5,834 participants free of stroke at baseline were included in the final analysis, 54.5% of whom were women. The baseline characteristics of the study population are presented in Table 1. The median age was 58.0 years, and BP and FPG were 127/74 mmHg and 5.7 mmol/L, respectively. The percentages of patients with hypertension and DM were 39.5% and 15.9%, respectively. During a 7-years follow-up period, 354 (6.1%) participants developed stroke. Compared to the non-stroke group, those who developed a stroke were older and had higher BP, FPG, TG, and hs-CRP levels. The proportions of patients with hypertension, DM and heart disease were higher in the stroke group than in the non-stroke group. All six obesity-related indices, including weight, WC, BMI, WHtR, LAP, and VAI, were higher in the stroke group than in the non-stroke group (Table 1).
The cutoff points and numbers of participants for each quartile group of obesity-related indices are shown in Supplementary Table S1. The incidence of stroke increased with each index, and the fourth quartile had the highest incidence of stroke. After adjusting for age, sex, smoking and alcohol consumption status, educational level, SBP, FPG, TC, LDL, Scr, UA, hs-CRP, and history of hypertension, DM, and heart diseases, all obesity indices were positively associated with the odds of stroke (Figure 2). Compared with the lowest quartiles, the highest quartiles had 64%–96% increased odds of incident stroke (all P < 0.05). Each SD increase was associated with a 15%–28% increased odds of stroke (all P < 0.05).
Figure 2. Association between baseline adiposity indices and incident stroke. Results are shown as odds ratios (95% CI) derived from logistic regression models adjusted for age, sex, smoking, drinking, educational levels, SBP, FPG, TC, LDL-C, Scr, UA, hs-CRP, history of hypertension, diabetes, and heart diseases.
Details of the sample distribution of the five change patterns of the indices are listed in Supplementary Table S1. The persistent-high pattern of each index was associated with the highest stroke incident rate. Univariate analysis revealed that compared with the low-stable pattern, the other four patterns had a significantly higher odds of incident stroke (Table 2). Similar results were obtained when adjusted for variables selected by DAGs analysis (Table 2, Model 1). The results did not change substantially after adjusting for demographic factors and physical and laboratory examination results (Table 2, Model 2). When disease status was further considered (Table 2, Model 3), the association between obesity-related indices and stroke was attenuated but remained significant. The odds ratios for the persistent-high patterns of all indices ranged from 1.73 to 3.37 (all P < 0.05). The increasing pattern had a higher odds of stroke, whereas the moderate pattern of weight, BMI, and WHtR showed odds of stroke comparable to the low-stable pattern. Sensitivity analysis by excluded those who developed hypertension or DM during the follow-up period yielded consistent results (Supplementary Table S2).
The present study, based on a nationally representative cohort with a median follow-up period of 7 years, demonstrates that current status and dynamic changes in body mass and fat mass are significantly associated with incident stroke. For immediate status, those in the highest quartile had a 64%–96% increased odds of stroke compared with those in the lowest quartile of each obesity-related index, including weight, WC, BMI, WHtR, LAP, and VAI. In addition, a persistent-high pattern for each of the six indices indicated the highest odds of stroke development. Moreover, an increasing pattern also increases the stroke odds.
Although the association between obesity and cardiovascular disease has been widely reported, the impact of dynamic changes in body mass or fat mass on health status has only recently come into focus. In the Chinese population, stable obesity across adulthood, weight gain from young to middle adulthood and weight loss from middle to late adulthood were associated with an increased mortality risk (8). Another study conducted in the United States showed that weight loss from obesity to overweight between early adulthood and mid-life appeared to be associated with a reduction in mortality risk compared to persistent obesity, suggesting a benefit of weight loss for obese individuals (12). More recently, Hussain et al. revealed that weight loss and WC decrease were associated with an increase in all-cause mortality (13). Several studies have reported an association between weight change and stroke with inconsistent results. A study from Japan showed that weight gain during middle age was associated with an increased risk of stroke in women (14). In contrast, results from Norway revealed that weight gain during early life but not mid-life was associated with an increased risk of stroke in healthy men (15). In the present study, conducted in Chinese middle-aged and older populations, decreasing, increasing, and persistent-high patterns of weight change were associated with a significantly higher odds of stroke, suggesting that maintaining low- or moderate-stable weight patterns after middle age may be most beneficial for reducing stroke risk. Similar results were found in a recent study in which weight loss did not reduce the risk of stroke in obese individuals (16).
In the present study, changes in BMI, WC and WHtR had similar effects on stroke and body weight. Several studies have reported an association between changes in WC and mortality; however, the conclusions remained inconsistent (17–20). Yuan et al. recently reported that both gain and loss in WC were associated with a higher mortality risk compared to the stable group (9), similar to our study that focused on the outcome of stroke. Therefore, maintaining a low- to moderate-stable pattern of WC levels, rather than increasing or decreasing them, may be beneficial for preventing stroke. To the best of our knowledge, the effects of visceral fat changes on stroke have rarely been evaluated. Our study measured fat accumulation using LAP and VAI: the immediate level of visceral fat and dynamic patterns were significantly associated with stroke. As expected, the low-stable pattern was associated with the lowest odds of stroke. In addition, among the other dynamic patterns, including the decreasing, moderate, increasing, and persistent-high patterns, the decreasing pattern had a lower odds of stroke development, indicating a potential benefit for stroke prevention by reducing visceral fat in those with moderate to high levels. The impact of visceral adiposity has been demonstrated to be greater than that of general and abdominal obesity (21, 22). Future studies should focus on the changes of body weight and WC and further explore the relationship between the change pattern of visceral fat and stroke and other cardiovascular diseases.
The strengths of the present study include the use of a nationally representative cohort with a prospective design that allowed us to evaluate the association between dynamic changes in body mass and fat mass and the odds of stroke and the fact that a series of confounding factors were considered to provide insights for the primary prevention of stroke. This study has some limitations that warrant further discussion. First, the changed patterns of obesity-related indices were based on quartile groups owing to the lack of a standard cut-off value, and we could only provide qualitative rather than quantitative results. In addition, data-driven methods, such as growth mixture modeling or latent class models, would be better for defining temporal patterns. Secondly, the interval between the two measurements was relatively short, and the effect of long-term changes on the outcome could not be assessed. However, a short-term change pattern may be more appropriate for risk assessment and intervention in a large sample population. Third, we failed to distinguish between intentional and unintentional changes in obesity status. Future studies should focus on the reasons for these changes in developing appropriate intervention strategies. It is also important to consider both diet and exercise parameters simultaneously. Finally, although adjusted for a series of known confounders, other unmeasured factors should be further considered in future studies.
In conclusion, not only immediate status but also dynamic changes in body mass and fat mass are significantly associated with incident stroke. Maintaining the low-stable pattern of body mass and fat mass as measured by weight, BMI, WHtR, LAP, and VIA may be an alternative strategy for primary stroke prevention. For those with moderate levels, maintaining stability and decreasing variation (rather than increasing or decreasing) may have the best benefits, and an appropriate reduction is recommended for those with high obesity-related indices.
The original contributions presented in the study are included in the article/Supplementary Material, further inquiries can be directed to the corresponding author. Data of the China Health and Retirement Longitudinal Study are available at its website (http://charls.pku.edu.cn/).
The studies involving humans were approved by The institutional review board of Peking University. The studies were conducted in accordance with the local legislation and institutional requirements. The participants provided their written informed consent to participate in this study.
ML: Conceptualization, Formal analysis, Investigation, Methodology, Visualization, Writing – original draft, Writing – review & editing. SZ: Investigation, Methodology, Writing – review & editing. SG: Conceptualization, Formal analysis, Methodology, Supervision, Writing – original draft, Writing – review & editing.
The author(s) declare that no financial support was received for the research, authorship, and/or publication of this article.
We are grateful to the entire CHARLS study team and to those participants who participated in the CHARLS study.
The authors declare that the research was conducted in the absence of any commercial or financial relationships that could be construed as a potential conflict of interest.
All claims expressed in this article are solely those of the authors and do not necessarily represent those of their affiliated organizations, or those of the publisher, the editors and the reviewers. Any product that may be evaluated in this article, or claim that may be made by its manufacturer, is not guaranteed or endorsed by the publisher.
The Supplementary Material for this article can be found online at: https://www.frontiersin.org/articles/10.3389/fcvm.2023.1269358/full#supplementary-material
1. Benjamin EJ, Muntner P, Alonso A, Bittencourt MS, Callaway CW, Carson AP, et al. Heart disease and stroke statistics-2019 update: a report from the American heart association. Circulation. (2019) 139(10):e56–528. doi: 10.1161/CIR.0000000000000659
2. GBD 2019 Stroke Collaborators. Global, regional, and national burden of stroke and its risk factors, 1990–2019: a systematic analysis for the global burden of disease study 2019. Lancet Neurol. (2021) 20(10):795–820. doi: 10.1016/S1474-4422(21)00252-0
3. Roth GA, Mensah GA, Johnson CO, Addolorato G, Ammirati E, Baddour LM, et al. Global burden of cardiovascular diseases and risk factors, 1990–2019: update from the GBD 2019 study. J Am Coll Cardiol. (2020) 76(25):2982–3021. doi: 10.1016/j.jacc.2020.11.010
4. Feigin VL, Roth GA, Naghavi M, Parmar P, Krishnamurthi R, Chugh S, et al. Global burden of stroke and risk factors in 188 countries, during 1990–2013: a systematic analysis for the global burden of disease study 2013. Lancet Neurol. (2016) 15(9):913–24. doi: 10.1016/S1474-4422(16)30073-4
5. Marini S, Merino J, Montgomery BE, Malik R, Sudlow CL, Dichgans M, et al. Mendelian randomization study of obesity and cerebrovascular disease. Ann Neurol. (2020) 87(4):516–24. doi: 10.1002/ana.25686
6. Dale CE, Fatemifar G, Palmer TM, White J, Prieto-Merino D, Zabaneh D, et al. Causal associations of adiposity and body fat distribution with coronary heart disease, stroke subtypes, and type 2 diabetes Mellitus: a Mendelian randomization analysis. Circulation. (2017) 135(24):2373–88. doi: 10.1161/CIRCULATIONAHA.116.026560
7. Forlivesi S, Cappellari M, Bonetti B. Obesity paradox and stroke: a narrative review. Eat Weight Disord. (2021) 26(2):417–23. doi: 10.1007/s40519-020-00876-w
8. Chen C, Ye Y, Zhang Y, Pan XF, Pan A. Weight change across adulthood in relation to all cause and cause specific mortality: prospective cohort study. Br Med J. (2019) 367:l5584. doi: 10.1136/bmj.l5584
9. Yuan Y, Liu K, Zheng M, Chen S, Wang H, Jiang Q, et al. Analysis of changes in weight, Waist circumference, or both, and all-cause mortality in Chinese adults. JAMA Netw Open. (2022) 5(8):e2225876. doi: 10.1001/jamanetworkopen.2022.25876
10. Zhao Y, Hu Y, Smith JP, Strauss J, Yang G. Cohort profile: the China health and retirement longitudinal study (CHARLS). Int J Epidemiol. (2014) 43(1):61–8. doi: 10.1093/ije/dys203
11. Cui C, He C, Sun Q, Xu Z, Li Q, Yue S, et al. Association between visceral adiposity index and incident stroke: data from the China health and retirement longitudinal study. Nutr Metab Cardiovasc Dis. (2022) 32(5):1202–9. doi: 10.1016/j.numecd.2022.01.031
12. Xie W, Lundberg DJ, Collins JM, Johnston SS, Waggoner JR, Hsiao CW, et al. Association of weight loss between early adulthood and midlife with all-cause mortality risk in the US. JAMA Netw Open. (2020) 3(8):e2013448. doi: 10.1001/jamanetworkopen.2020.13448
13. Hussain SM, Newman AB, Beilin LJ, Tonkin AM, Woods RL, Neumann JT, et al. Associations of change in body size with all-cause and cause-specific mortality among healthy older adults. JAMA Netw Open. (2023) 6(4):e237482. doi: 10.1001/jamanetworkopen.2023.7482
14. Kisanuki K, Muraki I, Yamagishi K, Kokubo Y, Saito I, Yatsuya H, et al. Weight change during middle age and risk of stroke and coronary heart disease: the Japan public health center-based prospective study. Atherosclerosis. (2021) 322:67–73. doi: 10.1016/j.atherosclerosis.2021.02.017
15. Prestgaard E, Mariampillai J, Engeseth K, Erikssen J, Bodegård J, Liestøl K, et al. Change in body weight and long-term risk of stroke and death in healthy men. Stroke. (2020) 51(5):1435–41. doi: 10.1161/STROKEAHA.119.027233
16. Katsoulis M, Stavola BD, Diaz-Ordaz K, Gomes M, Lai A, Lagiou P, et al. Weight change and the onset of cardiovascular diseases: emulating trials using electronic health records. Epidemiology. (2021) 32(5):744–55. doi: 10.1097/EDE.0000000000001393
17. Klingberg S, Mehlig K, Lanfer A, Björkelund C, Heitmann BL, Lissner L. Increase in waist circumference over 6 years predicts subsequent cardiovascular disease and total mortality in nordic women. Obesity (Silver Spring). (2015) 23(10):2123–30. doi: 10.1002/oby.21203
18. Lee WJ, Peng LN, Loh CH, Chen LK. Effect of body weight, Waist circumference and their changes on mortality: a 10-year population-based study. J Nutr Health Aging. (2018) 22(8):959–64. doi: 10.1007/s12603-018-1042-4
19. Roswall N, Li Y, Sandin S, Ström P, Adami HO, Weiderpass E. Changes in body mass index and waist circumference and concurrent mortality among Swedish women. Obesity (Silver Spring). (2017) 25(1):215–22. doi: 10.1002/oby.21675
20. Mousavi SV, Mohebi R, Mozaffary A, Sheikholeslami F, Azizi F, Hadaegh F. Changes in body mass index, waist and hip circumferences, waist to hip ratio and risk of all-cause mortality in men. Eur J Clin Nutr. (2015) 69(8):927–32. doi: 10.1038/ejcn.2014.235
21. Alwash SM, McIntyre HD, Mamun A. The association of general obesity, central obesity and visceral body fat with the risk of gestational diabetes mellitus: evidence from a systematic review and meta-analysis. Obes Res Clin Pract. (2021) 15(5):425–30. doi: 10.1016/j.orcp.2021.07.005
22. Zheng J, Hu Y, Xu H, Lei Y, Zhang J, Zheng Q, et al. Normal-weight visceral obesity promotes a higher 10-year atherosclerotic cardiovascular disease risk in patients with type 2 diabetes mellitus-a multicenter study in China. Cardiovasc Diabetol. (2023) 22(1):137. doi: 10.1186/s12933-023-01876-7
Keywords: stroke, obesity, dynamic change, risk, primary prevention
Citation: Lin M, Zhou S and Gu S (2023) Association between dynamic change patterns of body mass or fat mass and incident stroke: Results from the China health and retirement longitudinal study (CHARLS). Front. Cardiovasc. Med. 10:1269358. doi: 10.3389/fcvm.2023.1269358
Received: 4 August 2023; Accepted: 6 November 2023;
Published: 21 November 2023.
Edited by:
Rodrigo Bazan, São Paulo State University, BrazilReviewed by:
Stefan Gross, University Medicine Greifswald, Germany© 2023 Lin, Zhou and Gu. This is an open-access article distributed under the terms of the Creative Commons Attribution License (CC BY). The use, distribution or reproduction in other forums is permitted, provided the original author(s) and the copyright owner(s) are credited and that the original publication in this journal is cited, in accordance with accepted academic practice. No use, distribution or reproduction is permitted which does not comply with these terms.
*Correspondence: Shanhong Gu MzE0OTk1NDMxQHFxLmNvbQ==
Disclaimer: All claims expressed in this article are solely those of the authors and do not necessarily represent those of their affiliated organizations, or those of the publisher, the editors and the reviewers. Any product that may be evaluated in this article or claim that may be made by its manufacturer is not guaranteed or endorsed by the publisher.
Research integrity at Frontiers
Learn more about the work of our research integrity team to safeguard the quality of each article we publish.