- 1Division of Internal Medicine, Department of Medicine, Stanford University, Stanford, CA, United States
- 2Stanford Cardiovascular Institute, Stanford, CA, United States
- 3Division of Cardiovascular Medicine, Department of Medicine, Stanford University, Stanford, CA, United States
- 4Department of Computer Science, Stanford University, Stanford, CA, United States
Introduction: Left ventricular hypertrophy (LVH) detection techniques on by electrocardiogram (ECG) are cumbersome to remember with modest performance. This study validated a rapid technique for LVH detection and measured its performance against other techniques.
Methods: This was a retrospective cohort study of patients at Stanford Health Care who received ECGs and resting transthoracic echocardiograms (TTE) from 2006 through 2018. The novel technique, Witteles-Somani (WS), assesses for S- and R-wave overlap on adjacent precordial leads. The WS, Sokolow-Lyon, Cornell, and Peguero-Lo Presti techniques were algorithmically implemented on ECGs. Classification metrics, receiver-operator curves, and Pearson correlations measured performance. Age- and sex-adjusted Cox proportional hazard models evaluated associations between incident cardiovascular outcomes and each technique.
Results: A total of 53,333 ECG-TTE pairs from 18,873 patients were identified. Of all ECG-TTE pairs, 21,638 (40.6%) had TTE-diagnosed LVH. The WS technique had a sensitivity of 0.46, specificity of 0.66, and AUROC of 0.56, compared to Sokolow-Lyon (AUROC 0.55), Cornell (AUROC 0.63), and Peguero-Lo Presti (AUROC 0.63). Patients meeting LVH by WS technique had a higher risk of cardiovascular mortality [HR 1.18, 95% CI (1.12, 1.24), P < 0.001] and a higher risk of developing any cardiovascular disease [HR 1.29, 95% CI (1.22, 1.36), P < 0.001], myocardial infarction [HR 1.60, 95% CI (1.44, 1.78), P < 0.005], and heart failure [HR 1.24, 95% CI (1.17, 1.32), P < 0.001].
Conclusions: The WS criteria is a rapid visual technique for LVH detection with performance like other LVH detection techniques and is associated with incident cardiovascular outcomes.
Introduction
Left ventricular (LV) hypertrophy (LVH) is the presence of increased LV mass and is most commonly driven by a pathologic response to chronic afterload stressors (e.g., hypertension, aortic valve disease) or inherited myopathies (e.g., hypertrophic cardiomyopathy) (1). It has been correlated with all-cause and cardiovascular mortality, as well as the incidence of other cardiovascular diseases (e.g., atrial fibrillation, heart failure), suggesting a possible role for earlier intervention (2). Existing techniques for LVH detection on electrocardiograms (ECG) are insensitive and can be laborious to remember and implement at the bedside (3). Artificial intelligence (AI) models have shown promise in improving screening (4), but suffer from deployment issues given the infancy of healthcare implementation science and infrastructure limitations to expand to resource-limited care centers, especially in primary care (5). We hypothesized that high early precordial lead (directed away from the LV) S-wave amplitudes and high later precordial lead (directed towards the LV) R-wave amplitudes would suggest a high or delayed LV depolarization signal, and that overlap of these on adjacent precordial leads would be associated with LVH. We therefore developed a fast and simple technique for LVH detection by ECG based on any QRS complex overlap on the precordial leads, validated its performance against existing techniques in a large validation cohort, and evaluated its association with the incidence of future cardiovascular events.
Methods
The Witteles–Somani (WS) criteria is defined here as the overlap of QRS complexes between adjacent precordial leads, which can easily be detected visually (Figures 1A–D). The criteria were automated in this analysis as the sum of the height of the S-wave from lead Vi and height of the R-wave from lead Vi + 1 exceeding 20 mm, which is equal to 2 mV on conventional 12-lead ECG displays calibrated at 10 mm per 1 mV.
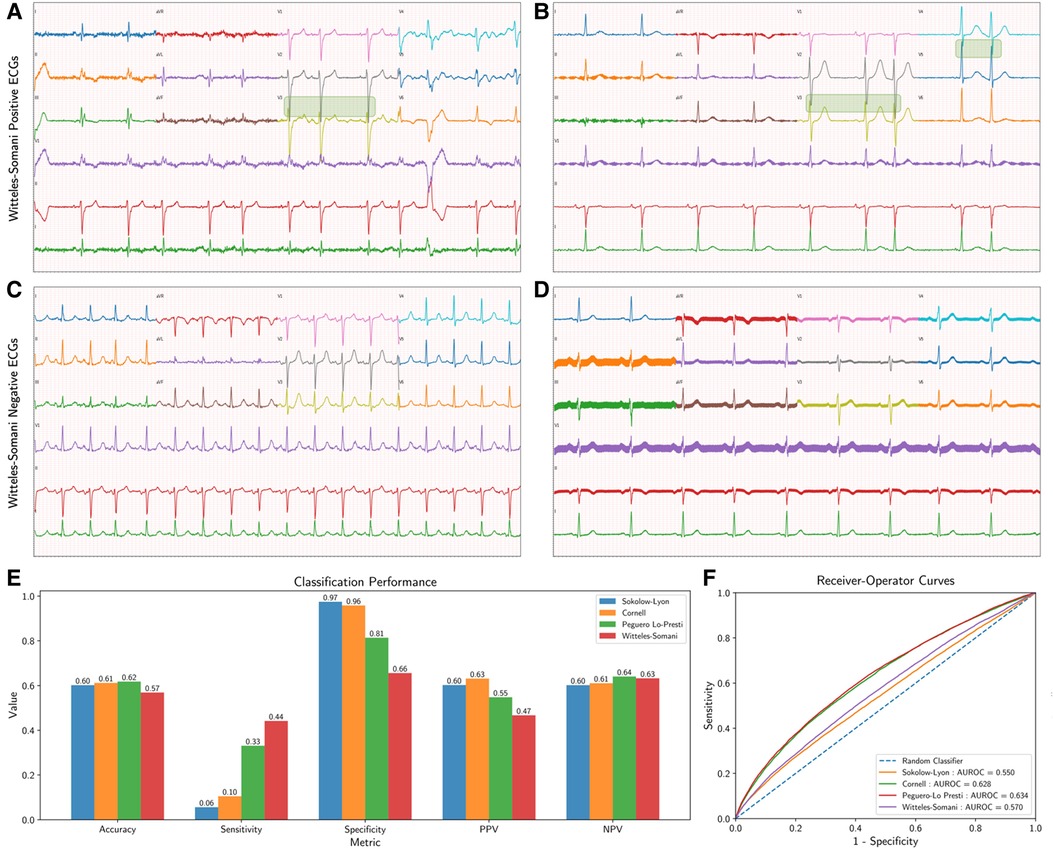
Figure 1. Witteles–Somani technique and performance. This figure demonstrates two examples of WS-positive (A,B) and WS-negative ECGs (C,D); areas of precordial overlap contributing to the positive diagnosis are overlaid with a green box. Performance measures for the various LVH detection techniques are also shown; performance on simple classification of LVH at their pre-specified thresholds (E) and changes in sensitivity and specificity as demonstrated by receiver-operator curves at various thresholds (F). LVH, left ventricular hypertrophy; WS, Witteles–Somani technique.
We conducted a retrospective cohort analysis that included patients ≥18 years of age at Stanford Health Care who had a resting transthoracic echocardiogram (TTE) and a 12-lead resting ECG performed between March 1, 2006 and May 31, 2018. ECGs closest in time to and within one year of each TTE were used to create a unique ECG-TTE pair. ECGs with bundle branch blocks (left and right), paced rhythms, or quality control warnings were excluded from this study. Demographic data and medical history were collected from the electronic health record. Cardiovascular mortality and incident cardiovascular diseases including heart failure and myocardial infarction were collected from diagnosis codes (Supplementary Table S1) in the medical record.
LV mass index (LVMI) for each echocardiogram was calculated using the Devereaux formula for LVH and normalized to the body surface area (BSA); LVH was defined as LVMI >95 g/m2 in males and LVMI >115 g/m2 in females (6). This study was approved by the Stanford University Institutional Review Board (IRB-41045). Full details on data acquisition, representation, and exclusion criteria are discussed in the Supplementary Methods.
To compare the WS criteria with other described methods of ECG-based LVH detection, we algorithmically implemented the Sokolow–Lyon, Cornell, and Peguero–Lo Presti techniques for evaluation from these ECGs (7–9). Because all LVH detection techniques rely on R- and S-wave amplitudes, QRS complexes from raw ECG waveforms were identified using the Christov algorithm (10). Additional details on implementation are discussed in the Supplementary Methods.
We generated point estimates for classification metrics—accuracy, sensitivity, specificity, positive predictive value (PPV), negative predictive value (NPV), and odds ratios. The McNemar test was used to assess agreement for LVH detected by each technique with LVH detected by TTE (null hypothesis). We performed sensitivity analyses to determine how sex-based threshold adjustment and incorporation of other visual ECG signs previously linked to etiologies of HCM (e.g., ST-segment depressions, left atrial enlargement, and T-wave inversions) could affect the performance of the WS technique (11). We generated receiver-operator curves (ROC) and their areas (AUROC) by using R- and S-wave measurements from each technique (maximum across the median of all criterion values across all heartbeats, MMCV; see Supplementary Methods) to assess threshold-independent performance. Next, we calculated the Pearson statistic to assess the linear correlation between the MMCV for each ECG and the LVMI. Finally, we developed age- and sex-adjusted Cox proportional hazards models for each technique and TTE-based LVH to determine the hazard ratios for cardiovascular mortality and incident myocardial infarction, heart failure, and any cardiovascular disease (defined as the incidence of MI, stroke/hemorrhage, sudden cardiac death, and heart failure hospitalization). Additional details are discussed in the Supplementary Methods.
Results
A total of 53,333 ECG-TTE pairs from 18,873 patients were included in the analysis. In this cohort, the average age was 67.1 years ± 16.1 years and 49.8% were female, 6.1% were Black, 13.3% were Asian, and 7% were Hispanic. Of all ECG-TTE pairs, 21,638 (40.6%) had TTE-diagnosed LVH.
On univariate analysis, patients who were positive by the WS technique were more likely to be male (65.9% vs. 45.1%, P < 0.001) and be dialysis dependent (6.2% vs. 5.2%, P < 0.001), but less likely to have hypertension (45.6% vs. 47.7%, P < 0.001), diabetes (13.4% vs. 15.8%, P < 0.001), and prior MI (8.3% vs. 9.5%, P < 0.001). There were no statistical differences in age and history of heart failure, coronary artery disease, stroke, or ASCVD between the WS-positive and WS-negative cohorts (Table 1).
The WS technique had a sensitivity of 0.44, a specificity of 0.66, and an accuracy of 0.57 for detecting LVH. Other performance measures of WS and other LVH criteria are shown in Figure 1E and Supplementary Table S3. All techniques—Sokolow–Lyon (χ2 = 18148.4, P < 0.001), Cornell (χ2 = 15725.6, P < 0.001), Peguero–Lo Presti (χ2 = 3603.6, P < 0.001), and WS (χ2 = 59.3, P < 0.001), rejected the null hypothesis of statistical similarity between technique-based LVH and TTE-based LVH. The WS technique had an AUROC 0.56 for classifying LVH independent of pre-established thresholds, compared to Sokolow–Lyon (AUROC 0.55), Cornell (AUROC 0.63), and Peguero–Lo Presti (AUROC 0.63) (Figure 1F). Adjusting the threshold for LVH detection based on patient sex by 10 mm (2 “big” boxes on traditional 12-lead ECGs) increased the performance of the WS technique (AUROC 0.60, sensitivity 0.75, specificity 0.37), as shown in Supplementary Figure S2. Model performance from combining various techniques together and from inclusion of other visual ECG criteria is shown in Supplementary Tables S4, S5, respectively. Correlations of each technique with LVMI are shown in Supplementary Figure S3.
WS-positive ECG-TTE pairs had a higher risk of cardiovascular mortality [HR 1.18, 95% CI (1.12, 1.24), P < 0.001] and a higher risk of developing any cardiovascular disease [HR 1.29, 95% CI (1.22, 1.36), P < 0.001], myocardial infarction [HR 1.60, 95% CI (1.44, 1.78), P < 0.005], and heart failure [HR 1.24, 95% CI (1.17, 1.32), P < 0.001] (Figure 2). These associations remained statistically significant when using the MMCV instead (Supplementary Figure S4). Analyses for the other LVH detection techniques were also performed, demonstrating that these, except Sokolow–Lyon, were also associated with higher risk of mortality and development of CVD, MI, and HF to similar degrees as the WS technique (Supplementary Figures S5–S8 and Supplementary Table S6).
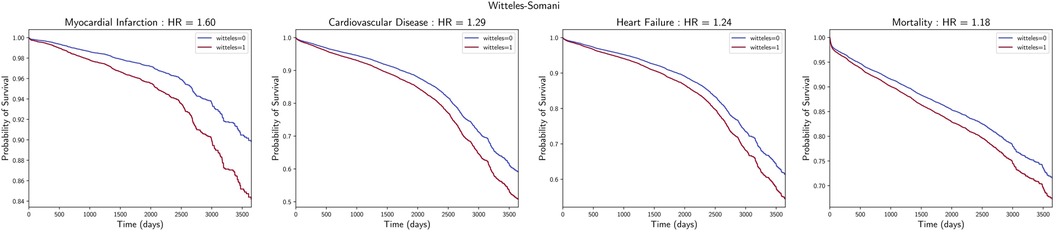
Figure 2. Association with cardiovascular outcomes. Survival curves of incidental myocardial infarction, cardiovascular disease, heart failure, and mortality, with associated hazard ratios and P-values for those with and without LVH, as detected by the WS technique.
Discussion
In this brief report, we describe a novel technique for LVH detection from the ECG which can be performed with rapid visual assessment. We evaluate the performance of this technique, along with those of the Sokolow–Lyon, Cornell, and Peguero–Lo Presti techniques, on a large ECG-TTE linked dataset. We find that the WS technique has similar test characteristics as other commonly employed ECG-based techniques and is simpler to remember and implement. In addition, the WS technique criteria had a statistically significant correlation with LVMI and is associated with 10-year cardiovascular mortality and development of myocardial infarction, heart failure, and any cardiovascular disease.
Our work leverages a large institutional dataset to improve assessment of established LVH criteria and our novel WS criteria. The dataset in this study, composed of over 123,000 ECGs and 35,000 TTEs across over 30,000 patients, is much larger than the datasets used in other primary validation studies of LVH criteria (3). In our dataset, we found lower sensitivities for the three established LVH techniques than those reported in literature elsewhere. By nature of its larger size, our dataset may have captured a broader spectra of disease manifestations, such as varying degrees of hypertensive heart disease, and potentially other confounders of elevated LV mass on TTE but without LVH, such as infiltrative myocardial diseases (e.g., amyloidosis). The decreased incidence of hypertension in the WS-positive group may reflect capture of individuals with high voltages without the presence of LVH, as seen in young athletes for whom lateral ST-depressions and T-wave inversions are stronger discriminatory features (11). While sensitivity analyses on the WS technique failed to show an improvement in performance by incorporating these repolarization features, adjustment for female sex modestly increased the overall performance, at the cost of increasing the technique's complexity.
The WS technique is unique in that it relies on either high R- and S-voltages between adjacent leads, which favors high-amplitude isoelectric signals that likely reflect myocardial hypertrophy (barring longer chest-wall to myocardial distances, which may be present in obese patients or those with obstructive lung disease). Other techniques, by focusing on R and S waves in non-adjacent leads, can embed other contextual information. For example, a prominent S-wave in V4, a critical component of the Peguero–Lo Presti technique, may reflect delayed R-wave progression, the differential for which includes LVH and HCM.
Effective screening techniques for LVH require a highly sensitive, moderately specific, and low-cost solution and remain an active area of research. The WS technique detects LVH more sensitively at the cost of lower specificity, both of which are further enhanced when adjusting for sex (Supplementary Figure S2). Combinatorial evaluation of all LVH techniques did not reveal one combination that maximized sensitivity without a corresponding drop in specificity (Supplementary Table S4). Incorporating other diagnostic ECG findings known to correlate with LVH, such as left atrial enlargement, ST-depressions, and T-wave inversions, increased the total specificity, but with a significant drop in sensitivity (Supplementary Table S5). While beyond the scope of this present work, future work may investigate the impact of combining these criteria with elements from clinical history (e.g., demographics, comorbidities), physical exam findings (e.g., S4 on cardiac exam), and laboratory findings (e.g., brain natriuretic peptide, troponin) on LVH screening, as well as the cost effectiveness and clinical impact of a LVH screening pipeline. Ultimately, the performance for LVH detection across all techniques was modest. Artificial intelligence techniques like deep learning have demonstrated the potential to improve LVH detection on ECG, which far outperforms these criteria (12, 13). However, until these models can be made widely accessible to use or become naturally embedded in pre-existing ECG machines, manual techniques like the ones in this manuscript remain the likely most commonly utilized option for evaluating LVH from an ECG.
Ultimately, LVH has a long history of carrying prognostic value, which continues to fuel the development of techniques to increase its detection. Despite similar percentages HF, CAD, ASCVD, and stroke in the WS-positive and WS-negative groups, and surprisingly decreased proportions of hypertension and diabetes in the WS-positive group, WS-positive patients had higher age- and sex-adjusted risk of incidental cardiovascular outcomes and mortality, continuing to corroborate the importance of this technique and LVH as independent risk factors for cardiovascular disease. Dissecting the etiological and genetic mechanisms that drive the resulting radiomic and electrical phenotypes of LVH remain an area of active research, which may improve current prognostic understandings of LVH to specific subpopulations and guide more targeted therapy.
Conclusion
In conclusion, we demonstrate a novel and simplified ECG technique to detect LVH, and measure its performance, along with established LVH techniques, on the largest ECG-TTE linked dataset for this task. The WS technique, which relies on the simple assessment of visually overlapping QRS complexes in the precordial leads, is comparatively easier to remember, faster to perform, and has similar performance to established techniques.
Data availability statement
The datasets presented in this article are not readily available because; Personally identifiable health data that is currently limited to the authors of the article and those covered under the IRB. Requests to access the datasets should be directed to; SS,c3NvbWFuaUBzdGFuZm9yZC5lZHU=.
Ethics statement
The studies involving humans were approved by Stanford University Institutional Review Board (IRB-41045). The studies were conducted in accordance with the local legislation and institutional requirements. Written informed consent for participation was not required from the participants or the participants’ legal guardians/next of kin in accordance with the national legislation and institutional requirements.
Author contributions
SS, JH, and MP had full access to all the data in the study and takes responsibility for the integrity of the data and the accuracy of the data analysis. Concept and design: SS and RW. Acquisition, analysis, or interpretation of data: SS, JH, EA, and MP. Drafting of the manuscript: SS. Critical revision of the manuscript for important intellectual content: SS, JH, RW, EA, and MP. Statistical analysis: SS. Obtained funding: EA and MP. Supervision: EA, RW, and MP. All authors contributed to the article and approved the submitted version.
Funding
MVP is employed as a consult by Apple Inc and receives funding from NIH/NHLBIR01HL136390. All authors declare that the research was conducted in the absence of any relevant commercial or financial relationships that could be construed as potential conflicts of interest.
Conflict of interest
EA and MP reports consulting fees from Apple Inc., Boston Scientific, Biotronik Inc., Bristol Myers Squibb, ReadMyECG, Johnson & Johnson, and has an equity interest in ReadMyECG. Stanford University has filed a provisional patent on the described work.
The remaining authors declare that the research was conducted in the absence of any commercial or financial relationships that could be construed as a potential conflict of interest.
Publisher's note
All claims expressed in this article are solely those of the authors and do not necessarily represent those of their affiliated organizations, or those of the publisher, the editors and the reviewers. Any product that may be evaluated in this article, or claim that may be made by its manufacturer, is not guaranteed or endorsed by the publisher.
Supplementary material
The Supplementary Material for this article can be found online at: https://www.frontiersin.org/articles/10.3389/fcvm.2023.1251511/full#supplementary-material
References
1. Nakamura M, Sadoshima J. Mechanisms of physiological and pathological cardiac hypertrophy. Nat Rev Cardiol. (2018) 15(7):387–407. doi: 10.1038/s41569-018-0007-y
2. Levy D, Garrison RJ, Savage DD, Kannel WB, Castelli WP. Prognostic implications of echocardiographically determined left ventricular mass in the framingham heart study. N Engl J Med. (1990) 322(22):1561–6. doi: 10.1056/NEJM199005313222203
3. Tavares CDAM, Samesima N, Hajjar LA, Godoy LC, Padrão EMH, Lazar Neto F, et al. Clinical applicability and diagnostic performance of electrocardiographic criteria for left ventricular hypertrophy diagnosis in older adults. Sci Rep. (2021) 11(1):11516. doi: 10.1038/s41598-021-91083-9
4. Somani S, Russak AJ, Richter F, Zhao S, Vaid A, Chaudhry F, et al. Deep learning and the electrocardiogram: review of the current state-of-the-art. Europace. (2021) 10. doi: 10.1093/europace/euaa377
5. Haug CJ, Drazen JM. Artificial intelligence and machine learning in clinical medicine, 2023. N Engl J Med. (2023) 388(13):1201–8. doi: 10.1056/NEJMra2302038
6. Devereux RB, Alonso DR, Lutas EM, Gottlieb GJ, Campo E, Sachs I, et al. Echocardiographic assessment of left ventricular hypertrophy: comparison to necropsy findings. Am J Cardiol. (1986) 57(6):450–8. doi: 10.1016/0002-9149(86)90771-X
7. Sokolow M, Lyon TP. The ventricular complex in left ventricular hypertrophy as obtained by unipolar precordial and limb leads. Am Heart J. (1949) 37(2):161–86. doi: 10.1016/0002-8703(49)90562-1
8. Devereux RB, Casale PN, Eisenberg RR, Miller DH, Kligfield P. Electrocardiographic detection of left ventricular hypertrophy using echocardiographic determination of left ventricular mass as the reference standard. Comparison of standard criteria, computer diagnosis and physician interpretation. J Am Coll Cardiol. (1984) 3(1):82–7. doi: 10.1016/S0735-1097(84)80433-7
9. Peguero JG, Lo Presti S, Perez J, Issa O, Brenes JC, Tolentino A. Electrocardiographic criteria for the diagnosis of left ventricular hypertrophy. J Am Coll Cardiol. (2017) 69(13):1694–703. doi: 10.1016/j.jacc.2017.01.037
10. Christov II. Real time electrocardiogram QRS detection using combined adaptive threshold. Biomed Eng Online. (2004) 3(1):28. doi: 10.1186/1475-925X-3-28
11. Bent RE, Wheeler MT, Hadley D, Knowles JW, Pavlovic A, Finocchiaro G, et al. Systematic comparison of digital electrocardiograms from healthy athletes and patients with hypertrophic cardiomyopathy. J Am Coll Cardiol. (2015) 65(22):2462–3. doi: 10.1016/j.jacc.2015.03.559
12. Kwon JM, Jeon KH, Kim HM, Kim MJ, Lim SM, Kim K-H, et al. Comparing the performance of artificial intelligence and conventional diagnosis criteria for detecting left ventricular hypertrophy using electrocardiography. Europace. (2020) 22(3):412–9. doi: 10.1093/europace/euz324
Keywords: ECG, LVH, LVH detection, echo, left ventricular hypertrophy
Citation: Somani S, Hughes JW, Ashley EA, Witteles RM and Perez MV (2023) Development and validation of a rapid visual technique for left ventricular hypertrophy detection from the electrocardiogram. Front. Cardiovasc. Med. 10:1251511. doi: 10.3389/fcvm.2023.1251511
Received: 1 July 2023; Accepted: 8 August 2023;
Published: 23 August 2023.
Edited by:
Bert Vandenberk, University Hospitals Leuven, BelgiumReviewed by:
Weichieh Lee, Chi Mei Medical Center, TaiwanAgeliki Laina, National and Kapodistrian University of Athens, Greece
© 2023 Somani, Hughes, Ashley, Witteles and Perez. This is an open-access article distributed under the terms of the Creative Commons Attribution License (CC BY). The use, distribution or reproduction in other forums is permitted, provided the original author(s) and the copyright owner(s) are credited and that the original publication in this journal is cited, in accordance with accepted academic practice. No use, distribution or reproduction is permitted which does not comply with these terms.
*Correspondence: Sulaiman Somani c3NvbWFuaUBzdGFuZm9yZC5lZHU=