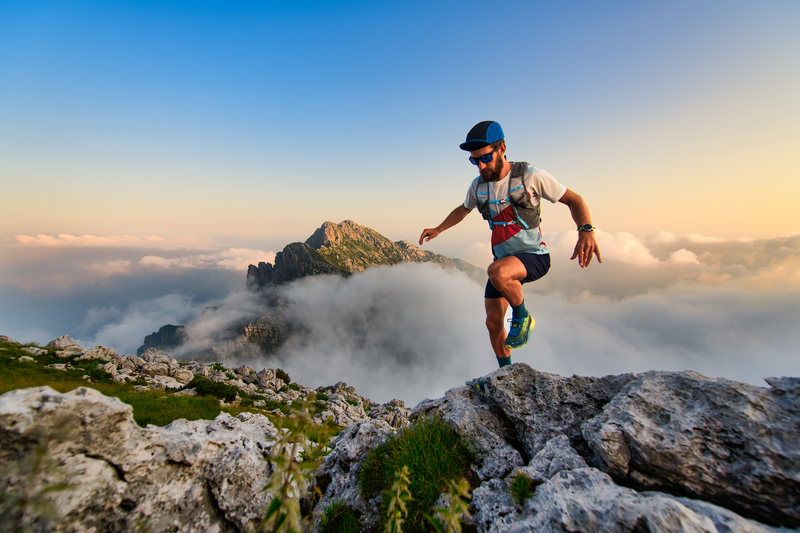
95% of researchers rate our articles as excellent or good
Learn more about the work of our research integrity team to safeguard the quality of each article we publish.
Find out more
ORIGINAL RESEARCH article
Front. Cardiovasc. Med. , 06 September 2023
Sec. Thrombosis and Haemostasis
Volume 10 - 2023 | https://doi.org/10.3389/fcvm.2023.1224462
This article is part of the Research Topic Examining Genetic and Epigenetic Regulation in Cardiovascular Development, Regeneration and Disease View all 10 articles
Background: Venous thrombosis (VT) is multifactorial trait that contributes to the global burden of cardiovascular diseases. Although abundant single nucleotide polymorphisms (SNPs) provoke the susceptibility of an individual to VT, research has found that the five most strongly associated SNPs, namely, rs6025 (F5 Leiden), rs2066865 (FGG), rs2036914 (F11), rs8176719 (ABO), and rs1799963 (F2), play the greatest role. Association and risk prediction models are rarely established by using merely the five strongly associated SNPs. This study aims to explore the combined VT risk predictability of the five SNPs and well-known non-genetic VT risk factors such as aging and obesity in the Hungarian population.
Methods: SNPs were genotyped in the VT group (n = 298) and control group (n = 400). Associations were established using standard genetic models. Genetic risk scores (GRS) [unweighted GRS (unGRS), weighted GRS (wGRS)] were also computed. Correspondingly, the areas under the receiver operating characteristic curves (AUCs) for genetic and non-genetic risk factors were estimated to explore their VT risk predictability in the study population.
Results: rs6025 was the most prevalent VT risk allele in the Hungarian population. Its risk allele frequency was 3.52-fold higher in the VT group than that in the control group [adjusted odds ratio (AOR) = 3.52, 95% CI: 2.50–4.95]. Using all genetic models, we found that rs6025 and rs2036914 remained significantly associated with VT risk after multiple correction testing was performed. However, rs8176719 remained statistically significant only in the multiplicative (AOR = 1.33, 95% CI: 1.07–1.64) and genotypic models (AOR = 1.77, 95% CI: 1.14–2.73). In addition, rs2066865 lost its significant association with VT risk after multiple correction testing was performed. Conversely, the prothrombin mutation (rs1799963) did not show any significant association. The AUC of Leiden mutation (rs6025) showed better discriminative accuracy than that of other SNPs (AUC = 0.62, 95% CI: 0.57–0.66). The wGRS was a better predictor for VT than the unGRS (AUC = 0.67 vs. 0.65). Furthermore, combining genetic and non-genetic VT risk factors significantly increased the AUC to 0.89 with statistically significant differences (Z = 3.924, p < 0.0001).
Conclusions: Our study revealed that the five strongly associated SNPs combined with non-genetic factors could efficiently predict individual VT risk susceptibility. The combined model was the best predictor of VT risk, so stratifying high-risk individuals based on their genetic profiling and well-known non-modifiable VT risk factors was important for the effective and efficient utilization of VT risk preventive and control measures. Furthermore, we urged further study that compares the VT risk predictability in the Hungarian population using the formerly discovered VT SNPs with the novel strongly associated VT SNPs.
Venous thrombosis (VT) is one of the three leading causes of cardiovascular disease (CVD)-related mortality with a significant genetic predisposition (1–3). It is a multifactorial trait that contributes to the global burden of CVD (4–7). In Europe, although overall CVD-related morbidity is decreasing, mortality remains substantially high. CVD is the leading cause of mortality in Europe, accounting for over 3.9 million deaths annually (8–10). Furthermore, approximately 60 million CVD premature deaths (death < 70 years) have been reported in Europe (10).
VT is a major health problem with a significant annual incidence (7.62/100,000) and mortality (3.70/100,000) (11). A higher burden of CVD-related mortality has been reported in Central and Eastern Europe (8, 12). Hungary shares the highest proportion of this mortality (8, 13). CVDs remain the most prominent cause of death in Hungary (13). As of 2014, approximately 35,000 women and 27,000 men have died from CVDs annually, accounting for 55% and 45% of all deaths for women and men, respectively (13). The age-standardized CVD death rate in Hungary is more than double the European Union (EU) average reported in 2014 (13). The availability of prophylaxis could significantly avert this burden by targeting high-risk individuals for treatment (11, 14). According to Rudolf Virchow's triad explanation (15), thrombosis is the result of three major factors, namely, blood flow stasis (16, 17), endothelial injury (18–20), and hypercoagulability (21). The inheritable prothrombotic factors influence VT risk via the coagulation process (21, 22), whereas the non-inheritable risk factors influence VT risk either via stasis or endothelial injury (23, 24).
Various studies have established the impact of heritable factors on VT risk (25–27). The incidence of repeated hospitalization due to VT is twofold higher in people with affected families than that in the general population (1–3). Although abundant single nucleotide polymorphisms (SNPs) provoke the susceptibility of an individual to VT (28–31), research has found that the five most strongly associated SNPs, namely, rs6025 (Leiden mutation) in the F5 gene, rs1799963 (prothrombin G20210A) in the coagulation factor 2 gene (F2), rs8176719 (non-O blood type) in the ABO gene, rs2036914 in the coagulation factor eleven gene (F11), and rs2066865 in the fibrinogen gamma gene (FGG), play the greatest role in determining VT incidence and recurrence in genetically vulnerable individuals (29, 32, 33).
The Leiden mutation is one of the most dominant inheritable VT risk factors that increase the burden of VT in genetically vulnerable individuals (34–36). The Leiden mutation/F5 prevalence is unevenly distributed across Europe with an average prevalence of 4% in the general population. The highest frequency is reported in Southeastern Europe and Northern Europe, whereas the lowest frequency is reported in Eastern and Western Europe (37, 38). The Leiden mutation prevalence is highest in European descent populations (3%–8%) (34, 39), followed by Caucasian Americans (5%). However, it is highly rare in African Americans (1.2%) and Asian-Americans (0.45%) (34) and absent in Africans (40, 41). Similarly, the prothrombin gene mutation/F2, often known as the G20210A mutation, is the second most prevalent inheritable VT risk in Caucasians (2%–4%) (39), particularly those of European ancestry (4%) and Caucasian Americans (2%). However, it is less prevalent in African Americans, accounting for approximately 0.4% (one in 250), and highly rare in Africans and Asians (39, 42).
Often, due to their coexistence and possible gene‒gene interaction, the prothrombin gene mutation (rs1799963) and Leiden mutation (rs6025) SNPs were studied together (43). Furthermore, studies showed that the ancestral distribution of coagulation factor 11 (rs2036914) is similar in both Caucasians and African Americans (44, 45). Studies indicated that O blood-type individuals are at lower risk of VT than non-O blood-type individuals (46, 47), who are at a higher risk of VT (48–51). In addition, Kinsella et al. reported that the risk of venous thromboembolism (VTE) is higher in African Americans and non-O blood-type individuals than that in Caucasians and O blood-type individuals (52).
An individual who is a carrier of multiple variants is more vulnerable to VT. Studies have indicated that the combination of strongly associated VT SNPs [rs6025 (F5), rs1799963 (F2), rs8176719 (ABO), rs2036914 (F11), and rs2066865 (FGG)] poses a greater VT risk than that risk occurring by an individual SNP (29, 53). The genetic risk score (GRS) of strongly associated VT variants results in the greatest risk compared with a larger number of SNP combinations. De Haan et al. (29) showed that the VT risk prediction of the 5-SNP risk score is equivalent to that of the 31-SNP risk score.
In addition, studies have indicated that dual exposure to VT risk factors (genetic and non-genetic) increases the susceptibility of an individual to VT (4, 29). Aging and obesity are well-known non-inheritable VT risk factors that hasten the onset of VT (29). As a result of multiple anatomical and pathophysiological changes, the elderly are prone to age-related cardiovascular morbidity and mortality (54–56). Aging plays a major role in the higher incidence of VT risk (1%) in elderly individuals (19, 54–57). The diminished efficiency of the calf muscle pump due to aging could lead to peripheral blood reflux and stasis resulting in thrombosis formation (54). Furthermore, age-related endothelial dysfunction is also a contributing factor to the higher incidence of VT in elderly individuals compared with that in younger individuals (19). Valve thickness, muscle fiber atrophy, and reduced endothelial anticoagulant properties are some pathophysiological changes that increase the VT risk among elderly individuals (54, 55). Correspondingly, obese individuals are at higher VT risk than normal-weight individuals. Previously conducted studies showed that the VT risk was two- to sixfold higher in obese individuals than that in normal-weight individuals [body mass index (BMI) = 20–24.9 kg/m2] (58–62). A study indicated that the VT risk was higher among aged (>50 years old) and obese individuals [61).
Stratifying higher-risk individuals based on their genetic profiling for thromboprophylaxis is important for efficient utilization of the available resources (29). Furthermore, the possibility of reducing unexpected consequences of massive supplementation of prophylactic treatment would be high (63). Although the 5-SNP impact on VT risk is huge, association and risk prediction models are rarely established by using merely five strongly associated SNPs. No study has yet been conducted to explore the VT risk predictability of the combined five strongly associated prothrombotic SNPs in the VT subjects from the Hungarian population. Consequently, this study aims to explore the VT risk predictability of the combined five SNPs [rs6025 (F5 Leiden), rs2066865 (FGG), rs2036914 (F11), rs8176719 (ABO), and rs1799963 (F2)] in the Hungarian population.
A total of 698 subjects were involved in the case‒control study, of which 298 were VT patients and 400 were healthy controls. The VT patients were recruited consecutively by the Division of Clinical Laboratory Science, Department of Laboratory Medicine, Faculty of Medicine, University of Debrecen during a 1-year period. VT diagnosis was established by standard diagnostic modalities, such as color Doppler ultrasound and phlebography at the Department of Internal Medicine. The controls were selected from the general population via a comprehensive health survey (see survey details and the created database elsewhere) and were free from VT according to a self-report questionnaire conducted 12 months prior to the survey (64).
DNA was extracted from the peripheral blood using a MagNA Pure LC system (Roche Diagnostics, Basel, Switzerland) with a MagNA Pure LC DNA Isolation Kit–Large Volume according to the manufacturer's instructions. The extracted DNA was eluted in a 200 μl MagNA Pure LC DNA Isolation Kit–Large Volume elution buffer.
Based on the genome-wide association study (GWAS) results (30, 65, 66) and our previously conducted studies (4, 67), we identified and considered the five strongly associated prothrombotic SNPs, namely, rs6025 (F5), rs2066865 (FGG), rs2036914 (F11), rs8176719 (ABO), and rs1799963 (F2), in our current study. We considered them due to their confirmed large effect size and potential predictability of inheritable VT risk (29, 67). The assay design and genotyping were performed by the Karolinska University Hospital, Stockholm, Sweden, Mutation Analysis Core Facility (MAF). A MassARRAY platform (Sequenom, CA, USA) with iPLEX Gold chemistry was used for genotyping. Quality control, validation, and concordance analysis were conducted by the MAF.
The weighted GRS (wGRS) and unweighted GRS (unGRS) were computed to identify the combined effect of the included SNPs on VT risk. In the GRS, the individuals were assigned based on the total number of risk-increasing alleles. Consequently, “0,” “1,” and “2” codes were given for the absence, heterozygosity, and homozygosity of risk alleles, respectively. When the risk allele was found to be protective, the coding for the homozygous risk allele became “0” and “2” for the other homozygous allele (67). Accordingly, the unGRS was simply calculated by adding all risk alleles in a given locus with the assumption that all alleles had the same effect. To comprehend the stronger relationship of some SNPs with VT, we also calculated the wGRS by assigning weights to the risk allele of each SNP corresponding to the logarithm of the average risk estimates reported in the previously conducted genetic association study (29).
Moreover, to determine which SNP is more influential in its discriminatory accuracy of the area under the receiver operating characteristic curve (AUC), we added each SNP one by one into a model. Therefore, we started with the SNP with the highest odds ratio (OR), i.e., the Leiden mutation (rs6025) in the F5 gene, and assessed whether adding more SNPs in a model could improve the AUC. We continued adding all other SNPs into a model until we verified that adding more SNPs into a model could not reveal any significant discriminatory accuracy.
We considered age (≥60 years), sex, and obesity (BMI ≥ 30 kg/m2) as non-genetic VT risk factors. We included each non-genetic risk factor and their combination with genetic VT risk factors into a model to verify the difference in the AUC and their VT risk predictability in the study population. A logistic regression model was used to generate a combined risk score of genetic and non-genetic VT risk factors.
Statistical tests were computed using the PLINK (version 1.9) and IBM SPSS (version 26.0) statistical software. The Mann‒Whitney U-test was used to compare the age, BMI, and GRS distribution in the study population. The Shapiro–Wilk normality test was used to test the distribution of quantitative variables. The Hardy–Weinberg equilibrium (HWE) and risk allele frequency differences between the VT group and control group were estimated using the X2 test. The association between the five SNPs and VT risk was assessed by the OR with their respective 95% confidence interval (CI) under all genetic models, namely, the multiplicative, additive, dominant, recessive, and genotypic models. Likewise, a logistic regression analysis was also used to compute the OR with 95% of individual SNPs and genetic, non-genetic, and combined VT risk factors.
In addition, the area under the receiver operating characteristic (ROC) curve was determined to assess how well its score classifies the VT group and control group. In general, the AUC ranged from 0.5 (no discrimination between the VT group and control group) to 1.0 (perfect discrimination). We compared the AUCs of the genetic, non-genetic, and combined risk models. The SPSS IBM version 26.0 was used to calculate the ROC curves and AUCs. The Bonferroni multiple testing correction was employed to prevent multiple comparison problems (0.05/5, p < 0.01). Statistically significant variables were declared at a conventional p-value of 0.05.
The Hungarian Scientific Council on Health Research committee approved the protocol (61327-2017/EKU). All participants provided written consent before their participation.
In total, 698 subjects were enrolled in the case–control study, of which 298 were VT patients and 400 were healthy controls. All subjects with complete genotypic and covariate data were considered for the analyses. The proportion of male participants (51%) in the VT group was higher than that in the control group (44%). The age distribution of the VT group was shifted toward the elderly group, and their mean age was significantly higher than that of the control group (63.4 ± 16.4 vs. 43.8 ± 12.6 years, p < 0.001) (Supplementary Figure S1). However, the distribution of BMI values (kg/m2) did not differ significantly (28.2 ± 8.2 vs. 27.2 ± 5.5, p = 0.76). The marker check and detailed information of each SNP, including rs (SNP identifier), base pair position (BP), chromosome number (CHR), and major and minor alleles, are listed in Table 1.
The genotypic results were available for 698 subjects: VT patients (n = 298) and healthy controls (n = 400). All SNPs were tested to determine whether the observed allele frequencies were in accordance with the HWE; no significant deviation from the HWE was detected in the study population (Table 1). The risk allele frequencies of the five prothrombotic SNPs analyzed in the study are listed in Table 2. The risk allele frequencies of rs6025 (F5), rs2036914 (F11), and rs8176719 (ABO) were higher in the VT group than those in the control group, and the differences remained statistically significant after multiple testing correction was performed (p < 0.01) (Table 2).
Table 2. Risk allele frequency comparison of the VT group and control group in the Hungarian population.
In addition, we also computed the protective allele frequency of the ABO gene (DEL); its frequency was higher in the control group than that in the VT group (Supplementary Table S1).
The association strengths regarding VT risk using complete genetic association models (multiplicative, additive, dominant, recessive, and genotypic models) were estimated. Only the Leiden mutation (rs6025) and F11 (rs2036914) remained significant after adjustment for multiple testing correction (p < 0.01). In particular, the Leiden mutation variant strongly influenced the VT risk in the Hungarian population (p < 0.001): among the patients with VT due to the Leiden mutation, the OR of VT risk ranged from 3.25 (heterozygous genotypic for risk variant; OR = 3.25, 95% CI: 2.22–4.76) to 19.67 (OR = 19.6, 95% CI: 2.57–150.4) in the recessive model/ those who were homozygous for risk variant.
The rs8176719 (ABO) remained statistically significant only in the multiplicative (OR = 1.33, 95% CI: 1.07–1.64) and genotypic models (OR = 1.77, 95% CI: 1.14–2.73); nevertheless, it lost its significance in other models after adjustment for multiple testing correction. Similarly, the rs8176719 (ABO) protective variant remained statistically significant only in the multiplicative model (OR = 0.75, 95% CI: 0.61–0.93) (Supplementary Table S2). In addition, the FGG (rs2066865) expressed a significant association with VT risk in the multiplicative, additive, and dominant models before multiple testing correction; however, it lost its significance after adjustment was performed. Conversely, the F2 (rs1799963) did not show any statistically significant association with VT directly with any of the used models (Table 3).
Table 3. Genetic association test results in the VT group and control group of the study population: implication to determine the inheritable VT disease risk factors in the Hungarian population.
The unGRS and wGRS of the five SNPs were computed for the 298 VT patients and 400 healthy controls. The unGRS ranged from 0 to 6 (3.46 ± 1.31) and 0 to 7 (2.77 ± 1.28) for the VT and control groups, respectively (Figure 1A). The wGRS ranged from 0 to 4.6 (1.93 ± 0.97) and 0 to 4.7 (1.37 ± 0.78) for the VT and control groups (Figure 1B).
Figure 1. Unweighted (A) and weighted (B) GRS distribution comparison among the VT group and the control group of the Hungarian population.
The distributions of other covariate variables including the wGRS and unGRS were significantly distinct (p < 0.001) between the two groups (Supplementary Table S3). The test revealed significant differences (case vs. control) in age (median = 65; 44, p < 0.001), BMI (median = 28.72; 26.75, p < 0.001), unGRS (median = 3; 3, p < 0.001), and wGRS (median = 1.79; 1.34, p < 0.001). Although the median unGRS values for the VT group and control group were similar, a larger unGRS value was more frequent in the VT group than in the control group.
Table 4 lists the multivariate logistic regression analysis results of covariate variables adjusted for sex and age. Of the well-known non-genetic VT risk factors, age and obesity were significantly associated with VT risk in the study population, which was higher in the VT group than that in the control group (Table 4). The VT risk was 12.8 times higher in the elderly subjects aged ≥60 years than that among the subjects aged below 60 years (AOR = 12.83, 95% CI: 8.38–19.63). Similarly, the VT risk was 2.3 times higher in the obese subjects (BMI > 30 kg/m2) than that in the normal-weight subjects (AOR = 2.28, 95% CI: 1.51–3.42). Furthermore, the wGRS remained statistically significant after we adjusted for both sex and age (AOR = 2.69, 95% CI: 1.74–4.19 and AOR = 2.24, 95% CI: 1.51–3.32, respectively). However, the unGRS lost its statistical significance (AOR = 0.88, 95% CI: 0.65–1.18) in the multivariate regression analysis model (Table 4).
We calculated the ROC curve to assess how well the score classified VT in the case and control groups. The AUC of the SNPs ranged from 0.51 (95% CI: 0.47–0.55, p = 0.64) for rs1799963 in F2 to 0.62 (95% CI: 0.57; 0.66, p < 0.001) for rs6025 in F5. The discriminative accuracy of the model improved by adding each SNP (Figure 2). We started with the Leiden mutation (rs6025) with the highest effect size and ended with rs2036914 (F11) with the lowest effect size among the five SNPs. The addition of each SNP increased the AUC after F2 (rs1799963).
Figure 2. The change in the discriminatory accuracy of the AUC of the genetic risk score after adding each SNP into a model; we started with the Leiden mutation (rs6025) with the highest effect size and ended with rs2036914 (F11) with the lowest effect size of the five SNPs. The addition of each SNP increases the AUC value after F2 (rs1799963).
The AUC of the 5-SNP risk score was 0.68 (95% CI: 0.64; 0.72). The variability proportion explained by the Leiden mutation (rs6025) was higher than that of the 5-SNP risk score (8% vs. 7%). Furthermore, approximately 39% of the variability observed was attributed to the combination of genetic and non-genetic risk factors, which is higher than that of those factors independently (Table 5). Similarly, the ROC curve for the weighted 5-SNP risk score had an AUC of 0.68 (95% CI: 0.64–0.72), i.e., there was a 68% probability that a randomly selected VT patient will have a higher score than that of a randomly selected control subject. The wGRS was a better predictor for VT than the unGRS (AUC = 0.65, 95% CI: 0.60–0.69).
Table 5. Venous thrombosis risk predictability of the Leiden mutation, genetic risk, non-genetic risk, and combined model in the Hungarian population.
There was a difference between the discriminative accuracy of the 5-SNP risk score in men (AUC = 0.68, 95% CI: 0.62–0.74, p < 0.001) and women (AUC = 0.61: 95% CI: 0.55–0.67, p < 0.001). Moreover, the AUC of the wGRS of the 5-SNPs was significantly higher in men (AUC = 0.71, 95% CI: 0.65–0.76, p < 0.001) than that in women (AUC = 0.63, 95% CI: 0.57–0.69, p < 0.001).
We also evaluated the discriminative accuracy of well-known non-genetic VT risk factors such as age, sex, and obesity to explore their independent and combined VT risk predictability in the study population. The independent AUCs of age and obesity were 0.84, p < 0.0001, and 0.59, p < 0.001, respectively. The combination of all well-known VT risk factors changed the discriminative accuracy of the AUC to 0.85, p < 0.000. Similarly, when we added the non-genetic risk factors into the genetic risk factors, the AUC significantly projected to 0.89 (95% CI: 0.86–0.91) compared to that in the genetic (AUC = 0.68) or non-genetic risk factor predictability (AUC = 0.85; p < 0.0001) (Figure 3). The AUC difference in the combined and non-genetic risk factors was statistically significant (AUC = 0.039, 95% CI: 0.02–0.059, p < 0.0001). There was no significant AUC difference between men and women in the non-genetic (men: AUC = 0.81, 95% CI: 0.76–0.86 vs. women: AUC = 0.82, 95% CI: 0.78–0.87) and combined risk score (men: AUC = 0.87, 95% CI: 0.83–0.91 vs. women: AUC = 0.86, 95% CI: 0.82–0.90) models.
Figure 3. ROC curves for combined (genetic and non-genetic), genetic (five prothrombotic SNPs), and non-genetic (age, sex, BMI) risk models.
Although there are abundant SNPs that provoke VT events in genetically vulnerable individuals, the contributions of the five strongly associated SNPs, namely, rs6025 (F5), rs2066865 (FGG), rs2036914 (F11), rs8176719 (ABO), and rs1799963 (F2), to VT risk is immensely high (29, 32, 33). Moreover, previously conducted studies demonstrated the importance of these five prothrombotic SNPs in the relapse of inheritable VT (29, 32, 33). Consequently, we aimed to explore the combined genetic risk predictability of strongly associated VT SNPs and well-known non-genetic VT risk factors in the Hungarian population. Thus, stratifying high-risk individuals based on their genetic profiling might help for the efficient utilization of scarcely available thromboprophylaxis, which might reduce the premature death attributed to VT and CVDs.
In our present study, we considered five VT-associated SNPs to explore the genetic background of VT risk in the Hungarian population. Only the three SNPs, namely, rs6025 (F5), rs2036914 (F11), and rs8176719 (ABO), remained statistically significant after adjustment for multiple testing correction (p < 0.01). The highest VT risk was detected among the Leiden mutation carriers/rs6025 (OR = 3.52, 95% CI: 2.50–4.95). Its allele frequency was approximately threefold higher in the VT group (20%) than that in the control group (6.8%). Our findings were consistent with the finding of the previously conducted studies showing that the odds of VT risk are 3.5 (28) and 4.38 times higher for rs6025 (F5) variant carriers than those of non-carriers (68).
Moreover, numerous studies suggest that the Leiden mutation is vastly prevalent in Caucasians, particularly those of European descent. It is one of the most influential inheritable VT risk factors that increase the burden of VT in genetically vulnerable individuals (34–36). These findings support our study’s result that F5 is highly prevalent in the case group (20%). Furthermore, it was strongly associated with the trait in all genetic association models. This highlights the fact that the Leiden mutation is an independent predictor of VT risk (28, 35, 69) and its contribution to the burden of VT is remarkable (69), particularly for genetically susceptible individuals and Caucasians (34). Likewise, the risk allele frequency and VT risk for rs2036914 (F11) and rs8176719 (ABO) were higher in the case group even after adjustment for multiple testing correction. A previously conducted study revealed that F11 (rs2036914) is an independent predictor of VT (70), which is supported by our result that the risk was 1.38 times higher in the case group than that in the control group. Studies showed that VT risk distribution due to F11 (rs2036914) is similar in Caucasians and African Americans (44, 45).
The allele frequency of the ABO SNP (rs8176719) was more prevalent in the control group (47.4% vs. 40.4%). In addition, it was revealed that the VT risk was lower (OR = 0.75, 95% CI: 0.61–0.93) among the subjects with the rs8176719 variant. Furthermore, the VT risk was 1.33 times higher in the risk variant carriers. Studies indicated that the O blood-type individuals are at a lower VT risk compared with the non-O blood-type individuals (46, 47). On the other hand, several studies showed that non-O blood-type individuals (A, AB, and B) were at a higher VT risk compared with non-O blood-type individuals (48–51). Our findings were also consistent with those of the previously conducted studies. Fang et al. reported that the VTE risk is higher in African Americans and non-O blood-type individuals than that in Caucasians and O blood-type individuals (52).
Although the risk allele frequencies and VT risk were not distinct in the case of prothrombin mutation (rs1799963), it was the second most prevalent risk variant in the Hungarian population. The reason for the lack of statistical significance despite the large OR might be attributed to the limited number of our VT patients. Our findings were also in line with those of the previously conducted studies showing that rs1799963 is more prevalent in European descent populations than in others (Americans, African Americans, Asians, and Africans) (23). Several studies showed that approximately two- to fourfold VT risk was attributed to a hypercoagulability state that resulted from a mutation in the prothrombin gene/rs1799963 (35, 71, 72).
Studies indicated that pooled variants have more impact on VT risk determination than a single variant (29, 53); consequently, we computed the wGRS and unGRS to determine the VT risk in the study population. Our findings showed that the wGRS is an independent predictor of VT risk in the study population, and its value was 2.37 times higher in the VT group than in the control group. Previously conducted studies also supported our findings (29, 53).
The impact of non-genetic risk factors on VT risk is also appreciable. Our study showed that VT was more prevalent in elderly (≥ 60 years) subjects (58.1% vs. 10.5%; p < 0.0001). Likewise, the odds of VT risk for elderly subjects were 12-fold higher than that of those aged <60 years. The VT risk increases with age due to different factors, such as anatomical (54), pathophysiological (54–57), and hormonal derangement (73). Consequently, it hastens and increases the vulnerability of elderly subjects to VT risk and other CVDs (19, 55–57). Furthermore, our findings showed that the VT risk is 2.28 times higher in the obese subjects than that in the normal-weight subjects. This finding is in line with those of the previously conducted studies that showed that obesity is an independent predictor of VT risk (58–62).
The ability to predict the risk of a certain event before its occurrence is important in clinical epidemiology. Precise risk prediction helps control an event at as early a stage as possible (74–77) and offers to use the available resources effectively and efficiently (74–77). We used the ROC curves to establish individual and combined VT risk predictability of the SNPs and non-inheritable VT risk factors to develop a risk stratification tool.
In our study, the highest AUC was obtained for the Leiden mutation (AUC = 0.62), whereas the lowest AUC was obtained for the prothrombin mutation/F2 (0.52). The addition of each SNP into the model after F5 increased the AUC in general. Our finding is in line with that of the studies showing that adding more SNPs into the model increases the AUC to a certain extent, but after a certain level, the AUC does not change, despite adding more explanatory variables into the model (29, 78).
We also found that the wGRS is a better predictor of VT risk than individual SNPs (0.68 vs. 0.62) and their combination with non-genetic risk factors yields a larger AUC with higher discriminatory accuracy (AUC = 0.89). This finding is consistent with that of the previous studies showing that the combination of clinical and genetic risk factors increases the VT risk eight times more than either the genetic or the clinical model alone (79, 80).
Our study tried to verify the VT risk predictability in the Hungarian population only by using strongly associated VT SNPs [rs6025 (F5), rs1799963 (F2), rs8176719 (ABO), rs2036914 (F11), and rs2066865 (FGG)] mainly relating to the recurrence and higher incidence of VT risk and well-known non-genetic VT risk factors (age, obesity, and sex), which helped distinguish the higher-risk individuals for the prevention and control of VT in the study population. Furthermore, our study indicated the possibility of efficiently and effectively utilizing the available resource for risk prediction in the given population. However, our study lacked the comparison of formerly identified strongly associated VT SNPs with the novel loci, which are strongly associated with VT risk as well (66, 81–83). As a result, we urged further study that considers the novel and strongly associated VT SNPs and the formerly identified SNPs and their comparison on the VT risk predictability in the Hungarian population.
Altogether, the Leiden mutation, F11, and ABO risk alleles are highly prevalent and strongly determine the VT risk in the Hungarian population. The pooled genetic risk variants are more influential than a single variant alone. The combined model is the best predictor of VT risk, so stratifying high-risk individuals based on their genetic profiling and well-known non-modifiable VT risk factors is important for the effective and efficient utilization of VT risk preventive and control measures. Furthermore, our study lacks the comparison of formerly identified VT SNPs with the novel SNPs, which are strongly associated with VT risk. This might provide new insight into the VT risk and its determinants in the Hungarian population. As a result, we urged further study that considers the novel and strongly associated VT SNPs and the formerly identified SNPs and their comparison on the VT risk predictability in the Hungarian population.
The original contributions presented in the study are included in the article/Supplementary Material, further inquiries can be directed to the corresponding author.
The studies involving humans were approved by the Ethical Committee of the University of Debrecen. The studies were conducted in accordance with the local legislation and institutional requirements. The participants provided their written informed consent to participate in this study.
SN: data handling, writing and interpreting the results, and preparing statistical analysis. MM: participation in the statistical analyses. ZB: review, editing, and finalizing the manuscript. JS and RÁ: institutional background and funding framework. SF: guiding the data analyses, developing the methodology, and writing of the manuscript. All authors contributed to the article and approved the submitted version.
This work was supported by the Stipendium Hungaricum Scholarship Programme of the Tempus Public Foundation, the European Union under the European Regional Development Fund (GINOP-2.3.2-15-2016-00005), and the National Research, Development and Innovation Office, Hungarian Ministry of Innovation and Technology (Grant No. OTKA K139293).
The authors declare that the research was conducted in the absence of any commercial or financial relationships that could be construed as a potential conflict of interest.
All claims expressed in this article are solely those of the authors and do not necessarily represent those of their affiliated organizations, or those of the publisher, the editors and the reviewers. Any product that may be evaluated in this article, or claim that may be made by its manufacturer, is not guaranteed or endorsed by the publisher.
The Supplementary Material for this article can be found online at: https://www.frontiersin.org/articles/10.3389/fcvm.2023.1224462/full#supplementary-material
1. Zöller B, Pirouzifard M, Svensson PJ, Holmquist B, Stenman E, Elston RC, et al. Familial segregation of venous thromboembolism in Sweden: a nationwide family study of heritability and complex segregation analysis. J Am Heart Assoc. (2021) 10:24. doi: 10.1161/JAHA.120.020323
2. Zöller B, Li X, Ohlsson H, Ji J, Sundquist J, Sundquist K. Family history of venous thromboembolism as a risk factor and genetic research tool. Thromb Haemost. (2015) 114(5):890–900. doi: 10.1160/TH15-04-0306
3. Zöller B, Ohlsson H, Sundquist J, Sundquist K. Family history of venous thromboembolism (VTE) and risk of recurrent hospitalization for VTE: a nationwide family study in Sweden. J Thromb Haemost. (2014) 12(3):306–12. doi: 10.1111/jth.12499
4. Natae SF, Kósa Z, Sándor J, Merzah MA, Bereczky Z, Pikó P, et al. The higher prevalence of venous thromboembolism in the Hungarian Roma population could be due to elevated genetic risk and stronger gene-environmental interactions. Front Cardiovasc Med. (2021) 8:647416. doi: 10.3389/fcvm.2021.647416
5. Heit JA, Spencer FA, White RH. The epidemiology of venous thromboembolism. J Thromb Thrombolysis. (2016) 41(1):3–14. doi: 10.1007/s11239-015-1311-6
6. Morange PE, Suchon P, Trégouët DA. Genetics of venous thrombosis: update in 2015. Thromb Haemost. (2015) 114(5):910–9. doi: 10.1160/TH15-05-0410
7. Raskob GE, Angchaisuksiri P, Blanco AN, Buller H, Gallus A, Hunt BJ, et al. Thrombosis: a major contributor to global disease burden. Arterioscler Thromb Vasc Biol. (2014) 34(11):2363–71. doi: 10.1111/jth.12698
8. Nichols M, Townsend N, Scarborough P, Rayner M. Cardiovascular disease in Europe: epidemiological update. J Clin Oncol. (2014) 32(21):1–192. doi: 10.1200/JCO.2015.61.5757
9. Tadayon S, Wickramasinghe K, Townsend N. Examining trends in cardiovascular disease mortality across Europe: how does the introduction of a new European standard population affect the description of the relative burden of cardiovascular disease? Popul Health Metr. (2019) 17(1):1–17. doi: 10.1186/s12963-019-0187-7
10. Townsend N, Kazakiewicz D, Lucy WF, Timmis A, Huculeci R, Torbica A, et al. Epidemiology of cardiovascular disease in Europe. Nat Rev Cardiol. (2022) 19(2):133–43. doi: 10.1038/s41569-021-00607-3
11. Cohen AT, Agnelli G, Anderson FA, Arcelus JI, Bergqvist D, Brecht JG, et al. Venous thromboembolism (VTE) in Europe—the number of VTE events and associated morbidity and mortality. Thromb Haemost. (2007) 98(4):756–64. doi: 10.1160/TH07-03-0212
12. Movsisyan NK, Vinciguerra M, Medina-Inojosa JR, Lopez-Jimenez F. Cardiovascular diseases in central and Eastern Europe: a call for more surveillance and evidence-based health promotion. Ann Glob Heal. (2020) 86(1):1–10. doi: 10.5334/aogh.2713
13. OECD/European Observatory on Health Systems and Policies. Hungary: Country Health Profile 2017. Brussels: State of Health in the EU, OECD Publishing, Paris/European Observatory on Health Systems and Policies (2017). 16 p. Available from: http://dx.doi.org/10.1787/9789264283411-en
14. Monreal M, Agnelli G, Chuang LH, Cohen AT, Gumbs PD, Bauersachs R, et al. Deep vein thrombosis in Europe—health-related quality of life and mortality. Clin Appl Thromb. (2019) 25:1–12. doi: 10.1177/1076029619883946
15. Kumar DR, Hanlin ER, Glurich I, Mazza JJ, Yale SH. Virchow’s contribution to the understanding of thrombosis and cellular biology. Clin Med Res. (2010) 8(3–4):168–72. doi: 10.3121/cmr.2009.866
16. Byrnes JR, Wolberg AS. New findings on venous thrombogenesis. Hamostaseologie. (2017) 37(1):25–35. doi: 10.5482/HAMO-16-09-0034
17. Bovill EG, Van Der Vliet A. Venous valvular stasis-associated hypoxia and thrombosis: what is the link? Annu Rev Physiol. (2011) 73:527–45. doi: 10.1146/annurev-physiol-012110-142305
18. Hosseinzadegan H, Tafti DK. Prediction of thrombus growth: effect of stenosis and Reynolds number. Cardiovasc Eng Technol. (2017) 8(2):164–81. doi: 10.1007/s13239-017-0304-3
19. Poredos P, Jezovnik MK. Endothelial dysfunction and venous thrombosis. Angiology. (2018) 69(7):564–7. doi: 10.1177/0003319717732238
20. Motto D. Endothelial cells and thrombotic microangiopathy. NIH Public Access. (2012) 32(2):208–14. doi: 10.1016/j.semnephrol.2012.02.007
21. Monie DD, DeLoughery EP. Pathogenesis of thrombosis: cellular and pharmacogenetic contributions. Cardiovasc Diagn Ther. (2017) 7(Suppl 3):S291–8. doi: 10.21037/cdt.2017.09.11
22. Previtali E, Bucciarelli P, Passamonti SM, Martinelli I. Risk factors for venous and arterial thrombosis. Blood Transfus. (2011) 9(2):120–38. doi: 10.2450/2010.0066-10
23. Huang SS, Liu Y, Jing ZC, Wang XJ, Mao YM. Common genetic risk factors of venous thromboembolism in Western and Asian populations. Genet Mol Res. (2016) 15(1):1–11. doi: 10.4238/gmr.15017644
24. Ashrani AA, Silverstein MD, Lahr BD, Petterson TM, Bailey KR, Melton LJ, et al. Risk factors and underlying mechanisms for venous stasis syndrome: a population-based case-control study. Vasc Med. (2009) 14(4):339–49. doi: 10.1177/1358863X09104222
25. Crous-Bou M, Harrington LB, Kabrhel C. Environmental and genetic risk factors associated with venous thromboembolism. Semin Thromb Hemost. (2016) 42(8):808–20. doi: 10.1055/s-0036-1592333
26. Jaworek T, Xu H, Gaynor BJ, Cole JW, Rannikmae K, Stanne TM, et al. Contribution of common genetic variants to risk of early-onset ischemic stroke. Neurology. (2022) 99(16):E1738–54. doi: 10.1212/WNL.0000000000201006
27. Zöller B, Svensson PJ, Dahlbäck B, Lind-Hallden C, Hallden C, Elf J. Genetic risk factors for venous thromboembolism. Expert Rev Hematol. (2020) 13(9):971–81. doi: 10.1080/17474086.2020.1804354
28. Klarin D, Emdin CA, Natarajan P, Conrad MF, Kathiresan S. Genetic analysis of venous thromboembolism in UK Biobank identifies the ZFPM2 locus and implicates obesity as a causal risk factor. Circ Cardiovasc Genet. (2017) 10(2):1–17. doi: 10.1161/CIRCGENETICS.116.001643
29. De Haan HG, Bezemer ID, Doggen CJ, Cessie SL, Reitsma PH, Arellano AR, et al. Multiple SNP testing improves risk prediction of first venous thrombosis. Blood. (2012) 120(3):656–63. doi: 10.1182/blood-2011-12-397752
30. Klarin D, Busenkell E, Judy R, Lynch J, Levin M, Haessler J, et al. Genome-wide association analysis of venous thromboembolism identifies new risk loci and genetic overlap with arterial vascular disease. Nat Genet. (2019) 51(11):1574–9. doi: 10.1038/s41588-019-0519-3
31. Rinde LB, Morelli VM, Småbrekke B, Mathiesen EB, Løchen ML, Njølstad I, et al. Effect of prothrombotic genotypes on the risk of venous thromboembolism in patients with and without ischemic stroke. The Tromsø Study. J Thromb Haemost. (2019) 17(5):749–58. doi: 10.1111/jth.14410
32. Van Hylckama Vlieg A, Flinterman LE, Bare LA, Cannegieter SC, Reitsma PH, Arellano AR, et al. Genetic variations associated with recurrent venous thrombosis. Circ Cardiovasc Genet. (2014) 7(6):806–13. doi: 10.1161/CIRCGENETICS.114.000682
33. Brotman DJ, Necochea AJ, Wilson LM, Crim MT, Bass EB. Predictive value of factor V Leiden and prothrombin G20210A in adults with venous thromboembolism and in family members of those with a mutation. JAMA. (2009) 301(23):2472–85. doi: 10.1001/jama.2009.853
34. Kujovich JL. Factor v Leiden thrombophilia. Genet Med. (2011) 13(1):1–16. doi: 10.1097/GIM.0b013e3181faa0f2
35. Simone JEB, De Stefano V, Leoncini E, Zacho J, Martinelli I. Risk of venous thromboembolism associated with single and combined effects of factor V Leiden, prothrombin 20210A and methylenetetrahydrofolate reductase C677T: a meta-analysis involving over 11,000 cases and 21,000 controls. Eur J Epidemiol. (2013) 28(8):621–47. doi: 10.1007/s10654-013-9825-8
36. Hosseini S, Kalantar E, Hosseini MS, Tabibian S, Shamsizadeh M, Dorgalaleh A. Genetic risk factors in patients with deep venous thrombosis, a retrospective case–control study on Iranian population. Thromb J. (2015) 13(1):4–9. doi: 10.1186/s12959-015-0064-y
37. Simka M, Latacz P. Factor V Leiden distribution—could it shed some light on the pre-history of Europe and the near east? Phlebol Rev. (2016) 2–3:40–5. doi: 10.5114/pr.2016.65518
38. Clark JSC, Adler G, Salkic NN, Ciechanowicz A. Allele frequency distribution of 1691G>A F5 (which confers factor V Leiden) across Europe, including Slavic populations. J Appl Genet. (2013) 54(4):441–6. doi: 10.1007/s13353-013-0166-9
39. Varga E. The genetics of Thrombophilia. The National Alliance for Thrombosis and Thrombophilia (NATT). (2013). p. 1–9.
40. Fall AOT, Proulle V, Sall A, Mbaye A, Ba PS, Diao M, et al. Risk factors for thrombosis in an African population. Clin Med Insights Blood Disord. (2014) 7:1–6. doi: 10.4137/CMBD.S13401
41. Abdi AA, Osman A. Prevalence of common hereditary risk factors for thrombophilia in Somalia and identification of a novel Gln544Arg mutation in coagulation factor V. J Thromb Thrombolysis. (2017) 44(4):536–43. doi: 10.1007/s11239-017-1543-8
42. Jadaon MM. Epidemiology of prothrombin G20210A mutation in the Mediterranean region. Mediterr J Hematol Infect Dis. (2011) 3(1). doi: 10.4084/MJHID.2011.054
43. Shafia S, Zargar MH, Khan N, Ahmad R, Shah ZA, Asimi R. High prevalence of factor V Leiden and prothrombin G20101A mutations in Kashmiri patients with venous thromboembolism. Gene. (2018) 654:1–9. doi: 10.1016/j.gene.2018.02.031
44. Austin H, De Staercke C, Lally C, Bezemer ID, Rosendaal FR, Hooper WC. New gene variants associated with venous thrombosis : a replication study in white and black Americans. J Thromb Haemost. (2011) 9:489–95. doi: 10.1111/j.1538-7836.2011.04185.x
45. Cushman M, Pankow JS. Prospective study of circulating factor XI and incident venous thromboembolism: the longitudinal investigation of thromboembolism etiology (LITE). Am J Hematol. (2015) 90(11):1047–51. doi: 10.1002/ajh.24168.Prospective
46. Vasan SK, Rostgaard K, Majeed A, Ullum H, Titlestad KE, Pedersen OB, et al. ABO blood group and risk of thromboembolic and arterial disease: a study of 1.5 million blood donors. Circulation. (2016) 133:1449–57. doi: 10.1161/CIRCULATIONAHA.115.017563
47. Dentali F, Sironi A, Ageno W, Turato S, Bonfanti C, Frattini F, et al. Non-O blood type is the commonest genetic risk factor for VTE: results from a meta-analysis of the literature. Semin Thromb Hemost. (2012) 38(5):535–47. doi: 10.1055/s-0032-1315758
48. Sun X, Feng J, Wu W, Peng M, Shi J. ABO blood types associated with the risk of venous thromboembolism in Han Chinese people: a hospital-based study of 200,000 patients. Sci Rep. (2017) 7:42925. doi: 10.1038/srep42925
49. Yu M, Wang C, Chen T, Hu S, Yi K, Tan X. ABO blood groups and risk of deep venous thromboembolism in Chinese Han population from Chaoshan region in south China. Saudi Med J. (2017) 38(4):396–9. doi: 10.15537/smj.2017.4.16349
50. Massimo Franchini MM. Non-O blood group: an important genetic risk factor for venous thromboembolism. Blood Transfus. (2013) 11(2):164–5. doi: 10.2450/2012.0087-12
51. Miñano A, Ordóñez A, España F, González-porras JR, Lecumberri R, Fontcuberta J, et al. AB0 blood group and risk of venous or arterial thrombosis in carriers of factor V Leiden or prothrombin G20210A polymorphisms. Haematologica. (2008) 93(5):729–34. doi: 10.3324/haematol.12271
52. Kinsella M, Monk C. Race, ABO blood type and VTE risk: not black and white. Transfusion. (2012) 23(1):1–7. doi: 10.1111/j.1537-2995.2012.03665.x
53. Skille H, Paulsen B, Hveem K, E. Gabrielsen M, Brumpton B, Hindberg K, et al. Combined effects of five prothrombotic genotypes and cancer on the risk of a first venous thromboembolic event. J Thromb Haemost. (2020) 18(11):2861–9. doi: 10.1111/jth.15011
54. Van Langevelde K, Šrámek A, Rosendaal FR. The effect of aging on venous valves. Arterioscler Thromb Vasc Biol. (2010) 30(10):2075–80. doi: 10.1161/ATVBAHA.110.209049
55. Engbers MJ, van Hylckama Vlieg A, Rosendaal FR. Venous thrombosis in the elderly: incidence, risk factors and risk groups. J Thromb Haemost. (2010) 8(10):2105–12. doi: 10.1111/j.1538-7836.2010.03986.x
56. Donato AJ, Machin DR, Lesniewski LA. Mechanisms of dysfunction in the aging vasculature and role in age-related disease. Circ Res. (2018) 123(7):825–48. doi: 10.1161/CIRCRESAHA.118.312563
57. Karasu A, Šrámek A, Rosendaal FR, van der Geest RJ, van Hylckama Vlieg A. Aging of the venous valves as a new risk factor for venous thrombosis in the elderly: the BATAVIA study. J Thromb Haemost. (2018) 16(1):96–103. doi: 10.1111/jth.13880
58. Genyan Yang WCH, De Staercke C. The effects of obesity on venous thromboembolism: a review. Open J Prev Med. (2012) 2(4):499–509. doi: 10.4236/ojpm.2012.24069
59. Morgan ES, Wilson E, Watkins T, Gao F, Hunt BJ. Maternal obesity and venous thromboembolism. Int J Obstet Anesth. (2012) 21(3):253–63. doi: 10.1016/j.ijoa.2012.01.002
60. Horvei LD, Brækkan SK, Mathiesen EB, Njølstad I, Wilsgaard T, Hansen JB. Obesity measures and risk of venous thromboembolism and myocardial infarction. Eur J Epidemiol. (2014) 29:821–30. doi: 10.1007/s10654-014-9950-z
61. Hotoleanu C. Association between obesity and venous thromboembolism. Med Pharm Reports. (2020) 93(2):162–8. doi: 10.15386/mpr-1372
62. Kabrhel C, Varraso R, Goldhaber SZ, Rimm EB, Camargo CA. Prospective study of BMI and the risk of pulmonary embolism in women. Obesity. (2009) 17(11):2040–6. doi: 10.1038/oby.2009.92
63. Kahn SR, Lim W, Dunn AS, Cushman M, Dentali F, Akl EA, et al. Prevention of VTE in nonsurgical patients. Antithrombotic therapy and prevention of thrombosis, 9th ed: American College of Chest Physicians evidence-based clinical practice guidelines. Chest. (2012) 141(2 Suppl):e195S–226S. doi: 10.1378/chest.11-2296
64. Ádány R, Pikó P, Fiatal S, Kósa Z, Sándor J, Bíró E, et al. Prevalence of insulin resistance in the Hungarian general and Roma populations as defined by using data generated in a complex health (interview and examination) survey. Int J Environ Res Public Health. (2020) 17(13):1–22. doi: 10.3390/ijerph17134833
65. Ghouse J, Tragante V, Ahlberg G, Rand SA, Jespersen JB, Leinøe EB, et al. Genome-wide meta-analysis identifies 93 risk loci and enables risk prediction equivalent to monogenic forms of venous thromboembolism. Nat Genet. (2023) 55:399–409. doi: 10.1038/s41588-022-01286-7
66. Zhang Z, et al. Genome-wide association analyses identified novel susceptibility loci for pulmonary embolism among Han Chinese population. BMC Med. (2023) 21(1):1–13. doi: 10.1186/s12916-023-02844-4
67. Fiatal S, Pikó P, Kósa Z, Sándor J, Ádány R. Genetic profiling revealed an increased risk of venous thrombosis in the Hungarian Roma population. Thromb Res. (2019) 179:37–44. doi: 10.1016/j.thromres.2019.04.031
68. Eppenberger D, Nilius H, Anagnostelis B, Huber CA, Nagler M. Current knowledge on factor V leiden mutation as a risk factor for recurrent venous thromboembolism: A Systematic Review and meta-analysis. Front Cardiovasc Med. (2022) 9:883986. doi: 10.3389/fcvm.2022.883986
69. Eppenberger D, Nilius H, Anagnostelis B, Huber CA, Nagler M. Current knowledge on factor V Leiden mutation as a risk factor for recurrent venous thromboembolism: a systematic review and meta-analysis. Front Cardiovasc Med. (2022) 9:1–13. doi: 10.3389/fcvm.2022.883986
70. Li Y, Bezemer ID, Rowland CM, Tong CH, Arellano AR, Catanese JJ, et al. Genetic variants associated with deep vein thrombosis : the F11 locus. J Thromb Haemost. (2009) 7:1802–8. doi: 10.1111/j.1538-7836.2009.03544.x
71. Bucciarelli P, De Stefano V, M. Passamont S, Tormene D, Legnani C, Rossi E, et al. Influence of proband’s characteristics on the risk for venous thromboembolism in relatives with factor V Leiden or prothrombin. Blood. (2016) 122(15):2555–62. doi: 10.1182/blood-2013-05-503649
72. Bezgin T, Kaymaz C, Tokgo HC. Thrombophilic gene mutations in relation to different manifestations of venous thromboembolism a single tertiary center study. Clin Appl Thromb Hemost. (2018) 24(1):100–6. doi: 10.1177/1076029616672585
73. Karppinen JE, Törmäkangas T, Kujala UM, Sipilä S, Laukkanen J, Aukee P, et al. Menopause modulates the circulating metabolome: evidence from a prospective cohort study. Eur J Prev Cardiol. (2022) 29(10):1448–59. doi: 10.1093/eurjpc/zwac060
74. Pate A, Emsley R, Ashcroft DM, Brown B, Van Staa T. Correction: the uncertainty with using risk prediction models for individual decision making: an exemplar cohort study examining the prediction of cardiovascular disease in English primary care (BMC Medicine). BMC Med. (2019) 17(1):1–16. doi: 10.1186/s12916-019-1404-8
75. Kamal SA, Yin C, Qian B, Zhang P. An interpretable risk prediction model for healthcare with pattern attention. BMC Med Inform Decis Mak. (2020) 20(11):1–11. doi: 10.1186/s12911-020-01331-7
76. Chapman BP, Lin F, Roy Sh, Ralph HB, Benedict RHB, Lyness JM. Health risk prediction models incorporating personality data: motivation, challenges, and illustration. Personal Disord. (2019) 10(1):46–58. doi: 10.1037/per0000300
77. Sharma V, Ali I, Van Der Veer S, Martin G, Ainsworth J, Augustine T. Adoption of clinical risk prediction tools is limited by a lack of integration with electronic health records. BMJ Health Care Inform. (2021) 28(1):2020–2. doi: 10.1136/bmjhci-2020-100253
78. Ahmad A, Sundquist K, Palmér K, Svensson PJ, Sundquist J, Memon AA. Risk prediction of recurrent venous thromboembolism: a multiple genetic risk model. J Thromb Thrombolysis. (2019) 47(2):216–26. doi: 10.1007/s11239-018-1762-7
79. Kolin DA, Kulm S, Elemento O. Prediction of primary venous thromboembolism based on clinical and genetic factors within the U.K. Biobank. Sci Rep. (2021) 11(1):1–9. doi: 10.1038/s41598-021-00796-4
80. Hodeib H, Youssef A, Allam AA, Selim A, Tawfik MA, Abosamak MF, et al. Genetic risk profiling associated with recurrent unprovoked venous thromboembolism. Genes (Basel). (2021) 12(6):1–11. doi: 10.3390/genes12060874
81. Lindström S, Wang L, Smith EN, Gordon W, Van Hylckama VA, De Andrade M, et al. Genomic and transcriptomic association studies identify 16 novel susceptibility loci for venous thromboembolism. Blood. (2019) 134(19):1645–57. doi: 10.1182/blood.2019000435
82. Thibord F, Klarin D, Brody JA, Chen MH, Levin MG, Chasman DI, et al. Cross-ancestry investigation of venous thromboembolism genomic predictors. Circulation. (2022) 146(16):1225–42. doi: 10.1161/CIRCULATIONAHA.122.059675
Keywords: venous thrombosis, risk prediction, single nucleotide polymorphisms (SNPs), cardiovascular risk (CVD), Hungarian population
Citation: Natae SF, Merzah MA, Sándor J, Ádány R, Bereczky Z and Fiatal S (2023) A combination of strongly associated prothrombotic single nucleotide polymorphisms could efficiently predict venous thrombosis risk. Front. Cardiovasc. Med. 10:1224462. doi: 10.3389/fcvm.2023.1224462
Received: 17 May 2023; Accepted: 3 August 2023;
Published: 6 September 2023.
Edited by:
Silvio Antoniak, University of North Carolina at Chapel Hill, United StatesReviewed by:
István Kiss, University of Pécs, Hungary© 2023 Natae, Merzah, Sándor, Ádány, Bereczky and Fiatal. This is an open-access article distributed under the terms of the Creative Commons Attribution License (CC BY). The use, distribution or reproduction in other forums is permitted, provided the original author(s) and the copyright owner(s) are credited and that the original publication in this journal is cited, in accordance with accepted academic practice. No use, distribution or reproduction is permitted which does not comply with these terms.
*Correspondence: Szilvia Fiatal ZmlhdGFsLnN6aWx2aWFAbWVkLnVuaWRlYi5odQ==
Disclaimer: All claims expressed in this article are solely those of the authors and do not necessarily represent those of their affiliated organizations, or those of the publisher, the editors and the reviewers. Any product that may be evaluated in this article or claim that may be made by its manufacturer is not guaranteed or endorsed by the publisher.
Research integrity at Frontiers
Learn more about the work of our research integrity team to safeguard the quality of each article we publish.