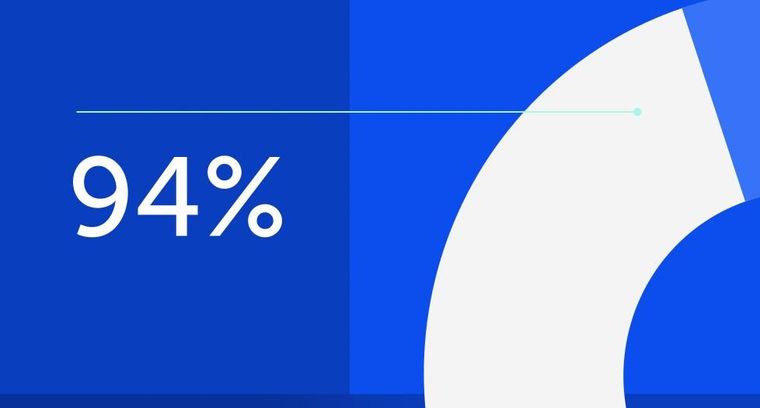
94% of researchers rate our articles as excellent or good
Learn more about the work of our research integrity team to safeguard the quality of each article we publish.
Find out more
ORIGINAL RESEARCH article
Front. Cardiovasc. Med., 31 August 2023
Sec. Atherosclerosis and Vascular Medicine
Volume 10 - 2023 | https://doi.org/10.3389/fcvm.2023.1216796
Background: Computational fluid dynamics (CFD) is emerging as an effective technology able to improve procedural outcomes and enhance clinical decision-making in patients with coronary artery disease (CAD). The present study aims to assess the state of knowledge, use and clinical acceptability of CFD in the diagnosis and treatment of CAD.
Methods: We realized a 20-questions international, anonymous, cross-sectional survey to cardiologists to test their knowledge and confidence on CFD as a technology applied to patients suffering from CAD. Responses were recorded between May 18, 2022, and June 12, 2022.
Results: A total of 466 interventional cardiologists (mean age 48.4 ± 8.3 years, males 362), from 42 different countries completed the survey, for a response rate of 45.9%. Of these, 66.6% declared to be familiar with the term CFD, especially for optimization of existing interventional techniques (16.1%) and assessment of hemodynamic quantities related with CAD (13.7%). About 30% of respondents correctly answered to the questions exploring their knowledge on the pathophysiological role of some CFD-derived quantities such as wall shear stress and helical flow in coronary arteries. Among respondents, 85.9% would consider patient-specific CFD-based analysis in daily interventional practice while 94.2% declared to be interested in receiving a brief foundation course on the basic CFD principles. Finally, 87.7% of respondents declared to be interested in a cath-lab software able to conduct affordable CFD-based analyses at the point-of-care.
Conclusions: Interventional cardiologists reported to be profoundly interested in adopting CFD simulations as a technology supporting decision making in the treatment of CAD in daily practice.
Coronary artery disease (CAD) continues to be the leading cause of morbidity and mortality in Western countries (1). From an epidemiological perspective, CAD affects approximately 200 million individuals and it is responsible for nearly 9 million deaths worldwide (2). CAD can be diagnosed using non-invasive or invasive tests, and the treatment options include pharmacological and interventional options. However, coronary angiography is still considered the gold standard technique for assessing CAD and providing guidance for percutaneous coronary interventions (PCI) (3). Thus, the development of novel diagnostic and therapeutic technologies has received considerable interest.
In recent years, computational fluid dynamics (CFD) has emerged as a methodology ready to shift to technology for cardiovascular medicine, potentially enhancing the understanding of pathophysiology, by simulating interventional procedures, and improving decision making regarding CAD treatment (4–8). CFD utilizes numerical techniques to solve and analyze problems involving fluid flows, including blood flow (4). Within the context of cardiovascular medicine, CFD has been extensively employed to investigate the role of hemodynamics in CAD pathophysiology (5, 9, 10). It has found widespread use in the functional assessment of CAD and in the elucidation of the role of hemodynamics in CAD initiation, progression, susceptibility and phenotype (4, 11, 12). Additionally, CFD has demonstrated its capability to describe and predict the hemodynamic responses before (13, 14) and after cardiovascular interventional procedures (15, 16), making it a valuable tool in the development and enhancement of intervention devices and treatment strategies (6, 17–21). Furthermore, current literature has highlighted that basic modeling concepts are now ripe for incorporation into the knowledge base of interventional cardiologists. In this regard, recent evidences have demonstrated the feasibility of independent management by interventional cardiologists of CFD-based technology for CAD-related predictions (22).
Until now, the adoption of CFD as supporting technology and the opportunities opened by its clinical application in the context of CAD have been hampered by the demanding computational cost to run simulations, especially when compared to current diagnostic imaging acquisitions. This has prevented the use of computational hemodynamics in large clinical studies, which in turn would be required to prove the utility of computer-based hemodynamic modelling, setting up a vicious cycle. New technological approaches are under development, either supplementing or replacing conventional CFD, with the goal of making cardiovascular modelling tasks compatible with clinical needs with respect to simulation runtimes (23). Despite these advancements, the input data required for the hemodynamic analysis are usually affected by uncertainties and measurement noise impacting the accuracy of the simulation results. Currently, there is a growing recognition on the need for appropriate methods of uncertainty quantification able to estimate the propagation of uncertainty from the model inputs to the simulation results, to increase their reliability and enable greater clinical impact (24, 25). Moreover, CFD is still perceived by interventional cardiologists as a technology for which most of them have never been trained. This represents a major barrier to its widespread adoption. For these reasons, the aim of this work was to conduct an independent, international survey to evaluate the awareness, knowledge, and interest of interventional cardiologists in utilizing CFD simulations for the management of CAD.
We designed a 20-item, international, anonymous survey to assess the awareness, knowledge, interest and use of CFD in the management of CAD. For this purpose, an electronic questionnaire in English language designed using the virtual Google Form, consisting of 20 multiple-choice (single or multiple answers) or open-ended questions, was sent via e-mail to suitable respondents identified by the authors. Specifically, all the authors were involved in spreading the survey sending via e-mail the virtual form to their contacts and potential participants, registering the number of invitations sent. All potential respondents were physicians with a cardiology certification, working as interventional cardiologists and directly involved in the diagnosis and treatment of CAD during their daily clinical practice. To evaluate the knowledge and utilization of CFD in a real-world context, no additional strict criteria (e.g., based on experience levels or the number of interventional procedures performed annually) were applied in the selection of respondents. Responses were recorded between May 18th, 2022, and June 12th, 2022, and the participation was voluntary. A reminder e-mail was dispatched to the recipients two weeks after the initial invitation to encourage their participation. Data security and the protection of participants’ data was guaranteed by the security measures applied by the Google Form platform, by ensuring access to the data only to the form creators and by not collecting identifiable personal information. Access to the data was restricted to the form creators only. Considering that no patient-specific, pre-procedural and post-procedural data were collected, and all participation by study sites was voluntary and anonymous, no ethics committee review was required.
The survey was composed by three different sections: (i) respondents’ demographic details, such as age, sex, place of work and number of coronary interventional procedures performed yearly; (ii) questions regarding basic CFD knowledge, its potential application in CAD patients, and the role that hemodynamic quantities derived from CFD simulations, such as wall shear stress (WSS) and helical flow (HF), have in CAD (5, 26–29) (Figure 1 shows an explanatory example of the hemodynamic quantities obtainable from CFD simulations); (iii) questions exploring the interest of interventional cardiologists to obtain a simple orientation course (a) on the basic pathophysiological mechanisms related to local hemodynamics and (b) on the clinical implications of patient-specific CFD-based analysis in CAD, as well as (c) on the availability of software applications performing a reliable CFD-based analysis that can be used directly in the operating room by interventional cardiologists irrespective to the presence of specialized personnel (e.g., biomedical engineers). The full survey is available in the Supplementary Data.
Figure 1. Example of hemodynamic quantities obtainable from computational fluid dynamics (CFD) simulations for a diseased right coronary artery model. (left) Color maps of wall shear stress (WSS) along the endothelial surface. The WSS is defined as the tangential stress due to the friction of the flowing blood on the endothelial surface. In the coronary artery model here reported, high WSS values are present at the stenosis region. (right) Isosurfaces of local normalized helicity (LNH) representing the counter-rotating helical flow structures that develop in the intravascular region of the coronary artery model. Positive/negative LNH values indicate right-handed/left-handed rotating fluid structures along the main flow direction and are displayed in red/blue, respectively. The diseased right coronary artery belongs to a patient recruited within the RELATE clinical trial (ClinicalTrials.gov Identifier: NCT04048005).
A descriptive statistical analysis was performed to assess respondents’ general characteristics. Continuous variables were expressed as mean ± standard deviation (SD), while the non-normally distributed variables were presented in terms of median and confidence interval [CI]. Categorical variables were presented as proportions. The normally distributed continuous variables were compared using the student's t-test or ANOVA (with Bonferroni's post hoc), whereas the non-Normally distributed variables were compared using the Mann–Whitney U-test. Comparisons between categorical variables were performed using χ2-test or Fisher's exact test. A multivariate regression analysis was computed to identify independent associations with knowledge of CFD in CAD patients. Variables with p < 0.1 characterizing the univariate analysis were included in the multivariate model. A cut-off of 40 years was chosen to differentiate between “expert” interventional cardiologists (i.e., cardiologists with ostensibly >10 years of clinical practice) and those with less experience. The knowledge of the pathophysiological role of WSS and HF was defined as the correct answer to all the related questions in the survey. Statistical analyses were performed using SPSS package version 20.0 (SPSS, Chicago, IL, USA).
A total of 466 interventional cardiologists (mean age 48.4 ± 8.3 years, males 362) from 42 different countries (Figure 2) completed the survey out of 1015 invitations, resulting in a response rate of 45.9%. The demographic and general characteristics of the respondents are presented in Table 1. Most interventional cardiologists were males, based in Europe (59.2%) and America (23.3%) (Figure 2) and practice in non-university teaching hospitals (41.0%). More than half of respondents (64.5%) performed more than 100 PCI yearly. Male respondents were older than women (49.0 ± 8.7 vs. 45.4 ± 6.0 years, p < 0.0001) and with a greater interventional experience (16.9 ± 8.7 vs. 12.0 ± 6.4 years, p < 0.0001). A further sub-analysis, stratifying respondents by continents, is provided into Supplementary Table S1.
Among all the respondents, 66.6% (n = 297) declared to be familiar with the term CFD; of these, 65.2% declared to be aware of clinical applications of CFD in CAD and coronary artery interventions. A further sub-analysis evidenced that most respondents declaring to have knowledge on CFD applications were younger (45.7 ± 7.2 vs. 53.6 ± 8.0 years old, p < 0.0001) and with less years of practice (13.4 ± 7.4 vs. 21.4 ± 8.3 years, p < 0.0001) compared to those who were unaware of CFD uses in CAD management. Most of respondents declared to be aware of CFD-based analysis applied to optimize existing interventional techniques (22.5%), followed by those deeming CFD simulations necessary to evaluate local hemodynamic quantities related with CAD (19.0%) or to quantify not invasively the fractional flow reserve (FFR) (18.7%) (Table 2), an index used for the assessment of the functional severity of coronary stenoses.
Only 118 respondents (26.5%) correctly answered to the question exploring the knowledge of the role of WSS in the atherogenic process. Conversely, 153 interventional cardiologists (34.3%) identified the correct answer regarding the pathophysiologic role of WSS after coronary artery stenting. Finally, 48.2% (n = 215) respondents correctly attributed an atheroprotective role to HF in the coronary arteries. Notably, in all the three previous questions no significant differences were registered in age between those who answered correctly or not.
As presented in Figure 3, irrespectively of age and years of practice the 85.9% (n = 383) of respondents would consider CFD-based analysis in daily interventional practice, for the evaluation of WSS and HF before procedures (82.7%, n = 369) and for the application of a baseline CFD simulation (89.0%, n = 397) to preliminary identify culprit lesions potentially leading to future acute myocardial infarctions. Regarding the interest in introducing CFD analysis in daily clinical practice, on a scale from one to five, where one represents the lowest and five the highest probability, 29.4% and 23.7% of respondents gave a score of four and five, respectively (Figure 4). The most common concerns related to the use of CFD in the clinical practice are the amount of time required to run a simulation and the need for proper training (34.8% and 33.8%, respectively) (Figure 5).
Figure 3. Interest in the use and application of computational fluid dynamics (CFD) in coronary artery disease.
Figure 4. Probability for computational fluid dynamics (CFD) use in future daily interventional practice graded from one to five. 1, lowest probability; 5, highest probability.
Figure 5. Reservations about the applications of computational fluid dynamics (CFD) in the clinical practice.
Most respondents (93.5%, n = 417) were interested in obtaining simple and basic explanation regarding CFD simulations and their application in CAD (Figure 6). Similarly, the majority of those surveyed declared to be interested in: (i) receiving a brief foundation course on the basic pathophysiological mechanisms related to local hemodynamics and on the clinical implications of CFD analysis in CAD (non-invasive functional assessment to detect flow-limiting stenosis for CAD treatment, prediction of adverse events prior or after intervention) (94.2%, n = 420); (ii) the availability of a software performing reliable patient-specific CFD simulations at the point-of-care irrespective to the presence of specialized personnel such as biomedical engineers (87.7%, n = 391) (Figure 6). Multivariate regression analysis evidenced that age <40 years (OR: 1.13, 95% CI: 1.08–1.19, p < 0.0001), working in a university hospital (OR: 1.85, 95% CI: 1.39–2.26, p < 0.001) and knowledge of the pathophysiological role of WSS and HF (OR: 1.12, 95% CI: 1.08–1.16, p = 0.01) were independently associated to consideration of CFD simulations in future daily clinical practice (Table 3).
Figure 6. Interest in receiving an adequate training and modern software for computational fluid dynamics (CFD).
Table 3. Multivariate regression analysis identifying the predictors of future use of CFD analysis in daily practice.
The findings of this survey suggest that most interventional cardiologists are interested in computer simulations of coronary hemodynamics and in their applications to CAD. In fact, 85.9% of respondents would consider CFD in daily interventional practice, while 89.0% would be interested in using computational simulations to pre-operatively identify the culprit lesions that may lead to future major cardiovascular events (MACE), typically including acute myocardial infarction, stroke and cardiovascular mortality (30). Moreover, 94.2% of participants are interested in receiving a brief and basic orientation course regarding the pathophysiological mechanisms associated with CFD analysis in CAD.
Recently, several surveys have been conducted in the field of interventional cardiology on various topics, such as the impact and management of PCI complications (31), the implementation of FFR in daily practice (32), and the adoption of American College of Cardiology appropriate use criteria for revascularization (33), among other areas of interest. However, our investigation stands out as the first attempt to assess and shed light on the current knowledge and interest regarding the use of CFD as a supporting technology in CAD management and decision making. The number of respondents align with or, in certain cases (32), exceed that of previous surveys. Moreover, the response rate was comparable (32) or higher (31, 33) than that reported by previous studies.
Examining the results of our survey in detail, it become apparent that despite 66.6% of respondents expressed familiarity with the term “CFD”, there was a widespread self-awareness of a skill gap in dealing with simulations as technology users and interpreting CFD-derived hemodynamic quantities such as WSS and HF. These results reflect a lack of basic training and education in computational medicine and its specific applications to CAD. Indeed, the integration of CFD with clinical imaging candidates to be the technological solution for minimally invasive patient assessment and for the obtainment of patient-specific evaluations of the hemodynamic risk associated with CAD, thus promoting the diffusion of precision medicine practices among interventional cardiologists (34–36).
As highlighted by our results, younger cardiologists exhibit greater level of confidence on CFD simulations. This suggests that CFD may become a routine aspect of daily practice in the near future, particularly for predicting physiological hemodynamic responses following cardiovascular interventional procedures (Table 4) (5, 9–11, 16–19, 22, 26, 27, 37–65). The reasons are probably multifactorial. Firstly, young generations tend to be more comfortable with and open to adopting new technologies, in particular computer models. Secondly, while young interventionalists are being trained, CFD techniques are being widely utilized for research purposes, despite their limited use in clinical practice (5). However, when we tested respondents’ knowledge of pathophysiological concepts related to or emerging from CFD-based applications to CAD, only a minority identified the correct answers. This indicates that the theoretical background for both the application of CFD simulations and the interpretation of their output in the clinical framework is still lacking. Respondents were often unfamiliar with the role of WSS in promoting (i) atherosclerosis at the early stage (29, 48), (ii) its progression (29, 48), or (iii) its impact on neointimal proliferation after stenting (66–68). Similarly, 51.8% of respondents answered incorrectly regarding the atheroprotective role of HF in coronary arteries (26–28). It seems that some respondents are updated regarding the significance of the hemodynamic quantities proposed by experts in CFD for cardiovascular flows, but they are not fully aware of the underlying links between specific hemodynamic patterns and biological aggravating events at the arterial wall.
Table 4. Computational fluid dynamics (CFD) applications in diagnosis and treatment of coronary artery disease (CAD).
CFD is a promising technology with the potential to provide researchers and clinicians with a deeper understanding of blood flow patterns, pressure gradients, and other hemodynamic variables for investigating the mechanisms underlying CAD. Furthermore, CFD can be utilized for device design and optimization, offering opportunities to enhance medical interventions and management of CAD. Additionally, patient-specific CFD can be employed in the context of precision medicine, e.g., to aid in preoperative planning and risk assessment, and for prognostic purposes, thus empowering interventional cardiologists to make informed decisions and improve patient outcomes during CAD-related procedures. Despite its potential, the widespread adoption of CFD in cardiology clinical practice is characterized by several challenges. These challenges encompass the necessity for additional validation studies and standardization, logistical and computational resource availability, seamless integration with existing clinical workflows, accessibility of CFD software and expertise, efficient data accessibility and sharing, and regulatory considerations. Recent technological advancements are addressing these challenges. The availability of increasingly powerful computational resources at lower costs along with the development of dedicated and user-friendly software for calculating hemodynamics quantities of interest for cardiologists [e.g., virtual FFR and WSS descriptors (4)] is making CFD simulations more accessible. Furthermore, the ability to run CFD simulations in the cloud holds the potential to eliminate the need for workstations directly in the cath-lab, thereby reducing logistic and computational resource barriers. The integration of CFD with existing diagnostic imaging techniques, such as coronary angiography, is already feasible. Recent clinical studies have successfully demonstrated the computation of WSS descriptors with timeframes compatible with clinical practice based on CFD simulations performed by cardiologists (22, 69, 70).
Along with the diffusion and utilization of CFD in CAD, the survey emphasized the critical need to expand educational opportunities for interventional cardiologists to enhance their knowledge of this technique. In fact, the survey responses indicate that these potential educational efforts would be well received. Our multivariate regression analysis showed that interventional cardiologists aged less than 45 years old, working in a university hospital and having adequate knowledge of CFD-based hemodynamic characterization were more likely to adopt CFD in future interventional practice. These results imply the possibility of CFD remaining a technique limited to academic environments among young interventionalists. Therefore, it would be essential to disseminate fluid mechanics and CFD basic knowledge to the future generations of interventional cardiologists by developing or integrating education on basic fluid mechanics and CFD into fellowship courses and specialized sessions of interventional congresses, thereby facilitating the expansion of their didactic curriculum. This educational approach will pave the way to even more patient-tailored strategies with the potential of improving patient outcomes. This calls for the development of innovative and collaborative programs in leading centers with the establishment of dedicated CFD teams involving trained interventional cardiologists, cardiac surgeons, and biomedical engineers. In this regard, nearly 90% of respondents expressed their belief that having dedicated and user-friendly software capable of autonomously conducting reliable CFD simulations, independent of dedicated personnel, would be beneficial in everyday clinical practice. This tendency reinforces the necessity of a streamlined and user-friendly methodology that simplifies cardiovascular hemodynamic calculation, requiring less time and lower costs while still ensuring model accuracy.
The main strength of this study is represented by the higher response rate obtained from 42 different countries located in five continents. Overall, the results of the survey may realistically reflect the attitude and knowledge of interventional cardiologists on the use of CFD simulations in CAD worldwide. Nevertheless, this study faces some limitations. Firstly, the selection of respondents was based on the diffusion of the survey through the worldwide contacts of the authors. However, the number of participants partially mitigate this aspect. Secondly, demographic and professional data of nonrespondents were not available for comparison with respondents. Therefore, we hypothesize that interventional cardiologists who completed the survey may have been more confident or interested in the subject, thus potentially biasing the results toward overestimating exposure and training in CFD analysis. However, it is important to note that our study participants from the USA exhibited demographic characteristics comparable to the total active population of interventional cardiologists in USA, according to the recent report by the Association of American Medical Colleges (71). This includes a substantial majority of male cardiologists (91.8%) and a higher proportion of younger practitioners (83.9% under age 55). While detailed demographic data were unavailable for other continents, the characteristics of respondents are consistent with the data from the USA, providing additional support for the validity of our study concerning the surveyed population. Lastly, a potential limitation lies in the possibility of bias introduced by the formulation of some questions. In particular, the wording and structure of the questions may have influenced the way participants interpreted and responded to them. Nevertheless, we emphasize that if such a bias is introduced, it is not significant enough to alter the overall findings of the study, which indicate a general interest among interventional cardiologists in CFD and a lack of training in CFD simulations and interpretation of CFD results.
In this international survey investigating the understanding and utilization of CFD as supporting technology for CAD diagnosis and treatment, the majority of interviewed interventional cardiologists expressed interest in the use of computer simulations of coronary hemodynamics and in their potential applications to CAD in everyday clinical practice. The survey revealed a prevalent self-perceived deficiency in using simulations and interpreting CFD-derived hemodynamic quantities such as WSS and HF. This observation underscores the significance of addressing the lack of basic training and education in computational medicine for CAD. It is essential to prioritize the enhancement of educational opportunities in CFD applications, particularly for young interventional cardiologists, as well as establish dedicated CFD teams consisting of trained interventional cardiologists, cardiac surgeons, and biomedical engineers. Additionally, further research is necessary to develop user-friendly CFD software. All of these measures are crucial for promoting the widespread adoption of CFD in clinical practice.
The raw data supporting the conclusions of this article will be made available by the authors upon request.
CCh, MZ, GR, DG, and UM: conceptualization. CCh, MZ, and DG: survey implementation. MZ: statistical analysis. UM: funding. CCh, MZ, GR, DG, and UM: visualization and writing (original draft). CCh, MZ, GR, FDA, GMDF, CCo, YSC, DG, and UM: writing (review and editing). All authors contributed to the article and approved the submitted version.
This work has been supported by the Italian Ministry of Education, University and Research (FISR2019_03221, CECOMES).
The authors thank Dr. Maurizio Lodi Rizzini (Politecnico di Torino) for the preparation of Figure 1.
CCo have received research grants from GE Healthcare, Coroventis Research, Medis Medical Imaging, Pie Medical Imaging, CathWorks, Boston Scientific, Siemens, HeartFlow, Abbott Vascular, and consultancy fees from Medyria, Cryotherapeutics, HeartFlow, OpSens, Abbott Vascular. YSC has received speaker honoraria, advisory board fees and research grant from Boston Scientific Inc. and research grant from Medtronic Inc.
The remaining authors declare that the research was conducted in the absence of any commercial or financial relationships that could be construed as a potential conflict of interest.
All claims expressed in this article are solely those of the authors and do not necessarily represent those of their affiliated organizations, or those of the publisher, the editors and the reviewers. Any product that may be evaluated in this article, or claim that may be made by its manufacturer, is not guaranteed or endorsed by the publisher.
The Supplementary Material for this article can be found online at: https://www.frontiersin.org/articles/10.3389/fcvm.2023.1216796/full#supplementary-material
1. Nowbar AN, Gitto M, Howard JP, Francis DP, Al-Lamee R. Mortality from ischemic heart disease. Circ Cardiovasc Qual Outcomes. (2019) 12:e005375. doi: 10.1161/CIRCOUTCOMES.118.005375
2. Safiri S, Karamzad N, Singh K, Carson-Chahhoud K, Adams C, Nejadghaderi SA, et al. Burden of ischemic heart disease and its attributable risk factors in 204 countries and territories, 1990–2019. Eur J Prev Cardiol. (2022) 29:420–31. doi: 10.1093/EURJPC/ZWAB213
3. Nakamura M. Angiography is the gold standard and objective evidence of myocardial ischemia is mandatory if lesion severity is questionable. Indication of PCI for angiographically significant coronary artery stenosis without objective evidence of myocardial ischemia (Pro). Circ J. (2011) 75:204–10. doi: 10.1253/CIRCJ.CJ-10-0881
4. Candreva A, De Nisco G, Lodi Rizzini M, D’Ascenzo F, De Ferrari GM, Gallo D, et al. Current and future applications of computational fluid dynamics in coronary artery disease. Rev Cardiovasc Med. (2022) 23:377. doi: 10.31083/J.RCM2311377/71978E59804F546A5B2FFEDC3D293117.PDF
5. Gijsen F, Katagiri Y, Barlis P, Bourantas C, Collet C, Coskun U, et al. Expert recommendations on the assessment of wall shear stress in human coronary arteries: existing methodologies, technical considerations, and clinical applications. Eur Heart J. (2019) 40:3421–33. doi: 10.1093/EURHEARTJ/EHZ551
6. LaDisa JF Jr, Ghorbannia A, Marks DS, Mason P, Otake H. Advancements and opportunities in characterizing patient-specific wall shear stress imposed by coronary artery stenting. Fluids (2022) 7:325. doi: 10.3390/FLUIDS7100325
7. Morris PD, Narracott A, Von Tengg-Kobligk H, Soto DAS, Hsiao S, Lungu A, et al. Computational fluid dynamics modelling in cardiovascular medicine. Heart. (2016) 102:18–28. doi: 10.1136/HEARTJNL-2015-308044/-/DC1
8. Peirlinck M, Costabal FS, Yao J, Guccione JM, Tripathy S, Wang Y, et al. Precision medicine in human heart modeling: perspectives, challenges, and opportunities. Biomech Model Mechanobiol. (2021) 20:803–31. doi: 10.1007/S10237-021-01421-Z
9. Stone PH, Saito S, Takahashi S, Makita Y, Nakamura S, Kawasaki T, et al. Prediction of progression of coronary artery disease and clinical outcomes using vascular profiling of endothelial shear stress and arterial plaque characteristics: the PREDICTION study. Circulation. (2012) 126:172–81. doi: 10.1161/CIRCULATIONAHA.112.096438
10. Stone PH, Maehara A, Coskun AU, Maynard CC, Zaromytidou M, Siasos G, et al. Role of low endothelial shear stress and plaque characteristics in the prediction of nonculprit major adverse cardiac events: the PROSPECT study. JACC Cardiovasc Imaging. (2018) 11:462–71. doi: 10.1016/J.JCMG.2017.01.031
11. Morris PD, Van De Vosse FN, Lawford P V, Hose DR, Gunn JP. “Virtual” (computed) fractional flow reserve: current challenges and limitations. JACC Cardiovasc Interv. (2015) 8:1009–17. doi: 10.1016/J.JCIN.2015.04.006
12. Geng Y, Liu H, Wang X, Zhang J, Gong Y, Zheng D, et al. Effect of microcirculatory dysfunction on coronary hemodynamics: a pilot study based on computational fluid dynamics simulation. Comput Biol Med. (2022) 146:105583. doi: 10.1016/J.COMPBIOMED.2022.105583
13. Du P, Zhu X, Wang JX. Deep learning-based surrogate model for three-dimensional patient-specific computational fluid dynamics. Phys Fluids. (2022) 34:081906. doi: 10.1063/5.0101128/2846694
14. Gharleghi R, Sowmya A, Beier S. Transient wall shear stress estimation in coronary bifurcations using convolutional neural networks. Comput Methods Programs Biomed. (2022) 225:107013. doi: 10.1016/J.CMPB.2022.107013
15. Mortier P, Wentzel JJ, De Santis G, Chiastra C, Migliavacca F, De Beule M, et al. Patient-specific computer modelling of coronary bifurcation stenting: the John Doe programme. EuroIntervention. (2015) 11(Suppl V):V35–9. doi: 10.4244/EIJV11SVA8
16. Chiastra C, Morlacchi S, Gallo D, Morbiducci U, Cárdenes R, Larrabide I, et al. Computational fluid dynamic simulations of image-based stented coronary bifurcation models. J R Soc Interface. (2013) 10:20130193. doi: 10.1098/rsif.2013.0193
17. Migliavacca F, Chiastra C, Chatzizisis YS, Dubini G. Virtual bench testing to study coronary bifurcation stenting. EuroIntervention. (2015) 11(Suppl V):V31–4. doi: 10.4244/EIJV11SVA7
18. Antoniadis AP, Mortier P, Kassab G, Dubini G, Foin N, Murasato Y, et al. Biomechanical modeling to improve coronary artery bifurcation stenting: expert review document on techniques and clinical implementation. JACC Cardiovasc Interv. (2015) 8:1281–96. doi: 10.1016/j.jcin.2015.06.015
19. Chiastra C, Migliori S, Burzotta F, Dubini G, Migliavacca F. Patient-specific modeling of stented coronary arteries reconstructed from optical coherence tomography: towards a widespread clinical use of fluid dynamics analyses. J Cardiovasc Transl Res. (2018) 11:156–72. doi: 10.1007/s12265-017-9777-6
20. Chatzizisis YS, Makadia J, Zhao S, Panagopoulos A, Sharzehee M, Khan B, et al. First-in-human computational preprocedural planning of left main interventions using a new everolimus-eluting stent. JACC Case Rep. (2022) 4:325–35. doi: 10.1016/J.JACCAS.2022.02.001
21. Zhao S, Wu W, Samant S, Khan B, Kassab GS, Watanabe Y, et al. Patient-specific computational simulation of coronary artery bifurcation stenting. Sci Rep. (2021) 11:16486. doi: 10.1038/S41598-021-95026-2
22. Candreva A, Pagnoni M, Lodi Rizzini M, Mizukami T, Gallinoro E, Mazzi V, et al. Risk of myocardial infarction based on endothelial shear stress analysis using coronary angiography. Atherosclerosis. (2021) 342:28–35. doi: 10.1016/J.ATHEROSCLEROSIS.2021.11.010
23. Arzani A, Wang JX, Sacks MS, Shadden SC. Machine learning for cardiovascular biomechanics modeling: challenges and beyond. Ann Biomed Eng. (2022) 50:615–27. doi: 10.1007/S10439-022-02967-4
24. Steinman DA, Migliavacca F. Editorial: special issue on verification, validation, and uncertainty quantification of cardiovascular models: towards effective VVUQ for translating cardiovascular modelling to clinical utility. Cardiovasc Eng Technol. (2018) 9:539–43. doi: 10.1007/s13239-018-00393-z
25. Fleeter CM, Geraci G, Schiavazzi DE, Kahn AM, Marsden AL. Multilevel and multifidelity uncertainty quantification for cardiovascular hemodynamics. Comput Methods Appl Mech Eng. (2020) 365:113030. doi: 10.1016/J.CMA.2020.113030
26. De Nisco G, Kok AM, Chiastra C, Gallo D, Hoogendoorn A, Migliavacca F, et al. The atheroprotective nature of helical flow in coronary arteries. Ann Biomed Eng. (2019) 47:425–38. doi: 10.1007/s10439-018-02169-x
27. De Nisco G, Hoogendoorn A, Chiastra C, Gallo D, Kok AM, Morbiducci U, et al. The impact of helical flow on coronary atherosclerotic plaque development. Atherosclerosis. (2020) 300:39–46. doi: 10.1016/j.atherosclerosis.2020.01.027
28. Zuin M, Rigatelli G, Zuliani G, Roncon L. Helical flow as a new determinant of coronary plaque vulnerability: a glimpse into the future. Rev Esp Cardiol (Engl Ed). (2021) 74:643. doi: 10.1016/J.REC.2021.02.007
29. Zhou M, Yu Y, Chen R, Liu X, Hu Y, Ma Z, et al. Wall shear stress and its role in atherosclerosis. Front Cardiovasc Med. (2023) 10:1083547. doi: 10.3389/fcvm.2023.1083547
30. Bosco E, Hsueh L, McConeghy KW, Gravenstein S, Saade E. Major adverse cardiovascular event definitions used in observational analysis of administrative databases: a systematic review. BMC Med Res Methodol. (2021) 21:241. doi: 10.1186/S12874-021-01440-5
31. Simsek B, Kostantinis S, Karacsonyi J, Hall A, Rangan BV, Croce KJ, et al. International percutaneous coronary intervention complication survey. Catheter Cardiovasc Interv. (2022) 99:1733–40. doi: 10.1002/CCD.30173
32. Tebaldi M, Biscaglia S, Pecoraro A, Fineschi M, Campo G. Fractional flow reserve implementation in daily clinical practice: a European survey. Int J Cardiol. (2016) 207:206–7. doi: 10.1016/J.IJCARD.2016.01.097
33. Welt FGP, Klein LW, Tamis-Holland J, Blankenship J, Duffy PL, Cigarroa J, et al. Views of appropriate use criteria for catheterization and percutaneous coronary revascularization by practicing interventional cardiologists: results of a survey of American college of cardiology interventional section members. Catheter Cardiovasc Interv. (2019) 93:875–9. doi: 10.1002/CCD.27931
34. Migliori S, Chiastra C, Bologna M, Montin E, Dubini G, Genuardi L, et al. Application of an OCT-based 3D reconstruction framework to the hemodynamic assessment of an ulcerated coronary artery plaque. Med Eng Phys. (2020) 78:74–81. doi: 10.1016/j.medengphy.2019.12.006
35. Tomizawa N, Nozaki Y, Fujimoto S, Takahashi D, Kudo A, Kamo Y, et al. A phantom and in vivo simulation of coronary flow to calculate fractional flow reserve using a mesh-free model. Int J Cardiovasc Imaging. (2021) 38:895–903. doi: 10.1007/S10554-021-02456-0
36. Vardhan M, Gounley J, Chen SJ, Chi EC, Kahn AM, Leopold JA, et al. Non-invasive characterization of complex coronary lesions. Sci Rep. (2021) 11:8145. doi: 10.1038/S41598-021-86360-6
37. Cutrì E, Zunino P, Morlacchi S, Chiastra C, Migliavacca F. Drug delivery patterns for different stenting techniques in coronary bifurcations: a comparative computational study. Biomech Model Mechanobiol. (2013) 12:657–69. doi: 10.1007/s10237-012-0432-5
38. Morlacchi S, Chiastra C, Cutrì E, Zunino P, Burzotta F, Formaggia L, et al. Stent deformation, physical stress, and drug elution obtained with provisional stenting, conventional culotte and tryton-based culotte to treat bifurcations: a virtual simulation study. EuroIntervention. (2014) 9:1441–53. doi: 10.4244/EIJV9I12A242
39. Escuer J, Schmidt AF, Peña E, Martínez MA, McGinty S. Mathematical modelling of endovascular drug delivery: balloons versus stents. Int J Pharm. (2022) 620:121742. doi: 10.1016/J.IJPHARM.2022.121742
40. McQueen A, Escuer J, Aggarwal A, Kennedy S, McCormick C, Oldroyd K, et al. Do we really understand how drug eluted from stents modulates arterial healing? Int J Pharm. (2021) 601:120575. doi: 10.1016/J.IJPHARM.2021.120575
41. Song J, Kouidri S, Bakir F. Review on the numerical investigations of mass transfer from drug eluting stent. Biocybern Biomed Eng. (2021) 41:1057–70. doi: 10.1016/J.BBE.2021.06.010
42. McGinty S. A decade of modelling drug release from arterial stents. Math Biosci. (2014) 257:80–90. doi: 10.1016/J.MBS.2014.06.016
43. Haley HA, Ghobrial M, Morris PD, Gosling R, Williams G, Mills MT, et al. Virtual (computed) fractional flow reserve: future role in acute coronary syndromes. Front Cardiovasc Med. (2021) 8:735008. doi: 10.3389/FCVM.2021.735008
44. Shah NR, Pierce JD, Kikano EG, Rahnemai-Azar AA, Gilkeson RC, Gupta A. CT Coronary angiography fractional flow reserve: new advances in the diagnosis and treatment of coronary artery disease. Curr Probl Diagn Radiol. (2021) 50:925–36. doi: 10.1067/J.CPRADIOL.2020.09.006
45. Taylor CA, Fonte TA, Min JK. Computational fluid dynamics applied to cardiac computed tomography for noninvasive quantification of fractional flow reserve: scientific basis. J Am Coll Cardiol. (2013) 61:2233–41. doi: 10.1016/J.JACC.2012.11.083
46. Hoogendoorn A, Kok AM, Hartman EMJ, De Nisco G, Casadonte L, Chiastra C, et al. Multidirectional wall shear stress promotes advanced coronary plaque development: comparing five shear stress metrics. Cardiovasc Res. (2020) 116:1136–46. doi: 10.1093/CVR/CVZ212
47. Kumar A, Hung OY, Piccinelli M, Eshtehardi P, Corban MT, Sternheim D, et al. Low coronary wall shear stress is associated with severe endothelial dysfunction in patients with nonobstructive coronary artery disease. JACC Cardiovasc Interv. (2018) 11:2072–80. doi: 10.1016/J.JCIN.2018.07.004
48. Morbiducci U, Kok AM, Kwak BR, Stone PH, Steinman DA, Wentzel JJ. Atherosclerosis at arterial bifurcations: evidence for the role of haemodynamics and geometry. Thromb Haemost. (2016) 115:484–92. doi: 10.1160/TH15-07-0597
49. Samady H, Eshtehardi P, McDaniel MC, Suo J, Dhawan SS, Maynard C, et al. Coronary artery wall shear stress is associated with progression and transformation of atherosclerotic plaque and arterial remodeling in patients with coronary artery disease. Circulation. (2011) 124:779–88. doi: 10.1161/CIRCULATIONAHA.111.021824
50. Tufaro V, Safi H, Torii R, Koo BK, Kitslaar P, Ramasamy A, et al. Wall shear stress estimated by 3D-QCA can predict cardiovascular events in lesions with borderline negative fractional flow reserve. Atherosclerosis. (2021) 322:24–30. doi: 10.1016/J.ATHEROSCLEROSIS.2021.02.018
51. Samady H, Molony DS, Coskun AU, Varshney AS, De Bruyne B, Stone PH. Risk stratification of coronary plaques using physiologic characteristics by CCTA: focus on shear stress. J Cardiovasc Comput Tomogr. (2020) 14:386–93. doi: 10.1016/J.JCCT.2019.11.012
52. Kumar A, Thompson EW, Lefieux A, Molony DS, Davis EL, Chand N, et al. High coronary shear stress in patients with coronary artery disease predicts myocardial infarction. J Am Coll Cardiol. (2018) 72:1926–35. doi: 10.1016/J.JACC.2018.07.075
53. Eshtehardi P, Brown AJ, Bhargava A, Costopoulos C, Hung OY, Corban MT, et al. High wall shear stress and high-risk plaque: an emerging concept. Int J Cardiovasc Imaging. (2017) 33:1089–99. doi: 10.1007/S10554-016-1055-1
54. De Nisco G, Chiastra C, Hartman EMJ, Hoogendoorn A, Daemen J, Calò K, et al. Comparison of swine and human computational hemodynamics models for the study of coronary atherosclerosis. Front Bioeng Biotechnol. (2021) 9:731924. doi: 10.3389/FBIOE.2021.731924
55. Genuardi L, Chatzizisis YS, Chiastra C, Sgueglia G, Samady H, Kassab GS, et al. Local fluid dynamics in patients with bifurcated coronary lesions undergoing percutaneous coronary interventions. Cardiol J. (2021) 28:321–9. doi: 10.5603/CJ.A2020.0024
56. Chiastra C, Dubini G, Migliavacca F. Hemodynamic perturbations due to the presence of stents. In: Ohayon J, Finet G, Pettigrew RI, editors. Biomechanics of coronary atherosclerotic plaque. London: Elsevier (2020). p. 257–78 doi: 10.1016/b978-0-12-817195-0.00011-1
57. Chiastra C, Mazzi V, Lodi Rizzini M, Calò K, Corti A, Acquasanta A, et al. Coronary artery stenting affects wall shear stress topological skeleton. J Biomech Eng. (2022) 144:061002. doi: 10.1115/1.4053503/1131202
58. Gosling RC, Morris PD, Silva Soto DA, Lawford PV, Hose DR, Gunn JP. Virtual coronary intervention: a treatment planning tool based upon the angiogram. JACC Cardiovasc Imaging. (2019) 12:865–72. doi: 10.1016/J.JCMG.2018.01.019
59. Corti A, Colombo M, De Nisco G, Rodriguez Matas JF, Migliavacca F, Chiastra C. Computational investigation of the role of low-density lipoprotein and oxygen transport in atherosclerotic arteries. In: Becker SM, Kuznetsov AV, de Monte F, Pontrelli G, Zhao D, editors. Modeling of mass transport processes in biological Media. London: Academic Press (2022). p. 139–213 doi: 10.1016/B978-0-323-85740-6.00017-0
60. Mahmoudi M, Farghadan A, McConnell DR, Barker AJ, Wentzel JJ, Budoff MJ, et al. The story of wall shear stress in coronary artery atherosclerosis: biochemical transport and mechanotransduction. J Biomech Eng. (2021) 143:041002. doi: 10.1115/1.4049026
61. Sakellarios A, Bourantas CV, Papadopoulou SL, Tsirka Z, De Vries T, Kitslaar PH, et al. Prediction of atherosclerotic disease progression using LDL transport modelling: a serial computed tomographic coronary angiographic study. Eur Heart J Cardiovasc Imaging. (2017) 18:11–8. doi: 10.1093/EHJCI/JEW035
62. Sakellarios AI, Tsompou P, Kigka V, Siogkas P, Kyriakidis S, Tachos N, Karanasiou G, Scholte A, Clemente A, Neglia D, et al. Non-invasive prediction of site-specific coronary atherosclerotic plaque progression using lipidomics, blood flow, and LDL transport modeling. Appl Sci (2021) 11:1976. doi: 10.3390/app11051976
63. Pleouras DS, Sakellarios AI, Tsompou P, Kigka V, Kyriakidis S, Rocchiccioli S, et al. Simulation of atherosclerotic plaque growth using computational biomechanics and patient-specific data. Sci Rep. (2020) 10:17409. doi: 10.1038/S41598-020-74583-Y
64. Nouri M, Jalali F, Karimi G, Zarrabi K. Image-based computational simulation of sub-endothelial LDL accumulation in a human right coronary artery. Comput Biol Med. (2015) 62:206–21. doi: 10.1016/J.COMPBIOMED.2015.04.013
65. Chiastra C, Migliavacca F. Modeling of blood flow in stented coronary arteries. In: Becker SM, Kuznetsov AV, editors. Heat transfer and fluid flow in biological processes. London: Elsevier (2015). p. 335–70.
66. Sanmartín M, Goicolea J, García C, García J, Crespo A, Rodríguez J, Goicolea JM. Influence of shear stress on in-stent restenosis: in vivo study using 3D reconstruction and computational fluid dynamics. Rev Esp Cardiol (2006) 59:20–7. doi: 10.1016/S1885-5857(06)60044-3
67. Koskinas KC, Chatzizisis YS, Antoniadis AP, Giannoglou GD. Role of endothelial shear stress in stent restenosis and thrombosis: pathophysiologic mechanisms and implications for clinical translation. J Am Coll Cardiol. (2012) 59:1337–49. doi: 10.1016/j.jacc.2011.10.903
68. Van der Heiden K, Gijsen FJH, Narracott A, Hsiao S, Halliday I, Gunn J, et al. The effects of stenting on shear stress: relevance to endothelial injury and repair. Cardiovasc Res. (2013) 99:269–75. doi: 10.1093/CVR/CVT090
69. Tufaro V, Torii R, Erdogan E, Kitslaar P, Koo BK, Rakhit R, et al. An automated software for real-time quantification of wall shear stress distribution in quantitative coronary angiography data. Int J Cardiol. (2022) 357:14–9. doi: 10.1016/J.IJCARD.2022.03.022
70. Kageyama S, Tufaro V, Torii R, Karamasis GV, Rakhit RD, Poon EKW, et al. Agreement of wall shear stress distribution between two core laboratories using three-dimensional quantitative coronary angiography. Int J Cardiovasc Imaging. (2023) 39:1581–92. doi: 10.1007/S10554-023-02872-4/FIGURES/5
71. Association of American Medical Colleges. Physician specialty data report. (2022). Available at: https://www.aamc.org/data-reports/workforce/report/physician-specialty-data-report (Accessed July 6, 2023).
Keywords: coronary artery disease, technology, precision medicine, modeling and simulation, computational fluid dynamics, hemodynamics, wall shear stress, helical flow
Citation: Chiastra C, Zuin M, Rigatelli G, D’Ascenzo F, De Ferrari GM, Collet C, Chatzizisis YS, Gallo D and Morbiducci U (2023) Computational fluid dynamics as supporting technology for coronary artery disease diagnosis and treatment: an international survey. Front. Cardiovasc. Med. 10:1216796. doi: 10.3389/fcvm.2023.1216796
Received: 4 May 2023; Accepted: 14 August 2023;
Published: 31 August 2023.
Edited by:
Hong Jin, Karolinska Institutet (KI), SwedenReviewed by:
Anne Cornelissen, University Hospital RWTH Aachen, Germany© 2023 Chiastra, Zuin, Rigatelli, D'Ascenzo, De Ferrari, Collet, Chatzizisis, Gallo and Morbiducci. This is an open-access article distributed under the terms of the Creative Commons Attribution License (CC BY). The use, distribution or reproduction in other forums is permitted, provided the original author(s) and the copyright owner(s) are credited and that the original publication in this journal is cited, in accordance with accepted academic practice. No use, distribution or reproduction is permitted which does not comply with these terms.
*Correspondence: Gianluca Rigatelli amFja3loZWFydEBsaWJlcm8uaXQ= Umberto Morbiducci dW1iZXJ0by5tb3JiaWR1Y2NpQHBvbGl0by5pdA==
†These authors have contributed equally to this work and share first authorship
Disclaimer: All claims expressed in this article are solely those of the authors and do not necessarily represent those of their affiliated organizations, or those of the publisher, the editors and the reviewers. Any product that may be evaluated in this article or claim that may be made by its manufacturer is not guaranteed or endorsed by the publisher.
Research integrity at Frontiers
Learn more about the work of our research integrity team to safeguard the quality of each article we publish.