- 1Department of Health Systems, Management and Policy, Colorado School of Public Health, University of Colorado Anschutz Medical Campus, Aurora, CO, United States
- 2Department of Human and Organizational Development, Vanderbilt University, Nashville, TN, United States
- 3Department of Cardiology, University of Colorado Denver School of Medicine, Aurora, CO, United States
Objective: Marginalized communities shoulder a disproportionate burden of cardiovascular disease (CVD) driven by concentrated neighborhood social risk factors. We provide a case study of systems science application to address geographic CVD health disparities at the community level – informing the science of CVD health disparities research.
Methods: We conducted a two-phased, multi-methods needs assessment in the Denver, Colorado area. Phase I consisted of a social network analysis to map a two-mode network of existing CVD prevention interventions and their implementing organizations. In Phase II, group model building (GMB) sessions with key community, public health, and healthcare provider stakeholders, were utilized to identify and visualize community factors contributing to disparities in CVD risk, producing a consensus-based causal loop diagram.
Results: Between May 2021 and June 2022, we conducted 24 virtual, semi-structured interviews in Phase I to describe CVD prevention interventions, and 7 virtual GMB sessions in Phase II to describe experiences of disparities in CVD risk. For the purposes of this paper, we focus on a subset of results for both phases. In Phase I we identified 89 active CVD prevention interventions, 29 of which addressed tobacco use. In Phase II, causal loop diagrams revealed root causes of disparities in CVD risk. We provide an example of a causal loop diagram that focuses on the community prevalence of tobacco use, identifying stress as a key underlying factor driving disparities. The integration of findings from both phases highlighted the alignment and misalignment between quit tobacco intervention goals and how they are being experienced in marginalized communities.
Conclusion: Systems science methods were useful to organize a large number of CVD prevention efforts, and evaluate the root causes of CVD health disparities in a high risk community. By integrating these two aspects, interventions may be reoriented to more effectively address the root causes of CVD health disparities.
Introduction
Healthy People 2030 establishes U.S. national public health goals that prioritize achieving health equity, which requires eliminating health disparities arising from social determinants of health and health literacy (1). Health disparities, or differences in health linked with social, economic, and environmental disadvantage, are well known to exist in CVD risk factors and outcomes (2–5). In 2015 the American Heart Association (AHA) issued a scientific statement highlighting “the most significant opportunities for reducing death and disability from CVD in the United States lie with addressing the social determinants of cardiovascular outcomes” (4).
The factors known to increase CVD risk are wide-ranging, from socioeconomic to psychosocial factors (4, 5). In combination, these factors make it possible to predict geographic areas of communities at highest CVD risk (5). While social determinants that drive disparities in CVD outcomes have been identified in research, strategies for addressing them are, to date, underdeveloped.
One primary strategy is to prioritize policy, systems and environmental (PSE) approaches to reduce disparities. For example, the Centers for Disease Control and Prevention has recommended action, such as regulating tobacco marketing, rather than solely relying on individual tobacco cessation interventions to reduce disparities in tobacco use (6). Similarly, the Institute of Medicine has identified PSE approaches to reduce disparities in population hypertension, emphasizing public health partnership interventions that improve density of supermarkets and safe physical activity spaces in inner cities (7).
When PSE approaches regarding CVD disparities are viewed through a health equity lens, however, it becomes evident that such a strategy is highly complex. Any health disparity is the end result of a complex and dynamic system occurring at the population level (8). Complex adaptive systems consist of different actors responding in dynamic ways with one another, creating feedback loops and emerging patterns of interactions between socio-ecological levels (8). Actors—e.g., policies, public health funding, local environment, etc. – all work in unique, often non-linear, and evolving ways to influence CVD disparities. Disentangling how best to address this complex system for more effective PSE approaches requires a modern methodological perspective.
Systems science methods were developed to help model complex and dynamic systems to identify key relationships, that when modified will have the greatest impact on improving population outcomes and reduce the likelihood of unintended consequences (9). The first step in systems science methods is building understanding around the system model (9). Studies on healthy eating, weight management, physical activity and tobacco use have constructed systems models to understand relationships among influential factors in environments and communities, to tease out which relationships to focus on to improve population health outcomes (10–13).
Incorporating health disparities into these models has identified additional factors that help explain ways in which PSE changes can better reach marginalized populations, avoiding unintended consequences (11, 14, 15). A comprehensive look at CVD prevention interventions, within communities of higher CVD risk and the context of health disparities in which they exist, has not yet been done to guide policy and population health leaders to identify key changes that can reduce geographic disparities in CVD risk.
Here we discuss the Cardiovascular Disease Equitable Prevention in Communities (CVD-EPIC) study. It is an example of how to use systems science to understand health disparities in CVD prevention, to make it more accessible to CVD prevention researchers. This community case study presents an overview of the two-phases of CVD-EPIC that aimed to:
1) Understand the network of actors involved in implementing CVD prevention interventions in Denver and their collaborative efforts;
2) Develop visual diagrams that capture the factors related to disparities in CVD risk faced by marginalized populations in Denver, Colorado and adjacent communities.
Our goal in describing the approach and initial findings from this project is to share an example of how to use systems science to understand health disparities in CVD prevention, that makes it more accessible to CVD prevention researchers and practitioners. Future research intends to use this knowledge, to develop a CVD prevention strategy in Denver that addresses health disparities in CVD risk.
Context
This study was approved as exempt from human subject research by the University of Colorado Institutional Review Board with IRB #21-3314. It is a multi-method, community-based participatory study conducted within a local public health jurisdiction. Its aim is to describe CVD interventions within the context of geographic health disparities. It took place in two phases, from May 2021 to June 2022, in the area of Denver, Colorado, USA.
In the United States, public health programs are primarily delivered through the support of local governments (16). Denver is a unique local government jurisdiction that is simultaneously a city and a county. The study was primarily focused on communities within the Denver area, but did spill over into adjacent communities within Aurora, Colorado, as well. The Institute for Public Health at Denver Health, a local health-focused agency within the largest safety net hospital in Denver, and Denver Department of Public Health and Environment, the local public health department, have historically shared local government responsibility for public health initiatives in Denver (17). They were identified as key partners and consulted early on to shape development of the research study.
Denver City and County was found to have high CVD risk at the county level, based on a prior social risk index of counties within the state of Colorado that incorporated percent of racial-ethnic minorities, college educated residents, and poverty (K. Henderson, personal communication, June 16, 2021). Study participants in both phases either played a key role in CVD prevention activities in Denver area communities, worked directly with high CVD risk sub-populations and/or were a member of a high CVD risk sub-population. Further participant recruitment details are provided later on.
Frameworks
To help classify CVD prevention primary goals and interventions, we used a combination of two frameworks. Life's Simple 7® is a list of evidence-based, individual activities promoted by the American Heart Association, that are known to reduce CVD risk (18). The same, or a similar set of activities, are commonly used in nationwide CVD health initiatives (2). At the time our study began, we did not include “Get Healthy Sleep” because Life's Essential 8TM had not yet been published (19). Activities focused on cardiovascular risk factors in our study included tobacco use, healthy weight, nutritious diet, physical activity, and managing diabetes, cholesterol and blood pressure. Since Life's Simple 7® goals can be achieved through a variety of mechanisms, it was necessary to incorporate an additional framework to ensure a wide variety of CVD prevention interventions were considered. The Prevention Impacts Simulation Model (PRISM) incorporates both individual-level and population-level interventions for CVD prevention, that are either prescriptive or facilitative, described by four broad categories: clinical, behavioral support, taxes and regulation, health promotion and access (20). PRISM is a system dynamics model that is capable of comparing the future impact of changing more than one CVD prevention intervention on population CVD risk through simulations. The benefit of using PRISM as a framework is that it is based on a credible systems model with adaptability to specific socioeconomic factors of different geographic areas (20).
Phase I: existing network of CVD prevention interventions
Social network analysis has been used in public health to examine the social ecological context prior to implementing a specific program, or a broad public health activity, to improve its efficacy (21–23). When addressing health equity as a part of CVD prevention in Denver area communities, key stakeholders conveyed that many diverse CVD prevention programs already existed, but there was no comprehensive list that explained what was already being done. This created a major gap in understanding how to approach health equity without knowing the current system already in place. Defining the system of CVD prevention from the perspective of those involved, was broken down into two components: (1) identify active CVD interventions being implemented in the Denver area, that targeted at least one of Life's Simple 7® activities; (2) understand the organizational context of these CVD interventions. A two-mode network approach was used to describe relationships among CVD interventions and the organizational network in place to implement or deliver them.
Participants
In this phase, semi-structured interviews in the style of a guided survey were conducted on a total of 24 participants from public health, clinical, state and local government, and community organizations (Table 1). A purposive and snowball sampling approach was initiated, with key informants identified as leaders in chronic disease prevention in the Denver area. These key informants were asked to identify activities and organizations they thought were vital to their organization's local work preventing CVD. Organizations they named were then contacted, introduced to the study, and invited to identify additional activities and organizations, using the same criteria. We conducted interviews until CVD interventions from all four PRISM categories had been included.
Network analysis methods with preliminary results
From April to October of 2021, we conducted semi-structured interviews (Supplementary Table S1). Interviews lasted up to 45 min and were transcribed. Participants were asked to name all of the CVD interventions with which they directly worked. Interventions were organized by a PRISM model category, and which of Life's Simple 7® activities were targeted. When interventions could not be categorized within the frameworks provided, a new category was created. Participants also listed up to eight primary collaborators, defined as anyone working outside their immediate department, division, or organization, who helped with implementing each intervention, and the strength of their relationship. If there were more than eight collaborators, participants provided an estimate of total collaborators to understand the breadth of collaboration. If time allowed, follow-up questions were asked about specific CVD interventions to glean in-depth knowledge. Usually there was only sufficient time to ask follow-up questions for one or two of the CVD interventions listed.
We identified a total of 89 active CVD interventions in the Denver area. To give an example of results, we will focus on the 29 active interventions that specifically worked towards the goal of “Quit Tobacco” from Life's Simple 7® (Table 2). Of the 29 interventions, 38% were classified as clinical, 38% as behavioral support, 31% as health promotion and access, and 21% as policy (taxes and regulation), though several overlapped in more than one PRISM category. Network analysis demonstrated which “Quit Tobacco” interventions had the greatest quantity of collaboration, and which types of organizations were more often collaborating with each other (Figure 1).
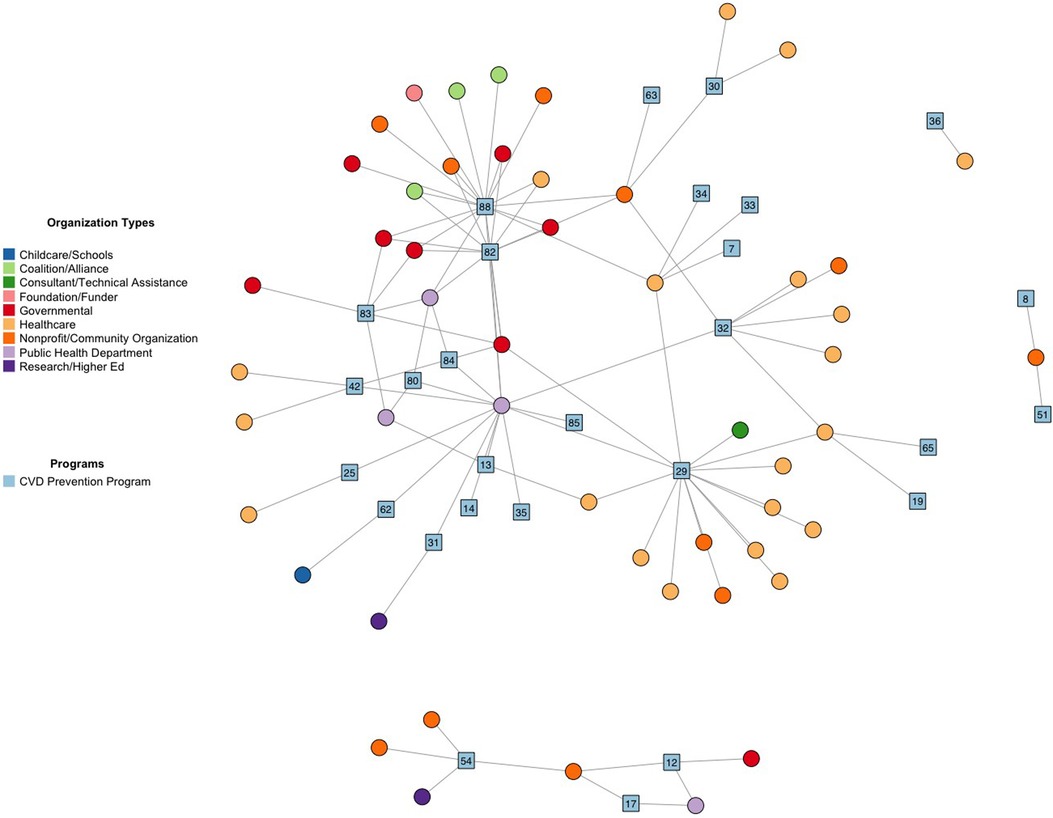
Figure 1. Social network plot of key partnerships implementing “Quit Tobacco” prevention activities in denver area. “Quit Tobacco” prevention programs are blue squares. Implementing organizations are circles. Participants were allowed to identify up to 8 key partnerships represented by undirected lines. Coalitions represent a large group of organizations up to 100+.
E-Referral to the Colorado Quitline (#29) was emphasized in interviews as a key clinical resource that was heavily promoted through media campaigns, and had multiple healthcare and community non-profit organizations working with the program (Table 2 and Figure 1). The Tobacco Flavor Ban (88) was a new policy proposal that several coalitions, health and community non-profits, and government agencies were working on to draft and pass. Coalitions were often large groups, ranging from dozens to over a hundred collaborating organizations, that were commonly involved in working on new policy proposals. Existing legislation, such as the Underage Tobacco Sales Compliance Program (83) or the Colorado Clean Indoor Air Act (84), primarily involved only public health departments and government agencies for implementation. Several behavioral and clinical interventions had only one to a few organizations working on them.
Phase II: health disparities related to CVD risk factors
The goal of Phase II was to understand the complex social and environmental context for health disparities, that affect CVD risk and outcomes across communities in the Denver area. Group model building is a participatory systems science method that visualizes community context through the visual aid of causal loop diagrams (12, 13, 24–26). The process of group model building is iterative, working with a variety of stakeholders to help articulate a problem and build shared understanding around it (24–26). Emphasis is placed on shared understanding from experience, rather than requiring evidence-based knowledge. However, it is possible to incorporate or compare causal loop diagram results with evidence-based knowledge, through initial or follow-up literature reviews. The causal loop diagram is the end product, made up of interconnected factors that influence each other and lead to a primary outcome, creating an overall picture of a problem's complexity (10–12, 27). Group model building was used in this phase, in order to develop causal loop diagrams that capture how health disparities related to CVD are experienced in the Denver area by marginalized populations.
Participants
A total of 14 participants from public health, clinical, or community groups were involved in group model building sessions (Table 1). Some participants from Phase I were asked to participate in Phase II. Purposive and snowball sampling were used to identify additional stakeholders. All community members had personal experience or interest in CVD prevention in their communities, but it was not a requirement to participate. Group model building sessions continued until thematic saturation had been reached, meaning no new additional factors contributing to health disparities were identified, and responses had become repetitive.
Group model building and causal loop diagram methods with preliminary results
From April to June of 2022, we conducted 7 virtual group model building sessions with 2 to 3 participants. Sessions lasted for up to 90 min. Variables for causal loop diagrams were captured for four of the Life's Simple 7® risk factors: not smoking, healthy eating, physical activity, and healthy weight. These were selected because they are lifestyle behaviors that generally promote health, to reduce risk of CVD without necessarily having developed CVD nor requiring clinical care (28). We intend to continue future studies that incorporate the clinical behaviors of managing blood pressure, controlling cholesterol, and reducing blood sugar.
Before a participant was scheduled to attend a virtual session, they were sent a short video introducing the main concepts behind causal loop diagrams to help set expectations. A publicly-shared script from Scriptapedia, for causal mapping with seed structure, was modified to guide the structure of the group model building sessions (29). At each session, the research team would provide at minimum a modeler and a facilitator. When possible, a recorder was also present to take notes, but all sessions were virtually recorded for future transcription. The modeler drew causal loop diagrams in real time, based on group feedback using the online application Miro Online Whiteboard (no version provided). Our modeler was a principal investigator who had the most experience with group model building. The facilitator presented and supported discussion around the main problem of health disparities related to CVD risk. Presentation of the problem was standardized, by using a script developed by the research team, to easily explain important concepts around health disparities and the goal of the research study (Supplementary Script S1).
Groups were provided with an example of a seed model, and a list of evidence-based factors from literature review, that contribute to CVD health disparities for each of the four CVD prevention goals (Supplementary Figure S1). The facilitator emphasized that the seed model and factors were merely prompts to consider, but groups were encouraged to generate their own ideas based on experience, and even disagree with or leave out the prompts. When groups listed immediate factors contributing to disparities, they were also encouraged to consider upstream factors, to generate relationships among factors represented by arrows. Follow-up questions and verifying accurate translation of participants' responses further supported discussion.
Using “Quit Tobacco” as an example of how causal loop diagrams were built, the research team combined the seven group models constructed during sessions into one diagram. First, a comprehensive list of all factors contributing to disparities in tobacco use prevalence in the Denver area was created, with immediate factors contributing to tobacco use prevalence organized into a left-hand column, and upstream factors organized into right-hand columns. Our research team found the easiest starting point was to build several seed models that were able to focus on a specific disparity contributing to differences in tobacco use prevalence, such as stress and differential access to stress coping mechanisms (Figure 2). In this way, causal loop diagrams could incorporate sufficient detail to reflect participants' experiences and distinguish between disparities showing up in different socio-ecological levels (e.g., individual-level disparities vs. community-level disparities). Future work will experiment with building a unified causal loop diagram for each CVD prevention risk factor, and compare them to evidence-based knowledge.
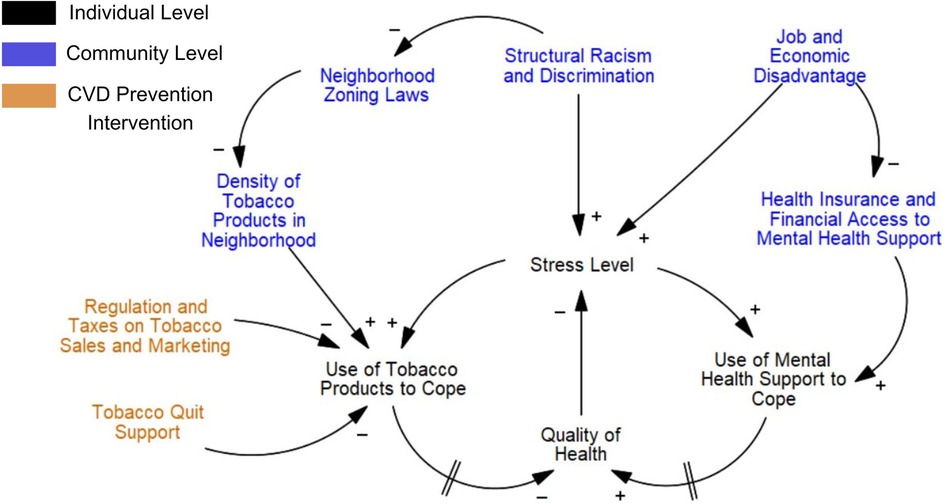
Figure 2. Causal loop diagram example on health disparities in tobacco use in the Denver area, focused on stress. This causal loop diagram was developed based on the experiences of participants. The goal is to understand why there is a geographic disparity in tobacco use prevalence between communities. Differences in factors contributing to stress, and then subsequent differences in access to tobacco products and mental health services to reduce stress levels, create a disparity in tobacco use prevalence. Arrows indicate the direction of relationships between factors. A positive symbol signifies a positive correlation. A negative symbol signifies an inverse correlation. Two dashes on an arrow reflect a delay in outcome.
Combining Phase I and Phase II preliminary results
Combining qualitative, network and group model building analysis produced a rich understanding of how the current CVD prevention system within a geographic area is being experienced differently, and potential sources of modification to reduce disparities identified by the community. For example, in Phase II group model building, participants frequently brought up stress and general mental well-being as a key part of understanding tobacco addiction (Figure 2). One community member stated, “The issue is the community doesn’t want to talk about smoking, they want to talk about mental health, and mental health is directly related to smoking.” Another community member discussed that therapists did not seem trained to address tobacco addiction treatment as a part of stress management, a gap in mental health and medical services he wanted to bridge.
“Quit Tobacco” interventions identified in Phase I qualitative and network analysis did not mention stress as an intervention focus nor disparities in mental health access. While many “Quit Tobacco” interventions were implemented at a population level and were considered a form of policy, the focus of interventions were primarily on reducing access to tobacco products. A difference in neighborhood zoning laws that can restrict retail tobacco sales, was addressed in both research phases as a key disparity current tobacco interventions are considering. However, current interventions are not coupled with ways to increase access to other forms of stress relief within communities, such as mental health access, for communities facing high levels of overall stress. By using multiple systems analysis approaches, it became evident when public health efforts aligned with community need, or when they fundamentally lacked overlap.
The combination of qualitative information from network analysis and group model building demonstrated that those working closely with community members while implementing CVD interventions were often aware of root causes for health disparities identified by community members. However, they did not always feel empowered to address root causes. One expert expressed frustration when trying to reverse geographic trends in tobacco retail density that was related to greater infractions in tobacco youth sales, “To be able to go back and get those zoning laws changed and get those proximity restrictions added after the fact when things are already there, it’s an even harder battle uphill.” Instead, there was a greater focus on policy to improve enforcement of restricting underage tobacco sales.
Similarly, there was recognition that tobacco cessation programs were trying to improve outreach to marginalized communities by increasing diversity in campaigns, ads and staffing, but this did not address the core distrust in healthcare institutions and programs from historical and current racism. As one expert commented, “There may be a little more effectiveness if these programs are designed inherently in the community and driven by them, not just faces on ads or screens, and I’m not saying that we don’t have enough minorities working in these spaces, but I’m talking about the overall design.”
Discussion
When addressing CVD prevention to improve population health, there is a growing emphasis on policy, systems, and environmental (PSE) approaches to be more effective, and better reach marginalized populations left out of clinical or individual-level interventions (3, 4, 6, 7). It is important to recognize, though, that PSE approaches are not inherently designed to fix population health disparities, unless making those disparities a focal point. Our community case study demonstrated that, within a geographic area classified as having high CVD risk, there were 29 active “Quit Tobacco” interventions out of a total of 89 active CVD prevention interventions. Of the “Quit Tobacco” interventions, 52% were identified as being implemented at the population level, with 21% specifically focused on policy interventions.
Regardless of population- or individual-level approaches, there was tension from both leaders in CVD prevention and community members that tobacco use interventions were insufficient to address root causes for tobacco addiction. Addressing disparities as a key component of this study illuminated additional considerations in tobacco use prevention, such as disparities in neighborhood zoning laws predicting tobacco product prevalence, the stress burden associated with racism and economic disadvantage, and disparities in accessing mental health services.
Based on the group model building sessions, participants talked about stress as being an underlying factor contributing to tobacco addiction that should be recognized within programs and activities. Several strategies were identified for shifting activities to address stress as a part of disparities in tobacco addiction. These included: increasing access to mental health services, and bridging training with mental health providers related to quit tobacco strategies. Colorado has recently launched a statewide mental wellness program, for youth to access a limited number of free counseling sessions, called I Matter, as well as a crisis hot line for suicide and substance use disorder interventions at any age (30, 31). It demonstrates potential policy changes that could be expanded to replace the Colorado Quitline, a call line solely focused on quit tobacco strategies, that has a high degree of collaboration among leaders in CVD prevention and is heavily promoted in Denver. Instead, call lines focusing on offering free or reduced-cost mental health interventions could be more effective and still allow for quit tobacco strategies to be incorporated.
Systems studies on chronic disease prevention have found that when focusing on health disparities in a prevention system, new key factors are often uncovered to explain the health disparity that had not previously been considered in prevention efforts (11, 14, 15). When considering health equity in tobacco control, a causal loop diagram built from literature review found similar root causes contributing to disparities as our study, such as structural racism, low-wage work, and tobacco retailer density (11). This suggests changing the focus of CVD interventions to root causes driving tobacco use disparities, such as low-wage work. In contrast, without a systems approach, a more common way suggested to achieve health equity in tobacco studies, is to tailor interventions to high-risk populations. This may include mobile cessation programs that are more accessible for low-income populations, or catering cessation language to high-risk demographics (32–34). Either a systems or non-systems approach to health equity may be effective, but it is important to recognize that they solve different problems.
It is worth further exploration to better understand why root causes for CVD-risk disparities are not showing up in the overall goals and design of CVD prevention research and interventions. Our study used the Life's Simple 7® framework, a common list of lifestyle and clinical goals to promote evidence-based practices that reduce CVD risk in individuals (18, 35, 36). Limiting the type of CVD interventions included in our Phase I to evidence-based practices ended up mostly excluding interventions that would address root cause disparities in tobacco use. PRISM, the second framework we used in our study, does acknowledge that racism or socio-economic status, for example, are known to have significant impacts on CVD population health outcomes, but these factors are currently used solely to understand differences in impact of CVD interventions, rather than be built into the model itself for intervention (20). This underscores the important influence of framework choice on guiding CVD prevention research and interventions.
Once we integrated more than one systems science method in our study, root causes for CVD risk disparities were discussed, and the full scale of the disparities were better understood. Systems science methods are still relatively new to prevention science research, and using more than one systems science method is even less common (37, 38). After using multiple systems science methods, community health studies on obesity prevention captured a more thorough understanding of community context and helped identify new progress measures to improve intervention efficacy (37, 39). Even outside of health research, multi-method approaches to systems science has been generally found to improve research contributions to solving complex problems (40).
A key goal of the initial phases of our CVD-EPIC study was to develop a shared understanding around health disparities in CVD prevention in a specific geographic location. The results presented in this paper are a first step in considering how to transition current CVD prevention efforts to better address root causes of health disparities in CVD risk. These findings will inform local public health experts and community members engaged in CVD prevention work.
Limitations
There are several limitations to consider. While this was a multi-methods two-phase study, it was not exhaustive and it is possible that there are additional CVD interventions that were not identified, or CVD prevention organizations that were overlooked. Public health delivery can vary widely across the U.S. based on several factors such as population size, governance, and financing (41). The Denver area is within a specific state and local public health jurisdiction, in a metropolitan area that may have different capacity for public health delivery compared to rural counties or counties within other states, that may impact results.
We found several benefits to using systems science methods to have a more comprehensive understanding of CVD prevention at the community level, however, analysis is time intensive. The time to process and analyze data was considerably longer than the data collection itself, underscoring the need for collaborative CVD prevention research teams and appropriate funding even at a pilot stage. Ideally, we had hoped to get feedback from group model building participants to improve internal validity of causal loop diagrams, for example, but we ran out of time. Instead, we will rely on evidence-based knowledge for comparison.
Conclusion
Multiple systems science methods were useful to better understand geographic disparities in CVD risk across communities in the Denver area. A large number of interventions at the population and individual level to reduce CVD risk were found to already exist within the Denver area. The approach of this community case study improves understanding of the root causes of disparities in CVD risk that may reorient current CVD interventions to better address health disparities.
Data availability statement
Anonymized data that support the findings of this study are available from the corresponding author, KLB, upon reasonable request.
Author contributions
MPF and KHH contributed to conception and design of the study. KB and MPF developed guided survey. KHH and KAH developed group model building guides. ND, KHH, KAH and KB contributed to group model building data development and refinement. MPF and KAH analyzed qualitative data. KAH conducted social network analysis. KB developed causal loop diagram. KB wrote manuscript. All authors contributed to the article and approved the submitted version.
Funding
This project was supported in part by the U.S. National Heart, Lung and Blood Institute (NHLBI) of the National Institutes of Health under award 5K12HL137862.
Acknowledgments
The authors would like to give special recognition to all study participants for their time and support. The authors thank Jimi Adams, PhD, MA, Leah Frerichs, PhD, MS, and River Chew, MSW, for feedback during the study.
Conflict of interest
The authors declare that the research was conducted in the absence of any commercial or financial relationships that could be construed as a potential conflict of interest.
Publisher's note
All claims expressed in this article are solely those of the authors and do not necessarily represent those of their affiliated organizations, or those of the publisher, the editors and the reviewers. Any product that may be evaluated in this article, or claim that may be made by its manufacturer, is not guaranteed or endorsed by the publisher.
Supplementary material
The Supplementary Material for this article can be found online at: https://www.frontiersin.org/articles/10.3389/fcvm.2023.1216436/full#supplementary-material
References
1. U.S. Department of Health and Human Services, Office of Disease Prevention and Health Promotion. Health Equity in Healthy People 2030. Available at: https://health.gov/healthypeople/priority-areas/health-equity-healthy-people-2030 (August 15, 2022).
2. Egan BM, Li J, Sutherland SE, Jones DW, Ferdinand KC, Hong Y, et al. Sociodemographic determinants of life's simple 7: implications for achieving cardiovascular health and health equity goals. Ethn Dis. (2020) 30(4):637–50. doi: 10.18865/ed.30.4.637
3. Diaz CL, Shah NS, Lloyd-Jones DM, Khan SS. State of the nation's cardiovascular health and targeting health equity in the United States: a narrative review. JAMA Cardiol. (2021) 6(8):963–70. doi: 10.1001/jamacardio.2021.1137
4. Havranek EP, Mujahid MS, Barr DA, Blair IV, Cohen MS, Cruz-Flores S, et al. Social determinants of risk and outcomes for cardiovascular disease: a scientific statement from the American heart association. Circulation. (2015) 132(9):873–98. doi: 10.1161/CIR.0000000000000228
5. Schultz WM, Kelli HM, Lisko JC, Varghese T, Shen J, Sandesara P, et al. Socioeconomic status and cardiovascular outcomes: challenges and interventions. Circulation. (2018) 137(20):2166–78. doi: 10.1161/CIRCULATIONAHA.117.029652
6. Leeman J, Myers AE, Ribisl KM, Ammerman AS. Disseminating policy and environmental change interventions: insights from obesity prevention and tobacco control. Int J Behav Med. (2015) 22(3):301–11. doi: 10.1007/s12529-014-9427-1
7. Institute of Medicine Committee on Public Health Priorities to Reduce and Control Hypertension. A population-based policy and systems change approach to prevent and control hypertension. Washington (DC): National Academies Press (US) (2010). Copyright 2010 by the National Academy of Sciences. All rights reserved.
8. Diez Roux AV. Complex systems thinking and current impasses in health disparities research. Am J Public Health. (2011) 101(9):1627–34. doi: 10.2105/AJPH.2011.300149
10. Brennan LK, Sabounchi NS, Kemner AL, Hovmand P. Systems thinking in 49 communities related to healthy eating, active living, and childhood obesity. J Public Health Manag Pract. (2015) 21(Suppl 3):S55–69. doi: 10.1097/PHH.0000000000000248
11. Mills SD, Golden SD, O'Leary MC, Logan P, Hassmiller Lich K. Using systems science to advance health equity in tobacco control: a causal loop diagram of smoking. Tob Control. (2021) 32(3):287–95. doi: 10.1136/tobaccocontrol-2021-056695
12. Allender S, Brown AD, Bolton KA, Fraser P, Lowe J, Hovmand P. Translating systems thinking into practice for community action on childhood obesity. Obes Rev. (2019) 20(Suppl 2):179–84. doi: 10.1111/obr.12865
13. Waterlander WE, Luna Pinzon A, Verhoeff A, den Hertog K, Altenburg T, Dijkstra C, et al. A system dynamics and participatory action research approach to promote healthy living and a healthy weight among 10-14-year-old adolescents in Amsterdam: the LIKE programme. Int J Environ Res Public Health. (2020) 17(14):4928. doi: 10.3390/ijerph17144928
14. Taylor M, Higgins E, Francis M, Francis H. A multiparadigm approach to developing policy for the location of recreational facilities. Syst Res Behav Sci. (2011) 29(3):240–52. doi: 10.1002/sres.1100
15. Auchincloss AH, Riolo RL, Brown DG, Cook J, Diez Roux AV. An agent-based model of income inequalities in diet in the context of residential segregation. Am J Prev Med. (2011) 40(3):303–11. doi: 10.1016/j.amepre.2010.10.033
16. Goldsteen R, Goldsteen K, Dwelle T. Introduction to public health: Promises and practices. 2nd ed. New York, NY: Springer Publishing Company, LLC (2015).
17. Public Health Institute at Denver Health. About Us. Available at: https://www.phidenverhealth.org/about-us (June 24, 2022).
18. American Heart Association. Life’s Simple 7. Available at: https://www.heart.org/en/healthy-living/company-collaboration/well-being-works-better/lifes-simple-7 (June 24, 2022).
19. American Heart Association. Life’s Essential 8: Your checklist for lifelong good health. Available at: https://www.heart.org/en/healthy-living/healthy-lifestyle/lifes-essential-8 (December 23, 2022).
20. Hirsch G, Homer J, Trogdon J, Wile K, Orenstein D. Using simulation to compare 4 categories of intervention for reducing cardiovascular disease risks. Am J Public Health. (2014) 104(7):1187–95. doi: 10.2105/AJPH.2013.301816
21. Varda DM, Retrum JH. An exploratory analysis of network characteristics and quality of interactions among public health collaboratives. J Public Health Res. (2012) 1(2):170–6. doi: 10.4081/jphr.2012.e27
22. Wholey DR, Gregg W, Moscovice I. Public health systems: a social networks perspective. Health Serv Res. (2009) 44(5 Pt 2):1842–62. doi: 10.1111/j.1475-6773.2009.01011.x
23. Schoen MW, Moreland-Russell S, Prewitt K, Carothers BJ. Social network analysis of public health programs to measure partnership. Soc Sci Med. (2014) 123:90–5. doi: 10.1016/j.socscimed.2014.10.057
24. Frerichs L, Lich KH, Dave G, Corbie-Smith G. Integrating systems science and community-based participatory research to achieve health equity. Am J Public Health. (2016) 106(2):215–22. doi: 10.2105/AJPH.2015.302944
25. Siokou C, Morgan R, Shiell A. Group model building: a participatory approach to understanding and acting on systems. Public Health Res Pract. (2014) 25(1):e2511404. doi: 10.17061/phrp2511404
26. Lembani M, de Pinho H, Delobelle P, Zarowsky C, Mathole T, Ager A. A guide for participatory systems analysis using a group model building approach. London: SAGE Publications Ltd (2020). Available at: https://methods.sagepub.com/case/guide-participatory-systems-analysis-using-group-model-building-approach
27. McGlashan J, Johnstone M, Creighton D, de la Haye K, Allender S. Quantifying a systems map: network analysis of a childhood obesity causal loop diagram. PLoS One. (2016) 11(10):e0165459. doi: 10.1371/journal.pone.0165459
28. Claas SA, Arnett DK. The role of healthy lifestyle in the primordial prevention of cardiovascular disease. Curr Cardiol Rep. (2016) 18(6):56. doi: 10.1007/s11886-016-0728-7
29. Andersen DF, Calhoun A, Hovmand PS, Hower T, Rouwette E, Steins E, et al. Scriptapedia. Available at: https://en.wikibooks.org/wiki/Scriptapedia (October 16, 2021).
30. Colorado Crisis Services. I Matter.: Colorado Behavioral Health Administration. Available at: https://imattercolorado.org/ (June 12, 2023).
31. Colorado Crisis Services. Whatever You're Going Through, We'll Help You Through It: Colorado Behavioral Health Administration. Available at: https://coloradocrisisservices.org/ (June 12, 2023).
32. Costa A, Cortes M, Sena C, Nunes E, Nogueira P, Shivaji T. Equity-focused health impact assessment of Portuguese tobacco control legislation. Health Promot Int. (2018) 33(2):279–87. doi: 10.1093/heapro/daw076
33. Battalio SL, Pfammatter AF, Kershaw KN, Hernandez A, Conroy DE, Spring B. Mobile health tobacco cessation interventions to promote health equity: current perspectives. Front Digit Health. (2022) 4:821049. doi: 10.3389/fdgth.2022.821049
34. Kong AY, Henriksen L. Retail endgame strategies: reduce tobacco availability and visibility and promote health equity. Tob Control. (2022) 31(2):243–9. doi: 10.1136/tobaccocontrol-2021-056555
35. Pennant M, Davenport C, Bayliss S, Greenheld W, Marshall T, Hyde C. Community programs for the prevention of cardiovascular disease: a systematic review. Am J Epidemiol. (2010) 172(5):501–16. doi: 10.1093/aje/kwq171
36. Soltani S, Saraf-Bank S, Basirat R, Salehi-Abargouei A, Mohammadifard N, Sadeghi M, et al. Community-based cardiovascular disease prevention programmes and cardiovascular risk factors: a systematic review and meta-analysis. Public Health. (2021) 200:59–70. doi: 10.1016/j.puhe.2021.09.006
37. Hennessy E, Economos CD, Hammond RA. Integrating complex systems methods to advance obesity prevention intervention research. Health Educ Behav. (2020) 47(2):213–23. doi: 10.1177/1090198119898649
38. Lich KH, Ginexi EM, Osgood ND, Mabry PL. A call to address complexity in prevention science research. Prev Sci. (2013) 14(3):279–89. doi: 10.1007/s11121-012-0285-2
39. Appel JM, Fullerton K, Hennessy E, Korn AR, Tovar A, Allender S, et al. Design and methods of shape up under 5: integration of systems science and community-engaged research to prevent early childhood obesity. PLoS One. (2019) 14(8):e0220169. doi: 10.1371/journal.pone.0220169
40. Zolfagharian M, Romme G, Walrave B. Combining system dynamics modeling with other methods: A systematic review. (2015).
Keywords: health status disparities, health equity, systems analysis, systems theory, cardiovascular diseases/prevention & control, social determinants of health
Citation: Bauer KL, Haapanen KA, Demeke N, Fort MP and Henderson KH (2023) Increasing use of systems science in cardiovascular disease prevention to understand how to address geographic health disparities in communities with a disproportionate burden of risk. Front. Cardiovasc. Med. 10:1216436. doi: 10.3389/fcvm.2023.1216436
Received: 3 May 2023; Accepted: 26 June 2023;
Published: 13 July 2023.
Edited by:
Maria Grazia Modena, University of Modena and Reggio Emilia, ItalyReviewed by:
Chiara Leuzzi, IRCCS Local Health Authority of Reggio Emilia, ItalyElisa Lodi, University Hospital of Modena, Italy
© 2023 Bauer, Haapanen, Demeke, Fort and Henderson. This is an open-access article distributed under the terms of the Creative Commons Attribution License (CC BY). The use, distribution or reproduction in other forums is permitted, provided the original author(s) and the copyright owner(s) are credited and that the original publication in this journal is cited, in accordance with accepted academic practice. No use, distribution or reproduction is permitted which does not comply with these terms.
*Correspondence: Kyla L. Bauer a3lsYS4yLmJhdWVyQGN1YW5zY2h1dHouZWR1
†These authors have contributed equally to this work and share senior authorship