- 1Shandong Academy of Occupational Health and Occupational Medicine, Shandong First Medical University & Shandong Academy of Medical Sciences, Jinan, China
- 2School of Acupuncture and Tuina, Shandong University of Traditional Chinese Medicine, Jinan, China
- 3Neck-Shoulder and Lumbocrural Pain Hospital of Shandong First Medical University, Shandong First Medical University & Shandong Academy of Medical Sciences, Jinan, China
- 4Department of Development Planning and Discipline Construction, Shandong First Medical University & Shandong Academy of Medical Sciences, Jinan, China
Background: Osteoarthritis (OA) is a degenerative disease with high prevalence. Some observational studies have shown that patients with osteoarthritis often have co-existing cardiovascular diseases (CVD) such as atrial fibrillation (AF) and coronary atherosclerosis (CA). However, there is still a lack of stronger evidence confirming the association between osteoarthritis and cardiovascular disease. In this study, we used a bidirectional two-sample Mendelian randomization study to investigate the relationship between OA with AF and CA.
Methods: OA data from the UK Biobank and arcOGEN (Arthritis Research UK Osteoarthritis Genetics, a study that aimed to find genetic determinants of osteoarthritis and elucidate the genetic architecture of the disease) integration were selected for the study (n = 417,596), AF data were obtained from six studies (n = 1,030,836), and coronary atherosclerosis data were derived from the FinnGen (n = 218,792). MR analysis was performed primarily using the Inverse variance weighted (IVW) method, with MR Egger, weighted median, simple mode, weighted mode as supplements, sensitivity analysis was performed using Cochran Q statistic, and leave-one-out analysis.
Results: We found that OA and AF were positively associated [IVW: OR (95% CI): 1.11 (1.04, 1.19), P = 0.002], while OA and CA were negatively associated [IVW: OR (95% CI): 0.88 (0.79, 0.98), P = 0.02]. In the reverse MR analysis, no effect of AF on OA was found [IVW: OR (95% CI): 1.00 (0.97, 1.03), P = 0.84], meanwhile, CA and OA were found to be associated negatively [IVW: OR (95% CI): 0.95 (0.92, 0.99), P = 0.01]. No violations of MR assumptions were found in the sensitivity analysis.
Conclusion: This research confirms that OA is a risk factor for AF, and there is a mutual protective factor between OA and CA. However, further studies are still necessary to elucidate the underlying mechanisms.
1. Introduction
Osteoarthritis is a common degenerative joint disease mainly caused by cartilage injury (1). Established risk factors for osteoarthritis include age, obesity, inflammation, trauma, and genetic factors (2). The prevalence of OA is increasing with the global aging population. It is estimated to affect 250 million people worldwide (3).
Cardiovascular disease (CVD), one of the primary causes of death in the global population, includes both heart and vascular diseases such as arrhythmias and coronary artery disease (CAD) (4). As one of the most frequent arrhythmias, atrial fibrillation (AF) is a major risk factor for death, stroke and other diseases, and the incidence is on the rise over the years (5). According to the Framingham Heart Study (FHS), the incidence of AF has increased threefold over the past 50 years (6). Palpitations, weakness, dizziness and breathlessness are the main clinical symptoms of atrial fibrillation (7). Age is a significant risk factor for atrial fibrillation, with a prevalence of up to 10% in people over the age of 75, which is the same risk factor as for osteoarthritis. Atherosclerotic coronary artery disease is the leading cause of death from cardiovascular disease, accounting for almost 45% of all cases (8). The main clinical symptom of coronary atherosclerosis is exertional angina, sometimes with chest tightness. It's also common in the elderly (9).
In an observational study, arthritis is found to be a common comorbidity in patients with CVD [20.9% (19.5%–23.5%)] (10). A meta-analysis shows that OA is related to an increased risk of cardiovascular disease (11). Although epidemiology has linked OA and CVD, confounders such as age, obesity, sex, use of NSAIDs, and inflammation may affect the conclusions of observational studies. Furthermore, for both ethical and practical reasons, the relationship between OA and CVD cannot be verified by randomized clinical trials (12). Thus, the true causal relationship is still unclear.
Genome-Wide Association Study (GWAS) is a multicenter, large-sample, iterative validation study of gene-disease associations at the genome-wide level (13). Mendelian Randomization (MR) is an experimental method widely used in epidemiology to analyze the causal relationship between exposure and outcome factors by introducing an instrumental variable (iv) as an intermediate variable (14). Based on Mendel's law of independent distribution, gene-disease associations are not confounded by common confounders such as postnatal environment, socioeconomic status, behavioral factors, or reverse causality, and estimates of effects are much closer to the true picture (15). With the lack of randomized clinical trials, Mendelian randomization is an essential method for making inferences about causality.
Based on existing genetic databases, genetic variants controlling the pathogenesis of OA were used as instrumental variables to further investigate the effect of OA on the risk of developing atrial fibrillation and coronary artery disease. Our study aimed to elucidate the potential contribution of OA to both diseases at the genetic level by a two-sample MR method.
2. Materials and methods
2.1. Methods
We used a bidirectional two-sample Mendelian randomization method to confirm whether there is a causal association between OA with AF and CA. In order to ensure the results were reliable, the MR analysis had to meet the following three key assumptions: (1) the genetic effects in the analysis have a very strong association with exposure; (2) the instrumental variables, as exposure factors, should affect the outcome via the exposure factors we studied exclusively; and (3) Instrumental variables should be independent of confounders of exposure and outcome (16). Single nucleotide polymorphisms (SNPs) that strictly met the above three conditions were considered as iv (17). Figure 1 shows the primary description of the method.
2.2. Data sources
The OA data were obtained from a combination of UK Biobank and arcOGEN resources, including 39,427 cases of knee or hip osteoarthritis and a control sample of 378,169 (18).
Data on atrial fibrillation were obtained from a total of 60,620 cases of European ancestry and 970,216 controls from six contributing studies (The Nord-Trøndelag Health Study (HUNT), deCODE, the Michigan Genomics Initiative (MGI), DiscovEHR, UK Biobank, and the AFGen Consortium) (19).
Coronary atherosclerosis data were obtained from FinnGen (https://www.finngen.fi). 23,363 cases and 195,429 controls were included (20).
All data derived from publicly available GWAS data, which are available on the Integrative Epidemiology Unit (IEU) GWAS database (https://gwas.mrcieu.ac.uk), with no need for informed consent of the participant. Table 1 provides specific information on all materials.
2.3. SNP selection
For each SNP independent variable, defined as those without linkage disequilibrium, we set a genome-wide significance threshold P-value (P < 5 × 10−8), a linkage disequilibrium correlation coefficient r2 (r2 < 0.001), and the number of bases between two SNPs (kb > 10,000), with further quality control based on minor allele frequency >1%. Finally, 24 OA-associated SNPs (Table 2), 23 CA-associated SNPs (Table 3), and 108 AF-associated SNPs were extracted respectively (Supplementary File S1). In Mendelian randomization studies, weak instrumental variable bias is a very important issue. Weak instrumental variables are genetic variants that have low explanatory power for exposure. To avoid the presence of this bias, the F-statistic was introduced to assess this effect. No SNP with F (calculated as ) <10 were found in the results (21).
2.4. Statistical analyses
On the basis of the above data, we used the two-sample MR method to analyze the relationship between OA with AF and CA. The main analysis method used was inverse variance weighted (IVW) analysis, which assumed that all SNPs are valid instrumental variables and provide the most accurate estimates. The results obtained by IVW had high statistical power when all SNPs met the three main hypotheses (22). Meanwhile, to complement the results, four other methods were selected (MR Egger, weighted median, simple model, and weighted model) (23). Median estimates include weighted median, simple mode, and weighted mode. The weighted median method can provide consistent estimates when more than 50% of the weight comes from valid instrument variants. MR-Egger regression is able to generate estimates after accounting for horizontal pleiotropy, but with a lower degree of precision.
To make sure that the results were accurate, we used the Cochran's Q test to evaluate whether there was heterogeneity between the individual SNPs, with a P-value <0.05 judging the presence of heterogeneity in the results. MR Egger analysis was used to assess the multiplicity of SNPs, which can be considered absent if P > 0.05. In addition, leave-one-out analysis was conducted to determine if there was an instrumental variable with more influence on the results. The R package (TwoSampleMR) in R version 4.2.2 was used for all analyses.
3. Results
3.1. The causal effect between OA and AF
Based on the results of the IVW shown in Table 4, we found that OA increased the incidence of AF (IVW: OR (95% CI): 1.11 (1.04, 1.19), P = 0.002), which was consistent with the results of the Weighted median and Simple mode (Table 4). The results of the heterogeneity and pleiotropy tests were presented in Table 5, with P > 0.05, which could indicate that there was no heterogeneity or pleiotropy in the study. The scatter plot can visualize the positive associations between OA and CA (Figure 2A). The funnel plot (Figure 3A) is symmetric and consistent with the results of the heterogeneity analysis. The Forest plot of the combination of single SNP effects could be found in Figure 4A. According to the results of the leave-one-out analysis (Figure 5A), no SNPs with significant effects could be identified, which showed the stability of the results. Therefore, we were able to consider OA as a risk factor for AF.
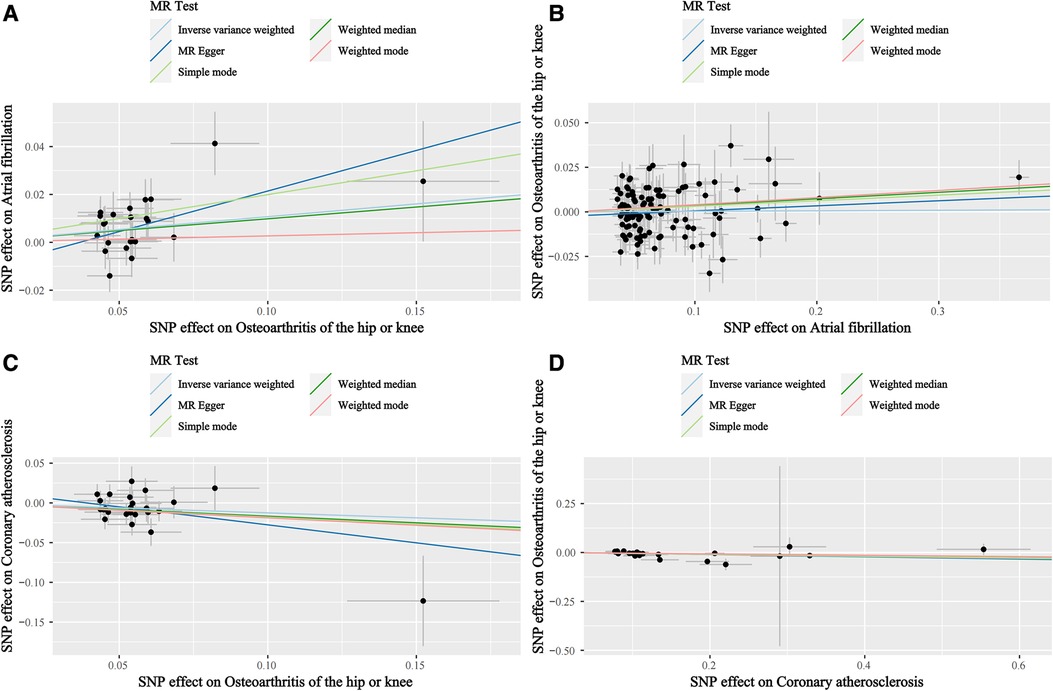
Figure 2. Scatterplots. (A) Scatterplots of the causal relationships between osteoarthritis and atrial fibrillation. (B) Scatterplots of the causal relationships between atrial fibrillation osteoarthritis and osteoarthritis. (C) Scatterplots of the causal relationships between osteoarthritis and coronary atherosclerosis. (D) Scatterplots of the causal relationships between coronary atherosclerosis and coronary atherosclerosis osteoarthritis.
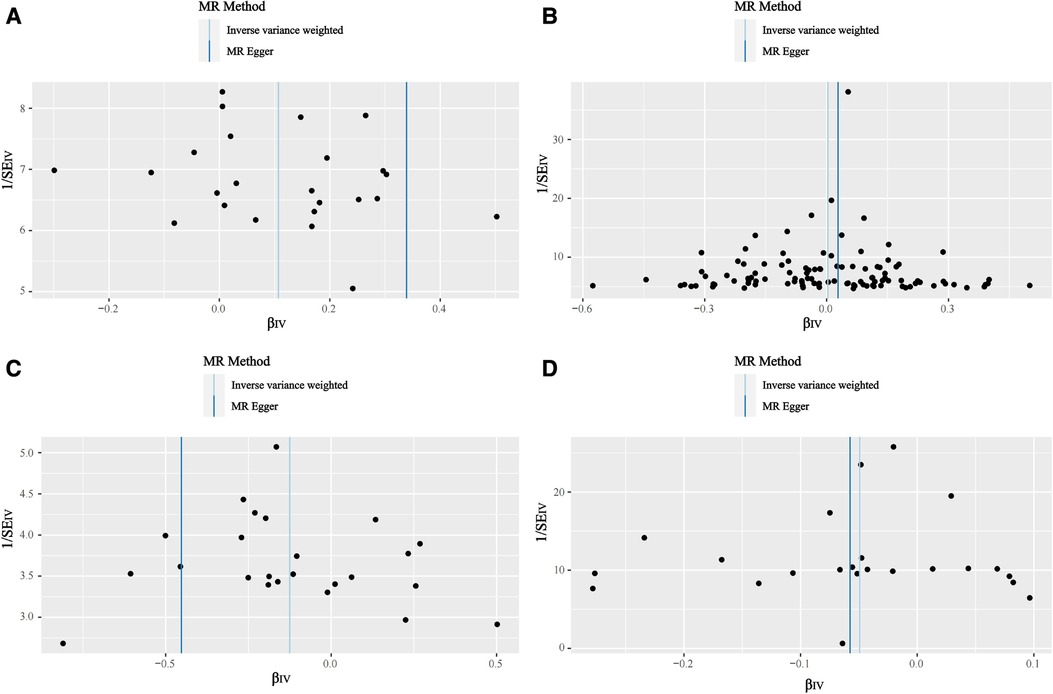
Figure 3. Funnel plot. (A) Funnel plot of the causal relationships between osteoarthritis and atrial fibrillation. (B) Funnel plot of the causal relationships between atrial fibrillation osteoarthritis and osteoarthritis. (C) Funnel plot of the causal relationships between osteoarthritis and coronary atherosclerosis. (D) Funnel plot of the causal relationships between coronary atherosclerosis and coronary atherosclerosis osteoarthritis.
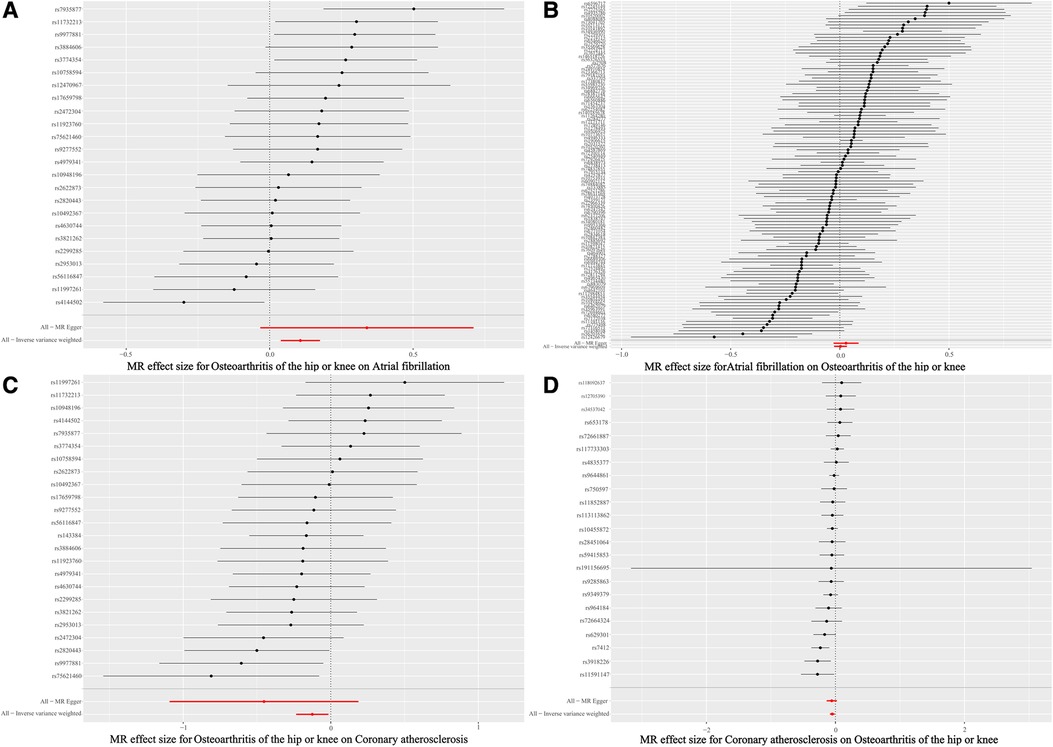
Figure 4. Single SNP effect combination forest plot. (A) Single SNP effect combination forest plot of the causal relationships between osteoarthritis and atrial fibrillation. (B) Single SNP effect combination forest plot of the causal relationships between atrial fibrillation osteoarthritis and osteoarthritis. (C) Single SNP effect combination forest plot of the causal relationships between osteoarthritis and coronary atherosclerosis. (D) Single SNP effect combination forest plot of the causal relationships between coronary atherosclerosis and coronary atherosclerosis osteoarthritis.
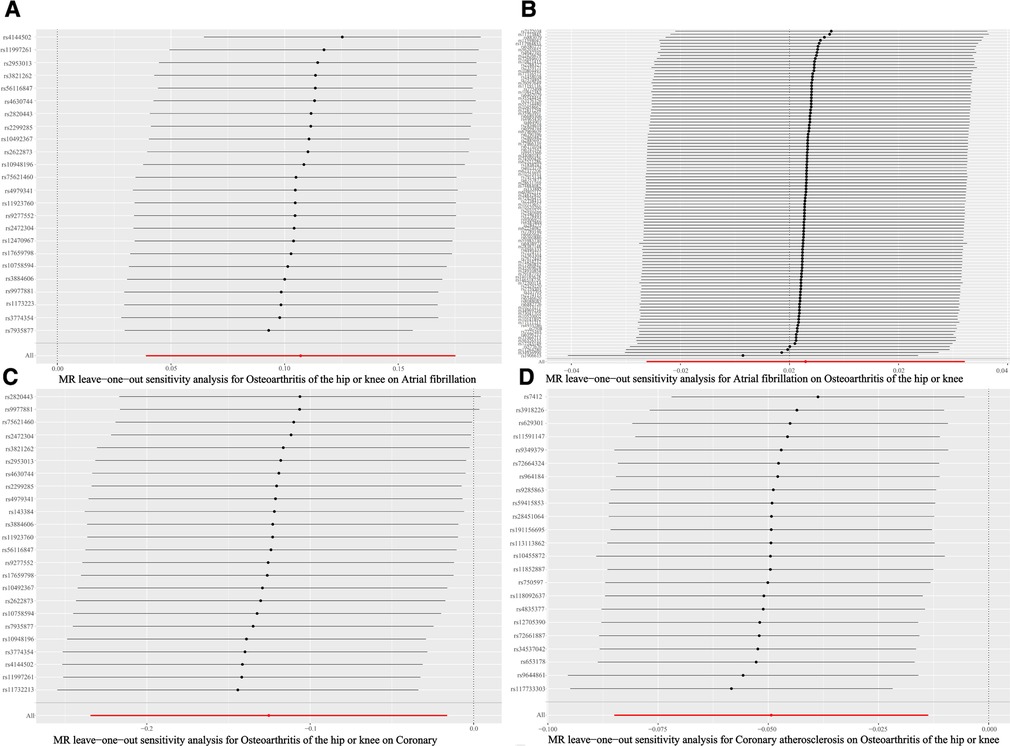
Figure 5. Forest plot of leave-one-out analysis. (A) Forest plot of leave-one-out analysis of the causal relationships between osteoarthritis and atrial fibrillation. (B) Forest plot of leave-one-out analysis of the causal relationships between atrial fibrillation osteoarthritis and osteoarthritis. (C) Forest plot of leave-one-out analysis of the causal relationships between osteoarthritis and coronary atherosclerosis. (D) Forest plot of leave-one-out analysis of the causal relationships between coronary atherosclerosis and coronary atherosclerosis osteoarthritis.
In contrast, AF has no effect on OA. The results were shown in Table 4, with P-values >0.05 for all five statistical methods.
3.2. The causal effect between OA and Ca
The results of the five statistical methods for the assessment of the causal relationship between OA and CA were shown in Table 4, indicating that OA was a protective factor for CA (IVW: OR (95% CI): 0.88 (0.79, 0.98), P = 0.02; Weighted median: OR (95% CI): 0.85 (0.73, 0.99), P = 0.03). P-values for heterogeneity and pleiotropy were >0.05 (Table 5), indicating that there was no heterogeneity or pleiotropy in the study. Scatter plots could be found in Figure 2C, forest plots of the combination of single SNP effects in Figure 4C, funnel plots in Figure 3C, and leave-one-out analysis plots in Figure 5C. The results were stable, and it could be considered that OA was a protective factor for CA.
Meanwhile, a negative associations was observed between CA and OA (Table 4). The results showed no heterogeneity or pleiotropy (Table 5), and according to the leave-one-out analysis results (Figure 5D), the results were stable.
4. Discussion
Using the genetic data in the open database, we performed an MR analysis of the association between OA with AF and CA. According to the results, OA and AF are positively associated, but no effect of AF on OA, while OA and CA are negatively associated with each other, in agreement with the results of some observational studies. A retrospective electronic medical record review from the United States found that osteoarthritis, a common comorbidity of atrial fibrillation, significantly increased the incidence of atrial fibrillation. The total number of cases was 2,433, of which 418 had AF and 216 also had OA (51.7%, P < 0.001) (24). Cross-sectional findings show a small negative association between HOA and cardiovascular events despite increased vascular pathology (25). The study included 2,264 men (mean age 76 years; SD 6) and 3,078 women (mean age 76 years; SD 6). Our study is the first to confirm a causal relationship between OA with AF and CA from a genetic perspective.
At present, the mechanisms of association between the three diseases are unclear. However, the causal relationship can be explained in the following ways. First, there are a number of risk factors in common between OA, AF and CA, such as advanced age and obesity, both of which contribute to the incidence of osteoarthritis and cardiovascular disease. Second, the use of NSAIDs (non-steroidal antiinflammatory drugs) for pain relief is more common in OA, which is also found to be related to an increasing prevalence of CVD (26). The primary mechanism by which NSAIDs cause heart failure is thought to be inhibition of renal prostaglandin synthesis, resulting in afferent small artery vasoconstriction and sodium and fluid retention. Thus, NSAIDs may counteract the physiological mechanisms by which the kidney compensates for impaired cardiac output (27). In contrast, the use of coronary medications leads to a decrease in the incidence of osteoarthritis. While angiotensin-aldosterone system modulators (captopril, enalapril, cloxazine) have both stimulatory and inhibitory effects on cartilage regeneration, beta-adrenergic receptor inhibitors may reduce the negative effects of the adrenergic system on cartilage formation, such as reduced ECM production (28). In addition, the painful symptoms of osteoarthritis have a deleterious effect not only on sedentary behavior, but also on physical activity levels, which are significant risk factors for atrial fibrillation (29). On the contrary, coronary atherosclerosis also reduces the amount of physical activity, which in turn may reduce the load on the knee and hip joints, thereby reducing the incidence of osteoarthritis (30).
The common mechanisms among the three diseases can be analyzed at the molecular level, mainly considering genetic mechanisms and inflammation. From a genetic point of view, a cohort study from the Framingham Offspring Study found that the risk of atrial fibrillation in offspring with at least one parent with atrial fibrillation was 1.85 times higher than without atrial fibrillation, increasing to 3.23 times after adjustment for age of onset of atrial fibrillation below 75 years (31). Familial atrial fibrillation is considered a major risk factor independent of other conventional risk factors (32). MiRNAs can also affect many genes associated with atrial fibrillation, making them viable molecular targets. For example, miR-21 and miR-133 may participate in structural remodeling of the atria through increased fibrosis (33). The Stockholm-Tartu Atherosclerosis Reverse Network Engineering Task (STARNET) study identified 224 gene-regulatory co-expression networks that could explain 54% of CAD heritability (34). A twin study confirmed a significant influence of genetic factors in women with hip osteoarthritis (35). Genetic factors are among the risk factors for all three diseases. There may be common genetic mechanisms, some of which are unknown to us.
Studies have shown that fibrosis is essential for the development of atrial fibrillation and that fibroblasts are important cytokines for atrial fibrosis (36). In particular, a biochemical signal, TGF-β, which is important to induce fibroblast differentiation, mediates the transcription of myofibroblast genes (37). In addition, mitochondria play a key regulatory role in fibroblast activation (38). In the pathogenesis of OA, the mitochondrial pathway has also been implicated in the apoptosis of chondrocytes induced by mechanical stress (39). TGF-β generally plays a chondroprotective role, but under some circumstances it also determines OA-like changes in healthy articular cartilage by promoting the differentiation and apoptosis of terminal chondrocytes (40). We speculate that there may be pathways that operate simultaneously in the development of both OA and AF. In addition, inflammation, which contributes to atrial remodeling and is associated with the occurrence and maintenance of atrial fibrillation, is an essential pathogenic mechanism in both osteoarthritis and atrial fibrillation. Results of a large prospective study suggest that inflammation is a powerful predictor of AF (41). It has been shown that NLRP3 inflammatory vesicle activity is significantly elevated in cardiomyocytes from patients with atrial fibrillation, which has implications for cardiac fibroblast activation (36). Significant inflammatory factors such as IL-6 and CRP have also been independently found to correlate with the incidence of AF (42).
AF, CA, and OA are all highly prevalent in the population and are often referred to as comorbidities (43). AF has a severe effect on the quality of life and is often associated with serious complications that lead to increased mortality, reduced quality of life, and expensive medical costs (44). Confirmation of a causal relationship between OA with AF and CA may help to prevent and manage the disease by screening high-risk groups, thereby reducing morbidity or delaying the disease process. For example, regular physical examinations in patients with osteoarthritis can detect the presence of cardiovascular disease at an early stage. In addition, health education efforts can be made to reduce the incidence of cardiovascular disease through lifestyle changes in patients with osteoarthritis.
The strength of this study is that it confirms for the first time the causal relationship between OA with AF and CA from a genetic perspective, avoiding the potential bias of observational studies. However, there are some limitations to this study as well. First, all data were obtained from the European population, which needs further validation to generalize to other populations. Second, the data were not stratified by sex, age, or disease severity. Nevertheless, the SNPs included in this study all meet the requirements for valid instrumental variables, so our results are reliable.
5. Conclusion
This study confirmed that OA leads to an increased prevalence of AF and decreased prevalence of CA by a bidirectional two-sample Mendelian randomization study. Although the results need to be validated by further clinical and basic experiments, they provide a new perspective for future research on the potential link between OA and CVD.
Data availability statement
The original contributions presented in the study are included in the article/Supplementary Material, further inquiries can be directed to the corresponding authors.
Ethics statement
Ethical review and approval was not required for the study on human participants in accordance with the local legislation and institutional requirements. Written informed consent for participation was not required for this study in accordance with the national legislation and the institutional requirements.
Author contributions
MY and WX designed the Mendelian randomization study, and performed data acquisition, analysis, and interpretation. JP and SX drafted the manuscript. MX and BS performed the observational data acquisition and analysis. HF and GY performed data interpretation. All authors contributed to the article and approved the submitted version.
Funding
This study was supported by Traditional Chinese Medicine high-level Talents Cultivation Project of Shandong Province, the Major Science and Technology Innovation Project of Shandong Province (grant no. 2022CXGC020510, 2021SFGC0502), the Academic Promotion Project of Shandong First Medical University (grant no. 2019QL003), Shandong Province Traditional Chinese Medicine Science and Technology project (grant no. M-2022253), the Shandong Provincial Central Government Guides Local Science and Technology Development Fund Projects (grant no. YDZX20203700002055).
Acknowledgments
We want to acknowledge the participants and investigators of the FinnGen study. We would also like to thank all the investigators and participants who contributed to the GWASs.
Conflict of interest
The authors declare that the research was conducted in the absence of any commercial or financial relationships that could be construed as a potential conflict of interest.
The reviewer JW declared a shared parent affiliation with the authors to the handling editor at the time of review.
Publisher's note
All claims expressed in this article are solely those of the authors and do not necessarily represent those of their affiliated organizations, or those of the publisher, the editors and the reviewers. Any product that may be evaluated in this article, or claim that may be made by its manufacturer, is not guaranteed or endorsed by the publisher.
Supplementary material
The Supplementary Material for this article can be found online at: https://www.frontiersin.org/articles/10.3389/fcvm.2023.1213672/full#supplementary-material
References
1. Allen KD, Thoma LM, Golightly YM. Epidemiology of osteoarthritis. Osteoarthr Cartil. (2022) 30(2):184–95. doi: 10.1016/j.joca.2021.04.020
2. Biver E, Berenbaum F, Valdes AM, De Carvalho IA, Bindels LB, Brandi ML, et al. Gut microbiota and osteoarthritis management: an expert consensus of the European society for clinical and economic aspects of osteoporosis, osteoarthritis and musculoskeletal diseases (ESCEO). Ageing Res Rev. (2019) 55. doi: 10.1016/j.arr.2019.100946
3. Hunter DJ, Bierma-Zeinstra S. Osteoarthritis. Lancet. (2019) 393(10182):1745–59. doi: 10.1016/S0140-6736(19)30417-9
4. Hindricks G, Potpara T, Dagres N, Arbelo E, Bax JJ, Blomstrom-Lundqvist C, et al. 2020 ESC guidelines for the diagnosis and management of atrial fibrillation developed in collaboration with the European association for cardio-thoracic surgery (EACTS): the task force for the diagnosis and management of atrial fibrillation of the European society of cardiology (ESC) developed with the special contribution of the European heart rhythm association (EHRA) of the ESC. Eur Heart J. (2021) 42(5):373–498. doi: 10.1093/eurheartj/ehaa612
5. Vizzardi E, Curnis A, Latini MG, Salghetti F, Rocco E, Lupi L, et al. Risk factors for atrial fibrillation recurrence: a literature review. J Cardiovasc Med. (2014) 15(3):235–53. doi: 10.2459/JCM.0b013e328358554b
6. Schnabel RB, Yin X, Gona P, Larson MG, Beiser AS, Mcmanus DD, et al. 50 Year trends in atrial fibrillation prevalence, incidence, risk factors, and mortality in the Framingham heart study: a cohort study. Lancet. (2015) 386(9989):154–62. doi: 10.1016/S0140-6736(14)61774-8
7. Baman JR, Passman RS. Atrial fibrillation. JAMA. (2021) 325(21):2218. doi: 10.1001/jama.2020.23700
8. Benjamin EJ, Virani SS, Callaway CW, Chamberlain AM, Chang AR, Cheng S, et al. Heart disease and stroke statistics-2018 update: a report from the American heart association. Circulation. (2018) 137(12):e67–492. doi: 10.1161/CIR.0000000000000558
9. Weber C, Noels H. Atherosclerosis: current pathogenesis and therapeutic options. Nat Med. (2011) 17(11):1410–22. doi: 10.1038/nm.2538
10. Tran J, Norton R, Conrad N, Rahimian F, Canoy D, Nazarzadeh M, et al. Patterns and temporal trends of comorbidity among adult patients with incident cardiovascular disease in the UK between 2000 and 2014: a population-based cohort study. PLoS Med. (2018) 15(3):e1002513. doi: 10.1371/journal.pmed.1002513
11. Nielen MM, Van Sijl AM, Peters MJ, Verheij RA, Schellevis FG, Nurmohamed MT. Cardiovascular disease prevalence in patients with inflammatory arthritis, diabetes mellitus and osteoarthritis: a cross-sectional study in primary care. BMC Musculoskelet Disord. (2012) 13:150. doi: 10.1186/1471-2474-13-150
12. Gianicolo EA, Eichler M, Muensterer O, Strauch K, Blettner M. Methods for evaluating causality in observational studies. Dtsch Arztebl Int. (2020) 116(7):101–7. doi: 10.3238/arztebl.2020.0101
13. Malik R, Chauhan G, Traylor M, Sargurupremraj M, Okada Y, Mishra A, et al. Multiancestry genome-wide association study of 520,000 subjects identifies 32 loci associated with stroke and stroke subtypes. Nat Genet. (2018) 50(4):524–37. doi: 10.1038/s41588-018-0058-3
14. Burgess S, Small DS, Thompson SG. A review of instrumental variable estimators for Mendelian randomization. Stat Methods Med Res. (2015) 26(5):2333–55. doi: 10.1177/0962280215597579
15. Bowden J, Holmes MV. Meta-analysis and Mendelian randomization: a review. Res Synth Methods. (2019) 10(4):486–96. doi: 10.1002/jrsm.1346
16. Lin L, Luo P, Yang M, Wang J, Hou W, Xu P. Causal relationship between osteoporosis and osteoarthritis: a two-sample Mendelian randomized study. Front Endocrinol. (2022) 13. doi: 10.3389/fendo.2022.1011246
17. Verweij N, Van De Vegte YJ, Van Der Harst P. Genetic study links components of the autonomous nervous system to heart-rate profile during exercise. Nat Commun. (2018) 9(1):898. doi: 10.1038/s41467-018-03395-6
18. Tachmazidou I, Hatzikotoulas K, Southam L, Esparza-Gordillo J, Haberland V, Zheng J, et al. Identification of new therapeutic targets for osteoarthritis through genome-wide analyses of UK biobank data. Nat Genet. (2019) 51(2):230–6. doi: 10.1038/s41588-018-0327-1
19. Nielsen JB, Thorolfsdottir RB, Fritsche LG, Zhou W, Skov MW, Graham SE, et al. Biobank-driven genomic discovery yields new insight into atrial fibrillation biology. Nat Genet. (2018) 50(9):1234–9. doi: 10.1038/s41588-018-0171-3
20. Kurki MI, Karjalainen J, Palta P, Sipilä TP, Kristiansson K, Donner K, et al. FinnGen: unique genetic insights from combining isolated population and national health register data. medRxiv: 2022.2003.2003.22271360 (2022).
21. Mensah-Kane J, Schmidt AF, Hingorani AD, Finan C, Chen Y, Van Duijvenboden S, et al. No clinically relevant effect of heart rate increase and heart rate recovery during exercise on cardiovascular disease: a Mendelian randomization analysis. Front Genet. (2021) 12:569323. doi: 10.3389/fgene.2021.569323
22. Hartwig FP, Davies NM, Hemani G, Davey Smith G. Two-sample Mendelian randomization: avoiding the downsides of a powerful, widely applicable but potentially fallible technique. Int J Epidemiol. (2016) 45(6):1717–26. doi: 10.1093/ije/dyx028
23. Bowden J, Davey Smith G, Haycock PC, Burgess S. Consistent estimation in Mendelian randomization with some invalid instruments using a weighted median estimator. Genet Epidemiol. (2016) 40(4):304–14. doi: 10.1002/gepi.21965
24. Shin J, Andrews M, Dejean L, Debski N, Exarchakis A, Fleming J, et al. Risk factors associated with atrial fibrillation in elderly patients. J Clin Med Res. (2023) 15(3):148–60. doi: 10.14740/jocmr4884
25. Jonsson H, Helgadottir GP, Aspelund T, Eiriksdottir G, Sigurdsson S, Ingvarsson T, et al. Hand osteoarthritis in older women is associated with carotid and coronary atherosclerosis: the AGES Reykjavik study. Ann Rheum Dis. (2009) 68(11):1696–700. doi: 10.1136/ard.2008.096289
26. Schulman S, Aisenberg J. Are NSAIDs double trouble? J Am Coll Cardiol. (2018) 72(3):268–70. doi: 10.1016/j.jacc.2018.04.062
27. Danelich IM, Wright SS, Lose JM, Tefft BJ, Cicci JD, Reed BN. Safety of nonsteroidal antiinflammatory drugs in patients with cardiovascular disease. Pharmacotherapy. (2015) 35(5):520–35. doi: 10.1002/phar.1584
28. Vaiciuleviciute R, Bironaite D, Uzieliene I, Mobasheri A, Bernotiene E. Cardiovascular drugs and osteoarthritis: effects of targeting ion channels. Cells. (2021) 10(10). doi: 10.3390/cells10102572
29. Zhang J, Johnsen SP, Guo Y, Lip GYH. Epidemiology of atrial fibrillation: geographic/ecological risk factors, age, sex, genetics. Card Electrophysiol Clin. (2021) 13(1):1–23. doi: 10.1016/j.ccep.2020.10.010
30. Cooper C, Coggon D. Physical activity and knee osteoarthritis. Lancet. (1999) 353(9171):2177–8. doi: 10.1016/S0140-6736(99)90094-6
31. Fox CS, Parise H, D'agostino RB Sr, Lloyd-Jones DM, Vasan RS, Wang TJ, et al. Parental atrial fibrillation as a risk factor for atrial fibrillation in offspring. JAMA. (2004) 291(23):2851–5. doi: 10.1001/jama.291.23.2851
32. Kornej J, Borschel CS, Benjamin EJ, Schnabel RB. Epidemiology of atrial fibrillation in the 21st century: novel methods and new insights. Circ Res. (2020) 127(1):4–20. doi: 10.1161/CIRCRESAHA.120.316340
33. Adam O, Lohfelm B, Thum T, Gupta SK, Puhl SL, Schafers HJ, et al. Role of miR-21 in the pathogenesis of atrial fibrosis. Basic Res Cardiol. (2012) 107(5):278. doi: 10.1007/s00395-012-0278-0
34. Koplev S, Seldin M, Sukhavasi K, Ermel R, Pang S, Zeng L, et al. A mechanistic framework for cardiometabolic and coronary artery diseases. Nat Cardiovasc Res. (2022) 1(1):85–100. doi: 10.1038/s44161-021-00009-1
35. Macgregor AJ, Antoniades L, Matson M, Andrew T, Spector TD. The genetic contribution to radiographic hip osteoarthritis in women: results of a classic twin study. Arthritis Rheum. (2000) 43(11):2410–6. doi: 10.1002/1529-0131(200011)43:11%3C2410::AID-ANR6%3E3.0.CO;2-E
36. Yao C, Veleva T, Scott L Jr, Cao S, Li L, Chen G, et al. Enhanced cardiomyocyte NLRP3 inflammasome signaling promotes atrial fibrillation. Circulation. (2018) 138(20):2227–42. doi: 10.1161/CIRCULATIONAHA.118.035202
37. Davis J, Burr AR, Davis GF, Birnbaumer L, Molkentin JD. A TRPC6-dependent pathway for myofibroblast transdifferentiation and wound healing in vivo. Dev Cell. (2012) 23(4):705–15. doi: 10.1016/j.devcel.2012.08.017
38. Sagris M, Vardas EP, Theofilis P, Antonopoulos AS, Oikonomou E, Tousoulis D. Atrial fibrillation: pathogenesis, predisposing factors, and genetics. Int J Mol Sci. (2021) 23(1). doi: 10.3390/ijms23010006
39. Xia B, Di C, Zhang J, Hu S, Jin H, Tong P. Osteoarthritis pathogenesis: a review of molecular mechanisms. Calcif Tissue Int. (2014) 95(6):495–505. doi: 10.1007/s00223-014-9917-9
40. Musumeci G, Castrogiovanni P, Trovato FM, Weinberg AM, Al-Wasiyah MK, Alqahtani MH, et al. Biomarkers of chondrocyte apoptosis and autophagy in osteoarthritis. Int J Mol Sci. (2015) 16(9):20560–75. doi: 10.3390/ijms160920560
41. Conen D, Ridker PM, Everett BM, Tedrow UB, Rose L, Cook NR, et al. A multimarker approach to assess the influence of inflammation on the incidence of atrial fibrillation in women. Eur Heart J. (2010) 31(14):1730–6. doi: 10.1093/eurheartj/ehq146
42. Aronson D, Boulos M, Suleiman A, Bidoosi S, Agmon Y, Kapeliovich M, et al. Relation of C-reactive protein and new-onset atrial fibrillation in patients with acute myocardial infarction. Am J Cardiol. (2007) 100(5):753–7. doi: 10.1016/j.amjcard.2007.04.014
43. Guh DP, Zhang W, Bansback N, Amarsi Z, Birmingham CL, Anis AH. The incidence of co-morbidities related to obesity and overweight: a systematic review and meta-analysis. BMC Public Health. (2009) 9:88. doi: 10.1186/1471-2458-9-88
Keywords: osteoarthritis, atrial fibrillation, coronary atherosclerosis, cardiovascular diseases, bidirectional Mendelian randomization, genome-wide association study, European
Citation: Yin M, Xu W, Pang J, Xie S, Xiang M, Shi B, Fan H and Yu G (2023) Causal relationship between osteoarthritis with atrial fibrillation and coronary atherosclerosis: a bidirectional Mendelian randomization study of European ancestry. Front. Cardiovasc. Med. 10:1213672. doi: 10.3389/fcvm.2023.1213672
Received: 3 May 2023; Accepted: 20 July 2023;
Published: 31 July 2023.
Edited by:
Chenyu Sun, AMITA Health, United StatesReviewed by:
Jiali Wang, Shandong University, ChinaLouis Jacob, Parc Sanitari Sant Joan de Déu, Spain
© 2023 Yin, Xu, Pang, Xie, Xiang, Shi, Fan and Yu. This is an open-access article distributed under the terms of the Creative Commons Attribution License (CC BY). The use, distribution or reproduction in other forums is permitted, provided the original author(s) and the copyright owner(s) are credited and that the original publication in this journal is cited, in accordance with accepted academic practice. No use, distribution or reproduction is permitted which does not comply with these terms.
*Correspondence: Hua Fan ZmFuaEBzZGZtdS5lZHUuY24= Gongchang Yu eXVnb25nY2hhbmdAc2RmbXUuZWR1LmNu
†These authors have contributed equally to this work and share first authorship