- 1Institute of Health Informatics, University College London, London, United Kingdom
- 2Institute of Cardiovascular Science, University College London, London, United Kingdom
- 3Liverpool Centre for Cardiovascular Science at University of Liverpool, Liverpool John Moores University and Liverpool Heart & Chest Hospital, Liverpool, United Kingdom
- 4Danish Center for Health Services Research, Department of Clinical Medicine, Aalborg University, Aalborg, Denmark
- 5Barts Heart Centre, Barts Health NHS Trust, London, United Kingdom
Background: Atrial fibrillation (AF) confers a major healthcare burden from hospitalisations and AF-related complications, such as stroke and heart failure. We performed an electronic health records-wide association study to identify the most frequent reasons for healthcare utilization, pre and post new-onset AF.
Methods: Prospective cohort study with the linked electronic health records of 5.6 million patients in the United Kingdom Clinical Practice Research Datalink (1998–2016). A cohort study with AF patients and their age-and sex matched controls was implemented to compare the top 100 reasons of frequent hospitalisation and primary consultation.
Results: Of the 199,433 patients who developed AF, we found the most frequent healthcare interactions to be cardiac, cerebrovascular and peripheral-vascular conditions, both prior to AF diagnosis (41/100 conditions in secondary care, such as cerebral infarction and valve diseases; and 33/100 conditions in primary care), and subsequently (47/100 conditions hospital care and 48 conditions in primary care). There was a high representation of repeated visits for cancer and infection affecting multiple organ systems. We identified 10 novel conditions which have not yet been associated with AF: folic acid deficiency, pancytopenia, idiopathic thrombocytopenic purpura, seborrheic dermatitis, lymphoedema, angioedema, laryngopharyngeal reflux, rib fracture, haemorrhagic gastritis, inflammatory polyneuropathies.
Conclusion: Our nationwide data provide knowledge and better understanding of the clinical needs of AF patients suggesting: (i) groups at higher risk of AF, where screening may be more cost-effective, and (ii) potential complications developing following new-onset AF that can be prevented through implementation of comprehensive integrated care management and more personalised, tailored treatment.
Clinical trial registration: NCT04786366
Background
Atrial fibrillation (AF) is the most frequently sustained cardiac arrhythmia worldwide (1). AF is a clinically heterogeneous condition that can have multiple distinct presentations, ranging from an absence of symptoms to palpitations, and from the development of heart failure or stroke to other cardiovascular and non-cardiovascular complications (2). Identification of disease associations or risk factors can be done following a hypothesis-driven approach based on the understanding of the disease pathophysiology, or through a “hypothesis-free” method, which may be advantageous in cases where there is an incomplete understanding of the pathophysiology, for example, genome-wide association studies (GWAS) to identify novel associations & involved pathways (3).
Similarly to GWAS, electronic health records (EHR) have been previously used for such an approach (4), and could be used for further characterizing the clinical heterogeneity of AF in the UK population through the identification of disease associations. This is important given that AF confers a major healthcare burden from hospitalisations and AF-related complications, such as stroke and heart failure.
We therefore conducted an EHR-wide association study to investigate the most frequent reasons for healthcare interactions pre- and post-AF diagnosis, as compared to individuals without AF, in both primary and secondary care (i.e., primary care consultations and hospitalizations). The findings of the study would provide a better understanding of additional healthcare utilization required in individuals prone to AF and may offer opportunities for screening and potentially preventing or delaying the development of the arrhythmia. Meanwhile, frequent clinical visits following an AF diagnosis help identify the progression of the disease and may be used to define AF clinical sub-phenotypes in routine care, whereas groups of AF patients with specific health service interaction patterns may benefit from tailored holistic or integrated care management approaches.
Methods
The Clinical Practice Research Datalink (CPRD) was established in 1987 (5) and as of 2018 includes 7,998,501 patients in the UK with linked data of primary care consultation, hospital data (Hospital Episodes Statistics, HES), national cancer registry (National Cancer Intelligence Network) and death registry data (Office for National Statistics, ONS) (6, 7). The data are generally representative of the age, gender and geographic distribution of the UK population (5), and showed high quality and completeness of clinical information recorded (6–8). The present study was approved by the Medicines and Healthcare products Regulatory Agency Independent Scientific Advisory Committee [17_205].
Our cohort was composed of individuals aged 18 years or older registered in the current primary care practice for at least one year. The study period was between 1 January 1998 and 31 May 2016, and individuals were excluded if they had a prior history of AF before study entry. AF was defined from the International Classification of Diseases (ICD), tenth revision as I48 from HES and Read codes G573400, G573500, 3,272.00, G573000, G573300, G573.00, G573z00 from CPRD.
We implemented a matched case-control study for investigating the most common problems and comorbidities of AF patients when compared to controls. The primary diagnoses at their general practice (GP) consultation and hospitalisation within five years before and after the diagnosis date in individuals with AF were compared with that of their age and sex-matched controls. For each AF patient, the most frequent GP visits recorded in CPRD and primary diagnosis for hospital admissions documented in HES were identified within five years before and after the initial AF diagnosis. Similarly, we summarised the top conditions for most frequent clinical visits pre and post-index date in matched controls.
We reported the frequencies (%) of the conditions as the most frequent reasons for GP visits and hospital admissions in AF patients and their matched controls. We then summarised the differences between individuals with and without AF by relative frequency (frequency ratio) and reported the leading 100 conditions with descending frequency ratios. The uncertainty of the ratios was estimated with bootstrap distributions based on 2,000 samples with the Balanced Bootstrap Resampling method. We reported the clinical conditions requiring hospitalisation by disease groups (Table 1). We performed the analyses in Statistical Analysis System (version 9.4) and R (version 3.6.1).
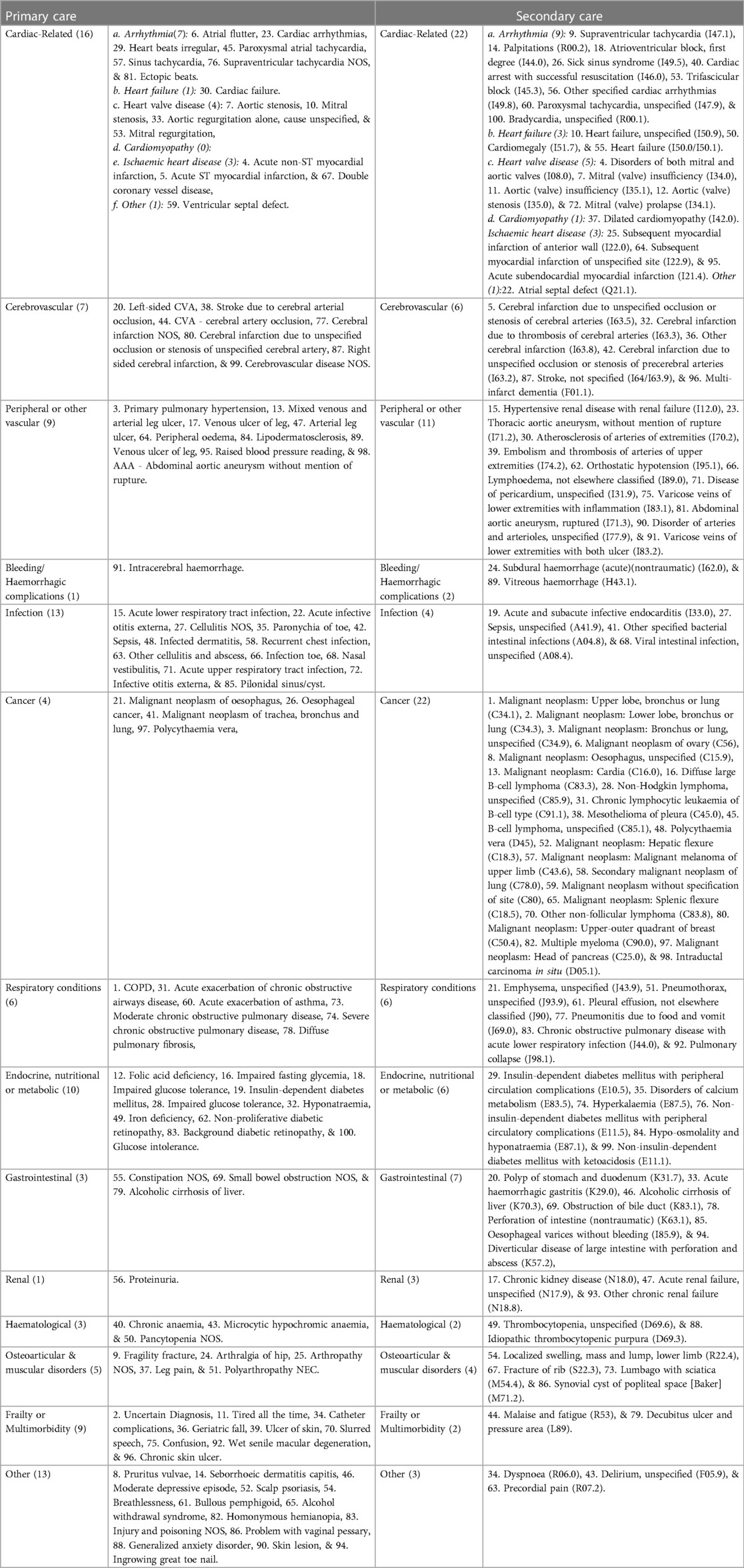
Table 1. Leading 100 frequent causes for hospitalisation or general practise consultation prior to the index date comparing AF and matched controls.
Results
Between 1 January 1998 and 31 May 2016, 7,998,501 participants in CPRD were eligible for linkage. After excluding individuals aged <18 at study entry (n = 1,061,689), not registered with primary care during the study period (n = 407,430), with invalid entry and exit dates into the study (n = 927,579) and with a previous diagnosis of AF (n = 44,238) we obtained a study cohort of 5,557,405 individuals. Over a median of 10.3 years of follow-up (interquartile range: 4.8–15.0) there were 199,433 (3.6%) patients with a diagnosis of new-onset AF, who constituted our cases. These were matched to an equal number of age and sex-matched non-AF controls. The mean age was 75.8 years (SD 12.7) in patients and 75.7 years (SD 12.7) in matched controls.
We observed a predominance of repeated hospitalisation or GP consultation owing to cardiac, cerebrovascular and peripheral vascular conditions prior to AF diagnosis (33 of the top 100 causes in primary care and 41 of the top 100 causes in secondary care) and also subsequently (47 of the top 100 causes in primary care and 48 of the top 100 causes in secondary care). A synthesis of our findings by disease groups is presented in Tables 1, 2.
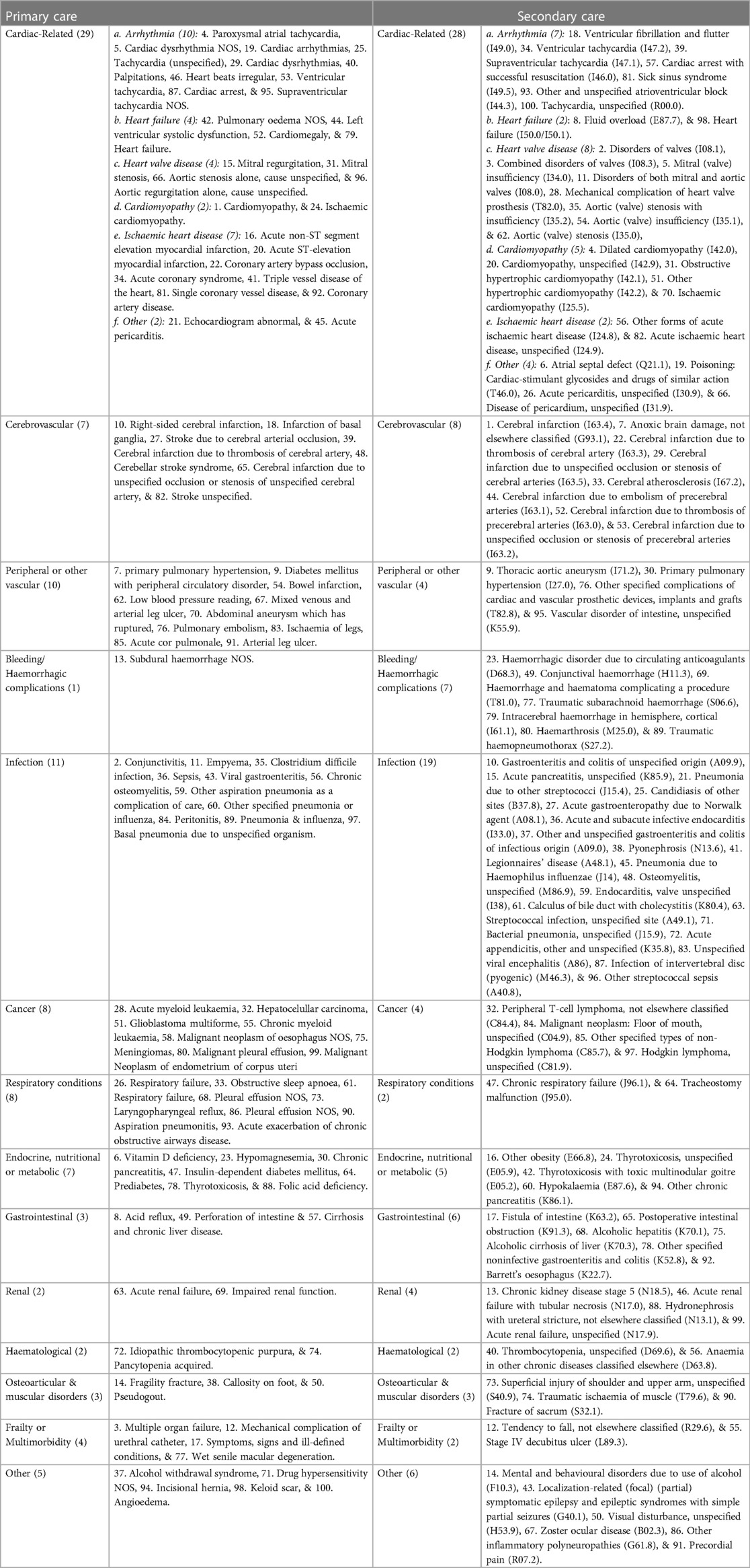
Table 2. Leading 100 frequent causes for hospitalisation or general practise consultation following the index date comparing AF and matched controls.
Compared to matched controls, the leading cardiovascular reasons of excess hospitalisation among individuals with AF prior to the initial AF diagnosis were “disorders of both mitral and aortic valves (I08.0)” (frequency ratio: 10.7), “cerebral infarction due to unspecified occlusion or stenosis of cerebral arteries (I63.5)” (frequency ratio: 10.6) and “mitral (valve) insufficiency (I34.0)” (frequency ratio: 9.2) (Supplementary Table S2). In the period prior to an AF diagnosis, the leading non-cardiovascular causes of more frequent hospitalisation compared AF to controls were “malignant neoplasm: Upper lobe, bronchus or lung (C34.1)”, “malignant neoplasm: Lower lobe, bronchus or lung (C34.3)”, and “malignant neoplasm: Bronchus or lung, unspecified (C34.9)” (frequency ratios: 19.0, 12.7, 12.7, respectively, Supplementary Table S2). Following an AF diagnosis, patients were more frequently hospitalised than controls for cardiovascular conditions such as “Cerebral infarction due to embolism of cerebral arteries (I63.4)”, “disorders of both mitral and tricuspid valves (I08.1)” and “combined disorders of mitral, aortic and tricuspid valves (I08.3)” (frequency ratio: 45.2, 30.6, 21.4, respectively) (Supplementary Table S3).
For frequent GP consultations, compared with matched controls, chronic obstructive pulmonary disease, primary pulmonary hypertension, and acute non-ST myocardial infarction were the leading conditions among AF patients prior to diagnosis. (Frequency ratio: 76.1, 35.1, 28.9, respectively. Supplementary Table S4) Individuals with AF were also more likely to frequently visit GP for conditions that seemed unclear at the time (uncertain diagnosis, frequency ratio: 48). After diagnosis, cardiomyopathy, conjunctivitis and multiple organ failure were the leading reasons for frequent GP consultations among AF patients vs. matched controls. (Supplementary Table S5).
The four iris-plots in Figure 1 represent the top reasons (displayed as relative risk vs. controls, the length of each bar, based on Supplementary Tables S2–S5) for healthcare utilization (primary and secondary care) prior and post new-onset AF. The 14 diagnostic groups are organized by different colours and clockwise. The left upper-panel shows an over-representation of cardiac and cancer-related hospitalizations prior to new-onset AF and the right upper-panel shows over-representation of cardiac and cerebrovascular hospitalizations following new-onset AF. In primary care we observed is over-representation of cardiac-related visits both pre and post new-onset AF. Also, representation of consultations due to infectious disease diagnoses seems to increase following new-onset AF.
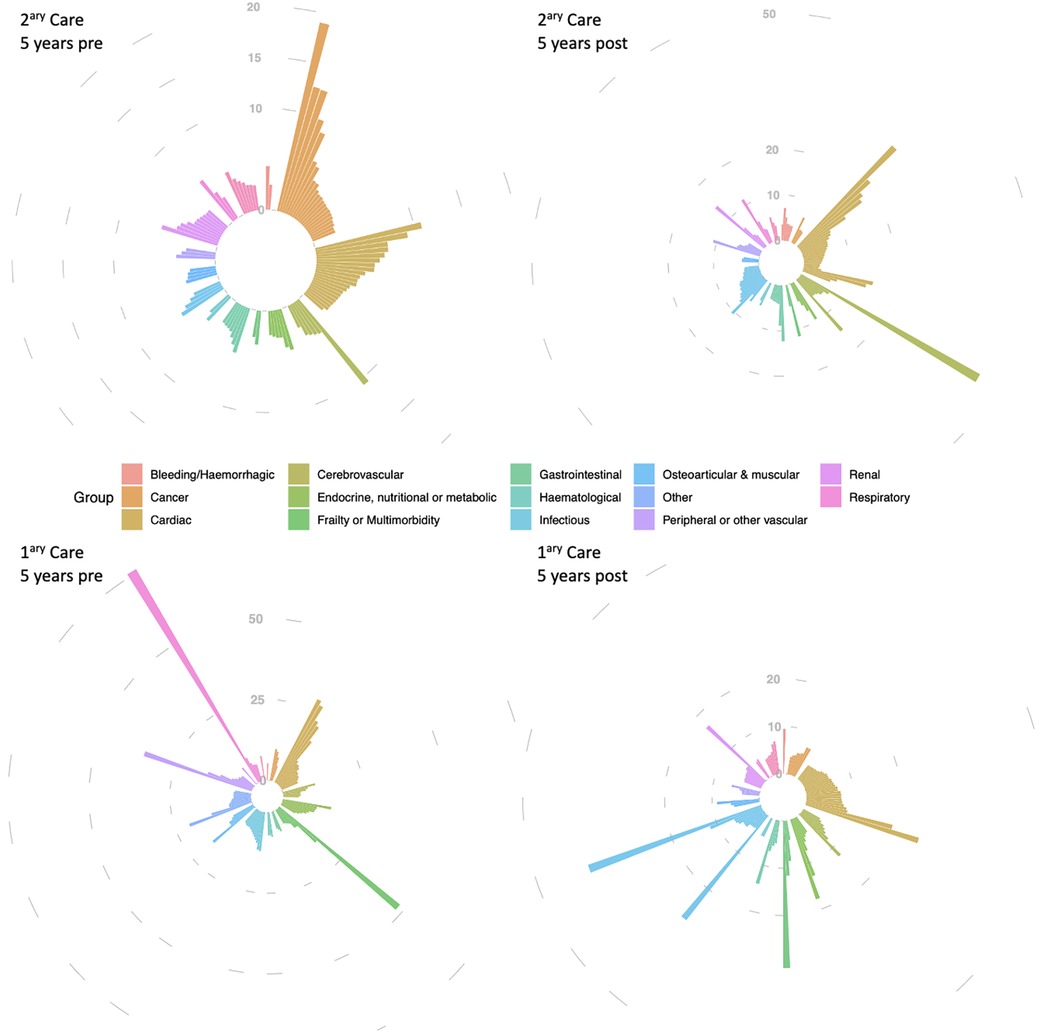
Figure 1. Top 100 reasons for hospitalisation (2ary care) and GP consultations (1ary care) in atrial fibrillation patients, compared to controls, within 5 years before and in the 5 years after new-onset AF diagnosis. The four iris-plots represent the top reasons (displayed as relative risk vs. controls) for healthcare utilization (primary and secondary care) prior and post new-onset AF. The 14 diagnostic groups are organized by different colours (legend) and clockwise as follows, as per the upper-left iris-plot: bleeding/haemorrhagic (12 h), cancer (1 h to 2 h), cardiac (3–4 h), cerebrovascular (5 h), “endocrine, nutritional or metabolic” & “frailty or multimorbidity” (6 h), gastrointestinal (7 h), haematological and infectious (8 h), osteoarticular & other (9 h), “peripheral other vascular” (10 h), renal (11 h), and respiratory (11–12 h). Different scales were used in the 4 iris-plots to accommodate with the different range of relative risks.
Discussion
We report, using a hypothesis-free approach, the most frequent reasons for repeated hospitalisations or primary care consultations by comparing patients with AF and their non-AF-matched peers. We found a predominance of repeated clinical visits due to cardiac, cerebrovascular and peripheral vascular conditions in AF patients vs. matched controls, suggesting the development of arrhythmia may contribute to the aggravation or worsening cardiovascular or cerebrovascular health.
Our findings are in agreement with previous literature providing evidence on important AF complications and comorbidities, such as stroke (9), vascular dementia (10), valvular heart disease (11), myocardial infarction (12), hypertrophic cardiomyopathy (13), and heart failure (14), but also raise hypotheses towards some other unknown or less reported associations with other vascular disorders, such as peripheral artery disease, aortic aneurysms, which may require validation in a different cohort. Some of these associations, like peripheral artery disease (15), aortic aneurysms (16), and ventricular arrhythmias (17) have been suggested in the literature. Also, we have observed other less frequent causes that have been episodically reported (summarised in Supplementary Table S1).
Our data show excess repeated clinical visits affecting multiple organ systems, including for cancer (especially prior to an AF diagnosis) and frequent visits due to infection (mainly after new-onset AF). The association between AF and cancer had previously been reported (18, 19). Similarly, sepsis (20) and other forms of infection (21) have been reported as important complications associated with new-onset AF.
Our findings further reinforce the importance and high prevalence of comorbidities such as obesity (22) and associated conditions like obstructive sleep apnoea (23), and dysglycaemia in the AF population (24). Some of the reasons for repeated clinical visits occurring prior to an AF diagnosis are potentially reversible, with folic acid or vitamin D deficiency, hyponatraemia, hypomagnesemia, hyperkalaemia, iron deficiency, impaired fasting glycemia and glucose tolerance, being a few examples. Other reasons can have their onset delayed with proper intervention, such as type 2 diabetes mellitus (25) and hypertension. Our results suggest the timely detection and treatment or prevention of some of these conditions may potentially prevent or defer the development of AF. For example, the reduction of AF progression with weight loss has been previously demonstrated in the REVERSE-AF study (26).
Some of the reasons for clinical visits we observed may be the consequence of AF-related interventions. Following the diagnosis of AF, we observed an increase in repeated clinical visits for bleeding events, potentially resulting from the increase in the uptake of anticoagulants. Similarly, we also observe visits due to thyrotoxicosis after the diagnosis of AF. The association of hyperthyroidism with AF is well known (27), and with the frequent use of amiodarone in our cohort (28), thyrotoxicosis could partly be due to its concomitant use.
To the best of our knowledge, some of the reasons for frequent clinical visits experienced by AF patients in this study (folic acid deficiency, pancytopenia, idiopathic thrombocytopenic purpura, seborrheic dermatitis, lymphoedema, angioedema, laryngopharyngeal reflux, rib fracture, haemorrhagic gastritis and inflammatory polyneuropathies) have not yet been reported in the literature, and further studies would be recommended.
We reported for the first time the four iris-plots of healthcare utilization for AF patients. Each of these four iris-plots together provides an “iris pattern” similar to the one observed in the human eye. The “iris pattern” is considered unique to each individual, utilized as a biometric measure used for authentication, and known to more accurate than a fingerprint (29).
Practice implications and future research
The current practice and management of AF emphasise more comprehensive management, as highlighted in the ABC (Atrial fibrillation Better Care) pathway (30) that promotes a holistic or integrated care approach that has been associated with improved clinical outcomes (31, 32) leading to its recommendations in guidelines (33, 34). Also, greater focus on comorbidities, such as the recent HEAD-2-TOES (35), going beyond the arrhythmia to associated cardiovascular and non-cardiovascular health conditions. We utilised nationwide data to provide knowledge and a better understanding of how AF patients frequently interact with the health system. Insights from healthcare contacts may facilitate improvements in preventive and treatment strategies for better management and prevention of subsequent outcomes. The shifting patterns of reasons for excess clinical visits in AF patients may contribute to the elucidation of the clinical heterogeneity of AF. Grouping the reasons AF patients seek medical attention by organ system or disease type, we propose the following AF clinical sub-phenotypes: (1) Vascular – associated with atherosclerotic, cerebrovascular and peripheral artery disease; (2) Myopathic – associated with heart failure, cardiomyopathies; (3) Valvular – associated with heart valve involvement; (4) Neoplastic – associated with cancer, potentially as part of inflammation or paraneoplastic syndrome; (5) Infectious – arising as a result of infection and in patients more prone to develop infection; (6) Endocrine or Metabolic – associated with obesity and impaired glucose tolerance or diabetes; (7) Senile – occurring as a result of aging and associated decline and comorbidities. Due to their nature and associated comorbidities, the partial overlap is likely and multiple clinical sub-phenotypes and treatment needs can coexist in the same individual. As an example, one patient may have AF with both Vascular and Metabolic clinical sub-phenotype, whilst a different patient can present with an overlap of Infectious and Senile AF clinical sub-phenotypes. Further studies are required for understanding the risk factors and prognosis of the proposed AF clinical phenotypes, and methods like cluster analyses or equivalent may be applicable. An in-depth understanding of these clinical phenotypes could not only improve the knowledge of the pathways involved and their interplay but optimise AF prevention and treatment.
A better understanding of the conditions and causes for frequent clinical visits preceding the diagnosis of AF may help identify higher-risk subgroups of patients, such as patients with cancer, cardiovascular, cerebrovascular, peripheral vascular disease, respiratory or gastrointestinal disease, for whom a targeted screening strategy may derive more pronounced benefits, including savings in healthcare costs (36). This is important given systematic screening of the general or certain population subgroups has yielded low or negative net benefits (37).
Research implications
An electronic health record (EHR)-wide association study has been previously conducted for better characterizing COVID-19 outcomes (4) but has not been utilized in the field of cardiovascular disease. In this first AF EHR-wide association study, we found the excess clinical visits associated with AF differed by the primary and secondary care and by before and after the diagnosis date. Our experience indicates the importance of considering the type of healthcare and timing of individuals interacting with the care system in applying the EHR-wide association study method.
In this paper we present the “iris pattern” for AF as a disease interacting with primary and secondary care. Even though “iris patterns” have not yet been reported for other diseases, we can hypothesize that as risk factors and their combination differ across different diseases, different “iris patterns” of healthcare utilization, possibly unique to each disease entity, may also exist. This hypothesis requires further clarification, but if proven may help tackle disease specific aspects at primary and secondary prevention level (i.e., prior and post disease onset).
Strength and limitations
The strengths of our research are the power of electronic health records enabling high-resolution analyses for detailed clinical conditions. The generalisability of our findings based on population electronic health records from routine care allows the findings to be applicable to the general clinical practice in comparable populations. The comprehensive scope of conditions that occur in the AF population and their non-AF peers as documented in our analyses raises the possibility and need for a more individualized system contemplating the needs of specific patients or groups of patients (AF clinical sub-phenotypes), some of whom represent clinically complex patients (38). However, there were also some limitations, including the absence of complete information on AF types (paroxysmal, persistent, permanent). However, the clinical utility of such a temporal pattern/episode-based classification remains inconclusive (39). Additionally, even though CPRD patients are thought to be broadly representative of the UK general population in terms of age, sex and ethnicity (40), and a recent study suggesting that CPRD populations may be representative of the general UK populations in terms of socioeconomic status and rural-urban classification (41), we lack the evidence to say if this population is truly representative of the total AF population in the UK. An ongoing study to develop an artificial intelligence model to identify AF patients in the UK may provide an answer to this important area of uncertainty (42). The study will compare two CPRD datasets: CPRD-Gold (which we have utilized to address our research protocol aiming at clarifying the natural history of AF in the UK) and CPRD-Aurum (established in 2017 and comprising 26.9 million additional individuals). Finally, our analyses did not utilize a competing risk framework and have not accommodated for this potential source of bias. Mortality is known to be higher in AF patients when compared to peers (12). Therefore, it is expected that mortality will be a competitive event in relation to the evaluated diagnoses in the post-AF comparisons performed in our analyses. The earlier mortality of AF patients may have precluded the occurrence of the events of interest being assessed, potentially leading to sub-diagnosis.
Conclusion
In this EHR-wide study for AF, we found cardiac, cerebrovascular and other vascular problems are still amongst the most frequent comorbidities in this population, but other disease groups, such as infection and cancer were also frequent. The need for early detection of AF and management of comorbidities may inform targeted early diagnosis and optimal care strategies for AF.
Data availability statement
The data analyzed in this study is subject to the following licenses/restrictions: Data may be obtained from a third party and are not publicly available. Data used in this study were accessed through NHS Digital that is subject to protocol approval and cannot directly be shared. Requests to access these datasets should be directed to Medicines Healthcare products Regulatory Agency - Clinical Practice Research Datalink, https://cprd.com.
Ethics statement
The present study was approved by the Medicines and Healthcare products Regulatory Agency Independent Scientific Advisory Committee [17_205]. The studies were conducted in accordance with the local legislation and institutional requirements. Written informed consent for participation was not required from the participants or the participants’ legal guardians/next of kin in accordance with the national legislation and institutional requirements.
Author contributions
S-CC access, analysis, and interpretation of data for the work and draft the manuscript. RP was Principal Investigator for the project, wrote the application and obtained access and approval for the analyses to the dataset. All authors contributed to the article and approved the submitted version.
Funding
S-CC is supported by the NIHR grants NIHR131227 & NIHR129463. AFS is supported by BHF grant PG/18/5033837, PG/22/10989, and the UCL BHF Research Accelerator AA/18/6/34223. RP is supported by the UCL BHF Research Accelerator AA/18/6/34223 and NIHR grant NIHR129463.
Acknowledgment
This study is based in part on data from the Clinical Practice Research Datalink obtained under licence from the UK Medicines and Healthcare products Regulatory Agency. The data is provided by patients and collected by the NHS as part of their care and support. The interpretation and conclusions contained in this study are those of the author/s alone. The OPCS Copyright © 2020, re-used with the permission of The Health & Social Care Information Centre. All rights reserved. Classification of Interventions and Procedures, codes, terms and text is Crown copyright (2016) published by Health and Social Care Information Centre, also known as NHS Digital and licensed under the Open Government Licence available at www.nationalarchives.gov.uk/doc/open-government-licence/open-government-licence.htm. The interpretation and conclusions contained in this study are those of the author/s alone.
Conflict of interest
The authors declare that the research was conducted in the absence of any commercial or financial relationships that could be construed as a potential conflict of interest.
Publisher's note
All claims expressed in this article are solely those of the authors and do not necessarily represent those of their affiliated organizations, or those of the publisher, the editors and the reviewers. Any product that may be evaluated in this article, or claim that may be made by its manufacturer, is not guaranteed or endorsed by the publisher.
Supplementary material
The Supplementary Material for this article can be found online at: https://www.frontiersin.org/articles/10.3389/fcvm.2023.1204892/full#supplementary-material
References
1. GBD 2017 Disease and Injury Incidence and Prevalence Collaborators. Global, regional, and national incidence, prevalence, and years lived with disability for 354 diseases and injuries for 195 countries and territories, 1990–2017: a systematic analysis for the global burden of disease study 2017. Lancet. (2018) 392(10159):1789–858. doi: 10.1016/S0140-6736(18)32279-7
2. Chung SC, Sofat R, Acosta-Mena D, et al. Atrial fibrillation epidemiology, disparity and healthcare contacts: a population-wide study of 5.6 million individuals. Lancet Reg Health Eur. (2021) 7:100157. doi: 10.1016/j.lanepe.2021.100157
3. Kitsios GD, Zintzaras E. Genome-wide association studies: hypothesis-“free” or “engaged”? Transl Res. (2009) 154(4):161–4. doi: 10.1016/j.trsl.2009.07.001
4. Salvatore M, Gu T, Mack JA, et al. A phenome-wide association study (PheWAS) of COVID-19 outcomes by race using the electronic health records data in Michigan medicine. J Clin Med. (2021) 10(7). doi: 10.3390/jcm10071351
5. Walley T, Mantgani A. The UK general practice research database. Lancet. (1997) 350(9084):1097–9. doi: 10.1016/S0140-6736(97)04248-7
6. Gallagher AM, Dedman D, Padmanabhan S, Leufkens HGM, de Vries F. The accuracy of date of death recording in the clinical practice research datalink GOLD database in England compared with the office for national statistics death registrations. Pharmacoepidemiol Drug Saf. (2019) 28(5):563–9. doi: 10.1002/pds.4747
7. Padmanabhan S, Carty L, Cameron E, Ghosh RE, Williams R, Strongman H. Approach to record linkage of primary care data from clinical practice research datalink to other health-related patient data: overview and implications. Eur J Epidemiol. (2019) 34(1):91–9. doi: 10.1007/s10654-018-0442-4
8. Herrett E, Thomas SL, Schoonen WM, Smeeth L, Hall AJ. Validation and validity of diagnoses in the general practice research database: a systematic review. Br J Clin Pharmacol. (2010) 69(1):4–14. doi: 10.1111/j.1365-2125.2009.03537.x
9. Michaud GF, Stevenson WG. Atrial fibrillation. N Engl J Med. (2021) 384(4):353–61. doi: 10.1056/NEJMcp2023658
10. Bunch TJ, Weiss JP, Crandall BG, May HT, Bair TL, Osborn JS, et al. Atrial fibrillation is independently associated with senile, vascular, and Alzheimer’s dementia. Heart Rhythm. (2010) 7(4):433–7. doi: 10.1016/j.hrthm.2009.12.004
11. Thomas KL, Jackson LR 2nd, Shrader P, Ansell J, Fonarow GC, Gersh B, et al. Prevalence, characteristics, and outcomes of valvular heart disease in patients with atrial fibrillation: insights from the ORBIT-AF (outcomes registry for better informed treatment for atrial fibrillation). J Am Heart Assoc. (2017) 6(12). doi: 10.1161/JAHA.117.006475
12. Odutayo A, Wong CX, Hsiao AJ, Hopewell S, Altman DG, Emdin CA. Atrial fibrillation and risks of cardiovascular disease, renal disease, and death: systematic review and meta-analysis. Br Med J. (2016) 354:i4482. doi: 10.1136/bmj.i4482
13. El-Battrawy I, Borggrefe M, Akin I. Atrial fibrillation in hypertrophic cardiomyopathy. JACC Heart Fail. (2018) 6(9):807. doi: 10.1016/j.jchf.2018.05.013
14. Vermond RA, Geelhoed B, Verweij N, Tieleman RG, Van der Harst P, Hillege HL, et al. Incidence of atrial fibrillation and relationship with cardiovascular events, heart failure, and mortality: a community-based study from The Netherlands. J Am Coll Cardiol. (2015) 66(9):1000–7. doi: 10.1016/j.jacc.2015.06.1314
15. O'Neal WT, Efird JT, Nazarian S, Alonso A, Heckbert SR, Soliman EZ. Peripheral arterial disease and risk of atrial fibrillation and stroke: the multi-ethnic study of atherosclerosis. J Am Heart Assoc. (2014) 3(6):e001270. doi: 10.1161/JAHA.114.001270
16. Hsu CC, Chien WC, Wang JC, Chung CH, Liao WI, Lin WS, et al. Association between atrial fibrillation and aortic aneurysms: a population-based cohort study. J Vasc Res. (2018) 55(5):299–307. doi: 10.1159/000493692
17. Fawzy AM, Bisson A, Bodin A, Herbert J, Lip GYH, Fauchier L. Atrial fibrillation and the risk of ventricular arrhythmias and cardiac arrest: a nationwide population-based study. J Clin Med. (2023) 12(3):70–8. doi: 10.3390/jcm12031075
18. Conen D, Wong JA, Sandhu RK, Cook NR, Lee IM, Buring JE, et al. Risk of malignant cancer among women with new-onset atrial fibrillation. JAMA Cardiol. (2016) 1(4):389–96. doi: 10.1001/jamacardio.2016.0280
19. Yuan M, Zhang Z, Tse G, Feng X, Korantzopoulos P, Letsas KP, et al. Association of cancer and the risk of developing atrial fibrillation: a systematic review and meta-analysis. Cardiol Res Pract. (2019) 2019:8985273. doi: 10.1155/2019/8985273
20. Desai R, Hanna B, Singh S, Omar A, Deshmukh A, Kumar G, et al. Trends and outcomes in sepsis hospitalizations with and without atrial fibrillation: a nationwide inpatient analysis. Crit Care Med. (2019) 47(8):e630–8. doi: 10.1097/CCM.0000000000003806
21. Corica B, Tartaglia F, Oliva A, Raparelli V, Cangemi R, Basili S, et al. Prevalence of new-onset atrial fibrillation in hospitalized patients with community-acquired pneumonia: a systematic review and meta-analysis. Intern Emerg Med. (2023) 18(1):127–35. doi: 10.1007/s11739-022-03135-1
22. Huxley RR, Lopez FL, Folsom AR, Agarwal SK, Loehr LR, Soliman EZ, et al. Absolute and attributable risks of atrial fibrillation in relation to optimal and borderline risk factors: the atherosclerosis risk in communities (ARIC) study. Circulation. (2011) 123(14):1501–8. doi: 10.1161/CIRCULATIONAHA.110.009035
23. Tung P, Levitzky YS, Wang R, Weng J, Quan SF, Gottlieb DJ, et al. Obstructive and central sleep apnea and the risk of incident atrial fibrillation in a community cohort of men and women. J Am Heart Assoc. (2017) 6(7). doi: 10.1161/JAHA.116.004500
24. Yang S, Choi EK, Han KD, Kwon S, Lee SY, Park J, et al. Risk of atrial fibrillation in relation to the time course of type 2 diabetes Mellitus and fasting blood glucose. Am J Cardiol. (2019) 124(12):1881–8. doi: 10.1016/j.amjcard.2019.09.009
25. Irons BK, Mazzolini TA, Greene RS. Delaying the onset of type 2 diabetes mellitus in patients with prediabetes. Pharmacotherapy. (2004) 24(3):362–71. doi: 10.1592/phco.24.4.362.33170
26. Middeldorp ME, Pathak RK, Meredith M, Mehta AB, Elliott AD, Mahajan R, et al. PREVEntion and regReSsive Effect of weight-loss and risk factor modification on Atrial Fibrillation: the REVERSE-AF study. Europace. (2018) 20(12):1929–35. doi: 10.1093/europace/euy117
27. Cho YY, Kim B, Choi D, Kim CH, Shin DW, Kim JS, et al. Graves’ disease, its treatments, and the risk of atrial fibrillation: a Korean population-based study. Front Endocrinol. (2022) 13:1032764. doi: 10.3389/fendo.2022.1032764
28. Chung SC, Lai A, Lip GYH, Lambiase PD, Providencia R. Impact of anti-arrhythmic drugs and catheter ablation on the survival of patients with atrial fibrillation: a population study based on 199 433 new-onset atrial fibrillation patients in the UK. Europace. (2023) 25(2):351–9. doi: 10.1093/europace/euac155
29. Daugman J, Downing C. Epigenetic randomness, complexity and singularity of iris patterns. Proc R Soc Lond. (2001) 268(1477):1737–40. doi: 10.1098/rspb.2001.1696
30. Lip GYH. The ABC pathway: an integrated approach to improve AF management. Nat Rev Cardiol. (2017) 14(11):627–8. doi: 10.1038/nrcardio.2017.153
31. Proietti M, Romiti GF, Olshansky B, Lane DA, Lip GYH. Improved outcomes by integrated care of anticoagulated patients with atrial fibrillation using the simple ABC (atrial fibrillation better care) pathway. Am J Med. (2018) 131(11):1359–66 e6. doi: 10.1016/j.amjmed.2018.06.012
32. Romiti GF, Pastori D, Rivera-Caravaca JM, Ding WY, Gue YX, Menichelli D, et al. Adherence to the “atrial fibrillation better care” pathway in patients with atrial fibrillation: impact on clinical outcomes-A systematic review and meta-analysis of 285,000 patients. Thromb Haemostasis. (2022) 122(3):406–14. doi: 10.1055/a-1515-9630
33. Hindricks G, Potpara T, Dagres N, Arbelo E, Bax JJ, Blomström-Lundqvist C, et al. 2020 ESC guidelines for the diagnosis and management of atrial fibrillation developed in collaboration with the European association for cardio-thoracic surgery (EACTS): the task force for the diagnosis and management of atrial fibrillation of the European society of cardiology (ESC) developed with the special contribution of the European heart rhythm association (EHRA) of the ESC. Eur Heart J. (2021) 42(5):373–498. doi: 10.1093/eurheartj/ehaa612
34. Chao TF, Joung B, Takahashi Y, Lim TW, Choi EK, Chan YH, et al. 2021 focused update consensus guidelines of the Asia pacific heart rhythm society on stroke prevention in atrial fibrillation: executive summary. Thromb Haemostasis. (2022) 122(1):20–47. doi: 10.1055/s-0041-1739411
35. Elliott AD, Middeldorp ME, Van Gelder IC, Albert CM, Sanders P. Epidemiology and modifiable risk factors for atrial fibrillation. Nat Rev Cardiol. (2023) 20(6):404–17. doi: 10.1038/s41569-022-00820-8
36. Burdett P, Lip GYH. Targeted vs. full population screening costs for incident atrial fibrillation and AF-related stroke for a healthy population aged 65 years in the United Kingdom. Eur Heart J Qual Care Clin Outcomes. (2022) 8(8):892–8. doi: 10.1093/ehjqcco/qcac005
37. Svendsen JH, Diederichsen SZ, Højberg S, Krieger DW, Graff C, Kronborg C, et al. Implantable loop recorder detection of atrial fibrillation to prevent stroke (the LOOP study): a randomised controlled trial. Lancet. (2021) 398(10310):1507–16. doi: 10.1016/S0140-6736(21)01698-6
38. Romiti GF, Proietti M, Bonini N, Ding WY, Boriani G, Huisman MV, et al. Clinical complexity domains, anticoagulation, and outcomes in patients with atrial fibrillation: a report from the GLORIA-AF registry phase II and III. Thromb Haemostasis. (2022) 122(12):2030–41. doi: 10.1055/s-0042-1756355
39. Hammond-Haley M, Providencia R, Lambiase PD. Temporal pattern/episode duration-based classification of atrial fibrillation as paroxysmal vs. Persistent: is it time to develop a more integrated prognostic score to optimize management? Europace. (2018) 20(FI_3):f288–98. doi: 10.1093/europace/eux178
40. Herrett E, Gallagher AM, Bhaskaran K, Forbes H, Mathur R, van Staa T, et al. Data resource profile: clinical practice research datalink (CPRD). Int J Epidemiol. (2015) 44(3):827–36. doi: 10.1093/ije/dyv098
41. Mahadevan P, Harley M, Fordyce S, Hodgson S, Ghosh R, Myles P, et al. Completeness and representativeness of small area socioeconomic data linked with the UK clinical practice research datalink (CPRD). J Epidemiol Community Health. (2022) 76(10):880–6. doi: 10.1136/jech-2022-219200
42. Nadarajah R, Wu J, Frangi AF, Hogg D, Cowan C, Gale C. Predicting patient-level new-onset atrial fibrillation from population-based nationwide electronic health records: protocol of FIND-AF for developing a precision medicine prediction model using artificial intelligence. BMJ Open. (2021) 11(11):e052887. doi: 10.1136/bmjopen-2021-052887
Keywords: arrhythmia, electronic health records, clinical visits, population study, hospitalization, primary care
Citation: Chung S-C, Schmit AF, Lip GYH and Providencia R (2023) Electronic health record-wide association study for atrial fibrillation in a British cohort. Front. Cardiovasc. Med. 10:1204892. doi: 10.3389/fcvm.2023.1204892
Received: 12 April 2023; Accepted: 12 September 2023;
Published: 28 September 2023.
Edited by:
Pietro Scicchitano, Azienda Sanitaria Localedella provincia di Bari (ASL BA), ItalyReviewed by:
Galaleldin Nagib Elkilany, Gulf Medical University, United Arab EmiratesJorge Rodríguez Capitán, Carlos III Health Institute (ISCIII), Spain
Copyright: © 2023 Chung, Schmidt, Lip and Providencia. This is an open-access article distributed under the terms of the Creative Commons Attribution License (CC BY). The use, distribution or reproduction in other forums is permitted, provided the original author(s) and the copyright owner(s) are credited and that the original publication in this journal is cited, in accordance with accepted academic practice. No use, distribution or reproduction is permitted which does not comply with these terms.
*Correspondence: Rui Providencia, ci5wcm92aWRlbmNpYUB1Y2wuYWMudWs=