- 1Department of Pharmacology, School of Basic Medical Sciences, Zhengzhou University, Zhengzhou, China
- 2Dow Institute for Advanced Biological and Animal Research, Dow University of Health Sciences, Karachi, Pakistan
- 3King Faisal Medical Complex (KFMC), Taif, Saudi Arabia
- 4Jamil-ur-Rahman Center for Genome Research, Dr. Panjwani Center for Molecular Medicine and Drug Research, International Center for Chemical and Biological Sciences, University of Karachi, Karachi, Pakistan
Heart Failure (HF) is a complex clinical syndrome in which the heart is unable to provide enough blood flow to meet metabolic needs and lacks efficient venous return. HF is a major risk factor for morbidity and mortality with cardiovascular diseases globally. Despite enormous research, the molecular markers relevant to disease prognosis and management remain not well understood. Here, we analyzed the whole transcriptomes of 18 failing hearts and 15 non-failing hearts (predominantly of Caucasian origin), by applying the standard in silico tools. The analyses revealed novel gene-markers including ALKBH5 of mRNA demethylation and KMT2E of histone modification processes, significantly over-expressed in the HF compared with the non-failing hearts (FDR < 0.05). To validate the over-expression of ALKBH5, we determined the global m6A level in hypoxic H9c2 cells using a dot blot assay. The global m6A level was found markedly lower in the hypoxic H9c2 cells than in the control cells. Additionally, the expression of ALKBH5 in the H9c2 cells was quantified by the qPCR and found to be 1.18 times higher at 12 h (p < 0.05), and 1.67 times higher at 24 h of hypoxia (p < 0.01) compared with the control cells, indicating a likely role of ALKBH5 in the failing cardiac cells. Furthermore, we identified several compounds through the virtual screening of 11,272 drug-like molecules of the ZINC15 database to inhibit the ALKBH5 in a molecular docking process. Collectively, the study revealed novel markers potentially involved in the pathophysiology of HF and suggested plausible therapeutic molecules for the management of the disease.
1. Introduction
Heart failure (HF) is leading in terms of prevalence and hospitalization worldwide. Cardiovascular diseases (CVDs) are associated with significantly higher morbidity, mortality, and healthcare expenditures, particularly among older adults (1). Despite improvements in the healthcare system and advancements in modern medicine, the prognosis of HF has not improved over the last decades, suggesting that several aspects of cardiac pathophysiology remain unresolved (2). Therefore, comprehension of the underlying mechanisms in HF can play a major role in disease prevention and management.
Epidemiological findings suggest that alcohol, obesity, smoking, hypertension, and coronary artery disease (CAD) are the risk factors for HF (3, 4). At molecular level, the recent research suggests that ventricular remodeling, compensatory mechanisms, inflammatory signaling, fibrosis, oxidative stress, mitochondrial dysfunction, and epigenetic modifications, including RNA transcript modifications, are significantly associated with HF progression and predict HF outcomes (5). Ventricular remodeling, which is a modification of the structure and function of the heart after injury to the myocardium, involves excitation-contraction coupling, Ca2+ handling, changes in energetic metabolism, contractile proteins or their regulatory elements, and cytoskeleton components (6). Compensatory mechanisms, such as activation of the adrenergic nervous system (Beta-Blocker Evaluation of Survival Trial Investigators, 2001) and renin-angiotensin-aldosterone system (RAAS) (7), also contribute to the onset of HF. Cardiac fibrosis is a determinant of HF outcome and is promoted by irregular deposition of extracellular matrix (ECM) proteins in the cardiac interstitium (8). Cardiac fibrosis leads to diastolic and systolic dysfunction of the left ventricle (9). Cardiac inflammation occurs primarily in response to cardiac injury such as ischemia. In short-term cardiac injury, activation of nuclear factor kappa B (NF-κB) induces a cardio-protective role (10). However, long-term heart damage activates the release of several proinflammatory cytokines and chemokines leading to HF (11). The activation of cell surface death receptors, such as Fas/FasL, and consequent programmed cell death are also found in failing hearts (12). Oxidative stress is also associated with ventricular dysfunction, with increased production of reactive oxygen species (ROS) stimulated by aldosterone, which is produced by the RAAS, observed in HF (13).
In addition to the afore-mentioned etiologies of HF, several studies have shown in the recent past that dysregulation of the epitranscriptomic modifiers such as mRNA N6-methyladenosine (m6A) writers (METTL3, and METTL14), readers (YTHDF1, YTHDF2, and YTHDF3), and erasers (FTO, ALKBH5), which affect the stability, splicing, translation efficiency, and degradation of the mRNA (14–18), have plausible roles in the pathophysiology of HF (19). These findings also suggest the potential use of these genes as new biomarkers in the HF. In this context, two m6A demethylases, fat mass and obesity–associated protein (FTO) and AlkB homolog 5 (ALKBH5) have been given particular emphasis in the translational cardiovascular research. The expression of FTO regulates the cardiac function and has been implicated in HF (20). However, the role of ALKBH5 demethylase has been less studied in HF. Previously, ALKBH5 inhibited apoptosis and promoted autophagy in nucleus pulposus cells by demethylating the FIP200 mRNA (21). So, there is a need to explore the involvement of ALKBH5 in HF patients.
The advent of robust approaches, such as whole transcriptome analysis, has enabled us to explore deeply and discover new therapeutic targets involved in the pathophysiology of HF. In this study, we aimed to analyze the whole transcriptomes of failing hearts of different age groups, in comparison with non-failing hearts to identify distinct gene expression profiles and their roles in the disease. Our findings revealed some novel gene markers of epitranscriptomic modification, and thus, suggested potential pharmacological targets in HF. Additionally, we performed molecular docking of over 11,000 compounds from the ZINC15 database to explore potential molecules for the development of new drugs.
2. Methodology
2.1. Data collection
The RNA-seq data of human left ventricles and related metadata of 18 HF patients and 15 healthy individuals were retrieved from the Gene Expression Omnibus (GEO, https://www.ncbi.nlm.nih.gov/geo). The study cohort related to the gene expression omnibus studies GSE57344, GSE147236, and GSE161472. The metadata was normalized to get uniform information about the samples. The RNA-seq data was fetched with the SRA Toolkit using the “SRR” IDs of the sample and then converted into the fastq format using the fastq-dump tool of the SRA Toolkit (22).
2.2. Bioinformatics analysis
The fastq files were assessed for the Phred quality scores using the FastQC tool v0.11.9 (23). The low-quality reads were filtered out using the Trimmomatic tool v0.40 (24). For the alignment of short sequence reads with reference, the STAR 2.7.10a pipeline (25) of The Cancer Genome Atlas (TCGA) was employed. The sequencing reads were aligned to the hg38 assembly of the human reference genome (ftp://hgdownload.soe.ucsc.edu/goldenPath). In the STAR analysis, first, the reference genome was indexed using the “genomeGenerate” module, followed by Alignment First Pass, Intermediate Index Generation, and Alignment Second Pass. The number of aligned reads in each gene was counted in the resultant BAM file using the featureCounts tool. For this, the reference genome-compatible genes’ coordinates (gtf) were obtained from the GENCODE database (https://www.gencodegenes.org/).
2.3. Comparison of genes expression changes
For determining the global gene expression profiling and differential gene expression, the raw read count data were transformed into fragments per kilo per million (FPKM) values using the R package (v.4.1.2) by applying the formula:
where, for the FPKM of the ith feature (gene), qi is the raw read count of the gene, li is the length of the gene, and ∑jqj corresponds to the total number of mapped fragments. The BioConductor package EdgeR was employed to perform the downstream analysis for determining the differential genes expression. First, the data was normalized by applying a weighted trimmed mean of M values (TMM) method. Then Fisher's exact test was applied to conduct a pairwise comparison of genes expression between the normal and HF samples for each gene. The edgeR's function “topTags” which employs Benjamini-Hochberg method was used to apply multiple corrections and to minimize the false discovery rate (FDR), also termed as the adjusted p-values. The FDR < 0.05 was considered significant for designating a gene as differentially expressed. Further, the unsupervised hierarchal clustering was performed to find out the distinct groups based on the expression profiles. To further extrapolate and to find the statistical significance among the groups identified in the hierarchal clustering, the Kruskal-Wallis test was performed. The expression profiles were further evaluated by performing principal components analysis (PCA). The unsupervised hierarchal clustering, Kruska-Wallis test, PCA, and the data visualizations were carried out by using the R-4.0.3 statistical package.
2.4. Gene annotation and gene/protein regulatory network
To determine the biological processes, gene set enrichment analysis and functional protein association networks related to the prioritized genes, the online STRING database (v.11.5) was used (https://string-db.org/). The protein-protein interactions (PPIs) were determined within the over-expressed and under-expressed genes. The protein-protein interactions with PPI score ≥0.4 were considered. The whole bioinformatics pipeline has been submitted to the GitHub repository https://github.com/Shakeel211/RNA-seq.
2.5. H9c2 cell culture and hypoxia model
Given the recent focus of biomedical research on epitranscriptomics in HF (17, 26), we selected one gene ALKBH5 (an RNA demethylase and epitranscriptomic marker) for validation in the cardiac cell line. We constructed the hypoxia model using the H9c2 (rat embryonic ventricle) cell line, as described in our previous study (27). The H9c2 cell line was purchased from the Type Culture Collection of the Chinese Academy of Sciences (Shanghai, China). An anaerobic workstation (AW400SG/TG; Electrotek) was used to prepare the cell hypoxia model. H9c2 cells were placed in the transfer chamber, exposed to high-purity N2, and then transferred into the anaerobic working chamber where mixed gas consisting of 10% H2, 10% CO2, and 80% N2 was introduced. Dulbecco's modified Eagle medium (DMEM, Corning Inc., Corning, NY, USA) supplemented with 10% fetal bovine serum (FBS) and 1% penicillin/streptomycin) was used for cell culture. When the cells reached 80%–90% confluency, the medium was replaced with serum-free DMEM medium, and cells were cultured for 12, and 24 h under hypoxia conditions, respectively.
2.6. m6A dot blot assay
To quantify m6A level in the hypoxia-induced and normal H9c2 cells, 500 ng RNAs were denatured by heating at 65°C for 5 min and spotted onto a nylon membrane (Sigma, USA) with a Bio-Dot apparatus (Bio-Rad, USA). The membrane was ultraviolet (UV) crosslinked, blocked, incubated with m6A antibody overnight at 4°C, and then incubated with HRP-conjugated anti-mouse IgG. After extensive washing, the membrane was visualized by the chemiluminescence system (Bio-Rad, USA). After visualization, the membrane was stained with 0.02% methylene blue (MB) in 0.3 M sodium acetate buffer (pH 5.2) to ensure consistency in the RNA amount of the samples on the membrane.
2.7. RNA extraction and quantitative PCR (qPCR) analysis, and western blotting
For performing the quantitative real-time PCR (qPCR) of ALKBH5, total RNA was isolated by using the TRIzol reagent (Roche, Germany), and reverse transcribed by using the PrimeScript RT Reagent Kit (Cat#RR047A, Takara Bio, China) following the recommended protocol of the manufacturer. The resultant cDNA was subject to qPCR by using the SYBR Green Master mix (Thermo Fisher Scientific, United States) on the 7,500 Fast Real-Time PCR system (Applied Biosystems; Thermo Fisher Scientific, United States). In the qPCR, the following thermocycling conditions were used: holding at 50°C for 2 min; pre-denaturation at 95°C for 2 min, followed by 40 cycles of 15 s at 95°C and 1 min at 60°C. For the melt curve, 95°C for 15 s, 60°C for 1 min, 95°C for 15 s, and 60°C for 15 s. were applied. The ALKBH5 mRNA levels were normalized to GAPDH levels. Following primers were used in the qPCR reaction: ALKBH5 Forward: 5′- CCCGAGGGCTTCGTCAACA-3′; Reverse: 5′-CGACACCCGAATAGGCTTGA-3′; GAPDH Forward: 5′-TCCCTCAAGATTGTCAGCAA-3′; Reverse: 5′-AGATCCACAACGGATACATT-3′. The relative expression was calculated using the 2−ΔΔCT method. For determining the statistical significance, one-way ANOVA was used. For the western blot analysis, total protein from H9c2 cells was collected using RIPA lysis buffer (#R0030, Solarbio,Beijing, China) plus protease inhibitor cocktail (#HY-K0010, MedChemExpress, New Jersey, USA). The membranes were incubated with primary antibodies against ALKBH5 (1:2000; #16837-1-AP, Proteintech, Wuhan, China), and GAPDH (1:10000; #60004-1-Ig, Proteintech) at 4°C overnight, followed by alkaline phosphatase-conjugated affinipure goat anti-rabbit IgG (H+L) (1:10000; Proteintech) for 2 h at 37°C. The blots were analyzed and quantified using ImageJ analysis software.
2.8. Virtual screening of drug-like compounds through the molecular docking
A virtual screening of 11,272 drug-like compounds from the ZINC15 database (www.zinc15.docking.org) was performed against the druggable active sites (putative targets) of the ALKBH5 protein (PDB ID 4NJ4) using the Molecular Operating Environment (MOE v2016.11) software (28). Prior to the docking, the druggable target sites (pockets) in the ALKBH5 were identified through the DoGSiteScorer server (https://proteins.plus). The MOE predicts accurately the binding affinities of the ligands with the protein through a predefined algorithm by scoring different docking orientations between the receptor and ligand in a ligand receptor interaction and records affinities of the best fit poses of the ligands. The docking was performed according to the protocol as described by the authors (28). The parameters used were as follows: Placement = Triangle matcher, Refinement = Induced fit, Scoring 1 = London dG kcal/mol, Scoring 2 = GBVI/WSA dG kcal/mol, Poses = 30, and Retain Poses = 5.
2.9. ADMET profiling of the lead compounds
The top 10 hits which passed the Lipinski's drug-like test and had minimum values of binding energies were selected as suitable inhibitors for performing the pharmacokinetics analysis including the absorption, distribution, metabolism, excretion and toxicity (ADMET) analysis. The ADMET analysis and other physicochemical parameters such as skin permeation were determined using the online ADMET prediction server (http://lmmd.ecust.edu.cn/admetsar2) and Swiss ADME (http://www.swissadme.ch/), respectively to validate the parameters for the suitable drug/binding candidates. Before performing the docking analysis, the structure of each ligand was optimized by calculating charges, applying force field (MMFF94x) and energy minimization.
2.10. Statistical analysis
The data are presented as means ± standard deviation (SD). Statistical significance was assessed using the unpaired student's t-test for experiments. p-value < 0.05 were considered statistically significant (*p < 0.05, **p < 0.01).
3. Results
3.1. Cohort characteristics
Characteristics of the study participants including the non-heart failing (NF) controls and heart failure (HF) patients are listed in Table 1. The study cohort predominantly comprised Caucasians (73%), whereas 15% of the participants were African-American, and 12% were Hispanic. The male:female ratio in the non-heart failing samples was 1.5, and in the heart failing sample was 1.57. The age of the non-heart failing individuals was 1–77 years (average 46.6 years, median 50 years), and of the HF patients was 16–68 years (average 39.38 years, median 38.5 years). The patients presented with HF due to cardiomyopathies.
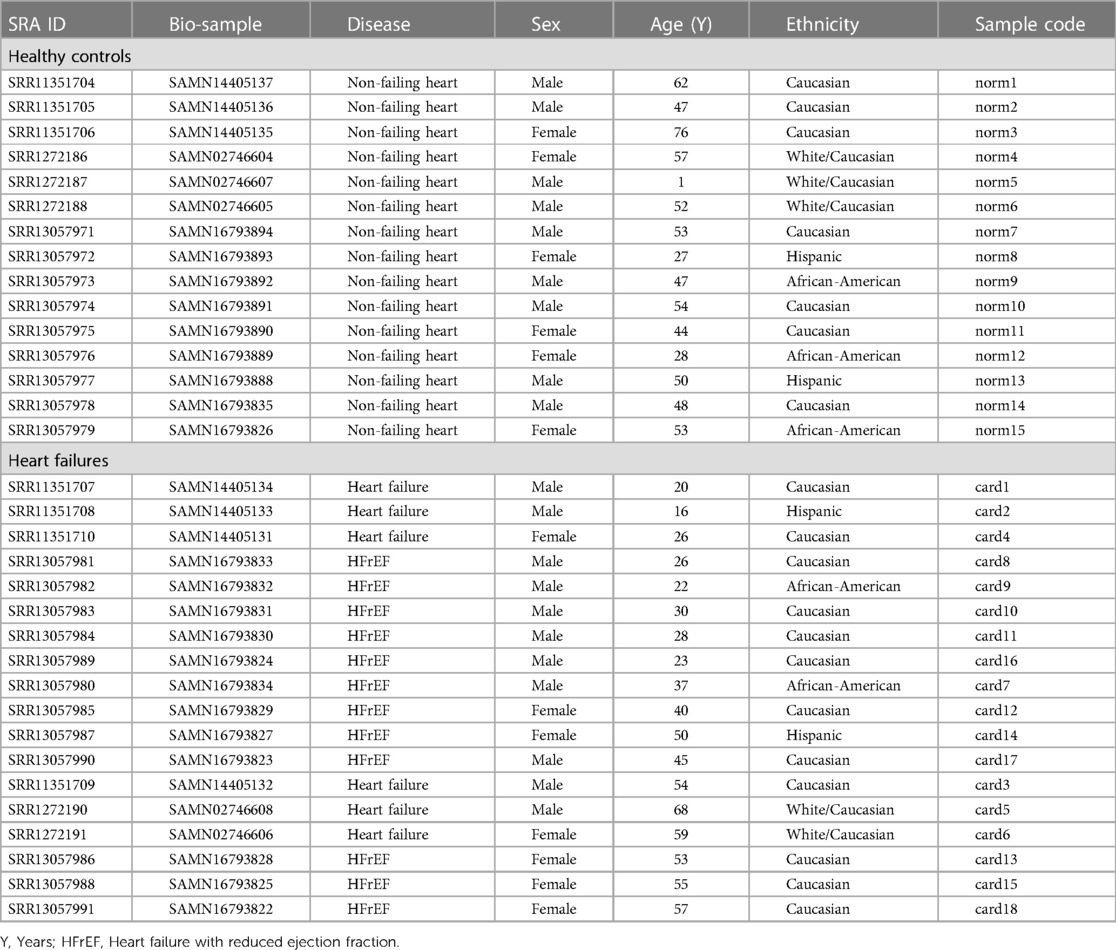
Table 1. Characteristics of the study cohort. The non-failing healthy hearts were included as controls, whereas, the failing hearts were included as diseases samples.
3.2. Transcriptome profiles of heart failure indicate the involvement of different signaling pathways
The transcriptomic profiling and differential gene expression was carried out schematically using the standard RNA-seq data analysis pipeline. The average FPKM was 9.5, whereas the median FPKM was found to be 4.1 (Supplementary Figure S1). The distribution of the FPKM values indicated a uniform trend, however, there were observable differences in the distribution in some bins (Supplementary Figure S2), indicating the likely differential expression of genes in these bins. For subsequent analysis, we selected the genes for which at least 50% of the samples had FPKM values of ≥0.5. This filtration resulted in 7,407 genes. Prior to the differential gene expression, a holistic view of the FPKM values was assessed, which indicated a mixed pattern of normal and HF samples (Supplementary Figure S3).
To determine the over-expressed and under-expressed genes in each sample, genes expression profiles were constructed. Taking FDR < 0.05 into account, there were 1,068 over-expressed genes (FDR < 0.05), and 924 under-expressed genes in the HF samples compared with the non-failing hearts (Supplementary Table S1). These included 498 over-expressed genes with a fold change (FC) ≥1.3 times higher in failing heart, and 413 under-expressed genes with FC ≤ 1.3 times less in failing heart.
The gene set enrichment analysis of the over-expressed genes using the online STRING database (29) indicated multiple processes involved in the pathophysiology of HF (FDR < 0.05) (Supplementary Table S2A). These included 18 GO terms related to chemotaxis and immune cell migration, 14 terms related to regulation of cytoskeleton, 14 Gene Ontology (GO) terms related to protein folding, 5 terms related to various blood cell degranulation, and 3 terms related to platelet activation and migration. Among the Reactome pathways, the majority of the genes including ANXA1, ANXA2, C3AR1, CCL2, CCL11, CD63, CXCL1, FSCN1, ITGB1, ITGB2, MAP2K3, PPIA, PPP2CB, RAP1B, TMEM30A, and TNFAIP3 were related with signaling by the interleukins and immune cells activation (Supplementary Table S3). Few genes including ACTG1, ARRB2, FN1, NRAS, and RAP1B were found related to JAK-STAT, MAPK, RAF1, BRAF, EPHB, and RAS signaling pathways. Notably, several genes were found associated with the platelets activation, signaling, degranulation, and aggregation, and included ACTN1, ANXA5, AVPR1A, CALU, CAP1, CD63, CDC37L1, DGKD, F2R, FAM3C, FCER1G, FN1, GNG12, HSPA5, MANF, PDPN, PFN1, PPIA, PTPN1, RAP1B, SERPINE1, THBS1, TUBA4A, and YWHAZ. Among these, five genes CAP1, DGKD, F2R, FAM3C, and GNG12 have been prioritized for the first time in HF (Supplementary Figure S4). We further investigated the expression level of the epigenetic gene involved in m6A and histone methylation and demethylation. A significantly (FDR < 0.05) increased expression of ALKBH5, VIRMA, KMT2E, and HDAC2, whereas, reduced expression and KDM4B, H2AW, H2BC8, H3C1, MTHFR, and CMBL were found in the HF patients compared to the control group (Figure 1). Several protein-protein interactions (PPIs) among the over-expressed genes were also observed. These included 10 PPIs with very high confidence (PPI score ≥0.9), 11 PPIs with high confidence (PPI score 0.7–0.9), and 35 PPIs with moderate confidence (PPI score 0.4–0.7) (Supplementary Table S2B).
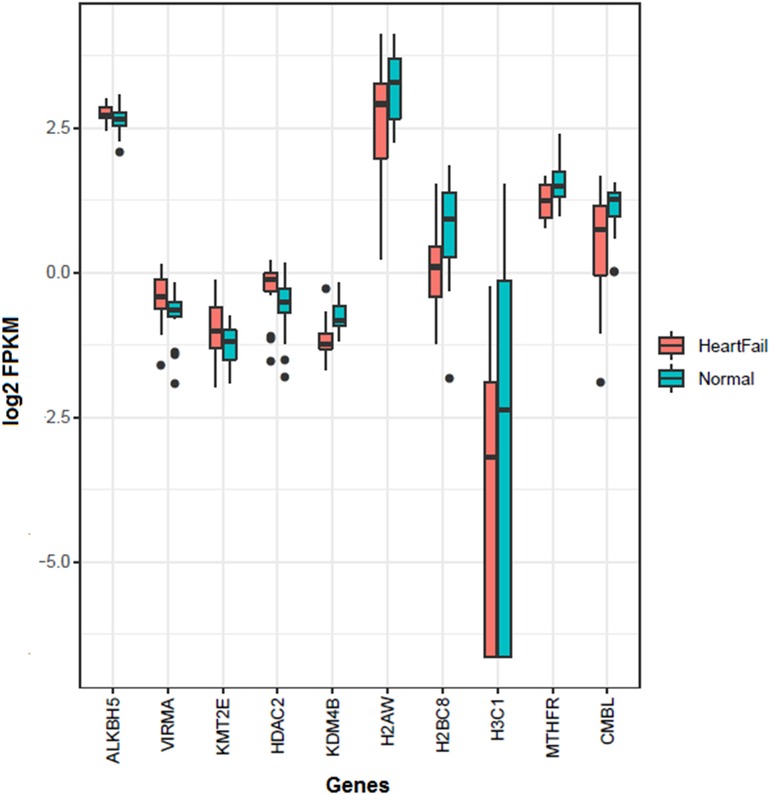
Figure 1. Significantly differentially expressed genes of m6A and histones methylation and demethylation in the heart failure compared with the normal hearts. Four genes (ALKBH5, VIRMA, KMT2E, and HDAC2) were over-expressed, whereas six genes (KDM4B, H2AW, H2BC8, H3C1, MTHFR, and CMBL) were down-expressed in the heart failures.
In addition, we compared the genes expression between the females and males heart failures to observe sex-wise distinct genes expression. This analysis highlighted 8 genes having lower expression in males compared with that in females. These genes included FN1, PTPN1, ITGA3, F2R, MYH9, ITGB1, ACTG1, and ACTN1 (Supplementary Figure S5). The annotation and protein-protein interaction using the online STRING database showed that these genes were related with KEGG pathways Regulation of actin cytoskeleton (hsa04810), Focal adhesion (hsa04510), and PI3K-Akt signaling pathway (hsa04151).
3.3. ALKBH5 expression, and global m6A methylation levels in the H9c2 cells
Out of the novel genes differentially expressed in the failing hearts, we selected ALKBH5 to validate its expression in the H9c2 cells under hypoxia. The ALKBH5 encodes an mRNA N6-methyladenosine dioxygenase which demethylates N(6)-methyladenosine (m6A) on RNAs. Given the RNA m6A demethylation role of ALKBH5, we determined the global m6A RNA methylation level in the H9c2 hypoxic and control cells using a dot blot assay (Figure 2A). In this analysis, time-dependent significant increase in ALKBH5 expression was observed in the H9c2 cells under hypoxia compared with normal H9c2 cells (p < 0.05), where prolonged hypoxia caused higher expression (Figure 2B). To further validate, we evaluated the ALKBH5 protein level in H9c2 cells using the western blotting method. The expression of ALKBH5 protein was significantly higher in the H9c2 cells under 24 h of hypoxia (Figures 2C,D). These results showed that the decrease of m6A abundance may be caused by the increase of ALKBH5 expression in hypoxic H9c2 cells.
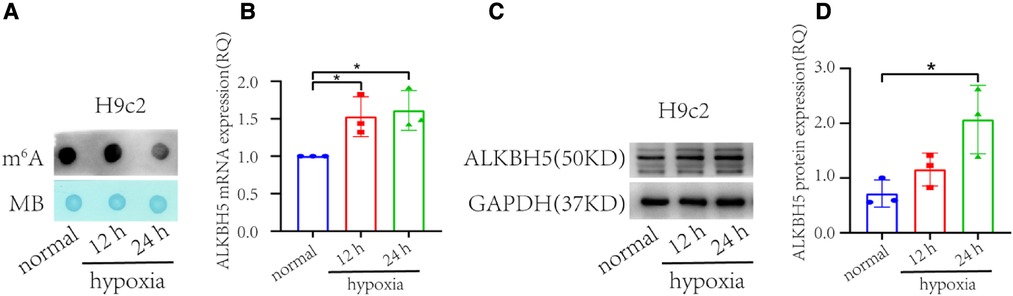
Figure 2. m6A abundance and ALKBH5 expression in H9c2 cells under hypoxia and normal (non-hypoxic) conditions (n = 3 each). (A) Dot blot assay representing time dependent markedly lower global m6A in the H9c2 cells under hypoxia than in normal (non-hypoxic) condition. (B) ALKBH5 gene expression in the H9c2 cell line under hypoxia, and normal conditions. (C) Western blot of ALKBH5 and GAPDH proteins in H9c2 cells. (D) The ALKBH5 protein expression in H9c2 cells inferred from the western blot using ImageJ. normal = H9c2 cells kept in non-hypoxic conditions; 12 h = H9c2 cells kept in hypoxic conditions for 12 h, 24 h = H9c2 cells kept under hypoxic conditions for 24 h. *p < 0.05, v.s. normal.
3.4. Distinct expression profiles in age-dependent heart failure
An unsupervised hierarchal clustering using the R package, revealed three distinct groups, one non-failing heart, and two HFs (Figure 3A). These indicated distinct expression profiles within the HF patients, indicating likely diverse mechanisms being involved in the HF. To further extrapolate this, a Kruskal-Wallis test was performed, which indicated diversity in the expression landscape among all HF samples (p < 0.001, df = 17). Furthermore, by performing principal components analysis (PCA) of the HF of different ages, three groups were identified (Figure 3B). The three HF groups observed in the PCA also exhibited significantly different expression profiles in the Kruskal-Wallis test (p < 0.01, df = 2). To further extrapolate, we identified 62 genes with ≥1.3 times higher expression and 191 genes having ≤1.3 times lower expression in group 1 patients with respect to group 2 and 3 patients (Supplementary Table S4). Group 1 comprised 6 patients (4 Caucasians, 1 African-American, and 1 Hispanic; 5 males and 1 female; an average age of 23.33 years), and we designated this group as the young-aged group. Likewise, in group 2, there were 4 patients (2 Caucasians, 1 African-American, and 1 Hispanic; 2 males and 2 females; with an average age of 43 years). The group was designated as the late-aged adult group. There were 57 differentially over-expressed genes and 9 genes that were less expressed in the Group 2 patients compared with the Group1 and Group3 patients (see Supplementary Table S5). The third group in the hierarchical clustering (Group 3) comprised 6 patients, all Caucasians (2 males, and 4 females), with an average age of 57.66 years, and was designated as the early-aged elder group. There were 215 differentially over-expressed genes and 193 under-expressed genes (see Supplementary Table S6).
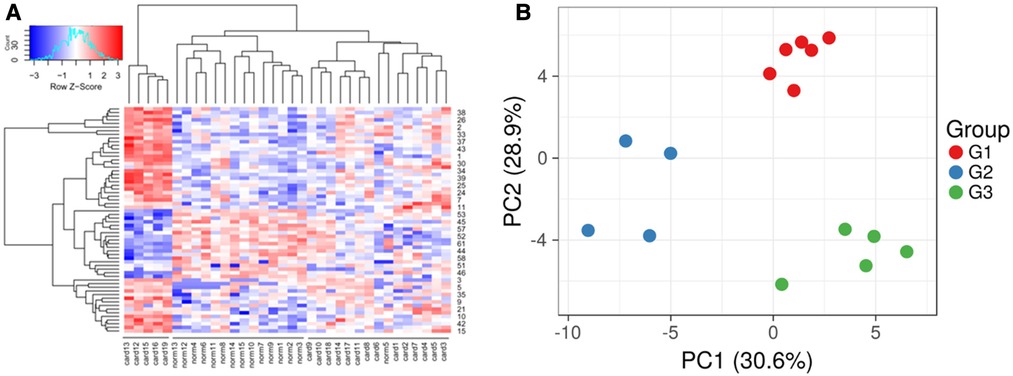
Figure 3. Expression profiles using unsupervised clustering. (A) The dendrogram shows three groups, including two groups of heart failure samples and one group of normal samples. (B) Divergence of the HF samples using principal components analysis. Three groups were observed (G1, G2, and G3), which were found to be age-related heart failure.
3.5. Molecular docking and ADMET profiling
The ALKBH5 protein structure (4NJ4) was downloaded from the RCSB PDB databank (https://www.rcsb.org/). Structurally, similar to the 2-oxoglutarate and iron-dependent oxygenase family members, ALKBH566–292 has a double-stranded-helix core fold. An HXD…H motif (comprising residues His204, Asp206, and His266) and three water molecules octahedrally coordinate the active site metal. The ALKBH5 has conserved active site residues and a nucleotide recognition lid as observed in other nucleic acid oxygenases (30). The energy minimized pdb structure of the protein was subjected to DoGSiteScorer server for identification of the putative active sites. The DoGSiteScorer identified 4 putative druggable pockets in the ALKBH5 (Figure 4). The DoGSiteScorer is a tool to examine a protein's structure and to recognize pockets to compute the druggability of protein cavities. The druggability score predicted by the DoGSiteScorer tool ranges from 0 to 1. A value closer to 1 indicates a highly druggable protein cavity where the ligand will bind with high affinity i.e., minimum binding energy.
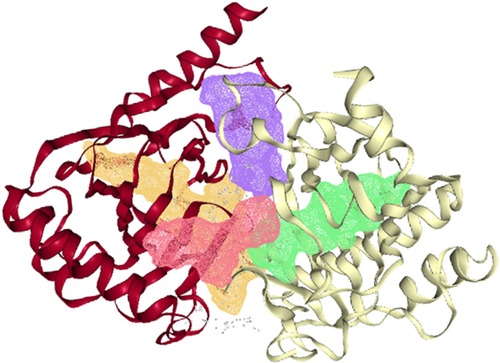
Figure 4. Four putative druggable pockets identified in the pdb structure of ALKBH5 (4nj4) through the online DoGSiteScorer tool. The four pockets are represented by green (druggability score 0.85), violet (score 0.83), yellow (score 0.81), and red (score 0.7) colors inside the ribbons of the protein.
For the virtual screening using the 11,272 compounds (ZINC15 database) by em-ploying the MOE software, 5 best poses for each of the ligand molecules were recorded to find the best hit. The resulting list contained the best hits for the target protein ALKBH5. The interactions between the ligand and the active site of the protein (target-ligand complex structure) were evaluated to highlight the specific residues involved in the putative target activity. The lower energy scores of the MOE program indicates a better ligand–protein binding complex formation compared to high energy values. From the virtual screening, 10 compounds having the least binding energies (dG) with the ALKBH5 were highlighted (Table 2). The lowest binding energy was found for ZINC78774792 compound (dG = −7.8428) which interacted with the Arg130, Asn193, Val202, and Ile268 residues of the active site of ALKBH5 (Figure 5A). There was an arene-H interaction between the ligand and Ile268 residue, 1 proton-acceptor interac-tion with Asn293, and 2 proton-donor interactions between the ligand and Arg130 and Val202 residues. The second lowest binding energy was found for ZINC00546946 (Dg = −7.6338) which interacted with His204 residue (Figure 5B). There was an arene-arene interaction between the ligand and the His204 residue. Three dimensional (3D) repre-sentation of both the docked compounds in the most druggable cavity of ALKBH5 through the DoGSiteScorer indicated the binding of both the compounds in the same orientation (Figure 6).
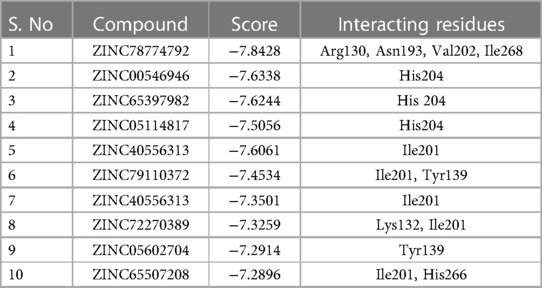
Table 2. Top 10 lead compounds shortlisted after the virtual screening of 11,272 compounds from the ZINC15 database, through the molecular docking to inhibit the ALKBH5 protein. The structures of these compounds have been shown in Supplementary Table S8.
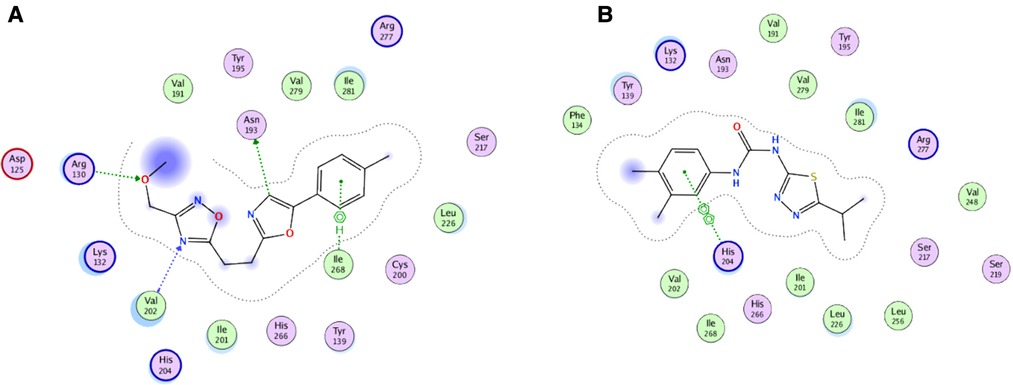
Figure 5. Two-dimensional (2D) representation of ligand-protein target interactions through the MOE. The top two best ranked docked compounds ZINC78774792 (A) and ZINC00546946 (B) in the most druggable cavity of ALKBH5.
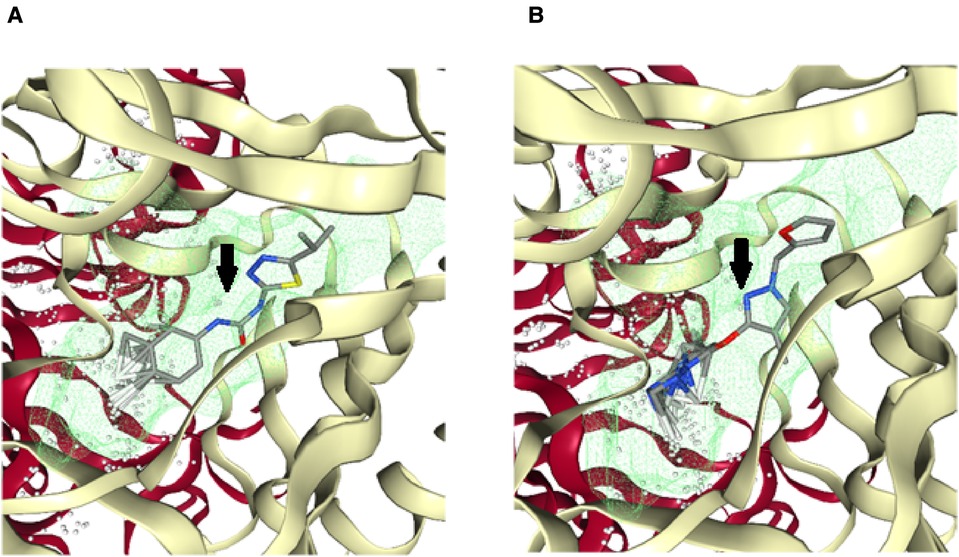
Figure 6. Three-dimensional (3D) cartoonic representation of the docked ligand-protein complexes through the DoGSiteScorer. The top 2 best ranked compounds ZINC78774792 (A) and ZINC00546946 (B) docked in the most druggable cavity of ALKBH5. The docked ligands have been pointed out through black arrows.
For the 10 selected compounds having the lowest binding energies, the ADMET properties (absorption, distribution, metabolism, excretion and toxicity) were studied to check their penetration and least side effects to human and other hosts, if any (Table 3). Out of the 10 short listed ligands, 5 molecules could cross the blood-brain-barrier, and 5 were found to be the substrates for P-glycoprotein (PGP). These compounds were found negative for causing mutagenicity in an Ames test, presumably indicating that these were not or least toxic to the host DNA replication or translation processes.
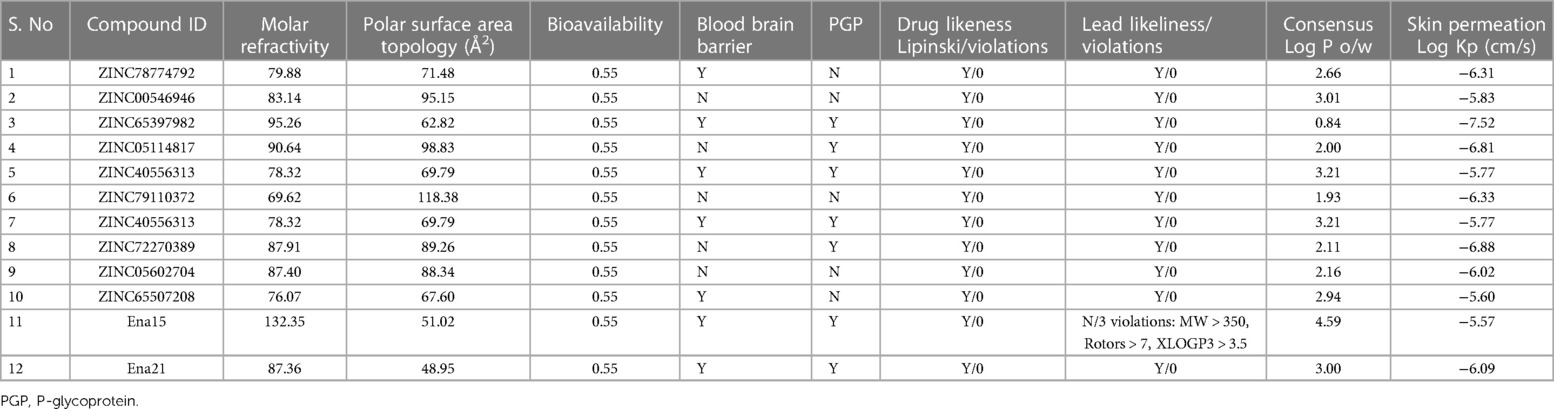
Table 3. Pharmacokinetic parameters of the top-scoring 10 ZINC compounds for the target ALKBH5. Pharmacokinetic parameters of 2 compounds (Ena15, and Ena21) from Takahashi et al. (35) also presented for comparisons.
4. Discussion
Despite several investigations on cardiac dysfunction, the early diagnosis, treatment accuracy, and prognosis for cardiac failure remains unclear. Therefore, it is essential to explore the molecular mechanisms underlying the occurrence and progression of HF. In this study, we performed a series of bioinformatics analyses to explore the key genes and distinct expression profiles involved in the pathophysiology of HF in different age groups. The analysis was carried out on predominantly Caucasian individuals to minimize ethnicity-related bias. Despite several already identified genes in the HF were replicated in this analysis, a few novel genes, previously not or rarely reported in HF, were prioritized with considerable statistical significance (FDR < 0.05). These included the genes involved in platelet activation pathways, such as CAP1, DGKD, F2R, FAM3C, and GNG12, and genes of epitranscriptomics/epigenetics (Supplementary Table S7). Moreover, by applying a machine learning approach, we were able to find expression profiles related to various pathways in different ages of HF patients.
Among the genes of epitranscriptomics regulation, ALKBH5 was found overexpressed in the HF. In a previous study, expression of FTO was found down-regulated causing an increase in global m6A in the HF due to ischemia compared with the normal heart (32). FTO-dependent N6-Methyladenosine regulates cardiac function during remodeling and repair (33). In the present study, which involves HF due to cardiomyopathy, no significant difference in the expression of FTO was observed between the HF and the normal (FDR > 0.05). Instead, the expression of ALKBH5 was found to be increased in HF (FDR < 0.05). In this context, we may hypothesize that the underlying etiology of HF i.e., cardiomyopathy might have resulted in the difference in the regulation of epitranscriptomics. The over-expression of ALKBH5 in the HF in the current analysis was found correlated with the significantly decreased global m6A methylation in the H9c2 cells (Figure 2A). The ALKBH5-induced global demethylation of RNAs may have caused altered splicing, degradation, and translation of the RNAs, as indicated previously (34), leading to the dysregulation of several cellular processes and resulting in HF. Collectively, these observations indicate a more likely role of ALKBH5 in the pathophysiology of HF.
Having prioritized the ALKBH5 through the in silico analysis and validated it through the estimation of global m6A level, ALKBH5 protein expression in H9c2 cells, we considered it a potential therapeutic agent for the treatment of HF. For this purpose, virtual screening (VS) of 11,272 drug-like compounds from the ZINC15 database was carried out in a molecular docking process using the MOE software, and the binding energies of the compounds with the ALKBH5 protein (PDB ID 4NJ4) were determined. The VS of a large number of compounds (drug libraries) is an effective approach to reduce the cost and development time of new drugs. The resulting list of compounds contained the best-hits for the putative ALKBH5 target. The 10 best-hit compounds short-listed in this study also passed the Lipinski's rule of five for the development of new drugs (31). The comparison of binding energies and ADMET parameters of the 10 best-hit compounds of this study with the Ena15 and Ena21 compounds, previously reported as potential inhibitors of ALKBH5 (35), indicated that the binding energy of Ena15 and Ena21 in the ALKBH5 active site was −7.3 kcal/mol, which was slightly higher than the binding energies of 6 out of 10 best-hit compounds found in this study (Table 2). In the ADMET properties, there were three violations from the Lead-likeliness rules for the Ena15 compound, whereas, the Ena21 and all the 10 best-hits of this study passed the Lead-likeliness rules. In this context, we can postulate that Ena21 and our 10 best-hits may be potential inhibitors of ALKBH5, and may be further implicated in drug development.
The epigenetic marker prioritized in this study KMT2E encodes a lysine methyltransferase 2E protein which is involved in chromatin remodeling. Previously, the role of KMT2E expression has been implicated in the prognosis of leukemias (36). However, the role of KMT2E in HF has not been reported yet. KMT2E can act as a potential novel drug target for HF after validating its cellular or organism models. The other genes found over-expressed in HF for the first time in this study included: CAP1 which encodes the cyclase-associated actin cytoskeleton regulatory protein 1, which is an important regulator of actin cytoskeleton remodeling and signal transduction pathways (37). and DGKD encodes diacylglycerol kinase delta, a cytosolic enzyme that phosphorylates diacylglycerol to produce phosphatidic acid, and is associated with the response to elevated platelet cytosolic Ca2+ (38). Here, the over-expression of DGKD might be the result of calcium overload due to the Ca2+ release by the dense tubular system (DTS) and Ca2+ entry into the platelets from the extracellular space, resulting in disruption of haemostasis and vessel integrity in cardiac muscles (39). However, these hypotheses should be validated in animal models in subsequent studies.
Cardiac physiology alters with the passage of age, and studies have suggested that age is an intrinsically a major independent risk factor for CVDs. For example, in various genetically modified aged mice models, the role of adrenergic and renin-angiotensin II signaling, insulin/insulin-like growth factor/PI3K pathway, mitochondrial oxidative stress, and nutrient signaling pathways were observed (40). However, incidences of HF are observed in the elderly as well as in the early ages. Here, we stratified the genes expression profiles in the HF of young-aged adults (average 23.33 years), late-aged adults (∼43 years), and early-aged elders (∼57.66 years) patients. These profiles indicated the likely distinct mechanisms underlying in the HF of different age groups. In young-aged adult patients, the pathways of energy homeostasis [Valine, leucine and isoleucine degradation (hsa00280), and 2-Oxocarboxylic acid metabolism (hsa01210)] were found activated indicating metabolic remodeling in the cardiac tissue and highly likely imparting a part to HF, which correlates with previous reports (41). In late-aged adult HF patients, the genes which are involved in thyroid hormone signaling pathway (hsa04919) and aldosterone-regulated sodium reabsorption pathway (hsa04960) were found predominantly over-expressed in the heart tissue. These pathways have been linked with the higher blood pressure (42). In the early-aged elders of HF, the genes involved in the activation of inflammatory processes, neutrophil chemotaxis, and actin filament organization were found over-expressed. While immune cell infiltrations have been well described in HF (43), recently, the role of actin filament organization has also been described in HF (44). Collectively, these findings suggest that distinct molecular mechanisms operate in the cardiac tissue and disrupt vital cellular processes leading to HF.
5. Conclusion
Given the complex pathophysiology of HF, multiple molecular etiologies impart their roles in the onset of this disease. This study identified novel markers including an epitranscriptomics gene ALKBH5 being over-expressed in the HF. By using the molecular docking, two ZINC15 compounds (ZINC78774792, and ZINC00546946) were prioritized as potential inhibitors which bound in the ALKBH5 active site with the lowest binding energies. Additionally, distinct gene expression profiles in the HF of young-aged adults (∼23 years), late-aged adults (∼43 years), and early-aged elders (∼57.6 years) were identified by employing the unsupervised hierarchal clustering. Additionally, the genes of actin cytoskeleton regulation (hsa04810), focal adhesion (hsa04510), and PI3K-Akt signaling pathway (hsa04151) were having lower expression in male heart failures compared with females. These preliminary findings of the study identified some of molecular mechanisms involved in heart failure. We suggest to validate these results in animal models to obtain definitive conclusions. Furthermore, the identified compounds may have potential for treating HF, and further investigations using animal models could evaluate their efficacy.
Data availability statement
The datasets presented in this study can be found in online repositories. The names of the repository/repositories and accession number(s) can be found in the article/Supplementary Material.
Authors contributions
SK, AG, SA, and L-GC performed data retrieval, data analysis, experiments, and manuscript write-up. IAK performed data analysis, reviewed manuscript and put intellectual insight. SK, AG, S-NH and MS conceived and designed the experiments. SK, MS, L-RZ, and S-NH contributed to the conceptualization, and manuscript writing.
Funding
This work was supported by the National Natural Science Foundation of China (No. 81670311).
Conflict of interest
The authors declare that the research was conducted in the absence of any commercial or financial relationships that could be construed as a potential conflict of interest.
Publisher's note
All claims expressed in this article are solely those of the authors and do not necessarily represent those of their affiliated organizations, or those of the publisher, the editors and the reviewers. Any product that may be evaluated in this article, or claim that may be made by its manufacturer, is not guaranteed or endorsed by the publisher.
Supplementary material
The Supplementary Material for this article can be found online at: https://www.frontiersin.org/articles/10.3389/fcvm.2023.1194311/full#supplementary-material
References
1. Savarese G, Lund LH. Global public health burden of heart failure. Cardiac Fail Rev. (2017) 3(1):7. doi: 10.15420/cfr.2016:25:2
2. Tomasoni D, Adamo M, Anker MS, von Haehling S, Coats AJ, Metra M. Heart failure in the last year: progress and perspective. ESC Heart Fail. (2020) 7(6):3505–30. doi: 10.1002/ehf2.13124
4. Komal S, Han SN, Cui LG, Zhai MM, Zhou YJ, Wang P, et al. Epigenetic regulation of macrophage polarization in cardiovascular diseases. Pharmaceuticals. (2023) 16(2):141. doi: 10.3390/ph16020141
5. Puggia I, Rowland TJ, Miyamoto SD, Sinagra G, Mestroni L. Molecular and cellular mechanisms in heart failure. In: Heart failure in the child and young adult. United States: Academic Press, Elsevier. (2018): p. 3–19.
6. Hill JA, Olson EN. Cardiac plasticity. N Engl J Med. (2008) 358(13):1370–80. doi: 10.1056/NEJMra072139
7. Cohn JN, Tognoni G. A randomized trial of the angiotensin-receptor blocker valsartan in chronic heart failure. N Engl J Med. (2001) 345(23):1667–75. doi: 10.1056/NEJMoa010713
8. Zeisberg EM, Tarnavski O, Zeisberg M, Dorfman AL, McMullen JR, Gustafsson E, et al. Endothelial-to-mesenchymal transition contributes to cardiac fibrosis. Nat Med. (2007) 13(8):952–61. doi: 10.1038/nm1613
9. Janicki JS, Brower GL. The role of myocardial fibrillar collagen in ventricular remodeling and function. J Card Fail. (2002) 8(6):S319–25. doi: 10.1054/jcaf.2002.129260
10. Misra A, Haudek SB, Knuefermann P, Vallejo JG, Chen ZJ, Michael LH, et al. Nuclear factor-κB protects the adult cardiac myocyte against ischemia-induced apoptosis in a murine model of acute myocardial infarction. Circulation. (2003) 108(25):3075–8. doi: 10.1161/01.CIR.0000108929.93074.0B
11. Gordon JW, Shaw JA, Kirshenbaum LA. Multiple facets of NF-κB in the heart: to be or not to NF-κB. Circ Res. (2011) 108(9):1122–32. doi: 10.1161/CIRCRESAHA.110.226928
12. Hilfiker-Kleiner D, Landmesser ULF, Drexler H. Molecular mechanisms in heart failure: focus on cardiac hypertrophy, inflammation, angiogenesis, and apoptosis. J Am Coll Cardiol. (2006) 48(9S):A56–66. doi: 10.1016/j.jacc.2006.07.007
13. Ooi BK, Goh BH, Yap WH. Oxidative stress in cardiovascular diseases: involvement of Nrf2 antioxidant redox signaling in macrophage foam cells formation. Int J Mol Sci. (2017) 18(11):2336. doi: 10.3390/ijms18112336
14. Yue Y, Liu J, He C. RNA N6-methyladenosine methylation in post-transcriptional gene expression regulation. Genes Dev. (2015) 29(13):1343–55. doi: 10.1101/gad.262766.115
15. Wang X, Zhao BS, Roundtree IA, Lu Z, Han D, Ma H, et al. N6-methyladenosine modulates messenger RNA translation efficiency. Cell. (2015) 161(6):1388–99. doi: 10.1016/j.cell.2015.05.014
16. Meyer KD, Patil DP, Zhou J, Zinoviev A, Skabkin MA, Elemento O, et al. 5′ UTR m6A promotes cap-independent translation. Cell. (2015) 163(4):999–1010. doi: 10.1016/j.cell.2015.10.012
17. Komal S, Zhang LR, Han SN. Potential regulatory role of epigenetic RNA methylation in cardiovascular diseases. Biomed Pharmacother. (2021) 137:111376. doi: 10.1016/j.biopha.2021.111376
18. Kmietczyk V, Riechert E, Kalinski L, Boileau E, Malovrh E, Malone B, et al. m6A-mRNA methylation regulates cardiac gene expression and cellular growth. Life Sci Alliance. (2019) 2(2):1–12. doi: 10.26508/lsa.201800233
19. Longenecker JZ, Gilbert CJ, Golubeva VA, Martens CR, Accornero F. Epitranscriptomics in the heart: a focus on m6A. Curr Heart Fail Rep. (2020) 17:205–12. doi: 10.1007/s11897-020-00473-z
20. Xiao MZ, Liu JM, Xian CL, Chen KY, Liu ZQ, Cheng YY. Therapeutic potential of ALKB homologs for cardiovascular disease. Biomed Pharmacother. (2020) 131:110645. doi: 10.1016/j.biopha.2020.110645
21. Li G, Song Y, Liao Z, Wang K, Luo R, Lu S, et al. Bone-derived mesenchymal stem cells alleviate compression-induced apoptosis of nucleus pulposus cells by N6 methyladenosine of autophagy. Cell Death Dis. (2020) 11(2):103. doi: 10.1038/s41419-020-2284-8
22. Leinonen R, Sugawara H, Shumway M, Collaboration INSD. The sequence read archive. Nucleic Acids Res. (2010) 39(suppl_1):D19–21. doi: 10.1093/nar/gkq1019
23. Andrews S. FastQC: a quality control tool for high throughput sequence data. Babraham bioinformatics. Cambridge, United Kingdom: Babraham Institute (2010).
24. Bolger AM, Lohse M, Usadel B. Trimmomatic: a flexible trimmer for illumina sequence data. Bioinformatics. (2014) 30(15):2114–20. doi: 10.1093/bioinformatics/btu170
25. Dobin A, Davis CA, Schlesinger F, Drenkow J, Zaleski C, Jha S, et al. STAR: ultrafast universal RNA-Seq aligner. Bioinformatics. (2013) 29(1):15–21. doi: 10.1093/bioinformatics/bts635
26. Leptidis S, Papakonstantinou E, Diakou KI, Pierouli K, Mitsis T, Dragoumani K, et al. Epitranscriptomics of cardiovascular diseases (review). Int J Mol Med. (2022) 49(1):1–21. doi: 10.3892/ijmm.2021.5064
27. Chang C, Wang SH, Xu LN, Su XL, Zeng YF, Wang P, et al. Glycogen synthase kinase 3 beta inhibitor SB216763 improves Kir2. 1 expression after myocardia infraction in rats. J Interv Card Electrophysiol. (2022) 63(2):239–48. doi: 10.1007/s10840-021-00963-7
28. Vilar S, Cozza G, Moro S. Medicinal chemistry and the molecular operating environment (MOE): application of QSAR and molecular docking to drug discovery. Curr Top Med Chem. (2008) 8(18):1555–72. doi: 10.2174/156802608786786624
29. Szklarczyk D, Gable AL, Nastou KC, Lyon D, Kirsch R, Pyysalo S, et al. The STRING database in 2021: customizable protein–protein networks, and functional characterization of user-uploaded gene/measurement sets. Nucleic Acids Res. (2021) 49(D1):D605–12. doi: 10.1093/nar/gkaa1074
30. Aik W, Scotti JS, Choi H, Gong L, Demetriades M, Schofield CJ, et al. Structure of human RNA N 6-methyladenine demethylase ALKBH5 provides insights into its mechanisms of nucleic acid recognition and demethylation. Nucleic Acids Res. (2014) 42(7):4741–54. doi: 10.1093/nar/gku085
31. Lipinski CA, Lombardo F, Dominy BW, Feeney PJ. Experimental and computational approaches to estimate solubility and permeability in drug discovery and development settings. Adv Drug Delivery Rev. (2012) 64:4–17. doi: 10.1016/j.addr.2012.09.019
32. Berulava T, Buchholz E, Elerdashvili V, Pena T, Islam MR, Lbik D, et al. Changes in m6A RNA methylation contribute to heart failure progression by modulating translation. Eur J Heart Fail. (2020) 22(1):54–66. doi: 10.1002/ejhf.1672
33. Mathiyalagan P, Adamiak M, Mayourian J, Sassi Y, Liang Y, Agarwal N, et al. FTO-Dependent m6A regulates cardiac function during remodeling and repair. Circulation. (2019) 139(4):518–32. doi: 10.1161/CIRCULATIONAHA.118.033794
34. Wang X, Li Z, Kong B, Song C, Cong J, Hou J, et al. Reduced m6A mRNA methylation is correlated with the progression of human cervical cancer. Oncotarget. (2017) 8(58):98918. doi: 10.18632/oncotarget.22041
35. Takahashi H, Hase H, Yoshida T, Tashiro J, Hirade Y, Kitae K, et al. Discovery of two novel ALKBH5 selective inhibitors that exhibit uncompetitive or competitive type and suppress the growth activity of glioblastoma multiforme. Chem Biol Drug Des. (2022) 100(1):1–12. doi: 10.1111/cbdd.14051
36. Damm F, Oberacker T, Thol F, Surdziel E, Wagner K, Chaturvedi A, et al. Prognostic importance of histone methyltransferase MLL5 expression in acute myeloid leukemia. J Clin Oncol. (2011) 29(6):682–9. doi: 10.1200/JCO.2010.31.1118
37. Rust MB, Marcello E. Disease association of cyclase-associated protein (CAP): lessons from gene-targeted mice and human genetic studies. Eur J Cell Biol. (2022) 101(2):151207. doi: 10.1016/j.ejcb.2022.151207
38. DGKD Gene—GeneCards|DGKD Protein|DGKD Antibody. Available at: https://www.genecards.org/cgi-bin/carddisp.pl?gene=DGKD (Cited November 27, 2022).
39. Davlouros P, Xanthopoulou I, Mparampoutis N, Giannopoulos G, Deftereos S, Alexopoulos D. Role of calcium in platelet activation: novel insights and pharmacological implications. Med Chem. (2016) 12(2):131–8. doi: 10.2174/157340641202160208195923
40. Dai DF, Chen T, Johnson SC, Szeto H, Rabinovitch PS. Cardiac aging: from molecular mechanisms to significance in human health and disease. Antioxid Redox Signal. (2012) 16(12):1492–526. doi: 10.1089/ars.2011.4179
41. Chen L, Song J, Hu S. Metabolic remodeling of substrate utilization during heart failure progression. Heart Fail Rev. (2019) 24(1):143–54. doi: 10.1007/s10741-018-9713-0
42. Tsilosani A, Gao C, Zhang W. Aldosterone-regulated sodium transport and blood pressure. Front Physiol. (2022) 13:770375. doi: 10.3389/fphys.2022.770375
43. Brenes-Castro D, Castillo EC, Vázquez-Garza E, Torre-Amione G, García-Rivas G. Temporal frame of immune cell infiltration during heart failure establishment: lessons from animal models. Int J Mol Sci. (2018) 19(12):3719. doi: 10.3390/ijms19123719
Keywords: AlkB homolog 5, epitranscriptomics, N6-methyladenosine, transcriptome, heart failure
Citation: Komal S, Gohar A, Althobaiti S, Ahmad Khan I, Cui L-G, Zhang L-R, Han S-N and Shakeel M (2023) ALKBH5 inhibitors as a potential treatment strategy in heart failure—inferences from gene expression profiling. Front. Cardiovasc. Med. 10:1194311. doi: 10.3389/fcvm.2023.1194311
Received: 28 March 2023; Accepted: 18 July 2023;
Published: 31 July 2023.
Edited by:
Mark J. Ranek, Johns Hopkins University, United StatesReviewed by:
Malik Bisserier, New York Medical College, United StatesKimberly M. Ferrero, Johns Hopkins University, United States
© 2023 Komal, Gohar, Althobaiti, Ahmad Khan, Cui, Zhang, Han and Shakeel. This is an open-access article distributed under the terms of the Creative Commons Attribution License (CC BY). The use, distribution or reproduction in other forums is permitted, provided the original author(s) and the copyright owner(s) are credited and that the original publication in this journal is cited, in accordance with accepted academic practice. No use, distribution or reproduction is permitted which does not comply with these terms.
*Correspondence: Muhammad Shakeel bXNoYWtlZWxfMjExQHlhaG9vLmNvbQ== Sheng-Na Han aGFuc2hlbmduYUAxMjYuY29t
†These authors have contributed equally to this work, and share first authorship