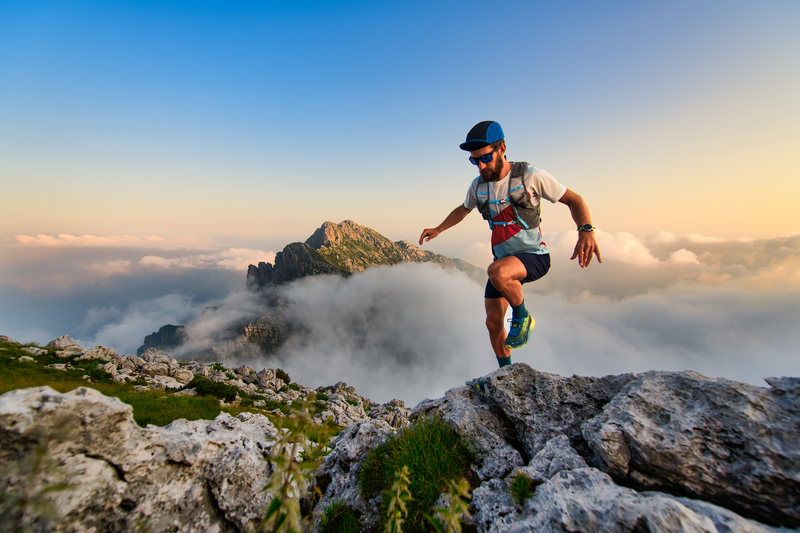
94% of researchers rate our articles as excellent or good
Learn more about the work of our research integrity team to safeguard the quality of each article we publish.
Find out more
ORIGINAL RESEARCH article
Front. Cardiovasc. Med. , 03 May 2023
Sec. Atherosclerosis and Vascular Medicine
Volume 10 - 2023 | https://doi.org/10.3389/fcvm.2023.1161761
This article is part of the Research Topic Revolutions in Human Biospecimen Study: Leveraging new technologies to explore the pathology, therapeutics and biomarkers for atherosclerotic disease View all 10 articles
Background: The intermediate metabolites associated with the development of atherosclerotic cardiovascular disease (ASCVD) remain largely unknown. Thus, we conducted a large panel of metabolomics profiling to identify the new candidate metabolites that were associated with 10-year ASCVD risk.
Methods: Thirty acylcarnitines and twenty amino acids were measured in the fasting plasma of 1,102 randomly selected individuals using a targeted FIA-MS/MS approach. The 10-year ASCVD risk score was calculated based on 2013 ACC/AHA guidelines. Accordingly, the subjects were stratified into four groups: low-risk (n = 620), borderline-risk (n = 110), intermediate-risk (n = 225), and high-risk (n = 147). 10 factors comprising collinear metabolites were extracted from principal component analysis.
Results: C4DC, C8:1, C16OH, citrulline, histidine, alanine, threonine, glycine, glutamine, tryptophan, phenylalanine, glutamic acid, arginine, and aspartic acid were significantly associated with the 10-year ASCVD risk score (p-values ≤ 0.044). The high-risk group had higher odds of factor 1 (12 long-chain acylcarnitines, OR = 1.103), factor 2 (5 medium-chain acylcarnitines, OR = 1.063), factor 3 (methionine, leucine, valine, tryptophan, tyrosine, phenylalanine, OR = 1.074), factor 5 (6 short-chain acylcarnitines, OR = 1.205), factor 6 (5 short-chain acylcarnitines, OR = 1.229), factor 7 (alanine, proline, OR = 1.343), factor 8 (C18:2OH, glutamic acid, aspartic acid, OR = 1.188), and factor 10 (ornithine, citrulline, OR = 1.570) compared to the low-risk ones; the odds of factor 9 (glycine, serine, threonine, OR = 0.741), however, were lower in the high-risk group. “D-glutamine and D-glutamate metabolism”, “phenylalanine, tyrosine, and tryptophan biosynthesis”, and “valine, leucine, and isoleucine biosynthesis” were metabolic pathways having the highest association with borderline/intermediate/high ASCVD events, respectively.
Conclusions: Abundant metabolites were found to be associated with ASCVD events in this study. Utilization of this metabolic panel could be a promising strategy for early detection and prevention of ASCVD events.
Cardiovascular disease (CVD) is a disorder of the heart/vessels known as the most important reason behind global mortality. The contribution of CVD to the death rate has increased continuously (1, 2). Therefore, it is crucial to identify high-risk individuals to prevent CVD and its associated complications and reduce the burden of the disease and mortality (3). To this purpose, different CVD risk assessment scoring systems have been developed so far (4). One of the recent most-applied tools is the 10-year atherosclerotic CVD (ASCVD) risk index that was presented by the American Heart Association (AHA)/ the American College of Cardiology (ACC) (5). This risk score was calculated based on several characteristics of individuals including age, sex, race, total cholesterol, high-density lipoprotein cholesterol (HDL-C), systolic blood pressure, antihypertensive treatment, smoking status, and diabetes mellitus history (6, 7).
Although there have been many advances in the development of predictive CVD risk assessment tools, the underlying molecular pathomechanisms of ASCVD events are largely unrecognized. This issue revealed the necessity for conducting metabolomics analysis (8). The metabolomics approach allows for a better understanding of the intermediate metabolites associated with ASCVD events and leads to the identification of new diagnostic and therapeutic strategies (9, 10). Indeed, physiologic perturbations, particularly in individuals at high risk of ASCVD events, can rapidly affect metabolite profiling, which can be targeted for disease management (11). Herein, several metabolomics studies have been conducted to determine the association between metabolic profiles and CVD risk, which showed that branched-chain amino acids and urea cycle-related metabolites, were associated with higher cardiovascular risk (12, 13). However, these studies identified the association between a number of acylcarnitines/amino acids and CVD risk, but the results are not easily comparable, e.g., isoleucine, leucine, and glutamine were associated with cardiovascular events in one study (13) while these metabolites were not associated with an increased incidence of cardiovascular events in another study (12). So, intermediate metabolites associated with developing ASCVD events remain largely unknown. Thus, to better understand the pathogenesis of the disease, in this study, we conducted the comprehensive metabolomics profiling of plasma in a large-scale Iranian population and determined the association between plasma metabolite levels and the 10-year ASCVD risk score.
1,102 individuals aged between 40 and 79 years old with LDL levels less than 190 mg/dl and no pre-existing coronary artery disease or myocardial infarction were randomly selected from a survey of Surveillance of Risk Factors of Non-Communicable Diseases in Iran (STEPs 2016). In brief, the STEPs 2016 protocol, previously published, includes 31,050 individuals older than 18 years from the rural and urban areas of 389 Iranian districts selected using a systematic cluster random sampling (14). The study protocol conforms to the ethical guidelines of the 1975 Declaration of Helsinki. The Ethics Committee of Tehran University of Medical Sciences and Endocrine & Metabolism Research Institute approved the study protocol with the ID number IR.TUMS.EMRI.REC.1395.00141, and written informed consent was obtained before participation.
The blood was sampled from peripheral venous following at least 12 h of overnight fasting and stored in sodium fluoride plus EDTA tubes. A part of the whole blood was used for HbA1c measurement and the plasma of the remaining blood was isolated for other biochemical laboratory testing using commercial Roche kits (Roche Diagnostics, Mannheim, Germany) and Cobas C311 auto analyzer.
The concentration of 30 acylcarnitines and 20 amino acids were measured in plasma using flow injection tandem mass spectrometry (triple quadrupole SCIEX API 3,200 with electrospray ionization) equipped with a Thermo Scientific Dionex UltiMate 3,000 standard HPLC system and a derivatization method with butanol-HCL (15). Briefly, the mixture of plasma samples and internal standard were centrifuged at 4°C. Supernatant fluids were collected in new vials and dried with nitrogen 99.9% at 45°C. Derivatization solution, a combination of 1-butanol and acetyl chloride, was then added to the vials with the aim of protein precipitation. Also, derivatization with butanol-HCL has the benefit of simultaneous derivatization of amino acids and acylcarnitines which makes the measurement, faster and cheaper. After vortexing, they were incubated at 65°C for 15 min with the advantage of the effective destruction of phospholipids. The samples were dried with nitrogen 99.9% at 45°C, then dissolved in 100 µl of mobile phase solution, a mix of water and acetonitrile, before injection. Ratios of the signals of the metabolites relative to the internal standards were used to make calibration curves and calculate analyte concentrations in the QC materials and samples.
The 10-year risk for primary atherosclerotic cardiovascular disease was calculated based on pooled cohort equations in the 2013 ACC/AHA Guidelines (16). The equation predicts the risk of stroke, nonfatal myocardial infarction, and coronary artery disease death within 10 years in subjects aged between 40 and 79 years old with LDL < 190 mg/dl and no pre-existing ASCVD events. The equation takes into account age, gender, race, HDL cholesterol, total cholesterol, systolic blood pressure, the use of hypertension drugs, smoking habits, and diabetes. The individuals were stratified into four groups according to their 10-year ASCVD risk score and the threshold was determined based on the special report from AHA/ACC (6): (1) Low-risk group (n = 620) with a score of 0% to 5%, (2) Borderline-risk group (n = 110) with a score of 5% to 7.5%, (3) Intermediate-risk group (n = 225) with a score of 7.5% to 20%, and (4) High-risk group (n = 147) with a score of 20% or more.
SPSS version 19.0 was used for statistical analyses. The workflow diagram of the statistical analysis is presented in Additional File S1. After checking the normality by Kolmogorov–Smirnov test, mean (± standard deviation) and median (interquartile range) were applied to describe continuous variables with and without normal distributions, respectively. Number and percentage (%) were applied to describe categorical variables. Chi-squared test was used to compare the frequency of categorical variables between the groups. ANOVA test and Bonferroni post hoc test were used to compare normally distributed variables between the groups. The Kruskal-Wallis and Mann–Whitney U tests were used to compare non-normally distributed variables between the groups. Benjamini-Hochberg method was applied for the adjustment of p-values obtained from the Kruskal-Wallis H test of the metabolite concentrations. The correlation between the metabolite concentrations and the 10-year ASCVD risk was determined using the Spearman correlation coefficient and the stepwise multiple linear regression analysis methods. To standardize the data on metabolite level, we calculated the natural logarithm of metabolite concentration and considered their Z value for logistic regression analysis and factor analysis. Binary logistic regression analysis was used to determine the odds ratio (OR) of metabolite profile in the borderline/intermediate/high-risk groups compared to the low-risk one. For factor analysis, the Kaiser-Meyer-Olkin (KMO) test and Bartlett's Test of Sphericity were used to investigate the adequacy of sample size and for statistical comparison of the correlation matrix with the identity matrix. KMO values ≥ 0.80 were considered credible. Principal component analysis (PCA) with varimax rotation was performed to reduce the metabolites into a smaller subclass of orthogonal (uncorrelated) factors. The extracted factors with eigenvalues higher than 1.0 and metabolites with loading scores more than 0.4 were considered important for the given PCA. The factor score was calculated through the sum of obtained metabolite concentrations multiplied by their loading matrix which was obtained from the rotated component matrix with the rotation method of Varimax with Kaiser Normalization (Additional File S2). Furthermore, MetaboAnalyst (Version 5.0) based on the metabolic Kyoto Encyclopedia of Genes and Genomes (KEGG) database was used for enrichment pathway analysis of metabolic alterations between study groups that were identified from logistic regression analysis. The enrichment ratio is calculated based on the observed hits divided by expected hits. A p-value less than 0.05 was considered significant in all analyses.
The study population comprised 1,102 participants with a mean age of 54.41 ± 10.22 years and a female percentage of 53.27%. The sociodemographic and laboratory characteristics of the low/borderline/intermediate/high ASCVD risk groups are shown in Table 1. The groups had no significant difference with regard to BMI and cholesterol levels (p-value ≥ 0.056).
Tables 2, 3 demonstrate the median (interquartile range) of plasma concentration of 30 acylcarnitines and 20 amino acids in the four study groups, respectively. For ease of visualization of the data scatter, the concentration of acylcarnitines/amino acids in the four study groups is plotted in Additional File S3. The correlation matrix between metabolites and general features of participants is shown in Figure 1. The significantly altered metabolites between male and female participants for the four study groups are shown in Additional File S4.
The result of the logistic regression on metabolites to discriminate borderline/intermediate/high ASCVD risk patients from low-risk ones is presented in Additional File S5. Patients with high ASCVD risk score were more likely to have an increase in the concentration of 24 acylcarnitines and nine amino acids (p-value ≤ 0.041) and were less likely to have an increase in five amino acids than low ASCVD risk group (p-value ≤ 0.010). Furthermore, an increase in 18 acylcarnitines and 13 amino acids was more likely to occur in intermediate ASCVD risk patients than in low ASCVD risk patients (p-value ≤ 0.044). However, an increase in two amino acids was less likely to occur in intermediate ASCVD risk patients than in low ASCVD risk patients (p-value ≤ 0.013). Patients with borderline ASCVD risk score were more likely to have an increase in 24 acylcarnitines and nine amino acids than low ASCVD risk patients (p-value ≤ 0.041) and were less likely to have an increase in five amino acids than low ASCVD risk patients (p-value ≤ 0.010). The logistic regression analysis was repeated after adjustment for BMI; in the new analysis, the significant p-values remained significant, and non-significant ones remained non-significant.
Additional File S6 shows the correlation between the metabolite profile and the 10-year ASCVD risk score. Thirty acylcarnitines and nine amino acids were positively associated with the ASCVD risk score (p ≤ 0.034) and four amino acids were inversely related to it (p ≤ 0.026). The strongest associations belonged to 3-OH-hexadecanoylcarnitine (C16OH, r = 0.279, p < 0.001), propionyl carnitine (C3, r = 0.262, p < 0.001), leucine (r = 0.256, p < 0.001), isovaleryl carnitine (C5, r = 0.251, p < 0.001), and 3-OH-isovalerylcarnitine (C5OH, r = 0.250, p < 0.001). The multiple linear regression on all measured metabolites is summarized in Table 4. Three acylcarnitines (methylmalonyl-/succinyl carnitine [C4DC], octenoyl carnitine [C8:1], and 3-OH-hexadecanoylcarnitine [C16OH]) and 11 amino acids (citrulline, histidine, alanine, threonine, glycine, glutamine, tryptophan, phenylalanine, glutamic acid, arginine, aspartic acid) were concluded as possible predictors of the 10-year ASCVD risk score.
In the factor analysis, the KMO coefficient was 0.874, indicating adequate sampling of data. A p-value of <0.001 from Bartlett's sphericity test suggested a statistical difference between the correlation and the identity matrix. Both tests revealed the data to be appropriate for factor analysis.
PCA analysis on the standardized metabolites resulted in 11 factors with an eigenvalue of more than one in the screen plot (Additional File S7). The logistic regression analysis of the borderline/intermediate/high-risk groups compared to the low-risk group is illustrated in Table 5. Patients with high ASCVD risk score were more likely to have an increase in factors 1, 2, 3, 5, 6, 7, 8, and 10 than low ASCVD risk group (p-value ≤ 0.009) and were less likely to have an increase in factor nine (p-value < 0.001). The intermediate-risk group was more likely to have an increase in factors 1, 3, 5, 6, 7, 8, and 10 than the low-risk group (p-value ≤ 0.001) and was less likely to have an increase in factor 9 than the low-risk group (p-value = 0.001). Patients with borderline ASCVD risk score were more likely to have an increase in factors 3, 5, 6, 7, and 10 than the low-risk group (p-value ≤ 0.009).
The results of pathway enrichment analysis of altered metabolites in low ASCVD risk group with borderline/intermediate/high ASCVD risk groups are summarized in Additional File S8. The differential metabolites between the low ASCVD risk group and borderline/intermediate/high ASCVD risk groups were mainly associated with the biological processes of “aminoacyl-tRNA biosynthesis”, “arginine biosynthesis”, “D-glutamine and D-glutamate metabolism”, “valine, leucine and isoleucine biosynthesis”, and “phenylalanine, tyrosine, and tryptophan biosynthesis” (Figures 2A–C). The metabolic pathways with the highest enrichment ratio in explaining the differences in metabolic profile between the low ASCVD risk group and the borderline/intermediate/high ASCVD risk groups were “D-glutamine and D-glutamate metabolism”, “phenylalanine, tyrosine, and tryptophan biosynthesis”, and “valine, leucine, and isoleucine biosynthesis”, respectively. The working model of the metabolic perturbance is shown in Figure 3.
Figure 2. Pathway enrichment analysis between (A) low ASCVD risk group and borderline ASCVD risk group, (B) low ASCVD risk group and intermediate ASCVD risk group, and (C) low ASCVD risk group and high ASCVD risk group. The color of each pathway is based on the p-value [-log(p): logarithm of the p-value, Fisher's test p-value].
It has been discovered that the metabolite profile has a significant correlation with ASCVD risk such as coronary artery disease. Moreover, certain metabolic features can be considered independent and valuable predictors of cardiovascular events with regard to early diagnosis, treatment strategies, and better risk stratification (13). In this study, the 10-year ASCVD risk score was used to classify individuals into four groups: low-, borderline-, intermediate-, and high-risk of ASCVD events. Consequently, their metabolite profile (the plasma concentration of 30 acylcarnitines and 20 amino acids) was measured to determine the association between metabolite profile and the risk of 10-year ASCVD events.
The results of our study indicated a positive correlation between all 30 acylcarnitines, alanine, glutamic acid, leucine, phenylalanine, tyrosine, valine, citrulline, ornithine, and proline and the 10-year ASCVD risk score and a negative correlation of glycine, threonine, serine, and histidine with this score. In accordance with our findings, Würtz P et al. (12) showed a positive correlation between the circulating concentrations of phenylalanine and tyrosine and cardiovascular risk. Ruiz-Canela M et al. (17) in a case-cohort study have shown the direct correlation between higher concentrations of branched-chain amino acids (BCAAs) including leucine and valine, and higher CVD risk. The important role of BCAAs as the predictive markers of cardiovascular events has been also suggested in another study by Hu W et al. (18). Vaarhorst AA et al. (19) reported that blood concentrations of valine, ornithine, and glutamate were associated with coronary heart disease. Shah SH et al. (13) found a considerable association between the plasma levels of glutamate, leucine, isoleucine, citrulline, C2, C8, C10:1, C14:2 and CVD risk in the same direction that we found in the current study. Besides, the elevated levels of serum C2 and C8 acylcarnitines are in relation to higher cardiovascular death risk. They could also be correlated with a modest increase in the risk of fatal/nonfatal acute myocardial infarction in patients with stable angina pectoris (20). As shown in Table 6, these findings from the previous studies are in line with our findings.
This study has also found from multiple linear analysis that C4DC, C8:1, and C16OH, citrulline, histidine, alanine, threonine, glycine, glutamine, tryptophan, phenylalanine, glutamic acid, arginine, and aspartic acid could be considered as new candidate biomarkers for the 10-year ASCVD risk. Based on a study by Hu W et al. (18), there is an independent association between BCAAs and intima-media thickness as a marker of coronary artery disease. Several other studies have also indicated a strong association between acylcarnitines and cardiovascular events (21, 22). In addition, metabolite disturbances can also contribute to the CVD risk factors such as diabetes mellitus as found in many studies (23–27). In this regard, plasma levels of BCAA and aromatic amino acids (AAAs) particularly tyrosine, phenylalanine, and isoleucine can predict both diabetes mellitus and CVD events (28, 29).
Metabolomics can help us identify new intermediate metabolites to better understand the underlying mechanisms responsible for higher 10-year ASCVD risk. For instance, the odds ratio between low- and high/intermediate-risk patients can be explained by certain underlying pathways including aminoacyl-tRNA biosynthesis, valine, leucine, and isoleucine biosynthesis, arginine biosynthesis, phenylalanine, tyrosine, and tryptophan biosynthesis, D-glutamine and D-glutamate metabolism, phenylalanine metabolism, and glutathione metabolism. The role of these underlying mechanisms in the development of cardiovascular events has been stated in previous studies (30–32). For instance, the aminoacyl-tRNA synthetases are found to have an important role in modifying the function of regulatory proteins in different cellular processes. The effects of aminoacyl-tRNA synthetase pathways on coronary arteries, aorta, cardiomyocytes, and fibroblasts and their strong association with angiogenesis and cardiomyopathy have been identified previously, and it suggested the potential role of aminoacyl-tRNA synthetase pathways as therapeutic/diagnostic targets of ASCVDs (30). In addition, the disturbed mechanisms of valine, leucine, isoleucine, and their α-keto acids were reported in animals suffering from cardiac events (31, 32). Arginine is another amino acid with an important role in different cellular processes such as protein biosynthesis. Furthermore, it is also involved in vascular tone and endothelial function affecting cardiovascular function (33). Another study has also introduced phenylalanine, tyrosine, and tryptophan biosynthesis (using multi-omics approaches) to be involved in vascular function alterations (34). Phenylalanine metabolism has also been shown to have considerable effects on predicting poor outcomes in critically ill patients presenting with heart failure (35). The pathogenic role of high phenylalanine levels in cardiac aging has also been found (36). On the other hand, glutathione metabolism is also known to have considerable effects on various cell functions during CVD events such as cytokine production and protein synthesis. In this regard, it has been stated that glutathione deficiency can be associated with heart attack and stroke (37). Besides, glutathione metabolism is associated with poor outcomes such as death or re-hospitalization in heart failure patients (38).
Published articles regarding the metabolomics analysis of CVD risk, particularly those on amino acids or acylcarnitines mainly utilize the Framingham risk score for risk estimation of CVD events (13, 18). Despite the effectiveness of the ASCVD risk score and its preference in many cases (39), there are not many articles that have used the ASCVD risk score for that purpose. On the other hand, there have been fewer investigations on acylcarnitines compared with other metabolites like amino acids. The other strength of this study is its large population and broad list of investigated metabolites, which makes its results more valuable. Besides, various acylcarnitines generally showed a stronger association with CVD risk in fasting subjects than in nonfasting subjects (20); so, we measured the concentration of metabolites in fasting plasma. However, this study also has some limitations. The cross-sectional nature of the study prevents us from finding any temporality and actual causality associations between the 10-year ASCVD risk and metabolite profile. This study was conducted on Iranian individuals, which limits the generalization of the results to different populations. Also, the risk of actual ASCVD events according to the metabolic profile was not investigated. Additionally, meaningful differences in the studied patient groups might have affected the metabolite levels. Although these variabilities were adjusted in the risk score estimation equation, their existence is one of the limitations of the present study. Despite all the benefits of metabolic profiling in predictive strategies, its generalizability and reproducibility have to be investigated through comprehensive studies to establish its potential usefulness in the clinical setting (40). Thus, longitudinal studies are needed to confirm the predictive value of the metabolite profile on the ASCVD risk.
This study identified many amino acids and acylcarnitines as metabolite fingerprints of individuals at higher risk of 10-year ASCVD events. Among them, our results suggest C4DC, C8:1, C16OH, citrulline, histidine, alanine, threonine, glycine, glutamine, tryptophan, phenylalanine, glutamic acid, arginine, and aspartic acid be associated with the 10-year ASCVD risk score and thus could be used as potential predictive biomarkers for the ASCVD events. Furthermore, several metabolic pathways associated with the development of 10-year ASCVD events were identified. Among them, “D-glutamine and D-glutamate metabolism”, “phenylalanine, tyrosine, and tryptophan biosynthesis”, and “valine, leucine, and isoleucine biosynthesis” showed the most influential pathways in this pathogenesis. These findings allow for a better understanding of mechanisms underlying ASCVD events and then were used in diagnostic and therapeutic strategies. However, more studies are still required to confirm this association in larger populations.
Acylcarnitines name: Free carnitine (C0), Acetyl carnitine (C2), Propionyl carnitine (C3), Malonyl carnitine (C3DC), Butyryl carnitine (C4), Methylmalonyl-/succinyl carnitine (C4DC), 3-OH-iso-/butyryl carnitine (C4OH), Isovaleryl carnitine (C5), Tiglylcarnitine (C5:1), 3-OH-isovalerylcarnitine (C5OH), Glutaryl carnitine (C5DC), Hexanoyl carnitine (C6), Octanoyl carnitine (C8), Octenoyl carnitine (C8:1), Decanoyl carnitine (C10), Decenoyl carnitine (C10:1), Dodecanoyl carnitine (C12), Tetradecanoyl carnitine (C14), Tetradecenoyl carnitine (C14:1), Tetradecadienoyl carnitine (C14:2), 3-OH-tetradecanoylcarnitine (C14OH), Hexadecanoyl carnitine (C16), 3-OH-hexadecanoylcarnitine (C16OH), 3-OH-hexadecenoylcarnitine (C16:1OH), Hexadecenoyl carnitine (C16:1), Octadecanoyl carnitine (C18), Octadecenoyl carnitine (C18:1), 3-OH-octadecanoyl carnitine (C18OH), 3-OH-octadecenoyl carnitine (C18:1OH), Octadecadienoyl carnitine (C18:2).
The original contributions presented in the study are included in the article/Supplementary Material, further inquiries can be directed to the corresponding author/s.
The studies involving human participants were reviewed and approved by Tehran University of Medical Sciences and Endocrine & Metabolism Research Institute. The patients/participants provided their written informed consent to participate in this study.
Study idea, HD, BL and FR; supervision, BL and FR; project administration, HD; data curation, FR; data collection, HD, SD, PR, SH, PK, MN, NR, AD, MY, BA, KK, NN, AK, FB and HAM; methodology, HD, BL and FR; data analyses, HD, SD, SH and FR; visualization, SH; writing—original draft, HD, SD and PR; All authors reviewed, edited, and approved the final manuscript. All authors contributed to the article and approved the submitted version.
The authors declare that the research was conducted in the absence of any commercial or financial relationships that could be construed as a potential conflict of interest.
All claims expressed in this article are solely those of the authors and do not necessarily represent those of their affiliated organizations, or those of the publisher, the editors and the reviewers. Any product that may be evaluated in this article, or claim that may be made by its manufacturer, is not guaranteed or endorsed by the publisher.
The Supplementary Material for this article can be found online at: https://www.frontiersin.org/articles/10.3389/fcvm.2023.1161761/full#supplementary-material.
CVD, cardiovascular disease; ASCVD, atherosclerotic cardiovascular disease; ACC, American college of cardiology; AHA, American heart association; HDL-C, high-density lipoprotein cholesterol; KMO, Kaiser-Meyer-Olkin; PCA, principal component analysis; KEGG, kyoto encyclopedia of genes and genomes; BMI, body mass index; BCAA, branched-chain amino acid; AAA, aromatic amino acid; WC, waist circumference; HC, hip circumference; SBP, systolic blood pressure; DBP, diastolic blood pressure; TG, triglyceride; Non-HDL-C, non-high-density lipoprotein cholesterol; HbA1c, hemoglobin A1c; FPG, fasting plasma glucose; OR, odds ratio.
1. Fu X, Xu J, Zhang R, Yu J. The association between environmental endocrine disruptors and cardiovascular diseases: a systematic review and meta-analysis. Environ Res. (2020) 187:109464. doi: 10.1016/j.envres.2020.109464
2. Münzel T, Hahad O, Sørensen M, Lelieveld J, Duerr GD, Nieuwenhuijsen M, et al. Environmental risk factors and cardiovascular diseases: a comprehensive expert review. Cardiovasc Res. (2022) 118(14):2880–902. doi: 10.1093/cvr/cvab316
3. Piko P, Kosa Z, Sandor J, Adany R. Comparative risk assessment for the development of cardiovascular diseases in the Hungarian general and roma population. Sci Rep. (2021) 11(1):3085. doi: 10.1038/s41598-021-82689-0
4. Sarrafzadegan N, Hassannejad R, Marateb HR, Talaei M, Sadeghi M, Roohafza HR, et al. PARS Risk charts: a 10-year study of risk assessment for cardiovascular diseases in eastern Mediterranean region. PLoS One. (2017) 12(12):e0189389. doi: 10.1371/journal.pone.0189389
5. Cauwenberghs N, Hedman K, Kobayashi Y, Vanassche T, Haddad F, Kuznetsova T. The 2013 ACC/AHA risk score and subclinical cardiac remodeling and dysfunction: complementary in cardiovascular disease prediction. Int J Cardiol. (2019) 297:67–74. doi: 10.1016/j.ijcard.2019.09.061
6. Lloyd-Jones DM, Braun LT, Ndumele CE, Smith Jr SC, Sperling LS, Virani SS, et al. Use of risk assessment tools to guide decision-making in the primary prevention of atherosclerotic cardiovascular disease: a special report from the American heart association and American college of cardiology. Circulation. (2019) 139(25):e1162–e77. doi: 10.1161/CIR.0000000000000638
7. Karmali KN, Goff DC, Ning H, Lloyd-Jones DM. A systematic examination of the 2013 ACC/AHA pooled cohort risk assessment tool for atherosclerotic cardiovascular disease. J Am Coll Cardiol. (2014) 64(10):959–68. doi: 10.1016/j.jacc.2014.06.1186
8. McGarrah RW, Crown SB, Zhang G-F, Shah SH, Newgard CB. Cardiovascular metabolomics. Circ Res. (2018) 122(9):1238–58. doi: 10.1161/CIRCRESAHA.117.311002
9. Kordalewska M, Markuszewski MJ. Metabolomics in cardiovascular diseases. J Pharm Biomed Anal. (2015) 113:121–36. doi: 10.1016/j.jpba.2015.04.021
10. Shah SH, Hauser ER, Bain JR, Muehlbauer MJ, Haynes C, Stevens RD, et al. High heritability of metabolomic profiles in families burdened with premature cardiovascular disease. Mol Syst Biol. (2009) 5(1):258. doi: 10.1038/msb.2009.11
11. Ussher JR, Elmariah S, Gerszten RE, Dyck JR. The emerging role of metabolomics in the diagnosis and prognosis of cardiovascular disease. J Am Coll Cardiol. (2016) 68(25):2850–70. doi: 10.1016/j.jacc.2016.09.972
12. Würtz P, Havulinna AS, Soininen P, Tynkkynen T, Prieto-Merino D, Tillin T, et al. Metabolite profiling and cardiovascular event risk: a prospective study of 3 population-based cohorts. Circulation. (2015) 131(9):774–85. doi: 10.1161/CIRCULATIONAHA.114.013116
13. Shah SH, Bain JR, Muehlbauer MJ, Stevens RD, Crosslin DR, Haynes C, et al. Association of a peripheral blood metabolic profile with coronary artery disease and risk of subsequent cardiovascular events. Circ: Cardiovasc Genet. (2010) 3(2):207–14. doi: 10.1161/CIRCGENETICS.109.852814
14. Djalalinia S, Modirian M, Sheidaei A, Yoosefi M, Zokaiee H, Damirchilu B, et al. Protocol design for large-scale cross-sectional studies of surveillance of risk factors of non-communicable diseases in Iran: STEPs 2016. Arch Iran Med. (2017) 20(9):608–16. doi: 10.34172/aim.2022.99
15. Arjmand B, Dehghanbanadaki H, Yoosefi M, Rezaei N, Mohammadi Fateh S, Ghodssi-Ghassemabadi R, et al. Association of plasma acylcarnitines and amino acids with hypertension: a nationwide metabolomics study. PloS One. (2023) 18(1):e0279835. doi: 10.1371/journal.pone.0279835
16. Goff Jr DJ, Lloyd-Jones DM, Bennett G, Coady S, D'Agostino RB, Gibbons R, et al. 2013 ACC/AHA guideline on the assessment of cardiovascular risk: a report of the American College of Cardiology/American Heart Association Task Force on Practice Guidelines. Circulation. (2014) 129(25 Suppl 2):S49–73. doi: 10.1161/01.cir.0000437741.48606.98
17. Ruiz-Canela M, Toledo E, Clish CB, Hruby A, Liang L, Salas-Salvadó J, et al. Plasma branched-chain amino acids and incident cardiovascular disease in the PREDIMED trial. Clin Chem. (2016) 62(4):582–92. doi: 10.1373/clinchem.2015.251710
18. Hu W, Sun L, Gong Y, Zhou Y, Yang P, Ye Z, et al. Relationship between branched-chain amino acids, metabolic syndrome, and cardiovascular risk profile in a Chinese population: a cross-sectional study. Int J endocrinol. (2016) 2016:8173905. doi: 10.1155/2016/8173905
19. Vaarhorst AA, Verhoeven A, Weller CM, Böhringer S, Göraler S, Meissner A, et al. A metabolomic profile is associated with the risk of incident coronary heart disease. Am Heart J. (2014) 168(1):45–52. e7. doi: 10.1016/j.ahj.2014.01.019
20. Strand E, Pedersen ER, Svingen GFT, Olsen T, Bjørndal B, Karlsson T, et al. Serum acylcarnitines and risk of cardiovascular death and acute myocardial infarction in patients with stable angina pectoris. J Am Heart Assoc. (2017) 6(2):e003620. doi: 10.1161/JAHA.116.003620
21. Deda O, Panteris E, Meikopoulos T, Begou O, Mouskeftara T, Karagiannidis E, et al. Correlation of Serum acylcarnitines with clinical presentation and severity of coronary artery disease. Biomolecules. (2022) 12(3):354. doi: 10.3390/biom12030354
22. Kalim S, Clish CB, Wenger J, Elmariah S, Yeh RW, Deferio JJ, et al. A plasma long-chain acylcarnitine predicts cardiovascular mortality in incident dialysis patients. J Am Heart Assoc. (2013) 2(6):e000542. doi: 10.1161/JAHA.113.000542
23. Wang TJ, Larson MG, Vasan RS, Cheng S, Rhee EP, McCabe E, et al. Metabolite profiles and the risk of developing diabetes. Nat Med. (2011) 17(4):448–53. doi: 10.1038/nm.2307
24. Wang-Sattler R, Yu Z, Herder C, Messias AC, Floegel A, He Y, et al. Novel biomarkers for pre-diabetes identified by metabolomics. Mol Syst Biol. (2012) 8(1):615. doi: 10.1038/msb.2012.43
25. Guasch-Ferré M, Ruiz-Canela M, Li J, Zheng Y, Bulló M, Wang DD, et al. Plasma acylcarnitines and risk of type 2 diabetes in a Mediterranean population at high cardiovascular risk. J Clin Endocrinol Metab. (2018) 104(5):1508–19. doi: 10.1210/jc.2018-01000
26. Storesund S, Svardal A, Lonnebakken M, Strand E, Berge R, Nygard O, et al. The associations of serum acylcarnitines with long term cardiovascular prognosis in patients with non-obstructive coronary artery disease. Atherosclerosis. (2021) 331:e204–e5. doi: 10.1016/j.atherosclerosis.2021.06.627
27. Gao F, Kovalik J-P, Zhao X, Chow VJ, Chew H, Teo LL, et al. Exacerbation of cardiovascular ageing by diabetes mellitus and its associations with acyl-carnitines. Aging. (2021) 13(11):14785–805. doi: 10.18632/aging.203144
28. Magnusson M, Lewis GD, Ericson U, Orho-Melander M, Hedblad B, Engström G, et al. A diabetes-predictive amino acid score and future cardiovascular disease. Eur Heart J. (2012) 34(26):1982–9. doi: 10.1093/eurheartj/ehs424
29. Tillin T, Hughes AD, Wang Q, Würtz P, Ala-Korpela M, Sattar N, et al. Diabetes risk and amino acid profiles: cross-sectional and prospective analyses of ethnicity, amino acids and diabetes in a south Asian and European cohort from the SABRE (southall and brent REvisited) study. Diabetologia. (2015) 58(5):968–79. doi: 10.1007/s00125-015-3517-8
30. Zou Y, Yang Y, Fu X, He X, Liu M, Zong T, et al. The regulatory roles of aminoacyl-tRNA synthetase in cardiovascular disease. Mol Ther Nucleic Acids. (2021) 25:372–87. doi: 10.1016/j.omtn.2021.06.003
31. Walejko JM, Christopher BA, Crown SB, Zhang G-F, Pickar-Oliver A, Yoneshiro T, et al. Branched-chain α-ketoacids are preferentially reaminated and activate protein synthesis in the heart. Nat Commun. (2021) 12(1):1680. doi: 10.1038/s41467-021-21962-2
32. Bhattacharya S, Granger CB, Craig D, Haynes C, Bain J, Stevens RD, et al. Validation of the association between a branched chain amino acid metabolite profile and extremes of coronary artery disease in patients referred for cardiac catheterization. Atherosclerosis. (2014) 232(1):191–6. doi: 10.1016/j.atherosclerosis.2013.10.036
33. Gambardella J, Khondkar W, Morelli MB, Wang X, Santulli G, Trimarco V. Arginine and endothelial function. Biomedicines. (2020) 8(8):277. doi: 10.3390/biomedicines8080277
34. Tam C-CF, Chan Y-H, Wong Y-K, Li Z, Zhu X, Su K-J, et al. Multi-omics signatures link to ticagrelor effects on vascular function in patients with acute coronary syndrome. Arterioscler Thromb Vasc Biol. (2022) 42(6):789–98. doi: 10.1161/ATVBAHA.121.317513
35. Chen W-S, Wang C-H, Cheng C-W, Liu M-H, Chu C-M, Wu H-P, et al. Elevated plasma phenylalanine predicts mortality in critical patients with heart failure. ESC Heart Failure. (2020) 7(5):2884–93. doi: 10.1002/ehf2.12896
36. Czibik G, Mezdari Z, Altintas DM, Bréhat J, Pini M, d’Humières T, et al. Dysregulated phenylalanine catabolism plays a key role in the trajectory of cardiac aging. Circulation. (2021) 144(7):559–74. doi: 10.1161/CIRCULATIONAHA.121.054204
37. Wu G, Fang Y-Z, Yang S, Lupton JR, Turner ND. Glutathione metabolism and its implications for health. J Nutr. (2004) 134(3):489–92. doi: 10.1093/jn/134.3.489
38. Cao TH, Jones DJL, Voors AA, Quinn PA, Sandhu JK, Chan DCS, et al. Plasma proteomic approach in patients with heart failure: insights into pathogenesis of disease progression and potential novel treatment targets. Eur J Heart Fail. (2020) 22(1):70–80. doi: 10.1002/ejhf.1608
39. Wang H, Sun Y, Wang S, Tao Y, Zhang L. Comparisons of the framingham and ASCVD risk scores for coronary heart disease risk prediction in Chinese men. Int J Cardiol. (2018) 266:269. doi: 10.1016/j.ijcard.2018.03.061
Keywords: cardiovascular disease, ASCVD, metabolomics, amino acids, acylcarnitine
Citation: Dehghanbanadaki H, Dodangeh S, Parhizkar Roudsari P, Hosseinkhani S, Khashayar P, Noorchenarboo M, Rezaei N, Dilmaghani-Marand A, Yoosefi M, Arjmand B, Khalagi K, Najjar N, Kakaei A, Bandarian F, Aghaei Meybodi H, Larijani B and Razi F (2023) Metabolomics profile and 10-year atherosclerotic cardiovascular disease (ASCVD) risk score. Front. Cardiovasc. Med. 10:1161761. doi: 10.3389/fcvm.2023.1161761
Received: 8 February 2023; Accepted: 17 April 2023;
Published: 3 May 2023.
Edited by:
Ying Wang, University of British Columbia, CanadaReviewed by:
Joshua Dubland, Provincial Health Services Authority, Canada© 2023 Dehghanbanadaki, Dodangeh, Parhizkar Roudsari, Hosseinkhani, Khashayar, Noorchenarboo, Rezaei, Dilmaghani-Marand, Yoosefi, Arjmand, Khalagi, Najjar, Kakaei, Bandarian, Aghaei Meybodi, Larijani and Razi. This is an open-access article distributed under the terms of the Creative Commons Attribution License (CC BY). The use, distribution or reproduction in other forums is permitted, provided the original author(s) and the copyright owner(s) are credited and that the original publication in this journal is cited, in accordance with accepted academic practice. No use, distribution or reproduction is permitted which does not comply with these terms.
*Correspondence: Farideh Razi Zi1yYXppQHR1bXMuYWMuaXI= Bagher Larijani ZW1yY0B0dW1zLmFjLmly
†These authors have contributed equally to this work
Disclaimer: All claims expressed in this article are solely those of the authors and do not necessarily represent those of their affiliated organizations, or those of the publisher, the editors and the reviewers. Any product that may be evaluated in this article or claim that may be made by its manufacturer is not guaranteed or endorsed by the publisher.
Research integrity at Frontiers
Learn more about the work of our research integrity team to safeguard the quality of each article we publish.