- 1Department of Radiology, Qilu Hospital (Qingdao), Cheeloo College of Medicine, Shandong University, Shandong, China
- 2Graduate School of Dalian Medical University, Dalian Medical University, Dalian, China
- 3Department of Radiology, The Affiliated Changzhou No. 2 People’s Hospital of Nanjing Medical University, Changzhou, China
- 4GE Healthcare, Precision Health Institution, Shanghai, China
Objective: The present study aimed to predict myocardial ischemia in coronary heart disease (CHD) patients based on the radiologic features of coronary computed tomography angiography (CCTA) combined with clinical factors.
Methods: The imaging and clinical data of 110 patients who underwent CCTA scan before DSA or FFR examination in Changzhou Second People’s Hospital, Nanjing Medical University (90 patients), and The First Affiliated Hospital of Soochow University (20 patients) from March 2018 to January 2022 were retrospectively analyzed. According to the digital subtraction angiography (DSA) and fractional flow reserve (FFR) results, all patients were assigned to myocardial ischemia (n = 58) and normal myocardial blood supply (n = 52) groups. All patients were further categorized into training (n = 64) and internal validation (n = 26) sets at a ratio of 7:3, and the patients from second site were used as external validation. Clinical indicators of patients were collected, the left ventricular myocardium were segmented from CCTA images using CQK software, and the radiomics features were extracted using pyradiomics software. Independent prediction models and combined prediction models were established. The predictive performance of the model was assessed by calibration curve analysis, receiver operating characteristic (ROC) curve and decision curve analysis.
Results: The combined model consisted of one important clinical factor and eight selected radiomic features. The area under the ROC curve (AUC) of radiomic model was 0.826 in training set, and 0.744 in the internal validation set. For the combined model, the AUCs were 0.873, 0.810, 0.800 in the training, internal validation, and external validation sets, respectively. The calibration curves demonstrated that the probability of myocardial ischemia predicted by the combined model was in good agreement with the observed values in both training and validation sets. The decision curve was within the threshold range of 0.1–1, and the clinical value of nomogram was higher than that of clinical model.
Conclusion: The radiomic characteristics of CCTA combined with clinical factors have a good clinical value in predicting myocardial ischemia in CHD patients.
1. Introduction
Coronary heart disease (CHD) is a frequent disease that seriously threatens people’s health. Coronary artery disease (CAD) is the main cause of cardiovascular disease death worldwide (1–3). Therefore, early detection and timely interventional treatment are very important for these patients. Patients with CHD mainly present with stable ischemic heart disease. The indication for surgery in these patients is not only the degree of coronary artery stenosis, but also the severity of myocardial ischemia. Therefore, accurate assessment of patients with myocardial ischemia will affect the clinician’s choice of treatment for these patients. Coronary computed tomography Angiography (CCTA) is currently the first choice for screening CHD patients, which is sensitive to the nature of plaque and the degree of stenosis (4, 5), but cannot directly obtain information about myocardial ischemia (6). At present, the gold standard models for the diagnosis of myocardial ischemia include fractional flow reserve (FFR), positron emission tomography (PET), and single-photon emission computed tomography (SPECT) (7). However, these detection methods are invasive and expensive, so they are not commonly applied in the clinic. Therefore, it is important to seek an economical and non-invasive examination method to evaluate myocardial ischemia.
Radiomics is a new quantitative imaging technique that is commonly employed in medical research because of its non-invasive nature and cost-effectiveness. In recent years, many studies have shown that machine learning-based radiomic algorithm can be employed to capture hidden features in imaging to assist clinicians in accurately diagnosing patients and predicting treatment responses (8–10). At present, radiomic analysis can be performed for the diagnosis of cardiomyopathy, myocardial infarction, and arrhythmia (11–13). Some studies have confirmed that radiomics can predict myocardial ischemia, but these experiments are only based on CCTA images and do not combine with other clinical indicators (14–19).
Therefore, the present study aimed to develop a diagnostic model for predicting myocardial ischemia by integrating radiomic features and clinical data.
2. Materials and methods
This retrospective study was approved by the Ethics Committee of Changzhou Second People’s Hospital, Nanjing Medical University. The inclusion criteria for patients were as follows: (i) patients with myocardial ischemia were confirmed by FFR ≤ 0.8 or DSA ≥ 90%; (ii) patients had no history of myocardial infarction; and (iii) CCTA images of patients showed no significant motion artifacts. The exclusion criteria were as follows: (i) the interval between CCTA and FFR or DSA was longer than 1 week; and (ii) patients had a history of other cardiac diseases or coronary artery bypass grafting or coronary stent implantation. Ultimately, 110 patients were enrolled from 2 sites in the study. The process of patient selection is illustrated in Figure 1.
2.1. Patient recruitment
All included patients from site 1 were randomly divided into the training (n = 64) and internal validation (n = 26) sets at a ratio of 7:3. The patients from site 2 were assigned to the external validation set (n = 20). In the training set, 34 patients had myocardial ischemia and the remaining 30 patients had normal myocardial blood supply, as verified by digital subtraction angiography (DSA) and fractional flow reserve (FFR) assessment. In the internal validation set, 14 patients had myocardial ischemia, and the remaining 12 patients had normal myocardial blood supply. In the external validation set, 10 patients had myocardial ischemia, and the remaining 10 patients had normal myocardial blood supply.
2.2. CT image acquisition
The CT scans were performed using a 256-detector CT (GE Revolution CT, GE Healthcare, America), dual source CT (SOMATOM Definition, Siemens Healthineers, Germany), and dual source CT (SOMATOM Force, Siemens Healthineers, Germany). The scan range of cardiac examination was from the thoracic entrance to the diaphragmatic surface of the heart (20). Table 1 shows the scanning parameters used in this study.
2.3. Image segmentation and feature extraction
Two radiologists (R1 and R2) with 5 years of work experience in the cardiovascular group imported the CCTA images into CQK software for automatic myocardial segmentation using a pre-trained V-NET model, the left ventricular myocardium were segmented and then visually checked and manually modified by the radiologists. R1 took 1 week to perform two operations on the images over an interval, and R2 operated only once. Ultimately, one patient’s image corresponded to three processing results. Finally, the extraction of myocardial radiomics features was performed using pyradiomics software (version 3.0.11). Specifically, resampling the image voxel size with 1*1*1, discretizing the CT gray value using binWidth 25.
2.4. Feature selection and model establishment
A total of 1,218 radiomic features were extracted, as shown in Figure 2. Pearson correlation coefficient was used to eliminate redundant radiomic features and select the best feature among the remaining radiomic features. First, radiomic features with ICCs > 0.75 were selected to ensure inter-observer consistency and intra-observer consistency. Secondly, the maximum redundancy minimum correlation (mRMR) and lasso regression algorithms were employed to reduce the dimension of the features. The 10-fold cross-test method was used to screen the optimal hyperparameter λ value of the lasso regression model and construct the radiomics model (Figure 3).
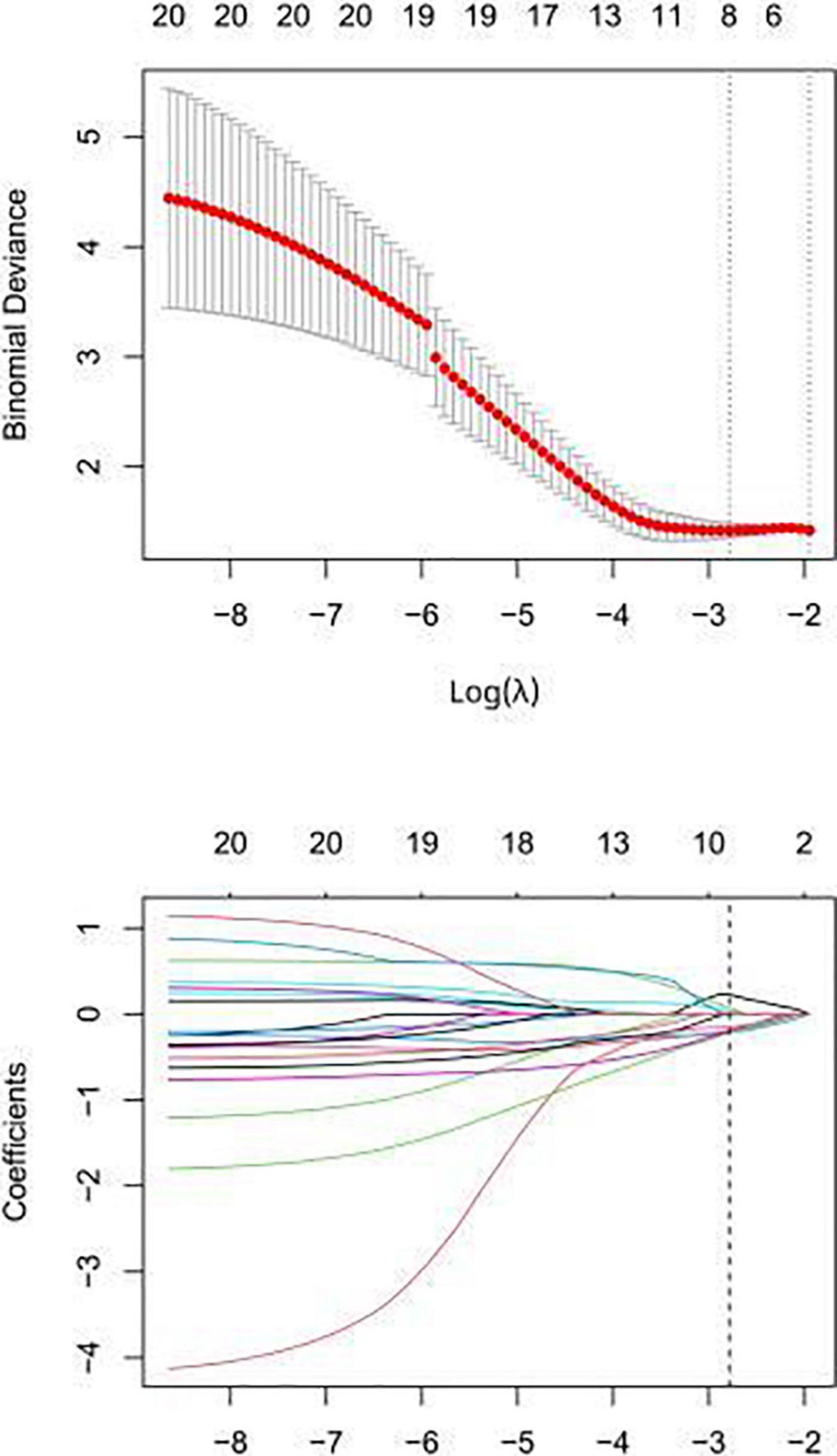
Figure 3. In the lasso regression model, the folding cross test is used to select the optimal value of the hyperparameter λ, and the lowest is the feature that best matches the true result.
Univariate logistic regression analysis was conducted to explore whether there was a significant difference in the characteristics between the two groups. Then, multivariable logistic regression was used to analyze those clinical factors and establish a combined clinical-radiological model and predictive nomogram. Besides, a predictive model was developed based on the selected clinical parameters without radiomics scores.
2.5. Model validation
ROC analyses were performed to evaluate the performance of radiomics model, clinical model and combined model in training and internal validation sets. The agreements between the observed ischemia probabilities and model predictive ischemia probabilities in training and internal validation sets were assessed by the calibration curves. The combined model’s clinical usefulness was studied using decision curve analyses. In order to validate the combined model’s generalization, we also evaluate the combined model in the external validation set.
2.6. Statistical analysis
In this study, SPSS 25.0 and R 4.0.22 were employed for statistical analyses. Measurement data were assessed by Shapiro–Wilk normality, and those with normal distribution were presented as ± S. Statistical differences between the two groups were compared by independent sample t-test. The enumeration data were presented as the number of cases, and the comparison between the two groups was carried out with chi-square test. Univariate and multivariate logistic regression methods were used to select clinical factors and included in the clinical factor prediction model. The differential diagnostic performance of radiomic model and combined diagnostic model was evaluated by ROC curve, and the area under curve (AUC), 95% CI, accuracy, specificity, and sensitivity were calculated. Statistical difference in AUC between pairwise models was compared by DeLong test.
3. Results
3.1. General patient information
Ninety patients were included in this study, including 39 females and 51 males. The general data of the patients and independent clinical factors (multiple logistic regression analysis) are presented in Tables 2, 3.
3.2. Feature selection and radiomic model construction
Feature overfitting was avoided by reducing the dimension of the features. Firstly, among the 322 features extracted from CCTA images, features with ICCs > 0.75 were considered to have good inter-observer consistency and intra-observer consistency. Then, in order to remove the irrelevant and redundant features and screen out the features with good consistency, the mRMR method was used for processing, and the number of features was reduced to 30. Finally, the subset was optimized by Lasso regression analysis (Figure 3). The selected features are displayed in Figure 4. The rad-score was determined as follows:
Radscore = 0.227*log_sigma_4_0_mm_3D_gldm_Small DependenceLowGrayLevelEmphasis − 0.194*wavelet_LLL_first order_ 10 Percentile − 0.212*wavelet_HLL_gldm_LargeDependence Emphasis + 0.035*wavelet_LHH_firstorder_Skewness − 0.197*log_ sigma_5_0_mm_3D_glszm_SmallAreaEmphasis − 0.148*log_sigma_ 4_0_mm_3D_glszm_SmallAreaHighGrayLevelEmphasis − 0.221* original_shape_Elongation + 0.072*wavelet_LHH_gldm_Small DependenceLowGrayLevelEmphasis + 0.138.
The model constructed by the selected eight radiomics features exhibited good discrimination ability, with AUC values of 0.826, 0.744 in the training and internal validation sets, respectively (Figure 5). The accuracy, sensitivity, and specificity of this model are presented in Table 4.
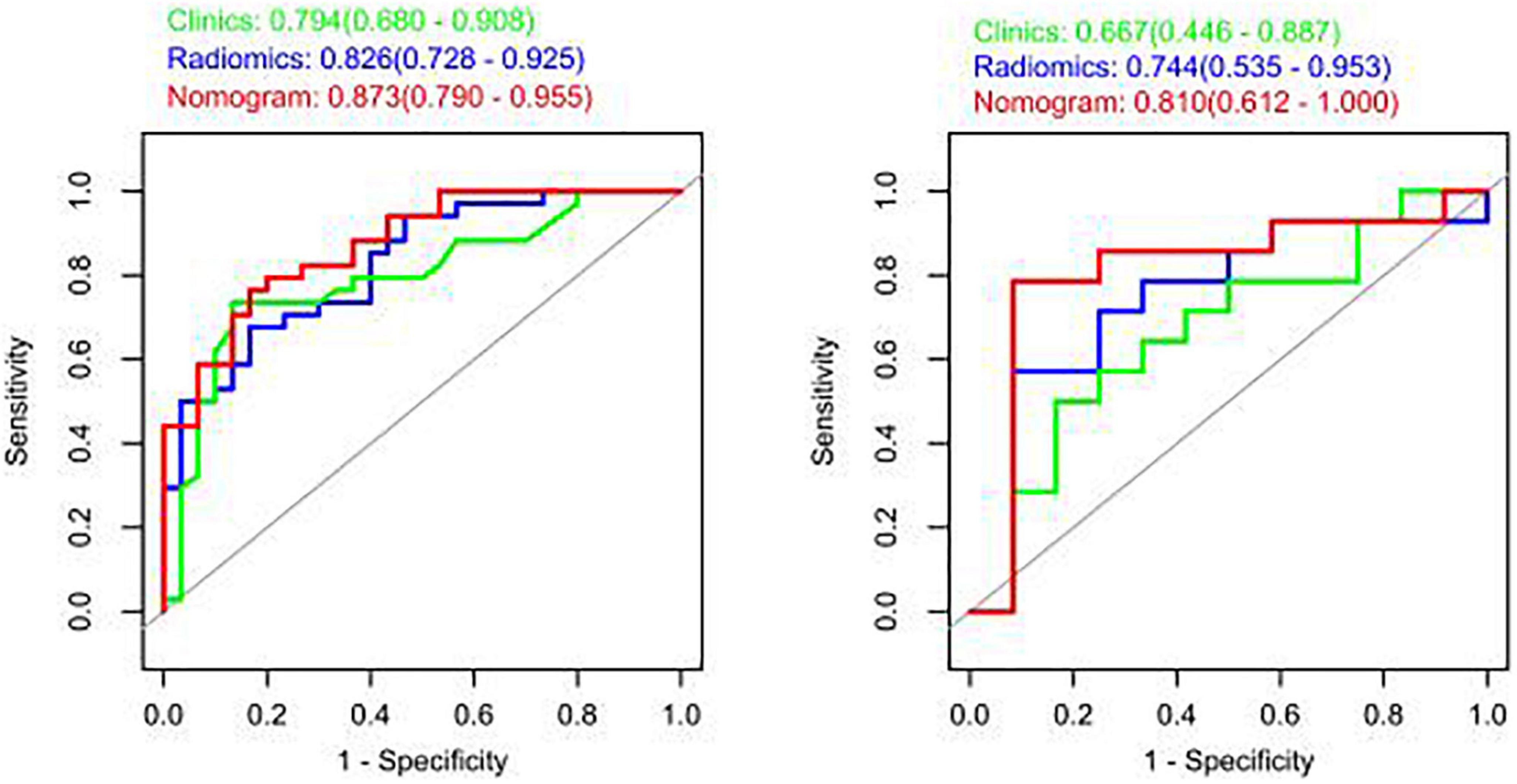
Figure 5. Receiver operating characteristic (ROC) curves of the radiomic, clinical, and integrated models.
3.3. Clinical value of the nomogram
The study showed that the combined model based on CCTA imaging features combined with key clinical factors was significantly superior to the prediction efficiency of the clinical model, and the AUC value was increased from 0.67 to 0.81 (Table 4 and Figure 5). Compared to the clinical model alone, the combination of clinical and radiomic features markedly enhanced the prediction of myocardial ischemia. The Delong test also indicated that the combined nomogram (AUC of 0.810 in the internal validation set) was a better predictor of myocardial ischemia than radiomics alone. A clinical-radiomic nomogram was constructed to visualize the models (Figure 6). The calibration curves of the integrated nomogram for predicting myocardial ischemia showed good agreement in the training and validation sets. Good calibration curves were detected, and the HL test indicated goodness of fit to the data (Figure 7). The nomo-score was calculated as follows:
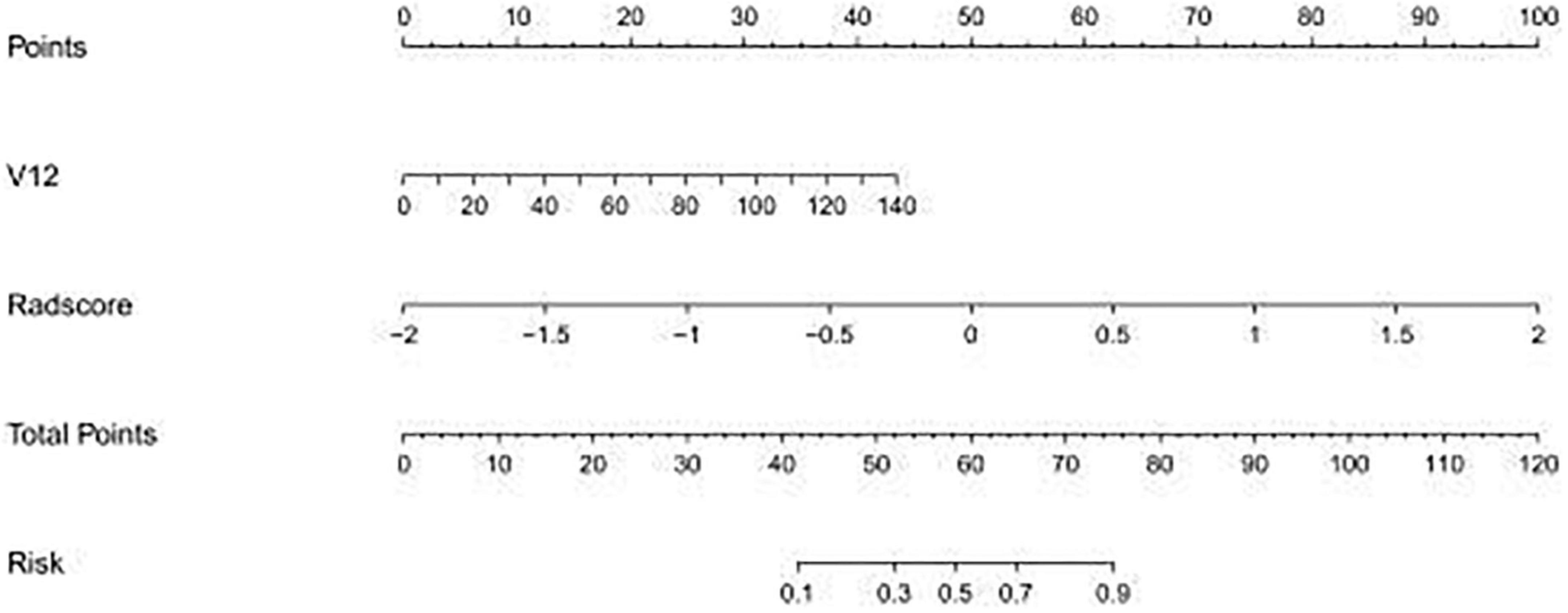
Figure 6. The established clinical-radiomic nomogram for predicting myocardial ischemia. V12, Gensini score.
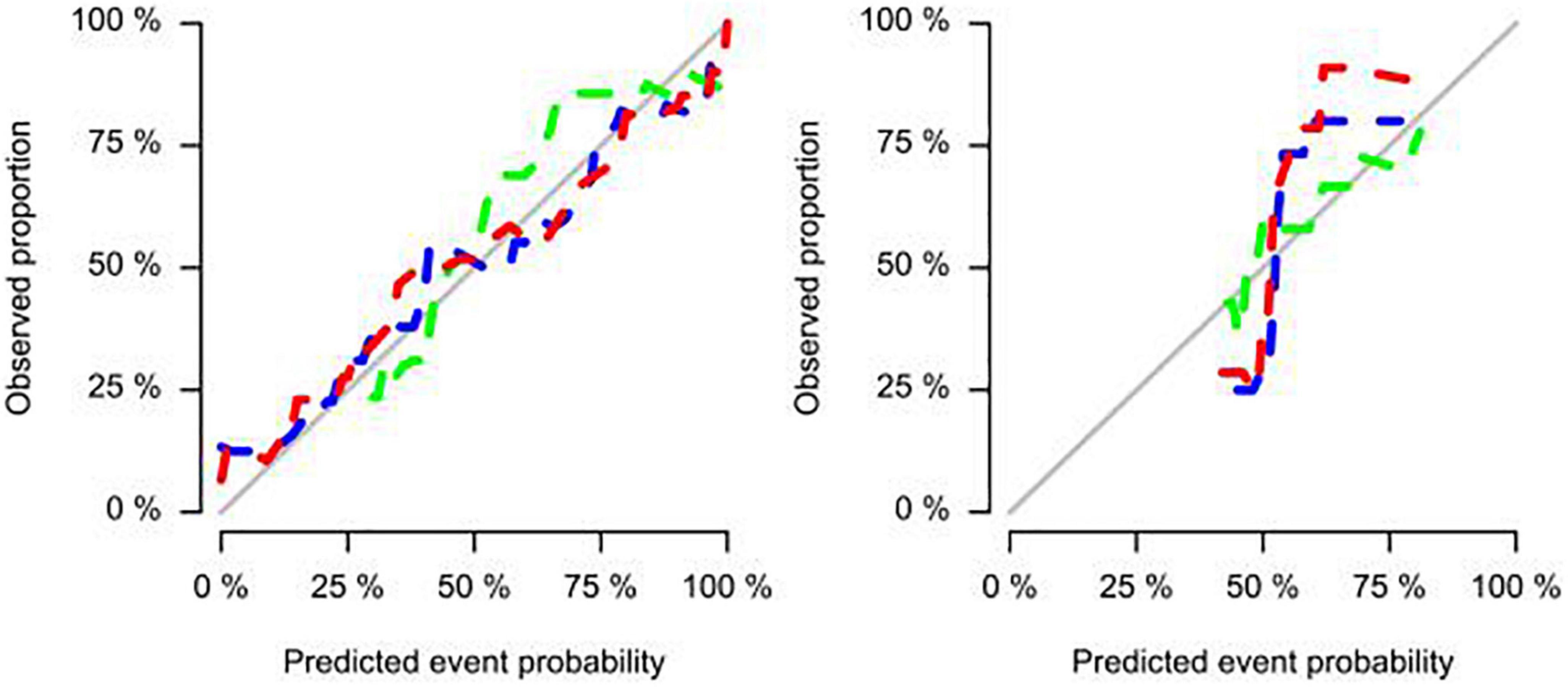
Figure 7. Calibration curves of the integrated nomogram for predicting myocardial ischemia in both training, internal validation, and external validation sets.
By adding radiomic features, the combined clinical-radiomics DCAs had better clinical utility, particularly the clinical DCA, indicating that the nomogram is robust clinical tool for predicting myocardial ischemia. The decision curve was within the threshold range of 0.1–1, and the clinical value of the nomogram was higher than that of the clinical model (Figure 8).
4. Discussion
To detect myocardial ischemia as early as possible, this study systematically analyzed the differences in clinically independent risk factors and radiomic characteristics between the two groups. The results of this study showed that one clinically independent risk factor and six radiomics features were associated with myocardial ischemia. This study compared clinical factors between the two groups, and showed that Gensini score was an independent predictor of myocardial ischemia, which were consistent with previous studies (21–23).
Radiomics is a method of transforming images to generate high-throughput data that can be mined. It has shown excellent performance in predicting the diagnosis, prognosis, staging, and treatment responses of cancer patients (24, 25). In this study, a predictive model was established based on CCTA radiomics. The AUC, sensitivity, specificity, accuracy, positive predictive, and negative predictive values of the model for predicting myocardial ischemia implying that the model has good diagnostic performance. To further enhance the performance of the model, this study combined clinical risk factors and radiomic features to establish a prediction model.
In this study, we developed a combined model incorporating CCTA and clinical variable to detect myocardial ischemia. Our results revealed that the combined DL model contributed to better performance for predicting myocardial ischemia with AUC values of 0.810 and 0.800 in the internal validation and external datasets. Hu and co-workers (26) evaluated 1,409 radiomics features from CTA images from patients with myocardial ischemia and constructed a logistic regression model that demonstrated AUC values of 0.762 and 0.671 for the training and test cohorts, respectively. In another study, Zhao et al. (27) extracted 385 radiomics features from target lesions on CTA images and constructed a logistic regression model, which demonstrated AUCs for predicting myocardial ischemia of 0.835 and 0.717 for the training and test cohorts, respectively. In the present study, the AUC values of the training set and internal validation set were 0.873 and 0.810, respectively, indicating that the prediction performance of our combined model was better than that of the model based only on image images.
As known that, the reproducibility and reliability of radiomics features were affected by the segmentation, as twice segmentation by same operator with different time, and by different operators were hardly exactly same, which caused radiomic features varied largely. But in our study, we used pre-trained VNET myocardium segmentation model to segment, which excluded the subjective deviation, and confirmed the segmentation at different time and with different operator were exactly same. This is a small advantage of this study.
Despite promising findings, our study has some limitations. Firstly, although the study included patients from two centers, the number of patients was still insufficient, only 110 patients were included in the study, which might cause overfitting, more data is needed in further to build a more robust model. However, we used independent internal validation dataset and external validation to validate the model, and found our model performed well in both two dataset, and their AUC values were close to AUC in training dataset, which decreased the overfitting suspicion. Nevertheless, in order to further validate the results of this study, the sample size should be further expanded in future multi-center studies. Secondly, retrospective studies may inevitably lead to selection bias. Finally, CCTA and clinical data alone are not perfect predictors of myocardial ischemia, and more valuable data, such as cardiac magnetic resonance, should be considered.
In conclusion, the diagnostic model based on CCTA radiomic features can predict myocardial ischemia. The combined diagnostic model with clinical risk score has higher clinical value, which provides more accurate diagnosis and treatment of myocardial ischemia.
Data availability statement
The data analyzed in this study is subject to the following licenses/restrictions: With the authorization of the patient or his immediate family and through the decision of the Ethics Committee of Changzhou Second People’s Hospital paid by Nanjing Medical University. Requests to access these datasets should be directed to dHhxMDEwNDAwMDVAMTYzLmNvbQ==.
Author contributions
Y-CY: substantial contributions to conception and design and drafting the manuscript and critical revision for important intellectual content. YD, Z-WW, R-HY, C-JP, S-FD, and X-QT: data acquisition, data analysis, and interpretation. All authors contributed to final approval of the version to be published and agreed to be accountable for all aspects of the work to ensure that questions regarding the accuracy or integrity of the work were appropriately investigate and resolved.
Funding
This work was supported by Jiangsu Medical Association [SYH-3201150-0015(2021010)].
Acknowledgments
The authors would like to express their gratitude to EditSprings (https://www.editsprings.cn) for the expert linguistic services provided.
Conflict of interest
The authors declare that the research was conducted in the absence of any commercial or financial relationships that could be construed as a potential conflict of interest.
Publisher’s note
All claims expressed in this article are solely those of the authors and do not necessarily represent those of their affiliated organizations, or those of the publisher, the editors and the reviewers. Any product that may be evaluated in this article, or claim that may be made by its manufacturer, is not guaranteed or endorsed by the publisher.
Footnotes
References
1. Yu W, Zhang F, Liu B, Wang J, Shao X, Yang M, et al. Incremental value of epicardial fat volume to coronary artery calcium score and traditional risk factors for predicting myocardial ischemia in patients with suspected coronary artery disease. J Nucl Cardiol. (2022) 29:1583–92. doi: 10.1007/s12350-021-02538-5
2. Karolyi, M, Gotschy A, Polacin M, Plein S, Paetsch I, Jahnke C, et al. Diagnostic performance of 3D cardiac magnetic resonance perfusion in elderly patients for the detection of coronary artery disease as compared to fractional flow reserve. Eur Radiol. (2023) 33:339–47. doi: 10.1007/s00330-022-09040-7
3. Shay C, Ning H, Daniels S, Rooks C, Gidding S, Lloyd-Jones D. Status of cardiovascular health in US adolescents: prevalence estimates from the National health and nutrition examination surveys (NHANES) 2005-2010. Circulation. (2013) 127:1369–76. doi: 10.1161/CIRCULATIONAHA.113.001559
4. Budoff M, Li D, Kazerooni E, Thomas G, Mieres J, Shaw L. Diagnostic accuracy of noninvasive 64-row computed tomographic coronary angiography (CCTA) compared with myocardial perfusion imaging (MPI): the PICTURE Study, a prospective multicenter trial. Acad Radiol. (2017) 24:22–9. doi: 10.1016/j.acra.2016.09.008
5. Zhou J, Yang J, Yang X, Chen Z, He B, Du L, et al. Impact of clinical guideline recommendations on the application of coronary computed tomographic angiography in patients with suspected stable coronary artery disease. Chin Med J. (2016) 129:135–41. doi: 10.4103/0366-6999.173434
6. La Grutta L, Toia P, Maffei E, Cademartiri F, Lagalla R, Midiri M. Infarct characterization using CT. Cardiovasc Diagn Ther. (2017) 7:171–88. doi: 10.21037/cdt.2017.03.18
7. Nagel E, Greenwood J, McCann G, Bettencourt N, Shah A, Hussain S, et al. Magnetic resonance perfusion or fractional flow reserve in coronary disease. N Engl J Med. (2019) 380:2418–28. doi: 10.1056/NEJMoa1716734
8. Xue T, Peng H, Chen Q, Li M, Duan S, Feng F. Preoperative prediction of KRAS mutation status in colorectal cancer using a CT-based radiomics nomogram. Br J Radiol. (2022) 95:20211014. doi: 10.1259/bjr.20211014
9. Yang L, Dong D, Fang M, Zhu Y, Zang Y, Liu Z, et al. Can CT-based radiomics signature predict KRAS/NRAS/BRAF mutations in colorectal cancer? Eur Radiol. (2018) 28:2058–67. doi: 10.1007/s00330-017-5146-8
10. Lambin P, Leijenaar R, Deist T, Peerlings J, de Jong E, van Timmeren J, et al. Radiomics: the bridge between medical imaging and personalized medicine. Nat Rev Clin Oncol. (2017) 14:749–62. doi: 10.1038/nrclinonc.2017.141
11. Alis D, Guler A, Yergin M, Asmakutlu O. Assessment of ventricular tachyarrhythmia in patients with hypertrophic cardiomyopathy with machine learning-based texture analysis of late gadolinium enhancement cardiac MRI. Diagn Interv Imaging. (2020) 101:137–46. doi: 10.1016/j.diii.2019.10.005
12. Si N, Shi K, Li N, Dong X, Zhu C, Guo Y, et al. Identification of patients with acute myocardial infarction based on coronary CT angiography: the value of pericoronary adipose tissue radiomics. Eur Radiol. (2022) 32:6868–77. doi: 10.1007/s00330-022-08812-5
13. Rodríguez-de-Vera J, Bernabé G, García J, Saura D, González-Carrillo J. Left ventricular non-compaction cardiomyopathy automatic diagnosis using a deep learning approach. Compute Methods Programs Biomed. (2022) 214:106548. doi: 10.1016/j.cmpb.2021.106548
14. Shu Z, Cui S, Zhang Y, Xu Y, Hung S, Fu L, et al. Predicting chronic myocardial ischemia using CCTA-based radiomics machine learning nomogram. J Nucl Cardiol. (2022) 29:262–74. doi: 10.1007/s12350-020-02204-2
15. Baessler B, Mannil M, Maintz D, Alkadhi H, Manka R. Texture analysis and machine learning of non-contrast T1-weighted MR images in patients with hypertrophic cardiomyopathy-preliminary results. Eur J Radiol. (2018) 102:61–7. doi: 10.1016/j.ejrad.2018.03.013
16. Baessler B, Luecke C, Lurz J, Klingel K, von Roeder M, de Waha S, et al. Cardiac MRI texture analysis of T1 and T2 maps in patients with infarctlike acute myocarditis. Radiology. (2018) 289:357–65. doi: 10.1148/radiol.2018180411
17. Amano Y, Suzuki Y, Yanagisawa F, Omori Y, Matsumoto N. Relationship between extension or texture features of late gadolinium enhancement and ventricular tachyarrhythmias in hypertrophic cardiomyopathy. Biomed Res Int. (2018) 2018:4092469.
18. Larroza A, Materka A, Lopez-Lereu M, Monmeneu J, Bodi V, Moratal D. Differentiation between acute and chronic myocardial infarction by means of texture analysis of late gadolinium enhancement and cine cardiac magnetic resonance imaging. Eur J Radiol. (2017) 92:78–83. doi: 10.1016/j.ejrad.2017.04.024
19. Schofield R, Ganeshan B, Kozor R, Nasis A, Endozo R, Groves A, et al. CMR myocardial texture analysis tracks different etiologies of left ventricular hypertrophy. J Cardiovasc Magn Reson. (2016) 18:O82. doi: 10.1186/1532-429X-18-S1-O82
20. Kolossvary M, Kellermayer M, Merkely B, Maurovich-Horvat P. Cardiac computed tomography radiomics: a comprehensive review on radiomic techniques. J Thorac Imaging. (2018) 33:26–34.
21. Dong M, Li C, Yang G, Gou Q, Zhao Q, Liu Y, et al. Diagnostic performance of coronary computed tomography angiography-derived fractional flow reverse in lesion-specific ischemia patients with different Gensini score levels. Ann Transl Med. (2022) 10:412. doi: 10.21037/atm-22-881
22. Wang K, Zheng Y, Wu T, Ma Y, Xie X. Predictive value of gensini score in the long-term outcomes of patients with coronary artery disease who underwent PCI. Front Cardiovasc Med. (2021) 8:778615. doi: 10.3389/fcvm.2021.778615
23. Qin Y, Yan G, Qiao Y, Ma C, Liu J, Tang C. Relationship between random blood glucose, fasting blood glucose, and gensini score in patients with acute myocardial infarction. Biomed Res Int. (2019) 2019:9707513. doi: 10.1155/2019/9707513
24. Yip S, Aerts H. Applications and limitations of radiomics. Phys Med Biol. (2016) 61:R150–66. doi: 10.1088/0031-9155/61/13/R150
25. Sundström M, Edlund K, Lindell M, Glimelius B, Birgisson H, Micke P, et al. KRAS analysis in colorectal carcinoma: analytical aspects of Pyrosequencing and allele-specific PCR in clinical practice. BMC Cancer. (2010) 10:660. doi: 10.1186/1471-2407-10-660
26. Hu W, Wu X, Dong D, Cui L, Jiang M, Zhang J, et al. Novel radiomics features from CCTA images for the functional evaluation of significant ischaemic lesions based on the coronary fractional flow reserve score. Int J Cardiovasc Imaging. (2020) 36:2039–50. doi: 10.1007/s10554-020-01896-4
Keywords: X-ray computed, diagnosis, differential, radiomics, myocardial ischemia
Citation: Yang Y-C, Dou Y, Wang Z-W, Yin R-H, Pan C-J, Duan S-F and Tang X-Q (2023) Prediction of myocardial ischemia in coronary heart disease patients using a CCTA–Based radiomic nomogram. Front. Cardiovasc. Med. 10:1024773. doi: 10.3389/fcvm.2023.1024773
Received: 22 August 2022; Accepted: 03 January 2023;
Published: 19 January 2023.
Edited by:
Filippo Cademartiri, Gabriele Monasterio Tuscany Foundation (CNR), ItalyReviewed by:
Jennifer Mancio, University of Porto, PortugalDavid Jean Winkel, University Hospital of Basel, Switzerland
Copyright © 2023 Yang, Dou, Wang, Yin, Pan, Duan and Tang. This is an open-access article distributed under the terms of the Creative Commons Attribution License (CC BY). The use, distribution or reproduction in other forums is permitted, provided the original author(s) and the copyright owner(s) are credited and that the original publication in this journal is cited, in accordance with accepted academic practice. No use, distribution or reproduction is permitted which does not comply with these terms.
*Correspondence: Xiao-Qiang Tang, dHhxMDEwNDAwMDVAMTYzLmNvbQ==