- 1Department of Cardiology, The First Affiliated Hospital of Kunming Medical University, Kunming, China
- 2Department of Emergency, The First Affiliated Hospital of Kunming Medical University, Kunming, China
Abstract: Septic cardiomyopathy (SCM) is a serious complication caused by sepsis that will further exacerbate the patient's prognosis. However, immune-related genes (IRGs) and their molecular mechanism during septic cardiomyopathy are largely unknown. Therefore, our study aims to explore the immune-related hub genes (IRHGs) and immune-related miRNA-mRNA pairs with potential biological regulation in SCM by means of bioinformatics analysis and experimental validation.
Method: Firstly, screen differentially expressed mRNAs (DE-mRNAs) from the dataset GSE79962, and construct a PPI network of DE-mRNAs. Secondly, the hub genes of SCM were identified from the PPI network and the hub genes were overlapped with immune cell marker genes (ICMGs) to further obtain IRHGs in SCM. In addition, receiver operating characteristic (ROC) curve analysis was also performed in this process to determine the disease diagnostic capability of IRHGs. Finally, the crucial miRNA-IRHG regulatory network of IRHGs was predicted and constructed by bioinformatic methods. Real-time quantitative reverse transcription-PCR (qRT-PCR) and dataset GSE72380 were used to validate the expression of the key miRNA-IRHG axis.
Result: The results of immune infiltration showed that neutrophils, Th17 cells, Tfh cells, and central memory cells in SCM had more infiltration than the control group; A total of 2 IRHGs were obtained by crossing the hub gene with the ICMGs, and the IRHGs were validated by dataset and qRT-PCR. Ultimately, we obtained the IRHG in SCM: THBS1. The ROC curve results of THBS1 showed that the area under the curve (AUC) was 0.909. Finally, the miR-222-3p/THBS1 axis regulatory network was constructed.
Conclusion: In summary, we propose that THBS1 may be a key IRHG, and can serve as a biomarker for the diagnosis of SCM; in addition, the immune-related regulatory network miR-222-3p/THBS1 may be involved in the regulation of the pathogenesis of SCM and may serve as a promising candidate for SCM therapy.
Introduction
Septic cardiomyopathy (SCM) is septic myocardial damage caused by severe sepsis and is an important cause of high mortality in non-cardiac intensive care units (1). The current general understanding of SCM is acute cardiac dysfunction caused by a dysregulated host response to infection (2). In addition, the presence of SCM in sepsis patients will induce/aggravate circulatory dysfunction and further deteriorate peripheral organ function, resulting in the mortality rate of patients up to 70% (3). Therefore, in-depth research on septic cardiomyopathy is very necessary.
Cardiomyocyte injury and myocardial contractile dysfunction are the main pathophysiological processes in SCM. The current clinical diagnosis of SCM can only rely on myocardial injury factors and echocardiography, which limits early intervention in SCM patients (4–8). Therefore, it is crucial to select appropriate predictive biomarkers for early intervention in SCM.
MicroRNAs (miRNAs) are a class of transcripts without protein-coding functions and play a regulatory role in inflammation (9), growth and development, and biological metabolism in eukaryotes (10). They can regulate gene expression by promoting messenger RNA (mRNA) degradation or inhibiting the mRNA post-transcriptional process (11, 12). With the development of high-throughput gene expression profiling technology, microarray analysis has been widely used to monitor the abnormal expression of genes or miRNAs in diseases (13). More importantly, circulating miRNAs are proposed as biomarkers for disease diagnosis and monitoring in inflammatory heart disease and sepsis-induced cardiac insufficiency (14, 15).
In SCM, abnormal/excessive immune responses are also considered to be one of the important causes of cardiomyocyte dysfunction. Previous studies have shown that interleukin-1β (IL-1β), activated by NLRP3 inflammasome, impairs cardiac function and causes cardiogenic atrophy and diastolic dysfunction in patients with sepsis (16). Activation of the cholinergic anti-inflammatory pathway (CAP) central alpha7 nicotinic acetylcholine receptor (alpha7nAChR) reverses immunosuppression and attenuates multiorgan dysfunction in T lymphocytes from septic rats (17). This suggests that immune response is an important process in myocardial injury in SCM. However, the exact mechanisms of the immune response and IRGs in SCM myocardial injury remain unclear.
Therefore, to determine the role of immune responses in SCM, we obtained DE-mRNAs in datasets GSE79962 by bioinformatics analysis. The ImmucellAI tool was used to analyze the differences in immune cell infiltration between the SCM and control samples of the dataset GSE79962. Subsequently, GO/KEGG enrichment analysis was performed on the DE-mRNAs. Based on the STRING online database, we constructed a protein-protein interaction (PPI) network for DE-mRNA and used Cytoscape-MCODE and CytoHubba plugins to identify significant clusters and central genes in the PPI. Then, we overlapped the hub genes with immune cell marker genes (ICMGs) to obtain immune-related hub genes (IRHGs) during SCM. qRT-PCR and GEO datasets were used to validate the expression of IRHGs. Not only that, after screening the target IRHGs, we predicted the upstream miRNAs of IRHGs from the relevant database. After dataset validation, the key immune-related miRNA-IRHG pair was constructed. We summarize the overall workflow of this study and present it in Figure 1.
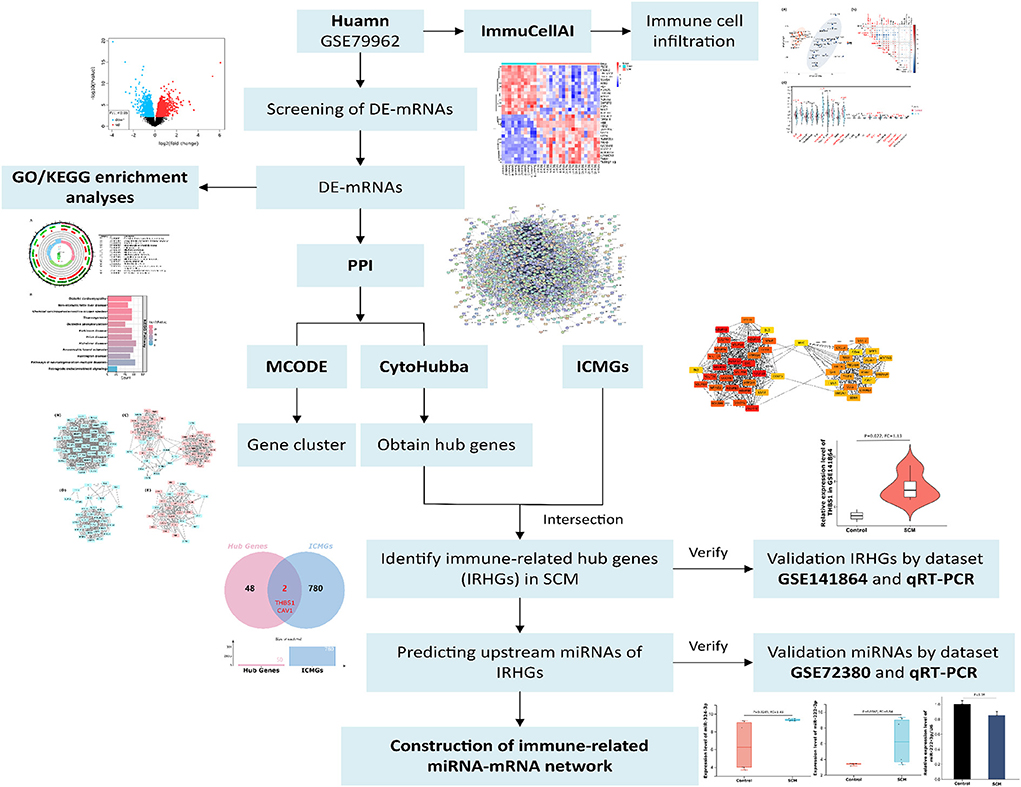
Figure 1. The overall workflow of this study. De-mRNAs: Differentially expressed mRNAs; GO: Gene Ontology; KEGG: Kyoto Encyclopedia of Genes and Genomes; ICMGs: immune cell marker genes.
Materials and methods
Expression profile of mRNA and miRNA in microarray data from human and mice with septic cardiomyopathy
Three human and mice septic cardiomyopathy datasets (GSE79962, GSE141864 and GSE72380) were obtained from the Gene Expression Omnibus (GEO) (https://www.ncbi.nlm.nih.gov/geo/) database. Datasets GSE79962 and GSE141864 analyzed mRNA transcript changes in cardiac tissue from patients with septic cardiomyopathy, and dataset GSE72380 collected cardiac tissue from septic mice for miRNA microarray sequencing. The detailed data of these SCM datasets are presented in Table 1. Therefore, we used these 3 datasets to screen and identify hub genes and hub gene-related miRNA-mRNA regulatory networks. The overall flow chart of this study is shown in Figure 1.
Immune cell infiltrate analyses
ssGSEA enrichment analysis is a method for assessing immune cell infiltration in samples in a dataset based on the expression levels of immune cell-specific marker genes. ImmuCellAI (ImmuCellAI, https://bioinfo.life.hust.edu.cn/web/ImmuCellAI/) (18) is an online tool that uses ssGSEA enrichment analysis to investigate immune cell infiltration in expression matrices. Upload the dataset's gene expression matrix to ImmuCellAI and compare it to the defined immune cell-related gene expression matrix, evaluate the quantity of 24 various types of immune cells in the samples, and compare immune cell infiltration in different samples. Then cluster analysis of immune cell infiltration matrix data, and draw a two-dimensional PCA cluster diagram. The correlation heat map shows the correlation of genes in 24 types of immune cell infiltration, and the violin plot shows the difference in immune cell infiltration.
Differentially expressed transcript analysis
The GEO2R (http://www.ncbi.nlm.nih.gov/geo/geo2r/) tool is an R-based web application for identifying differentially expressed mRNAs (DE-mRNAs) and miRNAs (DE-miRNAs) between SCM and control groups. A p-value < 0.05 and an absolute fold-change (|FC|) > 2.5 as the threshold for screening DE-mRNAs and DE-miRNAs. We use a volcano plot to show the overall expression and distribution of genes in the dataset GSE79962, and a heatmap to show the intergroup expression of the top 30 DE-mRNAs.
Functional enrichment analyses
To understand the characteristic biological properties of DE-mRNAs, the Metascape bioinformatics resource (Metascape, a gene annotation and analysis resource, http://metascape.org, version 3.5) (19) was used for functional enrichment analysis. A minimum overlap value of 3, p-value cutoff of 0.05 and minimum enrichment of 1.5 were set as thresholds. The DE-mRNAs screened in dataset GSE79962 were imported into Metascape to evaluate enriched biological process (BP), molecular function (MF) and cellular component (CC), and Kyoto Encyclopedia of Genes and Genomes (KEGG) pathways, and used circle diagram and histograms visualize the enrichment results for GO and KEGG.
Protein interaction analysis and acquisition of key gene clusters
Protein-protein interaction (PPI) networks have been generated from the STRING database (https://string-db.org/) (20). A rating of 0.4 (medium confidence) used to be set as a threshold. The number of nodes and edges of the PPI network is also obtained from STRING. Next, in order to obtain the gene clusters in the PPI network, the Cytoscape-Minimal Common Oncology Data Elements (MCODE) was used to identify significant gene clusters and obtain cluster scores (filter criteria: degree cut-off = 2; node score cut-off = 0.2; k-core = 2; max depth = 100) and visualize it in Cytoscape. The method of using MCODE to identify key genes of the network is recognized, and scholars have used this method to conduct related research (21).
Acquisition of SCM hub genes and SCM immune-related hub genes, and determination of target genes
CytoHubba is a plugin that can measure the importance of nodes in the network based on network characteristics. The Maximal Clique Centrality (MCC) algorithm was used in CytoHubba to filter the top 50 genes of the PPI network (22). Then, to further elucidate whether these 50 hub genes have immunomodulatory roles, we obtained 782 immune cell marker genes (ICMGs) from published articles (23), and crossed 50 hub genes with ICMGs to obtain hub genes with immunomodulatory effects in SCM, namely immune-related hub genes (IRHGs). IRHGs was further validated using dataset GSE141864 to identify target genes. This analysis of immune-related genes associated with diseases is practical and has been used by scholars for related studies. It is worth emphasizing that this analysis of immune-related genes associated with diseases is practical and has been used by scholars for related studies (24).
SCM sample collection and real-time quantitative reverse transcription-polymerase chain reaction analysis
Peripheral blood mononuclear cells (PBMCs) samples of patients with septic cardiomyopathy were collected from the Department of Emergency Medicine of the First Affiliated Hospital of Kunming Medical University, Yunnan Province, and medical information was collected by the Declaration of Helsinki after informed consent of the patients. This study was approved by the Ethics Committee of the First Affiliated Hospital of Kunming Medical University, Yunnan Province [(2022), ethical review L, No.23]. Male patients with a definite history of sepsis and a reduced left ventricular ejection fraction (LVEF) <0.5 and a confirmed diagnosis of septic cardiomyopathy were included in the study. Exclusion criteria were patients with: (i) a combination of severe arrhythmias; (ii) a combination of significant organ failure, including liver and kidney; and (iii) the presence of possible myocardial suppression disease. Three healthy males matched to the age of the SCM patients served as controls. Total RNA was isolated using TRIzol reagent (Invitrogen) followed by amplification grade (Invitrogen) DNase 1 for further processing. SuperScript™ III First-Strand Synthesis SuperMix (Cat. No. 11752050; Thermo Fisher Scientific Inc.) for reverse transcription of target genes. Target gene determination was performed using the StepOnePlus™ Real-Time PCR System (Cat. No. 4376600; Thermo Fisher Scientific Inc.) with GAPDH as an internal reference. To quantify miRNA expression, we used an optimized qRT-PCR assay based on SYBR green. Data were analyzed using the 2−ΔΔCt method, with U6 (NR_004394.1) as an internal control for target genes, respectively. The sequences and other characteristics of the primers used in the qRT-PCR analysis are shown in Table 3.
Diagnostic analysis of target genes in SCM
The receiver operating characteristic (ROC) curve was obtained by a “rating” method and a mathematical prediction method based on patient characteristics while meeting statistical calculation criteria. ROC curves were generated by analyzing the expression of markers with 1-specificity as the horizontal coordinate and sensitivity as the vertical coordinate. The area under the curve (AUC) of the ROC curve shows the probability that a randomly selected diseased subject will be (correctly) rated or ranked compared to a randomly selected non-diseased subject. In clinical applications, ROC curves are commonly used to assess the ability of biomarkers to distinguish between patients and non-patients with a given disease. Therefore, to elucidate the diagnostic value of target genes in SCM, ROC curve analysis was used in this study. AUC > 0.5 indicates that the marker has the ability to discriminate between healthy controls and patients with SCM (25, 26).
Construction of the miRNA-MRNA regulatory network
miRDB (http://mirdb.org) (27), TargetScan (https://www.targetscan.org/vert_80/), StarBase (http://starbase.sysu.edu.cn/) (28) and RNA22 (https://cm.jefferson.edu/rna22/) are four online public databases that collect relevant miRNA-mRNA interaction information. For miRNA target gene prediction, we used miRDB, TargetScan, and StarBase. Then, the miRNA prediction results of the three databases were intersected and further verified in the dataset GSE72380 to obtain the target miRNA. RNA22 was used to reverse predict the target genes of miRNAs for validation of the results. The expression levels of target miRNAs in peripheral blood mononuclear cells of SCM patients were detected by qRT-PCR. Violin plots were used to visualize miRNA expression.
Statistical analysis of microarray data
The Gene Expression Omnibus (GEO, http://www.ncbi.nlm.nih.gov/geo/) - GEO2R (http://www.ncbi.nlm.nih.gov/geo/geo2r/) was used to identify DEGs in this study. GEO2R is an online application based on the R language. After the user defines the group, GEO2R directly reads the original series matrix file and platform annotation file uploaded by the submitter and draws the boxplot and gene table (including P-values, t-statistics and fold changes, and gene annotations including gene symbols, gene names, gene ontology (GO) entries and chromosome locations, etc.) of the expression value distribution of the selected samples through the “boxplot” and “limma” R language scripts. P < 0.05, FC > 2.5 or FC < 0.4 was set as the threshold for screening differentially expressed genes (29).
Result
The landscape of immune cell infiltration in septic cardiomyopathy
PCA cluster analysis is a method for examining the consistency of biological repetition as well as group differences. PCA cluster analysis results of immune cell infiltration showed that gene expression levels in SCM samples and control samples were significantly different (Figure 2A). The correlation heatmap of 24 immune cells showed a significant positive correlation between CD8+ T and cytotoxic cells, T helper type 17 (Th17) cells and T follicular helper (Tfh) cells, B cells (B-cells) and gamma delta T cells and exhausted cells, exhausted cells and CD8+ T and cytotoxic cells. Significant negative correlations were found between neutrophils and B cells and depleting and cytotoxic cells, Th17 and B-cells, Tfh and B-cells (Figure 2B). The violin plot of the immune cell infiltration difference showed that, compared with the control sample, neutrophil cells, Th17 cells, Tfh cells and central-memory cells infiltrated more and exhausted cells, B-cells, gamma delta T cells, cytotoxic cells, Th2 cells, CD8+ T cells and DC infiltrated less (Figure 2C).
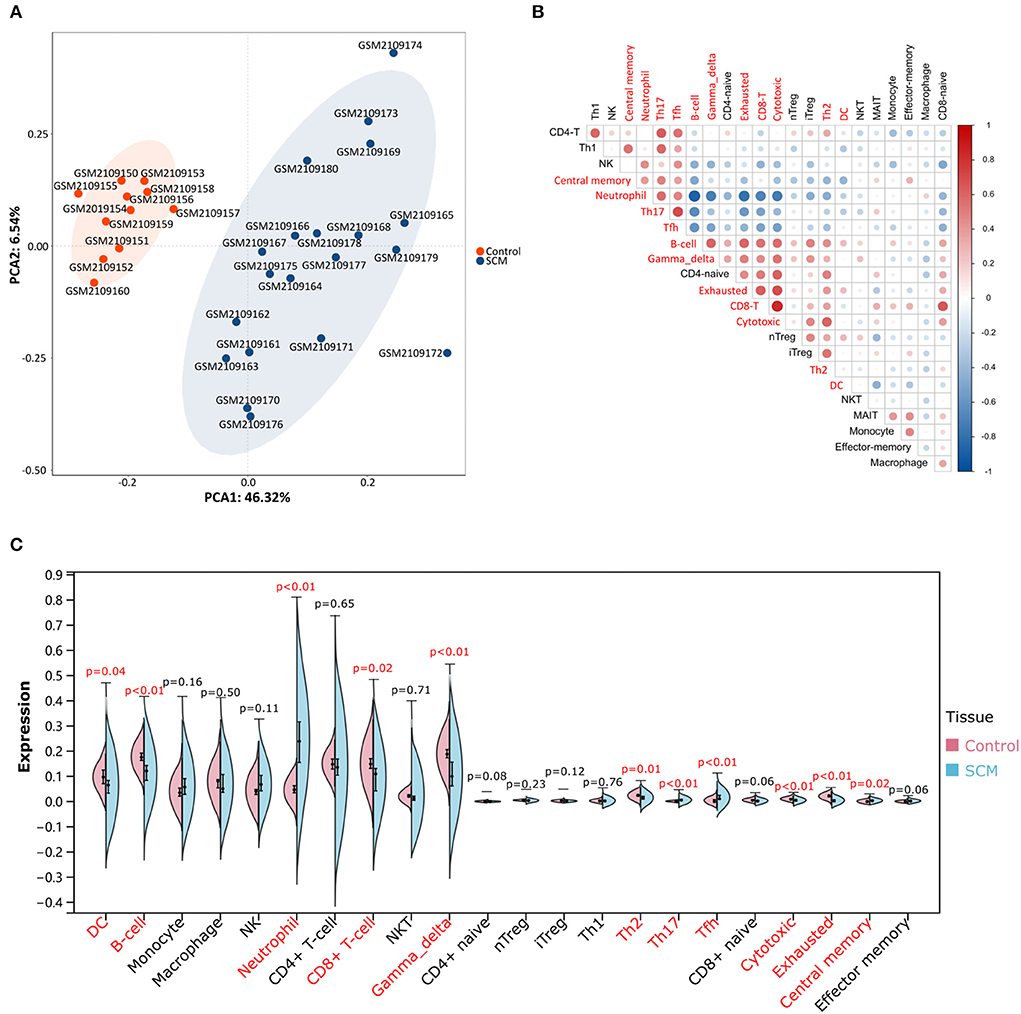
Figure 2. Evaluation and visualization of immune cell infiltration. (A) PCA cluster plot of immune cell infiltration between SCM samples and control samples. (B) Correlation heatmap of 24 immune cells. The size of the circle represents the strength of the correlation: the darker the color, the stronger the correlation; the color represents the correlation: blue represents a positive correlation, and blue represents a negative correlation. Markers in red font represent differences in the infiltration of this cell type between the two groups. (C) Violin plot of the proportion of 24 immune cells. Markers in red font indicate differences in immune infiltration of this cell type between the SCM group and the control group.
Identification of DE-mRNAs in cardiac tissue of patients with SCM
A total of three datasets were used in this paper, and specific information about the datasets, including GEO accession number, experiment type, species, source tissue, number of samples from SCM and controls, and dataset functions are summarized in Table 1. A total of 34 SCM samples and 19 control samples from these three datasets were employed in this paper. There are 51 samples in the GSE79962 dataset, from which we extracted 20 cardiac mRNA array expression profiles of patients with septic cardiomyopathy and 11 cardiac mRNA array expression profiles of controls for analysis. The volcano plot depicts the expression distribution of all the genes in GSE79962 (Figure 3A). A total of 1592 DE-mRNAs (788 up-regulated and 804 down-regulated) were identified in GSE79962. The top 30 DE-mRNAs with the most significant differential changes (top 15 up-regulated and top 15 down-regulated) were then chosen for analysis and heatmap visualization of their inter-group expression differences (Figure 3B). GSE141864 and GSE72380 have 259 DE-mRNAs and 34 DE-miRNAs, respectively.
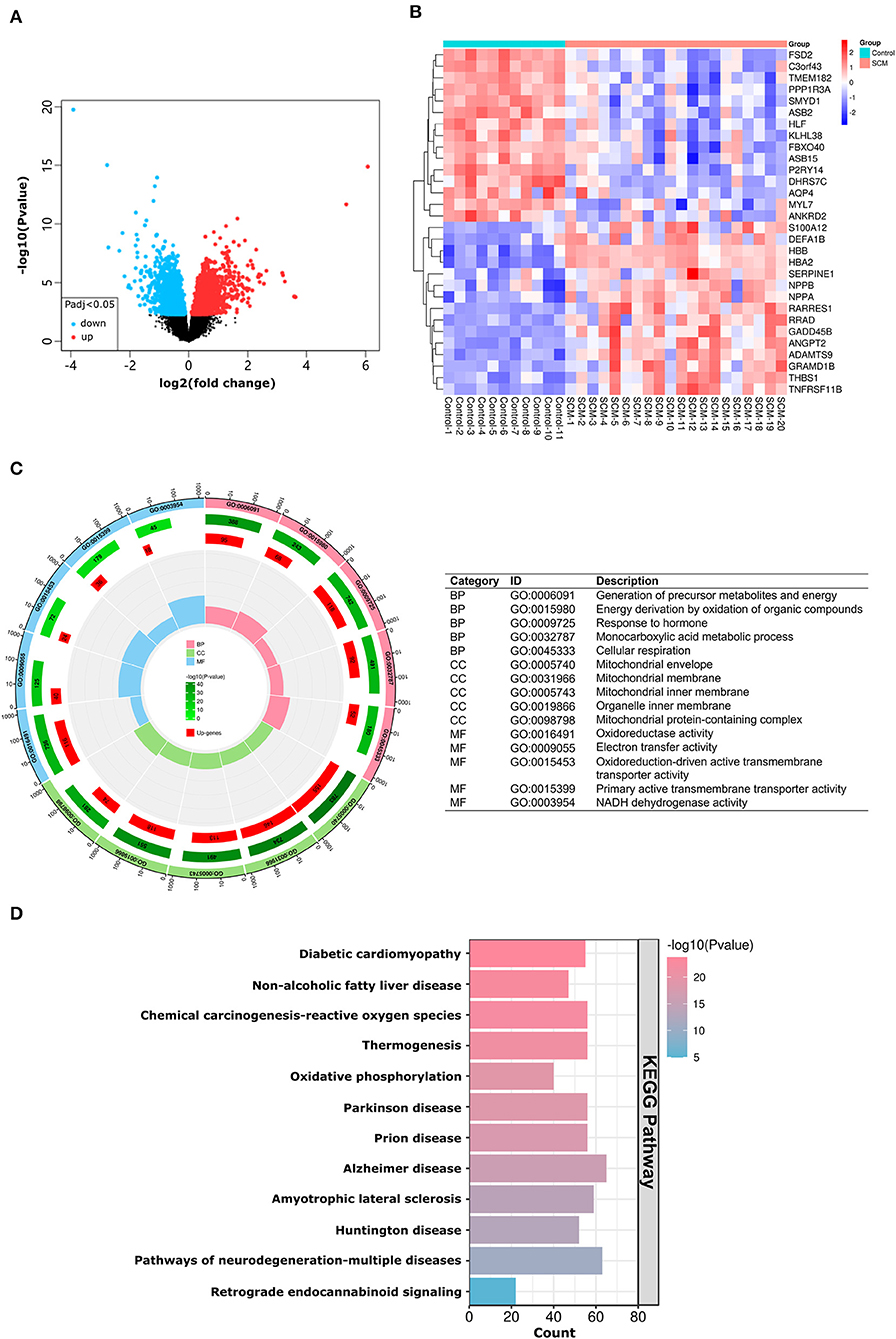
Figure 3. mRNA expression and DEGs enrichment analysis of dataset GSE79962. (A) Volcano plots corresponding to mRNA expression profiles in human hearts in the GSE79962 dataset. Red plots represent up-regulated mRNAs, black plots represent insignificant mRNAs, and blue plots represent down-regulated mRNAs. (B) Heatmap corresponding to the expression profile of the top 30 DE-mRNAs in human hearts in the GSE79962 dataset as determined by p-value. Red rectangles represent high expression and blue rectangles represent a low expression. (C) Circle plot showing the top 5 GO-enriched terms in BP, CC and MF. The most important BPs are involved in generation of precursor metabolites and energy, energy derivation by oxidation of organic compounds, and response to hormone; CC is involved in mitochondrial envelope, mitochondrial membrane and mitochondrial inner membrane; MF is involved in oxidoreductase activity, electron transfer activity, and oxidoreduction-driven active transmembrane transporter activity. (D) Histogram showing the most abundant KEGG pathway for DE-mRNA. The most important KEGG pathways are involved in diabetic cardiomyopathy, non-alcoholic fatty liver disease and chemical carcinogenesis-reactive oxygen species pathway. DE-mRNAs: Differentially expressed mRNAs; GO: Gene Ontology; BP: Biological Process; CC: Cellular Components; MF: Molecular Function; KEGG: Kyoto Encyclopedia of Genes and Genomes; The screening criteria for significantly enriched biological processes and pathways were Q < 0.05. Q-values are adjusted p-values.
Enrichment analysis of DE-mRNAs
According to the results of the GO analysis, these DE-mRNAs were linked to a variety of biological processes, molecular functions, and cellular components. The top three biological processes in which DE-mRNAs were involved were: generation of precursor metabolites and energy, energy derivation by oxidation of organic compounds, and response to hormone; the top three significant cellular components in which the DE-mRNAs participated were: mitochondrial envelope, mitochondrial membrane and mitochondrial inner membrane; the top three significant molecular functions related to those DE-mRNAs were: oxidoreductase activity, electron transfer activity, and oxidoreduction-driven active transmembrane transporter activity (Figure 3C). KEGG pathway analysis showed those DE-mRNAs to be significantly enriched in diabetic cardiomyopathy, non-alcoholic fatty liver disease and chemical carcinogenesis-reactive oxygen species pathway (Figure 3D). The results of GO/KEGG analysis of DE-mRNAs from Metascape online resources are shown in Supplementary Table S1.
PPI network analysis and MCODE cluster modules
The STRING online tool enables reciprocal analysis of differentially expressed genes and derives a PPI network containing network features and node information. The interaction network between proteins coded by DE-mRNAs, which was comprised of 1591 nodes and 12507 edges, was constructed by STRING and visualized (Figure 4A). The MCODE plugin was used to identify gene cluster modules. In this network, we identified four modules based on the filtering conditions, as shown in Figure 4. Cluster 1 had the highest cluster score (score: 36.811, 38 nodes and 681 edges) (Figure 4B), followed by cluster 2 (score: 16.235, 52 nodes, and 414 edges) (Figure 4C), cluster 3 (score: 15.862, 30 nodes, and 230 edges) (Figure 5D), and cluster 4 (score: 13.308, 27 nodes, and 173 edges) (Figure 4E). We then performed GO/KEGG analysis on cluster 1 and cluster 2 genes. The results showed that clusters 1 and 2 were mainly enriched in oxidative phosphorylation, ribosome biosynthesis and the HIF-1 signaling pathway, indicating that oxidation-related biological processes and pathways play an essential role in the pathogenesis of SCM. Meanwhile, the central position occupied by protein products in the PPI network suggests that genes in these clusters may be potential targets for SCM therapy. The results of GO/KEGG analysis of cluster 1 and cluster 2 are shown in Supplementary Table S2.
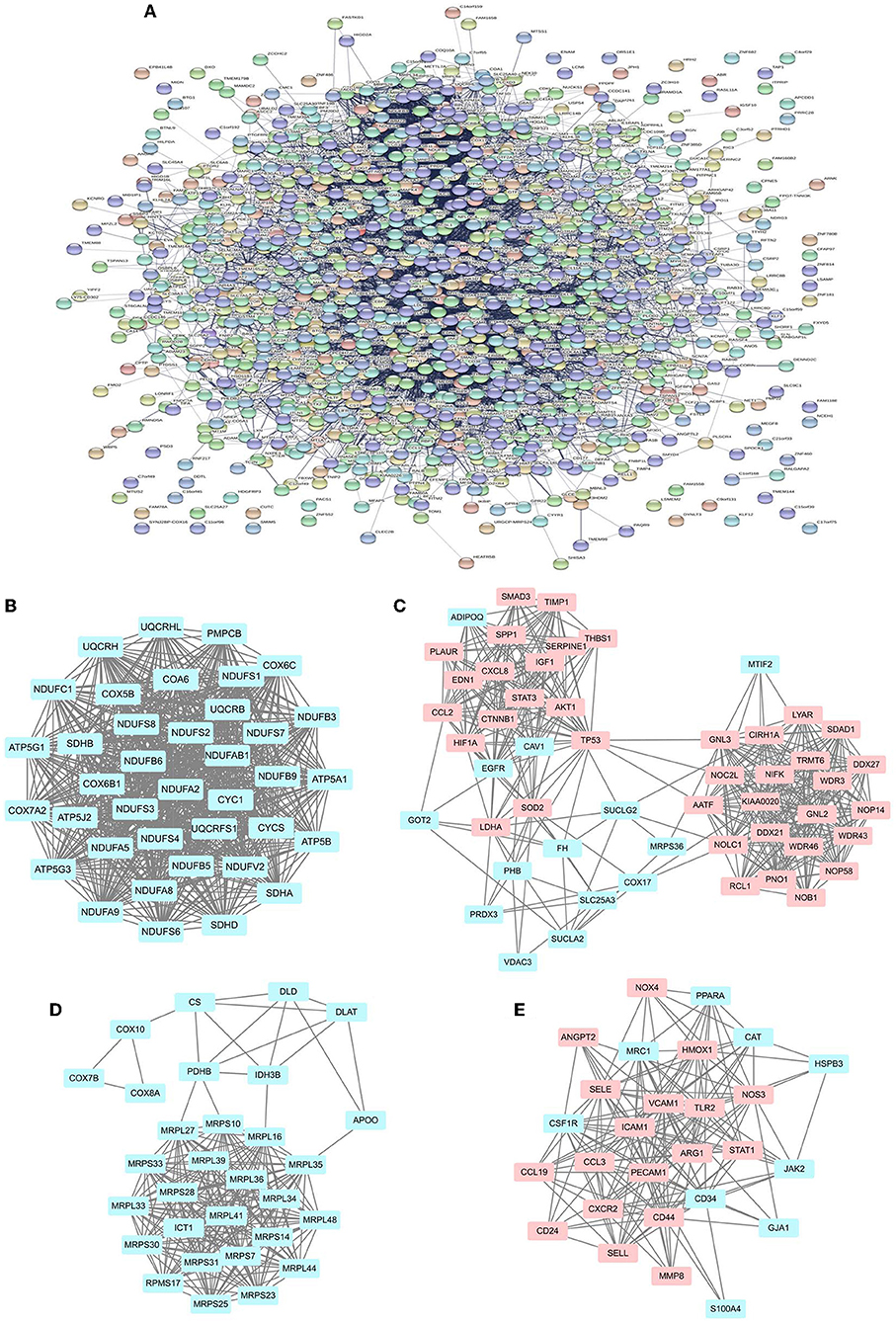
Figure 4. PPI network of DE-mRNAs and four cluster modules extracted by MCODE. (A) The interaction network between proteins encoded by DE-mRNA consists of 1,591 nodes and 12,507 edges. Each node represents a protein, and each edge represents a protein-protein association. The smaller the Q-value, the larger the shape size. (B–E) MCODE extracts four cluster modules. Cluster 1 had the highest cluster score (score: 36.811, 38 nodes and 681 edges), followed by cluster 2 (score: 16.235, 52 nodes, and 414 edges), cluster 3 (score: 15.862, 30 nodes, and 230 edges) and cluster 4 (score: 13.308, 27 nodes, and 173 edges). Blue indicates low expression of the gene, pink indicates high expression of the gene.
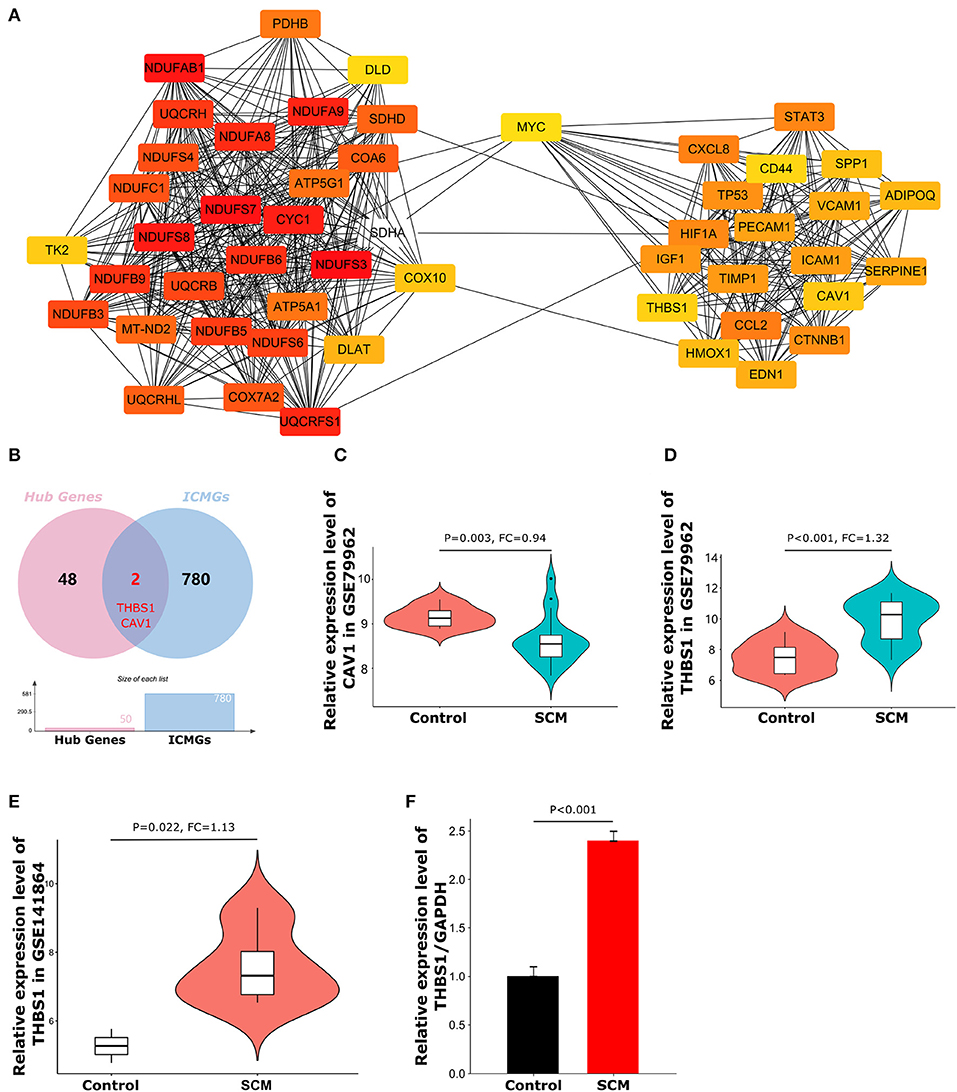
Figure 5. Top50 hub genes and expression of target genes. (A) Cluster plots represent the top 50 hub genes identified by CytoHubba. (B) Venn diagram of the intersection of hub genes and immune cell marker genes. (C) Expression levels of CAV1 in dataset GSE79962. (D) Expression levels of THBS1 in dataset GSE79962. (E) Expression levels of THBS1 in validation dataset GSE141864. (F) The expression level of THBS1 in peripheral blood mononuclear cells of SCM patients was detected by quantitative reverse transcription polymerase chain reaction.
Identification of CytoHubba hub genes and immune-related genes in SCM
CytoHubba is a plug-in that measures the importance of nodes in a network based on network features and provides 11 different topological analysis methods for biological network core element analysis. The Maximum Cluster Centrality (MCC) algorithm has better performance in predicting the accuracy of important proteins in the network. Therefore, the MCC algorithm was chosen in this study for the screening of focused key genes in the PPI network (22). To identify important genes in the PPI network, we used the CytoHubba plugin to identify hub genes. The top 50 hub genes (Figure 5A) were determined by the MCC algorithm on CytoHubba. Details of these hub genes are shown in Table 2. The hub gene refers to a gene that serves as a vital connection in the PPI network and may have a function in the regulation of SCM pathogenesis. Then, immune-related genes in SCM were also identified. We crossed 50 hub genes and 782 ICMGs and obtained 2 overlapping genes: CAV1 and THBS1 (Figure 5B). Figures 5C,D show the expression levels of CAV1 and THBS1 in dataset GSE79962. To obtain the target genes, we used the GSE141864 dataset as a validation dataset to verify whether 2 overlapping genes have a ubiquitous expression in SCM. Dataset GSE141864 validation results show that only THBS1 exists in both the dataset GSE141864 and the dataset GSE79962. And the expression of THBS1 in the GSE141864 dataset was upregulated (Figure 5E), which is consistent with the expression trend of THBS1 in the dataset GSE79962. Therefore, THBS1 was selected as the target gene for this study.
Validation of target genes
To further verify the reliability of the expression level of the target gene THBS1, we collected PBMCs from three clinical SCM patients and three normal individuals for qRT-PCR experiments. The primer sequences and other characteristics used for qRT-PCR analysis are shown in Table 3. As shown in Figure 5F, the expression level of THBS1 in PBMCs of SCM patients was significantly higher than that of normal controls (P < 0.01).
IRHG-THBS1 has excellent diagnostic efficacy for SCM
ROC curve analysis further demonstrated that THBS1 has a good role in discriminating SCM patients from healthy controls in the experimental dataset GSE79962 and the validation dataset GSE141864. The results showed that the area under the ROC curve (AUC) for the experimental dataset GSE79962 was 0.909 (Figure 6A), and the AUC for the validation dataset GSE141864 was 1.000 (Figure 6B). This indicates that THBS1 has a good ability to distinguish SCM patients from healthy controls, suggesting that THBS1 has potential diagnostic value for septic cardiomyopathy.
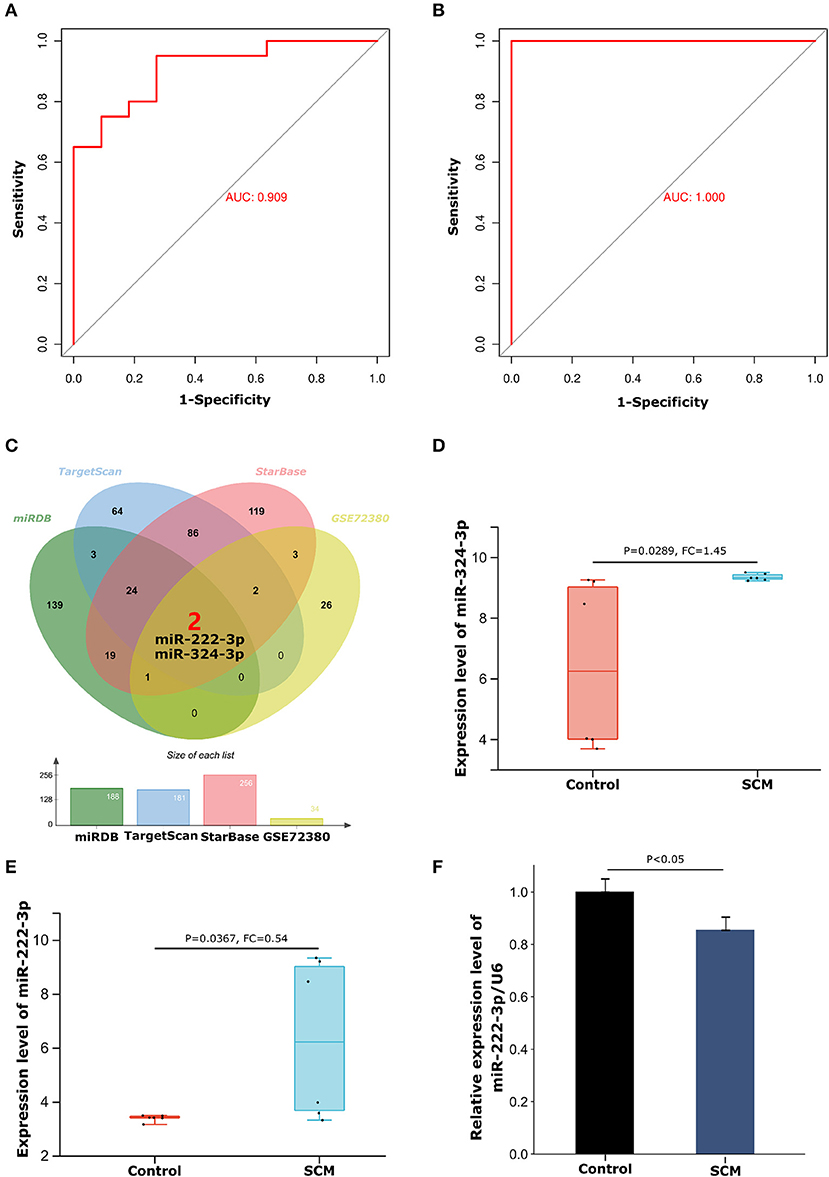
Figure 6. ROC curve diagnostic results of THBS1 and construction of the miRNA-mRNA regulatory network. (A) ROC curve of THBS1 in test dataset GSE79962. (B) ROC curve of THBS1 in validation dataset GSE14186. (C) Venn diagram of the intersection of THBS1's miRDB, TargetScan and StarBase database prediction results and GSE72380 dataset. (D) Expression levels of miR-324-3p in the GSE72380 dataset. (E) Expression levels of miR-222-3p in the GSE72380 dataset. (F) The expression level of miR-222-3p in peripheral blood mononuclear cells of SCM patients was detected by quantitative reverse transcription polymerase chain reaction.
Immune-related miR-222-3p/THBS1 network in SCM
From the miRDB, TargetScan and StarBase databases, we predicted 188, 181, and 256 miRNAs, respectively Details of the prediction results are shown in the Supplementary Table S3. We then took the intersecting miRNAs from the prediction results of the three databases and validated them using the GSE72380 dataset, resulting in 2 shared miRNAs: miR-324-3p and miR-222-3p (Figure 6C). The expression levels of miR-324-3p (P = 0.0289, FC = 1.45) and miR-222-3p (P = 0.0367, FC = 0.54) in the GSE72380 dataset are shown in Figures 6D,E. Functional studies of miRNAs show that miRNAs often act as mRNA sponges in the process of transcript regulation, inhibiting mRNA expression or promoting mRNA degradation, that is, miRNAs are negatively correlated with mRNA levels (30). Therefore, based on this mechanism of action of miRNAs, we selected the down-regulated miR-222-3p as the regulatory miRNAs of THBS1. Concomitantly, THBS1 was similarly predicted in the results of the RNA22 database for reverse prediction of target genes of miR-222-3p, which further enhanced the reliability of the results. The target gene prediction results of miR-222-3p are shown in Supplementary Table S4. Next, to verify the expression of miR-222-3p in SCM patients, PBMCs from SCM patients and healthy controls were extracted for qRT-PCR. The results showed that miR-222-3p was lowly expressed in PBMCs of SCM patients, which was consistent with the miRNA-mRNA regulatory hypothesis (Figure 6F). Thus, we finally obtained the miR-222-3p/THBS1 regulatory network with a regulatory role in SCM.
Discussion
The occurrence of septic cardiomyopathy is very detrimental to the survival and prognosis of septic patients. At the same time, the multiple organ chain reaction and circulatory system failure of SCM have also attracted scholars to study SCM. Currently, systolic or diastolic ventricular dysfunction and primary cardiomyocyte injury are commonly used as clinical criteria for the evaluation of SCM. However, the commonly used SCM diagnosis based on echocardiography (31), B-type natriuretic peptide (BNP) (32) and cardiac troponin I (cTnI) and other markers of myocardial injury have poor sensitivity and specificity, hindering early intervention in SCM. Therefore, finding more sensitive and specific biomarkers in SCM is crucial for early intervention in SCM.
With the development of genomics and the continuous maturation of gene sequencing technology, target gene technology is also increasingly used for disease localization, early diagnosis and treatment (33, 34). The construction of gene co-expression networks is an important direction of current disease research. By analyzing the changes of gene expression under pathological conditions, we can explore the key genes and mechanisms of disease development, reveal the changes of disease gene level, and provide direction for early diagnosis of disease (35).
The balance of the immune system plays an important role in maintaining the body's homeostasis, and an excessive or unregulated immune response will cause severe organ/tissue damage in the body. Existing studies suggest that the immune microenvironment plays an important role in the occurrence, progression, and susceptibility of sepsis (36, 37). For example, in the pathogenesis of SCM, it has been found that the activation of the complement system (38) and the increase of extracellular histones (39, 40) can further deteriorate the function of cardiomyocytes. Therefore, immune response may play an important regulatory role in SCM. However, the specific mechanism of action of IRGs in SCM remains unclear. Therefore, we aimed to analyze the SCM dataset by bioinformatics methods to explore the regulatory role of IRGs and their co-expression networks in SCM.
Microarray dataset analysis based on public databases is currently an effective method in the screening of disease target genes (41). The GSE79962 and GSE141864 datasets collected myocardial tissue samples from patients who died of sepsis and controls who died from sepsis and performed an array of data. Both datasets are well-organized and include abundant sample sizes, producing them good resources for bioinformatics analysis. In this study, we integrated the septic cardiomyopathy dataset with 782 immune cell marker genes (ICMGs), applied bioinformatics approaches to identify IRHGs with immunomodulatory roles in human SCM, and constructed immune-related miRNA-IRHGs regulatory axis in SCM, with the intention of improving our understanding of SCM pathogenesis and exploring its potential biomarkers. The dataset GSE79962 is used to investigate critical genes and mechanisms of SCM in this paper. To clarify the differences in immune cell composition between SCM myocardial tissue and normal myocardial tissue, we performed an immune cell infiltration analysis of the dataset GSE79962. The results of the analysis showed that there were 11 types of immune cells with significant differences in the degree of infiltration between SCM myocardial tissue and normal tissue. Th17 cells play an important role in the regulation of immune-related diseases (42), and previous findings suggest that an increase in neutrophils and Th17 cells may lead to a decrease in immune system defenses in patients with sepsis (43, 44); Studies on Tfh cells have shown that Tfh cells are associated with mortality warnings in patients with sepsis, and low Tfh cell levels may imply a poor prognosis for patients (45); Danahy et al. 's research shows that in sepsis, not only the total number of CD8+ T cells decreases, but also the antigen-driven proliferation capacity and effector function of CD8+ T cells are also impaired (46); These are consistent with the results of our immune infiltration analysis, which further confirmed the important role of abnormal immune response in the pathogenesis and progression of SCM.
After analyzing the immune infiltration of dataset GSE79962, DE-mRNAs of dataset GSE79962 were further screened and subjected to GO/KEGG analysis. A total of 788 up-regulated and 804 down-regulated SCM-related DE-mRNAs were identified in the GSE79962 dataset. And these DE-mRNAs are related to biological processes such as the generation of precursor metabolites and energy, energy derivation by oxidation of organic compounds, and response to hormone. To further screen genes with increasing regulatory roles in SCM, a PPI network based on DE-mRNAs in the STRING public database was constructed. Through the PPI network, we obtained 4 central clusters and the top 50 hub genes in DE-mRNAs. After crossing the Top 50 hub genes with ICMGs and validated by the GSE141864 dataset and qRT-PCR, the central target gene THBS1 with immune function in SCM was finally obtained. The ROC curve is an important step in the development of a test with the desired level of sensitivity and specificity (47) and is important in assessing whether a given test marker has a valid diagnostic ability in the early stages of the disease (26). The AUC values under the ROC curve of THBS1 were 0.909 and 1.000, indicating that THBS1 has a good ability to distinguish SCM patients from healthy people.
THBS1 (thrombospondin 1), a member of the thrombospondin family, is expressed in many tissues during embryonic development, but in healthy adults, it is associated with ischemia-reperfusion, tissue remodeling, immune system damage (rheumatoid synovium, glomerulonephritis), atherosclerotic lesions, and high glucose and high-fat induction. Xie et al.'s model of LPS-induced sepsis showed that THBS1 was also high in injured cardiomyocytes, accompanied by increased rates of inflammatory cytokines, ROS and apoptosis, indicating that THBS1 has a regulatory role in the inflammatory response of SCM, which coincides with our result that THBS1 is an immune gene (48); McMaken et al. showed that THBS1–/– mouse macrophages had stronger phagocytosis, increased bacterial clearance, indicating that THBS1 can be activated by innate Sexual immunity affects mortality in SCM mice (49); in addition, thrombocytopenia during sepsis is also associated with THBS1 (50); Sun et al. showed that knockdown of THBS1 level can inhibit the TGF-β/Smad3 pathway and alleviate sepsis-induced pyroptosis (51). These suggest that IRCGs-THBS1 is likely to modulate the development of SCM through ROS oxidative stress, enhanced macrophage clearance, or TGF-β-related pathways, providing research directions for the treatment of SCM and suggesting that THBS1 levels have high diagnostic value for SCM detection.
In addition, although the immune response is an important pathological process in SCM, immune-related regulatory networks in SCM are uncommon. Here, we construct a miRNA-IRHGs regulatory network for THBS1 to explore the potential mechanism of action of THBS1 in SCM immunity. In this study, the immune-related miR-222-3p/THBS1 signal axis in SCM was identified by bioinformatics analysis.
miR-222-3p/THBS1 regulatory axis
MicroRNAs have regulatory roles at both the transcriptional and post-transcriptional levels of gene information, and according to the mRNA repression effect of miRNAs (52), the level of target miRNAs should be negatively correlated with THBS1. Our results show that IRHGs-THBS1 is upregulated in SCM. This means that miRNAs upstream of IRHG-THBS1 should be at low levels in the SCM state. The LPS-induced SCM model mouse heart dataset GSE72380 was used as a validation dataset in this paper. Data from mouse myocardial tissue and in vivo expression data will demonstrate the stability and reliability of the experimental results. Finally, through database prediction, dataset GSE72380 and qRT-PCR validation, the down-regulated miR-222-3p in SCM was selected as the target miRNA.
Xu et al. performed miRNA array analysis on the plasma of septic mice and showed that the level of miR-222-3p in extracellular vesicles (EVs) was more than 1.5-fold higher than that in control mice, and miR-222-3p regulates cytokine production through downstream TLR7-MyD88 signaling, affecting SCM myocardial inflammation (53); However, the current research on miR-222-3p in sepsis and septic cardiomyopathy is scarce, but other scholars have studied the research of miR-222-3p in rheumatoid arthritis (54) and hepatitis (55), which indirectly reflects the inflammatory regulation of miR-222-3p and provides a reference for the follow-up research of miR-222-3p in SCM. Sun et al.'s (56) study on liver cancer showed that miR-222-3p could regulate the expression of THBS1 and affect the proliferation and apoptosis of hepatocellular carcinoma. However, there is a lack of research on the miR-222-3p expression levels in SCM cardiomyocytes and the mutual regulation of miR-222-3p with THBS1, which means that we still need to conduct further cellular or in vivo SCM experiments to verify them.
However, SCM is a complex immune system disease caused by pathogen invasion and immune system activation. Furthermore, multi-organ damage is involved in the development of SCM, which means that diagnostic markers for SCM are abundant and variable. Our findings demonstrate that THBS1, which is highly expressed in SCM cardiomyocytes, may be an important biomarker for SCM diagnosis. Compared with myocardial injury markers and inflammation-associated proteins with diagnostic support for SCM, such as troponin (cTnI, cTnT), B-type NP (BNP) (57) and inflammation-associated monocyte chemotactic protein 1 (MCP-1) (58), THBS1 identified in this study is not only elevated in human SCM cardiac tissue but also has miRNA regulatory mechanisms upstream of it. This further illustrates the sensitivity and diversity of THBS1 regulation. However, some scholars' studies have shown that THBS1 is involved not only in SCM, but also in atrial fibrillation (59) and tumor invasion (60), therefore, an in-depth exploration of the diagnostic and regulatory ability of THBS1 and its miR-222-3p/THBS1 signaling pathway in SCM, and testing whether THBS1 can be used as a stable biomarker for SCM diagnosis independent of other diseases, will be more home clarify the perspective of this study and provide the best possible de support for early clinical intervention and treatment of SCM.
From the published articles, we also found that Chen et al. (61), Kang et al. (62), Gong et al. (63), Li et al. (64), and Wang et al. (65). similarly applied bioinformatics approaches to study the hub genes in SCM. First and foremost, our studies with Chen et al. (61), Kang et al. (62), Li et al. (64), and Wang et al. (65) used the human SCM dataset GSE79962 as an experimental dataset, but we innovatively combined immune cell marker genes with SCM differentially expressed genes to identify the biomarker role of IRHG in SCM; Secondly, we also used MCODE to identify the central clusters of SCM differentially expressed genes to explore modules that play an important interaction in SCM and their involved pathways; Thirdly, different from the studies of Kang et al. (62) and Gong et al. (63), we also extracted human samples to verify the expression of target genes, and used ROC curves to analyze the diagnosticity of target genes for SCM. Finally, a miRNA-IRHG pair that is different from previous studies and has an immunomodulatory role in SCM was obtained: miR-222-3p/THBS1. Details of the comparison between this study and previous studies are presented in Table 4.
In summary, this study used bioinformatics analysis to innovatively combine the GEO database with immune cell marker genes to investigate the presence of immune-related diagnostic biomarkers THBS1 in infectious cardiomyopathy and to establish miR-222-3p/THBS1 regulatory pairs with immunomodulatory effects. The findings of this work demonstrate the biological regulatory role of epigenetic mechanisms in the progression of SCM and analyze important biomarkers and prospective immunotherapeutic targets for SCM, providing new therapeutic targets and directions for early intervention in SCM. However, studies on the epigenetic mechanisms of SCM are still lacking. The acquisition of myocardial tissues from SCM patients and the completion of the identification of SCM differentially expressed genes and their epigenetic mechanisms by gene sequencing technology will advance the realization of early clinical intervention in SCM and deepen our understanding of the pathogenesis of septic cardiomyopathy. The new working model used in this study on human SCM research is shown in Figure 7.
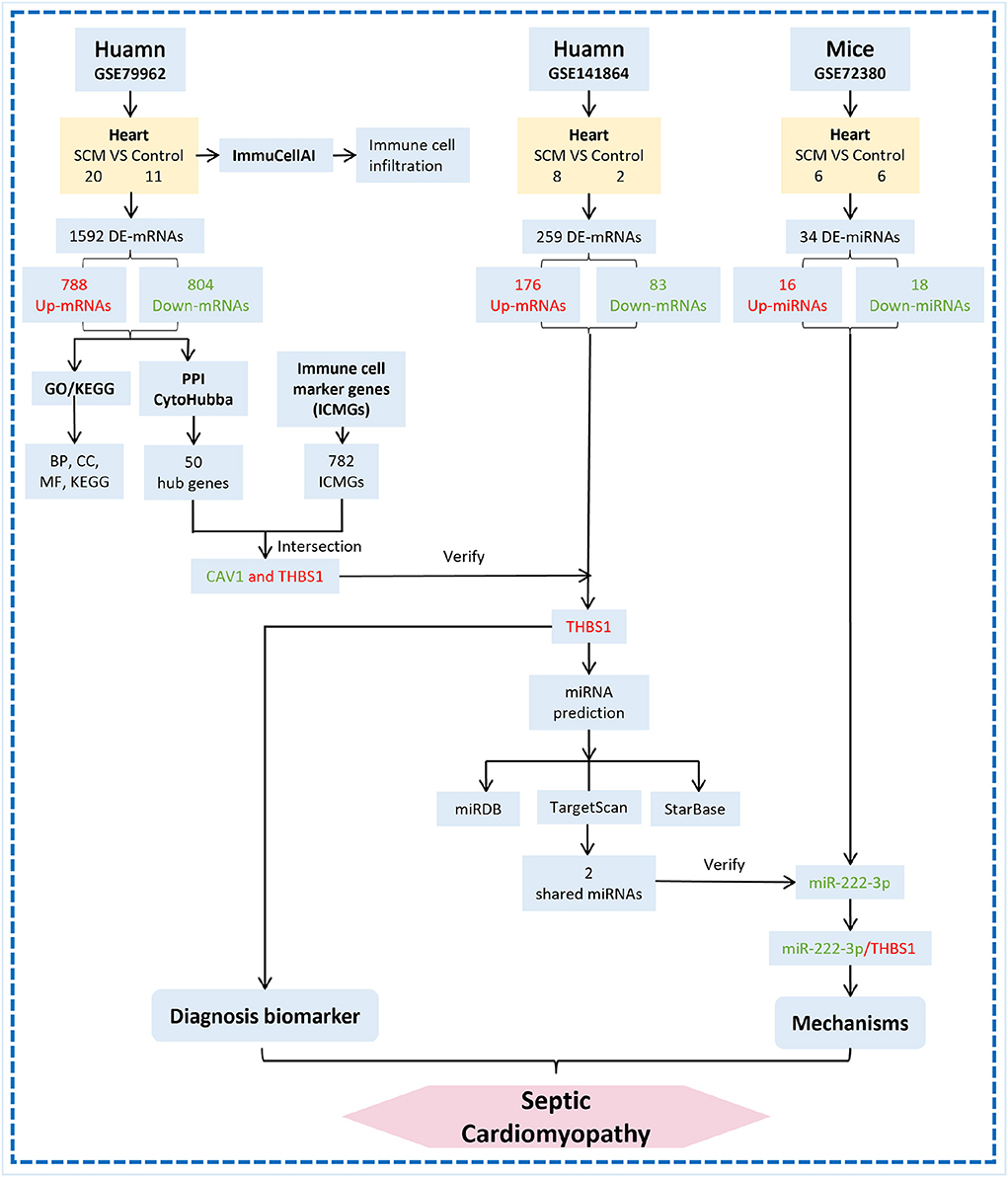
Figure 7. Flow chart of miRNA-mRNA regulatory network construction in cardiac tissue of SCM patients. The red word means upregulation and the green word means downregulation.
However, it is worth noting that there are still some restrictions on our study. First, bioinformatics analysis was the main method of this study, and we next need to construct an experimental animal model of SCM to study the expression level of miR-222-3p/THBS1 and its regulatory mechanism in vivo; second, although 19 control samples and 34 SCM samples have been used for the evaluation and experimental validation of this study, further expansion of the study sample size will enhance the credibility of our results. Additionally, only PBMCs from SCM patients were collected for the outcome validation of this study, and the sample size of PBMCs was not large enough, which may cause information bias to some extent. The further acquisition of more peripheral blood and even myocardial tissues from SCM patients for gene expression analysis would improve the credibility and authenticity of the results of this study. Last but not least, the dearth of intrinsic information regarding the level of SCM activity necessitates further analysis of the relationship between immune genes and their regulatory networks and disease and disease severity.
Data availability statement
Publicly available datasets were analyzed in this study. This data can be found at: https://www.ncbi.nlm.nih.gov/gds/?term$=$GSE79962; https://www.ncbi.nlm.nih.gov/gds/?term=GSE141864; https://www.ncbi.nlm.nih.gov/gds/?term=GSE72380.
Ethics statement
The studies involving human participants were reviewed and approved by Ethics Committee of the First Affiliated Hospital of Kunming Medical University, Yunnan Province [(2022), ethical review L, No. 23]. The patients/participants provided their written informed consent to participate in this study.
Author contributions
All authors listed have made a substantial, direct, and intellectual contribution to the work and approved it for publication.
Funding
This study was supported by the National Natural Science Foundation of China (Nos. 81860073 and 81760074), Special Foundation Projects of Joint Applied Basic Research of Yunnan Provincial Department of Science and Technology with Kunming Medical University [No. 2019FE001(-138)], Yunnan Provincial Department of Science and Technology (No. 202001AT070039), Yunnan Health Training Project of High Level Talents (No. H-2018032), 100 Young and Middle-aged Academic and Technical Backbones of Kunming Medical University (No. 60118260106), Young Talents of Yunnan Thousand Talents Plan (RLQN20200002), and Clinical Medcial Center for Cardiovascular and Cerebrovascular Disease of Yunnan Province (No. ZX2019-03-01).
Conflict of interest
The authors declare that the research was conducted in the absence of any commercial or financial relationships that could be construed as a potential conflict of interest.
Publisher's note
All claims expressed in this article are solely those of the authors and do not necessarily represent those of their affiliated organizations, or those of the publisher, the editors and the reviewers. Any product that may be evaluated in this article, or claim that may be made by its manufacturer, is not guaranteed or endorsed by the publisher.
Supplementary material
The Supplementary Material for this article can be found online at: https://www.frontiersin.org/articles/10.3389/fcvm.2022.971543/full#supplementary-material
References
1. Martin GS, Mannino DM, Eaton S, Moss M. The epidemiology of sepsis in the United States from 1979 through 2000. N Engl J Med. (2003) 348:1546–54. doi: 10.1056/NEJMoa022139
2. Singer M, Deutschman CS, Seymour CW, Shankar-Hari M, Annane D, Bauer M, et al. The third international consensus definitions for sepsis and septic shock (sepsis-3). Jama. (2016) 315:801–10. doi: 10.1001/jama.2016.0287
3. Blanco J, Muriel-Bombín A, Sagredo V, Taboada F, Gandía F, Tamayo L, et al. Incidence, organ dysfunction and mortality in severe sepsis: a Spanish multicentre study. Crit Care. (2008) 12:R158. doi: 10.1186/cc7157
4. Crouser ED. Mitochondrial dysfunction in septic shock and multiple organ dysfunction syndrome. Mitochondrion. (2004) 4:729–41. doi: 10.1016/j.mito.2004.07.023
5. Rudiger A, Singer M. Mechanisms of sepsis-induced cardiac dysfunction. Crit Care Med. (2007) 35:1599–608. doi: 10.1097/01.CCM.0000266683.64081.02
6. Hassoun SM, Marechal X, Montaigne D, Bouazza Y, Decoster B, Lancel S, et al. Prevention of endotoxin-induced sarcoplasmic reticulum calcium leak improves mitochondrial and myocardial dysfunction. Crit Care Med. (2008) 36:2590–6. doi: 10.1097/CCM.0b013e3181844276
7. Werdan K, Schmidt H, Ebelt H, Zorn-Pauly K, Koidl B, Hoke RS, et al. Impaired regulation of cardiac function in sepsis, SIRS, and MODS. Can J Physiol Pharmacol. (2009) 87:266–74. doi: 10.1139/Y09-012
8. Celes MR, Torres-Dueñas D, Malvestio LM, Blefari V, Campos EC, Ramos SG, et al. Disruption of sarcolemmal dystrophin and beta-dystroglycan may be a potential mechanism for myocardial dysfunction in severe sepsis. Lab Invest. (2010) 90:531–42. doi: 10.1038/labinvest.2010.3
9. Zhang ZB, Guo YF Li CY, Qiu CW, Guo MY. Selenium influences mmu-miR-155 to inhibit inflammation in Staphylococcus aureus-induced mastitis in mice. Food Funct. (2019) 10:6543–55. doi: 10.1039/C9FO01488H
10. Mendell JT, Olson EN. MicroRNAs in stress signaling and human disease. Cell. (2012) 148:1172–87. doi: 10.1016/j.cell.2012.02.005
11. Bartel DP. MicroRNAs: genomics, biogenesis, mechanism, and function. Cell. (2004) 116:281–97. doi: 10.1016/S0092-8674(04)00045-5
12. Bartel DP. MicroRNAs: target recognition and regulatory functions. Cell. (2009) 136:215–33. doi: 10.1016/j.cell.2009.01.002
13. Govindarajan M, Wohlmuth C, Waas M, Bernardini MQ, Kislinger T. High-throughput approaches for precision medicine in high-grade serous ovarian cancer. J Hematol Oncol. (2020) 13:134. doi: 10.1186/s13045-020-00971-6
14. Mirna M, Paar V, Rezar R, Topf A, Eber M, Hoppe UC, et al. MicroRNAs in inflammatory heart diseases and sepsis-induced cardiac dysfunction: a potential scope for the future? Cells. (2019) 8:1352. doi: 10.3390/cells8111352
15. Manetti AC, Maiese A, Paolo MD, De Matteis A, La Russa R, Turillazzi E, et al. MicroRNAs and sepsis-induced cardiac dysfunction: a systematic review. Int J Mole Sci. (2020) 22:321. doi: 10.3390/ijms22010321
16. Busch K, Kny M, Huang N, Klassert TE, Stock M, Hahn A, et al. Inhibition of the NLRP3/IL-1β axis protects against sepsis-induced cardiomyopathy. J Cachexia Sarcopenia Muscle. (2021) 12:1653–68. doi: 10.1002/jcsm.12763
17. Ren C, Li XH, Wang SB, Wang LX, Dong N, Wu Y, et al. Activation of central alpha 7 nicotinic acetylcholine receptor reverses suppressed immune function of T lymphocytes and protects against sepsis lethality. Int J Biol Sci. (2018) 14:748–59. doi: 10.7150/ijbs.24576
18. Miao YR, Zhang Q, Lei Q, Luo M, Xie GY, Wang H, et al. ImmuCellAI: a unique method for comprehensive T-cell subsets abundance prediction and its application in cancer immunotherapy. Adv Sci. (2020) 7:1902880. doi: 10.1002/advs.201902880
19. Zhou Y, Zhou B, Pache L, Chang M, Khodabakhshi AH, Tanaseichuk O, et al. Metascape provides a biologist-oriented resource for the analysis of systems-level datasets. Nat Commun. (2019) 10:1523. doi: 10.1038/s41467-019-09234-6
20. Szklarczyk D, Gable AL, Nastou KC, Lyon D, Kirsch R, Pyysalo S, et al. The STRING database in 2021: customizable protein-protein networks, and functional characterization of user-uploaded gene/measurement sets. Nucleic Acids Res. (2021) 49:D605–d12. doi: 10.1093/nar/gkaa1074
21. Qing J, Song W, Tian L, Samuel SB Li Y. Potential small molecules for therapy of lupus nephritis based on genetic effect and immune infiltration. Biomed Res Int. (2022) 2022:2259164. doi: 10.1155/2022/2259164
22. Chin CH, Chen SH, Wu HH, Ho CW, Ko MT, Lin CY. cytoHubba: identifying hub objects and sub-networks from complex interactome. BMC Syst Biol. (2014) 8:S11. doi: 10.1186/1752-0509-8-S4-S11
23. Bindea G, Mlecnik B, Tosolini M, Kirilovsky A, Waldner M, Obenauf AC, et al. Spatiotemporal dynamics of intratumoral immune cells reveal the immune landscape in human cancer. Immunity. (2013) 39:782–95. doi: 10.1016/j.immuni.2013.10.003
24. Qing JB, Song WZ Li CQ, Li YF. The diagnostic and predictive significance of immune-related genes and immune characteristics in the occurrence and progression of IgA nephropathy. J. Immunol Res. (2022) 2022:9284204. doi: 10.1155/2022/9284204
25. Hanley JA, McNeil BJ. The meaning and use of the area under a receiver operating characteristic (ROC) curve. Radiology. (1982) 143:29–36. doi: 10.1148/radiology.143.1.7063747
26. Mandrekar JN. Receiver operating characteristic curve in diagnostic test assessment. J Thorac Oncol. (2010) 5:1315–6. doi: 10.1097/JTO.0b013e3181ec173d
27. Chen Y, Wang X. miRDB: an online database for prediction of functional microRNA targets. Nucleic Acids Res. (2020) 48:D127–d31. doi: 10.1093/nar/gkz757
28. Li JH, Liu S, Zhou H, Qu LH. Yang JH. starBase v20: decoding miRNA-ceRNA, miRNA-ncRNA and protein-RNA interaction networks from large-scale CLIP-Seq data. Nucleic Acids Res. (2014) 42:D92–7. doi: 10.1093/nar/gkt1248
29. Barrett T, Wilhite SE, Ledoux P, Evangelista C, Kim IF, Tomashevsky M, et al. NCBI GEO: archive for functional genomics data sets—update. Nucleic Acids Res. (2013) 41:D991–5. doi: 10.1093/nar/gks1193
30. Bak RO, Mikkelsen JG. miRNA sponges: soaking up miRNAs for regulation of gene expression. Wiley Interdiscipl Rev RNA. (2014) 5:317–33. doi: 10.1002/wrna.1213
31. Parker MM, Shelhamer JH, Bacharach SL, Green MV, Natanson C, Frederick TM, et al. Profound but reversible myocardial depression in patients with septic shock. Ann Inte Med. (1984) 100:483–90. doi: 10.7326/0003-4819-100-4-483
32. Charpentier J, Luyt CE, Fulla Y, Vinsonneau C, Cariou A, Grabar S, et al. Brain natriuretic peptide: a marker of myocardial dysfunction and prognosis during severe sepsis. Crit Care Med. (2004) 32:660–5. doi: 10.1097/01.CCM.0000114827.93410.D8
33. Zeng X, Li Z, Zhu C, Xu L, Sun Y, Han S. Research progress of nanocarriers for gene therapy targeting abnormal glucose and lipid metabolism in tumors. Drug Deliv. (2021) 28:2329–47. doi: 10.1080/10717544.2021.1995081
35. van Dam S, Võsa U, van der Graaf A, Franke L, de Magalhães JP. Gene co-expression analysis for functional classification and gene-disease predictions. Brief Bioinform. (2018) 19:575–92. doi: 10.1093/bib/bbw139
36. Weber GF, Maier SL, Zönnchen T, Breucha M, Seidlitz T, Kutschick I, et al. Analysis of circulating plasmacytoid dendritic cells during the course of sepsis. Surgery. (2015) 158:248–54. doi: 10.1016/j.surg.2015.03.013
37. Ji J, Fan J. Neutrophil in reverse migration: role in sepsis. Front Immunol. (2021) 12:656039. doi: 10.3389/fimmu.2021.656039
38. Fattahi F, Frydrych LM, Bian G, Kalbitz M, Herron TJ, Malan EA, et al. Role of complement C5a and histones in septic cardiomyopathy. Mol Immunol. (2018) 102:32–41. doi: 10.1016/j.molimm.2018.06.006
39. Kalbitz M, Fattahi F, Grailer JJ, Jajou L, Malan EA, Zetoune FS, et al. Complement-induced activation of the cardiac NLRP3 inflammasome in sepsis. FASEB J. (2016) 30:3997–4006. doi: 10.1096/fj.201600728R
40. Fuchs TA, Brill A, Duerschmied D, Schatzberg D, Monestier M, Myers DD. Jr., et al. Extracellular DNA traps promote thrombosis. Proc Natl Acad Sci U S A. (2010) 107:15880–5. doi: 10.1073/pnas.1005743107
41. Ivandic BT, Mastitsky SE, Schönsiegel F, Bekeredjian R, Eils R, Frey N, et al. Whole-genome analysis of gene expression associates the ubiquitin-proteasome system with the cardiomyopathy phenotype in disease-sensitized congenic mouse strains. Cardiovasc Res. (2012) 94:87–95. doi: 10.1093/cvr/cvs080
42. Qing J, Li C, Hu X, Song W, Tirichen H, Yaigoub H, et al. Differentiation of T helper 17 cells may mediate the abnormal humoral immunity in IgA nephropathy and inflammatory bowel disease based on shared genetic effects. Front Immunol. (2022) 13:916934. doi: 10.3389/fimmu.2022.916934
43. Shen XF, Cao K, Jiang JP, Guan WX, Du JF. Neutrophil dysregulation during sepsis: an overview and update. J Cell Mol Med. (2017) 21:1687–97. doi: 10.1111/jcmm.13112
44. Liu Y, Wang X, Yu L. Th17, rather than Th1 cell proportion, is closely correlated with elevated disease severity, higher inflammation level, and worse prognosis in sepsis patients. J Clin Lab Anal. (2021) 35:e23753. doi: 10.1002/jcla.23753
45. Duan S, Jiao Y, Wang J, Tang D, Xu S, Wang R, et al. Impaired B-cell maturation contributes to reduced B cell numbers and poor prognosis in sepsis. Shock. (2020) 54:70–7. doi: 10.1097/SHK.0000000000001478
46. Danahy DB, Strother RK, Badovinac VP, Griffith TS. Clinical and experimental sepsis impairs CD8 T-cell-mediated immunity. Crit Rev Immunol. (2016) 36:57–74. doi: 10.1615/CritRevImmunol.2016017098
47. Hoo ZH, Candlish J, Teare D. What is an ROC curve? Emerg Med J. (2017) 34:357–9. doi: 10.1136/emermed-2017-206735
48. Xie Y, Zhang J, Jin W, Tian R, Wang R. Role of Thrombospondin-1 in sepsis-induced myocardial injury. Mole Med Rep. (2021) 24:869. doi: 10.3892/mmr.2021.12509
49. McMaken S, Exline MC, Mehta P, Piper M, Wang Y, Fischer SN, et al. Thrombospondin-1 contributes to mortality in murine sepsis through effects on innate immunity. PLoS ONE. (2011) 6:e19654. doi: 10.1371/journal.pone.0019654
50. Bedet A, Razazi K, Boissier F, Surenaud M, Hue S, Giraudier S, et al. Mechanisms of thrombocytopenia during septic shock: a multiplex cluster analysis of endogenous sepsis mediators. Shock. (2018) 49:641–8. doi: 10.1097/SHK.0000000000001015
51. Sun J, Ge X, Wang Y, Niu L, Tang L, Pan S. USF2 knockdown downregulates THBS1 to inhibit the TGF-β signaling pathway and reduce pyroptosis in sepsis-induced acute kidney injury. Pharmacol Res. (2022) 176:105962. doi: 10.1016/j.phrs.2021.105962
52. Saliminejad K, Khorram Khorshid HR, Soleymani Fard S, Ghaffari SH. An overview of microRNAs: biology, functions, therapeutics, and analysis methods. J Cell Physiol. (2019) 234:5451–65. doi: 10.1002/jcp.27486
53. Xu J, Feng Y, Jeyaram A, Jay SM, Zou L, Chao W. Circulating plasma extracellular vesicles from septic mice induce inflammation via MicroRNA- and TLR7-dependent mechanisms. J Immunol. (2018) 201:3392–400. doi: 10.4049/jimmunol.1801008
54. Yang Z, Lin SD, Zhan F, Liu Y, Zhan YW. LncRNA GAS5 alleviates rheumatoid arthritis through regulating miR-222-3p/Sirt1 signalling axis. Autoimmunity. (2021) 54:13–22. doi: 10.1080/08916934.2020.1846183
55. Zhang P, Yu J, Gui Y, Sun C, Han W. Inhibition of miRNA-222-3p Relieves staphylococcal enterotoxin b-induced liver inflammatory injury by upregulating suppressors of cytokine signaling 1. Yonsei Med J. (2019) 60:1093–102. doi: 10.3349/ymj.2019.60.11.1093
56. Sun Y, Shi P, Wu Q, Liu B, Yu Z, Jia H, et al. MiR-222-3p induced by hepatitis B virus promotes the proliferation and inhibits apoptosis in hepatocellular carcinoma by upregulating THBS1. Hum Cell. (2021) 34:1788–99. doi: 10.1007/s13577-021-00577-1
57. Ehrman RR, Sullivan AN, Favot MJ, Sherwin RL, Reynolds CA, Abidov A, et al. Pathophysiology, echocardiographic evaluation, biomarker findings, and prognostic implications of septic cardiomyopathy: a review of the literature. Crit Care. (2018) 22:112. doi: 10.1186/s13054-018-2043-8
58. Huang M, Cai S, Su J. The Pathogenesis of Sepsis and Potential Therapeutic Targets. International journal of molecular sciences. (2019) 20. doi: 10.3390/ijms20215376
59. Liu H, Yang J, Zhang J, Zheng T, Zhai Y. THBS1: a potential biomarker for atrial fibrillation. Int J Cardiol. (2021) 345:129. doi: 10.1016/j.ijcard.2021.10.152
60. Liu X, Xu D, Liu Z, Li Y, Zhang C, Gong Y, et al. THBS1 facilitates colorectal liver metastasis through enhancing epithelial-mesenchymal transition. Clin Transl Oncol. (2020) 22:1730–40. doi: 10.1007/s12094-020-02308-8
61. Chen M, Kong C, Zheng Z, Li Y. Identification of biomarkers associated with septic cardiomyopathy based on bioinformatics analyses. J Comput Biol. (2020) 27:69–80. doi: 10.1089/cmb.2019.0181
62. Kang K, Li J, Li R, Xu X, Liu J, Qin L, et al. Potentially critical roles of NDUFB5, TIMMDC1, and VDAC3 in the progression of septic cardiomyopathy through integrated bioinformatics analysis. DNA Cell Biol. (2020) 39:105–17. doi: 10.1089/dna.2019.4859
63. Gong CW, Yuan MM, Qiu BQ, Wang LJ, Zou HX, Hu T, et al. Identification and validation of ferroptosis-related biomarkers in septic cardiomyopathy via bioinformatics analysis. Front Genet. (2022) 13:827559. doi: 10.3389/fgene.2022.827559
64. Li J, Zhou L, Li Z, Yang S, Tang L, Gong H. Identification of crucial genes and infiltrating immune cells underlying sepsis-induced cardiomyopathy via weighted gene co-expression network analysis. Front Genet. (2021) 12:812509. doi: 10.3389/fgene.2021.812509
Keywords: septic cardiomyopathy, miRNA, immune-related gene, biomarker, miRNA-mRNA regulatory network
Citation: Li J, Sun G, Ma H, Wu X, Li C, Ding P, Lu S, Li Y, Yang P, Li C, Yang J, Peng Y, Meng Z and Wang L (2022) Identification of immune-related hub genes and miRNA-mRNA pairs involved in immune infiltration in human septic cardiomyopathy by bioinformatics analysis. Front. Cardiovasc. Med. 9:971543. doi: 10.3389/fcvm.2022.971543
Received: 17 June 2022; Accepted: 29 August 2022;
Published: 20 September 2022.
Edited by:
Francesca Innocenti, Careggi University Hospital, ItalyReviewed by:
Yafeng Li, The Fifth Hospital of Shanxi Medical University, ChinaQian Yang, First Affiliated Hospital of Chinese PLA General Hospital, China
Copyright © 2022 Li, Sun, Ma, Wu, Li, Ding, Lu, Li, Yang, Li, Yang, Peng, Meng and Wang. This is an open-access article distributed under the terms of the Creative Commons Attribution License (CC BY). The use, distribution or reproduction in other forums is permitted, provided the original author(s) and the copyright owner(s) are credited and that the original publication in this journal is cited, in accordance with accepted academic practice. No use, distribution or reproduction is permitted which does not comply with these terms.
*Correspondence: Zhaohui Meng, zhmeng@aliyun.com; Luqiao Wang, wlq8360@163.com
†These authors have contributed equally to this work and share first authorship