- Department of Cardiovasology, The First Hospital of Yulin, Yulin, China
Background: Numerous genetic studies have shown that genes are related to the pathogenesis of coronary heart disease (CHD). The main aim of this study was to confirm whether fibronectin type III domain containing 1 (FNDC1) polymorphisms correlate with the risk of CHD.
Methods: In this study, in order to assess the association between three FNDC1 single nucleotide polymorphisms (SNPs) and the risk of CHD, we conducted a case-control study involving 630 patients with CHD and 568 healthy controls using Agena MassARRAY (Agena Bioscience, San Diego, CA, USA). Genotype distribution in case and control groups was analyzed by Chi square test. Odds ratios (ORs) and 95% confidence intervals (CIs) were calculated by logistic regression models adjusted for age, sex, smoking, and alcohol consumption to assess the correlation between SNPs and CHD risk.
Results: Our results indicated that FNDC1-rs420137, -rs386360, and -rs7763726 played important roles in enhancing the risk of CHD. Subgroup analysis revealed that rs420137 increased the susceptibility to CHD in males, smokers, and patients aged ≤62 years. Rs360 had an increased risk of CHD in males, patients at aged ≤62 years, smokers, and non-drinkers. Furthermore, the association of rs7763726 with increased CHD risk was also observed in males, patients aged ≤62 years, smokers, and drinkers. Last but not least, these three SNPs we selected were protective factors against hypertension in CHD individuals.
Conclusion: Our research suggest that FNDC1-rs420137, -rs386360, and -rs7763726 variants may be regarded as novel biomarkers for predicting CHD risk and other specific mechanisms of action of CHD need to be further studied.
Introduction
Coronary heart disease (CHD) is a very common cardiovascular disease. It is estimated that the total number of deaths from cardiovascular disease is expected to increase to 23.3 million by 2030 (1). Since the 1970 s, CHD has been the main cause of death all over the world. In China, 700,000 people die of CHD annually (2). In addition, the morbidity of CHD is rising. The occurrence and development of CHD correlate with a variety of environmental factors, including social factors (aging, employment, income, and education), behavioral factors (dietary habits, drinking, smoking, and exercise), and metabolic factors obesity, diabetes mellitus (DM), triglyceride (TG), and high-density lipoprotein cholesterol (HDL-C) (3). However, an increasing number of studies have shown that genetic factors play a critical role in the progression of CHD. By far, more than 60 genetic variants have been identified to be related to an increased susceptibility to CHD in genome-wide association study (GWAS) analyses (4). Interestingly, studies have shown that single nucleotide polymorphisms (SNPs) can affect the level of gene production or the binding of targets, thus influencing the normal expression of genes and leading to the occurrence of diseases (5, 6).
Fibronectin type III domain containing 1 (FNDC1) is a protein-encoding and disease-related gene located in the 6q25.3 region of the human chromosome and contains 102,713 bases, with a length of ~1,894 and a molecular weight of 205.6 kDa (7, 8). Moreover, FNDC1, also known as activator of G-protein signaling 8 (AGS8), is a positive factor in modulating the signal transduction system. It is expressed in various types of tissues, including thyroid, heart, fetal cartilage, adipose, and kidney tissues (9–11). As an important extracellular matrix protein, FNDC1 plays a regulatory role in tumorigenesis and participates in cell migration, proliferation, and apoptosis. It is also involved in the pathogenesis of multiple blood and cardiovascular diseases (12). Previous studies have also shown that FNDC1 has a certain relationship with hypertension (13).
To our knowledge, the effects of FNDC1 gene polymorphisms on CHD have not been studied. Therefore, the present study explored the linkage between FNDC1 polymorphisms and CHD susceptibility, which has considerable implications for the diagnosis and treatment of CHD.
Materials and methods
Study population
A total of 630 CHD cases and 568 controls were recruited from the First Hospital of Yulin City. All patients were independently judged by at least two cardiologists and diagnosed as CHD according to blood examination, coronary angiography, standardized electrocardiogram (ECG), and echocardiography. Out-patients in the control group were from the same hospital during the same period as the cases. According to previous medical history and angiographic diagnosis, they had no CHD, no obvious ischemic changes on ECG and no symptoms of chest pain. Participants with other heart diseases (rheumatic heart disease, cardiomyopathy, concomitant heart valve disease, or congenital heart disease) were eliminated from the study. Furthermore, it should be noted that patients with a history of intravenous thrombolysis, coronary stenting, angioplasty bypass surgery, or coronary artery disease were also excluded. Moreover, clinical indicators and basic characteristics of subjects were collected from medical records.
DNA extraction
We collected 5 mL of fasting peripheral venous blood from each participant and put it in a test tube containing ethylene diamine tetraacetic acid (EDTA). According to the manufacturer's instructions, we extracted DNA from the collected blood samples using GoldMag-Mini whole blood genomic DNA purification kits (GoldMag Co., Ltd., Xi'an City, China).
Genotyping of SNPs
First of all, three variants of FNDC1 (rs420137, rs386360, and rs7763726) were selected from the HapMap database according to the minor allele frequency (MAF) >5% and the unbalanced r2 value >0.8. Then, we genotyped them by the Agena MassARRAY platform (Agena Bioscience, San Diego, CA, USA). Finally, the results of genotyping were processed and analyzed by Agena Bioscience Typer software (version 4.0).
Data analysis
The basic statistical analysis was conducted by Microsoft Excel (Microsoft Corp., Redmond, WA, USA) and SPSS 20.0 software (SPSS, Chicago, IL, USA). The t-test was used to assess continuous variables, while the Chi-square test was utilized to access categorical variables between samples. Meanwhile, the Chi-square test was used to evaluate whether the genotype distribution of these SNPs in the control group was in accordance with Hardy-Weinberg equilibrium (HWE), and the differences in the genotype and allele frequencies of these SNPs in two groups were also analyzed. PLINK 1.9 software (version 1.07) and Haploview 4.2 software were applied to analyze the association of genetic variants with CHD susceptibility under five genetic models, and odds ratios (ORs) and 95% confidence intervals (CIs) were calculated to confirm differences in this study. Besides, multifactor dimensionality reduction (MDR) was used to analyze the effects of SNP-SNP interactions on CHD susceptibility. Finally, a p-value < 0.05 indicated a statistical difference.
Results
Characteristics of subjects
The information on CHD patients is presented in Table 1. Patients with an average age of 62.16 ± 10.37 years included 429 males and 201 females, while controls with an average age of 61.46 ± 8.96 years included 360 males and 208 females. There was no statistically significant difference in the distribution of sex and age between the two groups (p > 0.05). In addition, the levels of HDL-C, TG, urea, alanine amino transferase (ALT), and blood glucose (BG) had no significant differences (p > 0.05) between controls and cases (Table 1). Nevertheless, other indicators [white blood cell count (WBC), red blood cell count (RBC), low-density lipoprotein cholesterol (LDL-C), platelet (PLT), hemoglobin (Hgb), total cholesterol (TC), absolute value of lymphocytes (LYMP), absolute value of neutrophils (NEUT), creatinine, and aspartate aminotransferase (AST)] were significantly different (p < 0.05) between the case and control groups (Table 1).
Association between FNDC1 polymorphisms and CHD risk
Basic information on three SNPs (rs420137, rs386360, and rs7763726) in FNDC1 is displayed in Table 2. All SNPs in the control group were in line with HWE (p > 0.05) and could be further analyzed. At the same time, we found that the frequency of the rs420137-G allele in the case group was significantly higher than that in the control group (p = 0.013, OR: 1.25, 95% CI: 1.05–1.48). The “C” allele of rs386360 (p = 0.010, OR: 1.26, 95% CI: 1.05–1.48) and the “A” allele of rs7763726 (p = 0.001, OR: 1.36, 95% CI: 1.13–1.64) were obviously related to an increased susceptibility to CHD.
As can be seen from Table 3, logistic regression analysis revealed that rs420137-GC was correlated with an increased risk of CHD under the co-dominant (p = 0.037, OR: 1.29, 95% CI: 1.02–1.65), dominant (p = 0.015, OR: 1.34, 95% CI: 1.06–1.68), and additive models (p = 0.011, OR: 1.27, 95% CI: 1.06–1.51). Rs386360-CA had a strong linkage with a higher susceptibility to CHD (co-dominant model: p = 0.026, OR: 1.31, 95% CI: 1.03–1.67; dominant model: p = 0.011, OR: 1.35, 95% CI: 1.07–1.70; additive model: p = 0.009, OR: 1.28, 95% CI: 1.07–1.53). It also showed that rs7763726-GA elevated CHD risk (co-dominant model: p = 0.001, OR: 1.50, 95% CI: 1.17–1.90; dominant model: p = 4.0E-04, OR: 1.52, 95% CI: 1.21–1.92; additive model: p = 7.0E-04, OR: 1.39, 95% CI: 1.15–1.69).
Subgroup analysis of the correlation between FNDC1 polymorphisms and CHD risk
We also performed stratified analyses of the association of three SNPs with CHD risk according to sex, age, smoking, drinking, hypertension, and diabetes to determine whether traditional risk factors influence the role of genetic variants in CHD. According to Table 4, we found that all variants increased the incidence of CHD under the allele, co-dominant, dominant, and additive models in males (rs420137: p = 0.006, 0.010, 0.004, and 0.006, respectively; rs386360: p = 0.007, 0.017, 0.006, and 0.006, respectively; rs7763726: p < 0.001, 0.001, 0.001, and 0.001, respectively). Rs420137 correlated with CHD risk in the allele (p = 0.047, OR: 1.29, 95% CI: 1.00–1.65), co-dominant (GG vs. CC p = 0.026, OR: 1.83, 95% CI: 1.01–3.31), recessive (p = 0.043, OR: 1.68, 95% CI: 0.95–2.99), and additive (p = 0.031, OR: 1.29, 95% CI: 1.00–1.65) models in individuals aged 62 years and younger.
Table 4 also shows that rs386360 had a higher risk of CHD under the co-dominant, recessive, and additive models (p = 0.027, 0.044, and 0.030, respectively) in patients aged ≤62 years. Rs7763726 had an increased risk of CHD in patients aged ≤62 years in the allele (p = 0.013, OR: 1.40, 95% CI: 0.14–1.08), co-dominant (p = 0.020, OR: 1.52, 95% CI: 1.08–2.15), dominant (p = 0.009, OR: 1.55, 95% CI: 1.12–2.16), and additive models (p = 0.008, OR: 1.42, 95% CI: 1.09–1.86).
Stratified analyses by smoking and drinking (Table 5) demonstrated that rs420137 was significantly related to a higher risk of CHD under the allele model in smokers (p = 0.006) and drinkers (p = 0.044), and under the dominant (p = 0.017), recessive (p = 0.036), and additive models (p = 0.006) in smokers. Rs386360 increased the risk of CHD in smokers (allele model: p = 0.004, OR:1.46, 95% CI: 0.13–1.13; co-dominant model with the “CA vs. AA” genotype: p = 0.045, OR: 1.43, 95% CI: 1.00–2.02; dominant model: p = 0.010, OR: 1.55, 95% CI: 1.11–2.16; recessive model: p = 0.032, OR: 2.08, 95% CI: 1.06–4.08; additive model: p = 0.003, OR: 1.49, 95% CI: 1.14–1.95) and non-drinkers (co-dominant model with the “CA vs. AA” genotype: p = 0.027, OR: 1.45, 95% CI: 1.04–2.01; dominant model: p = 0.028, OR: 1.43, 95% CI: 1.04–1.95). In addition, rs7763726 enhanced the incidence of CHD in smokers (allele model: p < 0.001, OR: 1.83, 95% CI: 0.14–1.38; co-dominant model with the “AG vs. AA” genotype: p < 0.001, OR: 2.13, 95% CI: 1.48–3.05; dominant model: p < 0.001, OR: 2.19, 95% CI: 1.55–3.10; additive model: p < 0.001, OR: 1.91, 95% CI: 1.42–2.57) and drinkers (allele model: p = 0.002, OR: 1.56, 95% CI: 1.18–2.06; co-dominant model with the “AG vs. AA” genotype: p = 0.002, OR: 1.75, 95% CI: 1.22–2.51; dominant model: p = 0.001, OR: 1.75, 95% CI: 1.24–2.46; additive model: p = 0.003, OR: 1.51, 95% CI: 1.15–1.98). Meanwhile, in Table 6, we found that rs420137, rs386360, and rs7763726 were protective factors against the occurrence of hypertension in CHD patients (rs420137: allele model: p = 0.004, OR: 0.70, 95% CI: 0.55–0.89; co-dominant model with the “GC” genotype: p < 0.001, OR: 0.53, 95% CI: 0.38–0.76; dominant model: p < 0.001, OR: 0.54, 95% CI: 0.38–0.76; additive model: p = 0.002, OR: 0.68, 95% CI: 0.53–0.87; rs386360: allele model: p = 0.005, OR: 0.71, 95% CI: 0.55–0.90; co-dominant model: p = 0.003, OR: 0.59, 95% CI: 0.41–0.83; dominant model: p = 0.001, OR: 0.58, 95% CI: 0.41–0.81; additive model: p = 0.002, OR: 0.67, 95% CI: 0.52–0.87; rs7763726: allele model: p = 0.004, OR: 0.69, 95% CI: 0.54–0.89; co-dominant model: p = 0.001, OR: 0.56, 95% CI: 0.40–0.79; dominant model: p = 0.001, OR: 0.57, 95% CI: 0.41–0.79; additive model: p = 0.002, OR: 0.67, 95% CI: 0.51–0.87). Nevertheless, after stratified by diabetes, no other SNP except rs7763726 was found in the recessive model (p = 0.011, OR: 0.29, 95% CI: 0.11–0.75) to reduce the risk of diabetes in CHD individuals (Table 6).
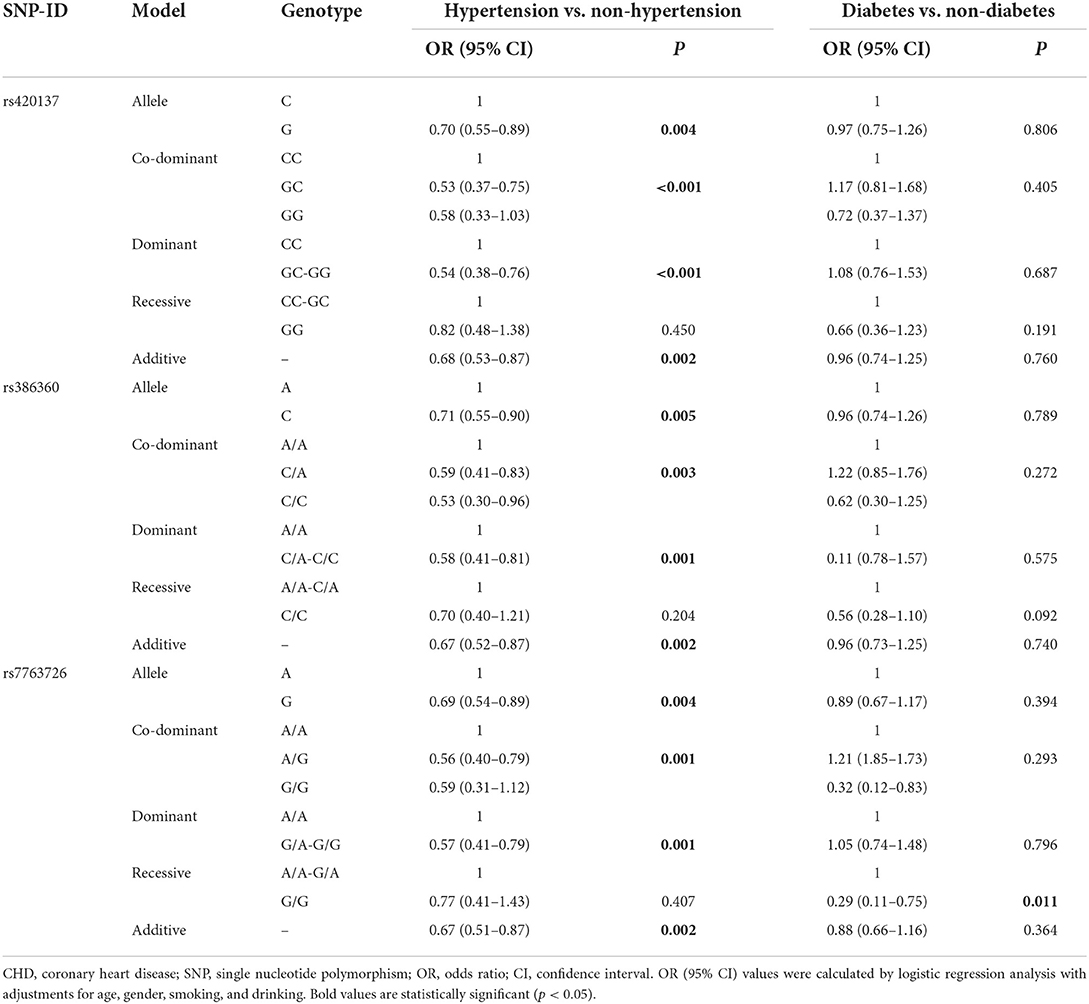
Table 6. FNDC1 SNPs associated with CHD risk stratified by hypertension vs. non-hypertension and diabetes vs. non-diabetes.
Haplotype and MDR analyses
Haplotype analysis was performed to analyze the association of FNDC1 polymorphisms with CHD risk, and one block including rs420137, rs386360 and rs7763726 was noted (Figure 1). Meanwhile, the connection between FNDC1 haplotypes and the risk of CHD is showed in Table 7. We found that the haplotype “GCG” (p = 0.002, OR: 1.34, 95% CI: 1.11–1.63) and the haplotype “CAA” (p = 0.004, OR: 1.29, 95% CI: 1.09–1.55) had a higher risk of CHD after adjustment.
MDR analysis showed that the best three-locus model was rs420137, rs386360, and rs7763726 (Bal. Acc. CV Training: 0.552; Bal. Acc. CV Testing: 0.548; CVC: 10/10; Testing Sensitivity: 0.647; Testing Specificity: 0.397; p = 0.0004, OR: 1.52; 95% CI: 1.20–1.92) (Table 8).
Discussion
CHD is a disease affected by many factors. Diverse environmental and genetic factors have been reported to be associated with the etiology of CHD (14). So far, scores of genetic studies have revealed that genes are related to the pathogenesis of CHD (15). It has been reported that FNDC1 can activate the cell surface receptor AGS8, regulate the intracellular distribution of vascular endothelial growth factor receptor 2 (VEGFR2), promote VEGF-A-induced vascular endothelial cell signal transduction and growth, promote tumor angiogenesis, and lead to increased tumor stage (16). Hence, we investigated the relationship between FNDC1 polymorphisms and CHD susceptibility by a case-control study. Our results indicated that FNDC1-rs420137, -rs386360, and -rs7763726 played an important role in enhancing the risk of CHD. Subgroup analysis showed that rs420137 increased the susceptibility to CHD in males, patients aged ≤62 years, and smokers. Rs386360 had a higher risk of CHD in males, patients aged ≤62 years, smokers, and non-drinkers. Moreover, the association of rs7763726 with increased CHD susceptibility was also revealed in males, patients aged ≤62 years, smokers, and drinkers. Last but not least, these three SNPs we selected were protective factors against hypertension in CHD individuals.
FNDC1 contains a major component of the fibronectin domain (17). Recent studies have shown that FNDC1 is closely linked to the occurrence of multiple illnesses (8, 18–21). It was also found that FNDC1 took part in VEGF-mediated endothelial cell angiogenesis, especially the distribution and transportation of VEGFR2, which had a strong tyrosine kinase activity and was a firsthand factor influencing angiogenesis (19, 22). The existing studies have also displayed that FNDC1 is a pathogenic gene of acute otitis media in children, and has a certain correlation with hypertension (8). In one study, the FNDC1 polymorphism (rs3003174) was associated with coronary artery aneurysm complications in Kawasaki disease (23). Previous study found that the expression of FNDC1 was up-regulated in cardiomyocytes induced by ischemia or hypoxia (24). Motohiko Sato et al. found FNDC1 isolated from rat hearts with repeated transient ischemia of collateral circulation may induce cardiomyocyte apoptosis under inflammatory or hypoxic conditions. Hypoxia induced FNDC1 mRNA expression in rat aortic smooth muscle cells, endothelial cells, or myocardial fibroblasts, suggesting a cardiomyocyte-specific adaptive mechanism including G protein signaling pathway remodeling (25). Bouchareb et al. (26) found that the mutations in the FNDC1 gene were associated with cardiovascular disease, indicating that FNDC1 polymorphisms may be involved in the development of CHD.
Sex and age are strong determinants of CHD risk (27), so is smoking (28). According to sex-stratified analysis, we noticed that the three SNPs in this study affected CHD susceptibility only in males, suggesting that there were gender differences in the influence of SNPs on the risk of CHD. At the same time, these three SNPs increased the risk of CHD in patients aged 62 years and younger and smokers. These results are consistent with previous studies indicating that genetic polymorphisms influence the susceptibility to CHD in males or patients aged ≤61 years (29, 30). Zhao et al. (31) found that the morbidity and mortality of cardiovascular diseases were correlated with the degree of blood pressure elevation. In our study, we observed that rs420137, rs386360 and rs7763726 could protect individuals with CHD from hypertension. These results indicate that patients with CHD need individualized treatment.
We need to emphasize that our study still has some limitations. First, our subjects were all Han Chinese. Second, the sample size was too small to validate the results. Third, CHD is a disease affected by a great number of risk factors. Therefore, we cannot completely exclude the potential impact of the other factors on its development.
Conclusions
In a word, this study indicated that FNDC1 polymorphisms (rs420137, rs386360, and rs7763726) were related to CHD risk, suggesting that these three variants may be new biomarkers for predicting CHD risk. To the best of our knowledge, this is the first study on the relationship between FNDC1 gene polymorphisms and CHD, and the specific mechanism of CHD still needs to be further studied.
Data availability statement
The original contributions presented in the study are included in the article/supplementary material, further inquiries can be directed to the corresponding author/s.
Ethics statement
This study was reviewed and approved by the Ethics Committee of the First Hospital of Yulin City, and conducted in accordance with the standards of the Declaration of Helsinki. Written informed consent was obtained from each participant included in the study.
Author contributions
HM contributed to the study design and performed statistical analysis. XH was responsible for manuscript preparation. XL and XD performed experiments. JH was responsible for checking the data. HZ and YZ contributed to data collection. All authors were responsible for drafting the manuscript, they have read, and approved the final version.
Acknowledgments
We thank all individuals for their participation. We are also grateful to the hospital staff for collecting blood samples and clinical information.
Conflict of interest
The authors declare that the research was conducted in the absence of any commercial or financial relationships that could be construed as a potential conflict of interest.
Publisher's note
All claims expressed in this article are solely those of the authors and do not necessarily represent those of their affiliated organizations, or those of the publisher, the editors and the reviewers. Any product that may be evaluated in this article, or claim that may be made by its manufacturer, is not guaranteed or endorsed by the publisher.
References
1. Mathers CD, Loncar D. Projections of global mortality, and burden of disease from 2002 to 2030. PLoS Med. (2006) 3:2011–30. doi: 10.1371/journal.pmed.0030442
2. Zhang XH, Lu ZL, Liu L. Coronary heart disease in China. Heart. (2008) 94:1126–31. doi: 10.1136/hrt.2007.132423
3. Fiatal S, Adany R. Application of single-nucleotide polymorphism-related risk estimates in identification of increased genetic susceptibility to cardiovascular diseases: a literature review. Front Public Health. (2017) 5:358. doi: 10.3389/fpubh.2017.00358
4. McPherson R, Tybjaerg-Hansen A. Genetics of coronary artery disease. Circ Res. (2016) 118:564–78. doi: 10.1161/CIRCRESAHA.115.306566
5. Li Q, Du Q. Associations between nine candidate genetic polymorphisms with coronary heart disease: a meta-analysis. Herz. (2020) 45:15–28. doi: 10.1007/s00059-019-4806-7
6. Vogel LK, Saebo M, Hoyer H, Kopp TI, Vogel U, Godiksen S, et al. Intestinal PTGS2 mRNA levels, PTGS2 gene polymorphisms, and colorectal carcinogenesis. PLoS ONE. (2014) 9:e105254. doi: 10.1371/journal.pone.0105254
7. Holan V, Trosan P, Cejka C, Javorkova E, Zajicova A, Hermankova B, et al. A comparative study of the therapeutic potential of mesenchymal stem cells and limbal epithelial stem cells for ocular surface reconstruction. Stem Cells Transl Med. (2015) 4:1052–63. doi: 10.5966/sctm.2015-0039
8. van Ingen G, Li J, Goedegebure A, Pandey R, Li YR, March ME, et al. Genome-wide association study for acute otitis media in children identifies FNDC1 as disease contributing gene. Nat Commun. (2016) 7:12792. doi: 10.1038/ncomms12792
9. Lapointe J, Li C, Higgins JP, van de Rijn M, Bair E, Montgomery K, et al. Gene expression profiling identifies clinically relevant subtypes of prostate cancer. Proc Natl Acad Sci USA. (2004) 101:811–6. doi: 10.1073/pnas.0304146101
10. To WS, Midwood KS. Plasma and cellular fibronectin distinct and independent functions during tissue repair. Fibrogenesis Tissue Repair. (2011) 4:1–17. doi: 10.1186/1755-1536-4-21
11. Williams LV, Veliceasa D, Vinokour E, Volpert OV. miR-200b inhibits prostate cancer EMT, growth and metastasis. PLoS ONE. (2013) 8:e83991. doi: 10.1371/journal.pone.0083991
12. Halper J, Kjaer M. Basic components of connective tissues and extracellular matrix: elastin, fibrillin, fibulins, fibrinogen, fibronectin, laminin, tenascins and thrombospondins. Adv Exp Med Biol. (2014) 802:31–47. doi: 10.1007/978-94-007-7893-1_3
13. Deng AY, Chauvet C, Menard A. Alterations in fibronectin type III domain containing 1 protein gene are associated with hypertension. PLoS ONE. (2016) 11:e0151399. doi: 10.1371/journal.pone.0151399
14. Mussatto KA, Hoffmann R, Hoffman G, Tweddell JS, Bear L, Cao Y, et al. Risk factors for abnormal developmental trajectories in young children with congenital heart disease. Circulation. (2015) 132:755–61. doi: 10.1161/CIRCULATIONAHA.114.014521
15. He B-X, LeiShi Qiu J, Tao L, Li R, Yang L, Zhao S-J, et al. Functional polymorphism in the CYP3A4 gene is associated with increased risk of coronary heart disease in the chinese han population. Basic Clin Pharmacol Toxicol. (2010) 108:208–13. doi: 10.1111/j.1742-7843.2010.00657.x
16. Sakima M, Hayashi H, Mamun AA, Sato M. VEGFR-3 signaling is regulated by a G-protein activator, activator of G-protein signaling 8, in lymphatic endothelial cells. Exp Cell Res. (2018) 368:13–23. doi: 10.1016/j.yexcr.2018.04.007
17. Gao M, Craig D, Lequin O, Campbell ID, Vogel V, Schulten K. Structure and functional significance of mechanically unfolded fibronectin type III1 intermediates. Proc Natl Acad Sci USA. (2003) 100:14784–9. doi: 10.1073/pnas.2334390100
18. Anderegg U, Breitschwerdt K, Köhler MJ, Sticherling M, Haustein U-F, Simon JC, et al. MEL4B3, a novel mRNA is induced in skin tumors and regulated by TGF-beta and pro-inflammatory cytokines. Exp Dermatol. (2005) 14:709–18. doi: 10.1111/j.0906-6705.2005.00349.x
19. Hayashi H, Mamun AA, Sakima M, Sato M. Activator of G-protein signaling 8 is involved in VEGF-mediated signal processing in angiogenesis. J Cell Sci. (2016) 129:1210–22. doi: 10.1242/jcs.181883
20. Sato M, Jiao Q, Honda T, Kurotani R, Toyota E, Okumura S, et al. Activator of G protein signaling 8 (AGS8) is required for hypoxia-induced apoptosis of cardiomyocytes: role of G betagamma and connexin 43 (CX43). J Biol Chem. (2009) 284:31431–40. doi: 10.1074/jbc.M109.014068
21. Wuensch T, Wizenty J, Quint J, Spitz W, Bosma M, Becker O, et al. Expression analysis of fibronectin type III domain-containing (FNDC) genes in inflammatory bowel disease and colorectal cancer. Gastroenterol Res Pract. (2019) 2019:3784172. doi: 10.1155/2019/3784172
22. Shibuya M. Vascular endothelial growth factor (VEGF) and its receptor (VEGFR) signaling in angiogenesis: a crucial target for anti- and pro-angiogenic therapies. Genes Cancer. (2011) 2:1097–105. doi: 10.1177/1947601911423031
23. Lin K, Zhang L, Wang Y, Li J, Xu Y, Che D, et al. FNDC1 polymorphism (rs3003174 C > T) increased the incidence of coronary artery aneurysm in patients with kawasaki disease in a southern chinese population. J Inflamm Res. (2021) 14:2633–40. doi: 10.2147/jir.S311956
24. Sato M, Cismowski MJ, Toyota E, Smrcka AV, Lucchesi PA, Chilian WM, et al. Identification of a receptor-independent activator of G protein signaling (AGS8) in ischemic heart and its interaction with Gbetagamma. Proc Natl Acad Sci USA. (2006) 103:797–802. doi: 10.1073/pnas.0507467103
25. Yuan C, Sato M, Lanier SM, Smrcka AV. Signaling by a non-dissociated complex of G protein βγ and α subunits stimulated by a receptor-independent activator of G protein signaling, AGS8. J Biol Chem. (2007) 282:19938–47. doi: 10.1074/jbc.M700396200
26. Bouchareb R, Guauque-Olarte S, Snider J, Zaminski D, Anyanwu A, Stelzer P, et al. Proteomic architecture of valvular extracellular matrix: FNDC1 and MXRA5 are new biomarkers of aortic stenosis. JACC Basic Transl Sci. (2021) 6:25–39. doi: 10.1016/j.jacbts.2020.11.008
27. Barrett-Connor E. Gender differences and disparities in all-cause and coronary heart disease mortality: epidemiological aspects. Best Pract Res Clin Endocrinol Metab. (2013) 27:481–500. doi: 10.1016/j.beem.2013.05.013
28. Hajar R. Risk factors for coronary artery disease: historical perspectives. Heart Views Offic J Gulf Heart Assoc. (2017) 18:109–14. doi: 10.4103/HEARTVIEWS.HEARTVIEWS_106_17
29. Chen X, Zhang Z, Wang X, Chen Y, Wang C. NT5C2 gene polymorphisms and the risk of coronary heart disease. Public Health Genom. (2020) 23:90–9. doi: 10.1159/000507714
30. Ma R, Zhu X, Bo Y. SCARB1 rs5888 gene polymorphisms in coronary heart disease: a systematic review and a meta-analysis. Gene. (2018) 678:S0378111918308862-. doi: 10.1016/j.gene.2018.08.024
Keywords: FNDC1, CHD, susceptibility, genetic polymorphism, SNPs
Citation: He X, Li X, Du X, Han J, Zhang H, Zhu Y and Ma H (2022) Rs420137, rs386360 and rs7763726 polymorphisms in fibronectin type III domain containing 1 are associated with susceptibility to coronary heart disease: Analysis in the Han population. Front. Cardiovasc. Med. 9:964978. doi: 10.3389/fcvm.2022.964978
Received: 09 June 2022; Accepted: 20 September 2022;
Published: 06 October 2022.
Edited by:
Olorunseun O. Ogunwobi, Hunter College (CUNY), United StatesReviewed by:
Priyanka Ghosh, The City University of New York, United StatesAmrita Bhat, University of Jammu, India
Copyright © 2022 He, Li, Du, Han, Zhang, Zhu and Ma. This is an open-access article distributed under the terms of the Creative Commons Attribution License (CC BY). The use, distribution or reproduction in other forums is permitted, provided the original author(s) and the copyright owner(s) are credited and that the original publication in this journal is cited, in accordance with accepted academic practice. No use, distribution or reproduction is permitted which does not comply with these terms.
*Correspondence: Honghong Ma, bWhoMTAwMSYjeDAwMDQwO3NvaHVjb20=
†These authors share first authorship