- 1Department of Medicine, McMaster University, Hamilton, ON, Canada
- 2Population Health Research Institute (PHRI), Hamilton, ON, Canada
- 3Thrombosis and Atherosclerosis Research Institute (TaARI), Hamilton, ON, Canada
- 4Department of Health Research Methods, Evidence, and Impact, McMaster University, Hamilton, ON, Canada
There is growing evidence that sex and gender differences play an important role in risk and pathophysiology of type 2 diabetes (T2D). Men develop T2D earlier than women, even though there is more obesity in young women than men. This difference in T2D prevalence is attenuated after the menopause. However, not all women are equally protected against T2D before the menopause, and gestational diabetes represents an important risk factor for future T2D. Biological mechanisms underlying sex and gender differences on T2D physiopathology are not yet fully understood. Sex hormones affect behavior and biological changes, and can have implications on lifestyle; thus, both sex-specific environmental and biological risk factors interact within a complex network to explain the differences in T2D risk and physiopathology in men and women. In addition, lifetime hormone fluctuations and body changes due to reproductive factors are generally more dramatic in women than men (ovarian cycle, pregnancy, and menopause). Progress in genetic studies and rodent models have significantly advanced our understanding of the biological pathways involved in the physiopathology of T2D. However, evidence of the sex-specific effects on genetic factors involved in T2D is still limited, and this gap of knowledge is even more important when investigating sex-specific differences during the life course. In this narrative review, we will focus on the current state of knowledge on the sex-specific effects of genetic factors associated with T2D over a lifetime, as well as the biological effects of these different hormonal stages on T2D risk. We will also discuss how biological insights from rodent models complement the genetic insights into the sex-dimorphism effects on T2D. Finally, we will suggest future directions to cover the knowledge gaps.
Introduction
Type 2 diabetes (T2D) is a metabolic disorder characterized by a combination of insulin resistance, (especially in adipose tissue, skeletal muscles and the liver), and relative insulin secretion deficiency (1). A wide variety of lifestyle factors, including excess body weight, smoking, and a sedentary lifestyle increase the risk of developing T2D (2). Globally, over 422 million individuals are affected by T2D worldwide and over 1.5 million deaths are annually attributed to T2D, ranking it among the top ten leading causes of mortality (3). Notably, epidemiological studies show that men develop insulin resistance and T2D earlier than women and at a lower BMI (4, 5). Premenopausal women have a reduced risk of developing T2D, compared to men or postmenopausal women (4, 6), but when women reach menopause, the risk becomes similar to that of men (7, 8).
Sex and gender differences play an important role in pathophysiology of T2D (4). Sex differences refer to biological differences, which are caused by differences in sex chromosomes, sex-specific gene expression, sex hormones, and their effects on organ systems. Gender differences refer to the effect of identities, expressions and societal roles and their implications on lifestyles. Both sex- and gender-specific biological and behavioral risk factors interact within a complex network to explain the differences in T2D risk and physiopathology in men and women. Polycystic ovary syndrome, gestational diabetes mellitus (GDM), and the age of menopause are three major woman-specific risk factors of T2D. For instance, women diagnosed with polycystic ovary syndrome typically have significant insulin resistance, regardless of body weight (9). Of women who had a history of GDM and were not given metformin or provided lifestyle interventions, almost 50% developed T2D within 10 years (10). T2D risk increases for women entering menopause before the age of 40, with a 30% increase in risk of T2D vs. women entering menopause from 50 to 54 years old (11). Moreover, women with T2D face an increased risk for cardiovascular disease that is at least two to four fold higher than the increase in cardiovascular disease risk seen in men with T2D (12). Premenopausal women without T2D are at a lower risk for cardiovascular disease than men without T2D of the same age, and much of this protection from cardiometabolic risk is thought to be due to the effects of estrogen, including estrogen receptor-mediated effects on lipid and glucose metabolism, endothelial function, and fat deposition (13). Estrogen appears to be cardioprotective unless T2D is present, but after the menopause, the protective effects are lost as estrogen deficiency develops (12).
From a biological perspective, T2D pathophysiology is partially driven by genetic factors (14). Thereby, progress in genetic studies have facilitated the identification of more than 300 loci associated with glucose-related variables and T2D. These studies have led to a better understanding of the biological pathways involved, as well as the common underlying biological mechanisms linking polycystic ovary syndrome and T2D (15), or GDM and T2D (16). Several rodent models of diabetes exhibiting an exaggerated form of sexual dimorphism have also been useful to delineate the mechanisms underlying the protection against T2D conferred by estrogens.
In this narrative review, we will focus on the current state of knowledge on the sex-specific effects of genetic factors associated with glucose-related traits, insulin resistance and T2D during the life course. Given that sex hormones dramatically change during a women's lifetime (e.g., ovarian cycle, pregnancy, and menopause), we will discuss the biological effects of these different hormonal stages on type 2 diabetes risk. We will further discuss how rodent models provide evidence on the effects of sex-dimorphism on type 2 diabetes risk. We will finally suggest future directions to cover the current knowledge gaps.
Genetic evidence for sexual dimorphism in type 2 diabetes
Regulatory differences in blood glucose and insulin levels are both heritable traits. When estimated separately, the single nucleotide polymorphism (SNP)-based heritability (17) for T2D is significantly higher in men than in women (18), which suggests differences in the underlying genetic determinants of T2D risk due to sex-dimorphic effects in specific genes (or loci).
Given the complex nature of T2D related traits, Genome wide association studies (GWAS) have been one of the most powerful approaches used to identify new loci. While the methodology has essentially remained unchanged since the publication of the first T2D GWAS (19), the list of associated genes has considerably expanded due to the increase in sample size, and the inclusion of samples of multiple ancestries (20, 21). However, the proportion of variance in glucose related traits is still not fully explained by the currently known loci, and gene × gene and gene × environment interactions are thought to contribute to this missing heritability, with gene × sex interactions being one of these modulators. Three main statistical approaches have been used to date to investigate sex-dimorphic effects. The first approach involves performing sex-stratified analysis, followed by a heterogeneity test of effect between the two sexes at each genetic locus. The second approach consists in performing a single analysis with sex and SNP × sex interaction terms in the statistical model tested (22). The third, referred to as a sex-differentiated test, combines data for both sexes in a single analysis, includes the number of X-chromosome copies (1 or 2) as an independent variable in the model and allows for testing heterogeneity of allelic effects between males and females at the cost of one degree of freedom (22). Each one of these approaches have their own limitations as to the ability and power to detect sex-dimorphic effects (19, 20). However, one major advantage of the first approach is the possibility of testing for sex-related heterogeneity using summary statistics of publicly available sex-stratified data, while the other two methods require individual level data.
The spectrum of sex-dimorphic effects varies from (i) different (significant) direction of effects between males and females, (ii) similar (significant) direction of effects with differences in magnitude of the effect between sexes (e.g., larger effect sizes in one of the two sexes), to (iii) sex-specific effects whereby the association is significantly observed in one of the sexes only; this last case scenario being an extreme example of the second. It is important to note that the distinction between these three cases heavily relies on the statistical method, power, and parameters used to determine the significance of a SNP-T2D association in each sex. Hence, intermediary/inconclusive scenarios that do not clearly fit in the above-mentioned categories can often be observed (e.g., a SNP with a GWAS significant association (p-value < 5 x 10−8) in one sex and suggestive association only (p-value < 1 × 10−6) in the other. Therefore, balanced sample sizes in the two sex groups (and hence a comparable statistical power) is a crucial component for sex-comparison analyses.
Human genes/loci with sex-dimorphic effects on glucose related-traits
Primary evidence of sex-specific effects in glucose metabolism-related genes/loci are rare, and replication attempts are even more sparse, due to the lack of power concomitant to the sex-stratified or the sex-interaction analyses. A large proportion of genes contributing to sex-dimorphic effects on FG and FI were described in a recent study lead by Lagou et al. (23). The authors conducted genome wide association studies (GWAS) in over 140,000 (for FG) and 98,000 (for FI) adult normoglycemic men and women (separately) of European ancestry from the Meta-Analyses of Glucose and Insulin-related traits Consortium (MAGIC). GWAS results were subsequently meta-analyzed and the heterogeneity of allelic effects between the two sexes was estimated (23). A targeted analysis for 36 and 19 previously established FG and FI loci that aimed to detect sex-dimorphic effects in these loci, was also conducted. To date, this study is largest one to investigate the modulating effect of sex on the genetic determinants of glucose-related traits on a genome wide level, complemented with genetic correlations and gene expression analysis. The absence of replication data represents the main limitations of this study.
Given the lack of replication studies and the weak significance of the associations in most studies, we investigated the sex-dimorphic effects of the autosomal genes/loci described in the literature with glucose-related traits [e.g., fasting glucose (FG), fasting insulin (FI), T2D], other correlated anthropometric traits including body mass index (BMI), waist to hip ratio (WHR), and GDM, using publicly available data from large genetic consortia, in order to identify the candidate genes with the most robust evidence of sex-dimorphic effect (spanning across multiple phenotypes). The list and description of genes/loci with the strongest evidence of sexual dimorphism across multiple traits is presented in Table 1. Results of an assessment of the sex-specific effects for each gene/locus on anthropometric and glucose-related traits is presented in Tables 1, 2.
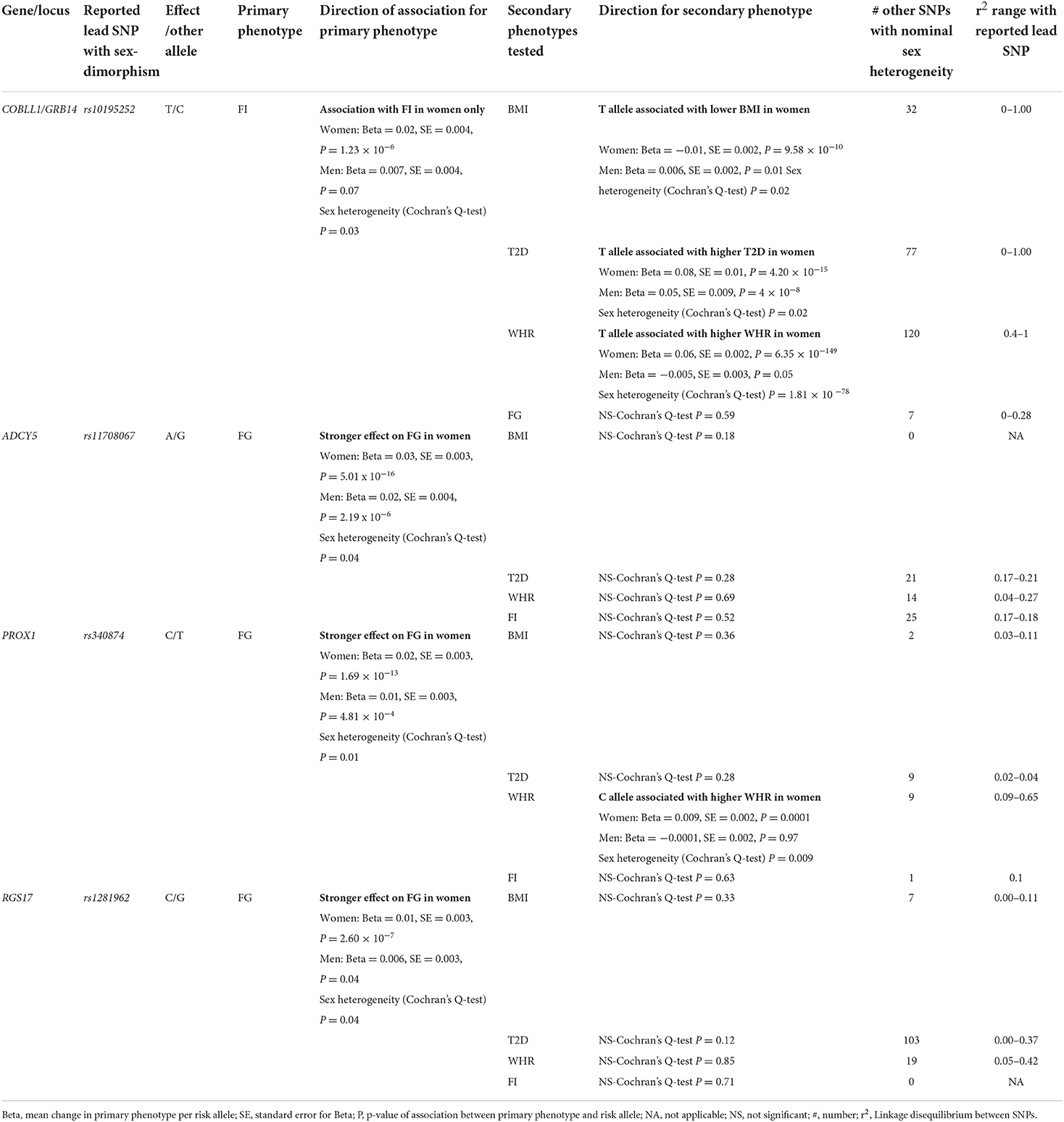
Table 1. Associations of sex dimorphic effects of most important genes identified to date on type 2 diabetes and related phenotypes.
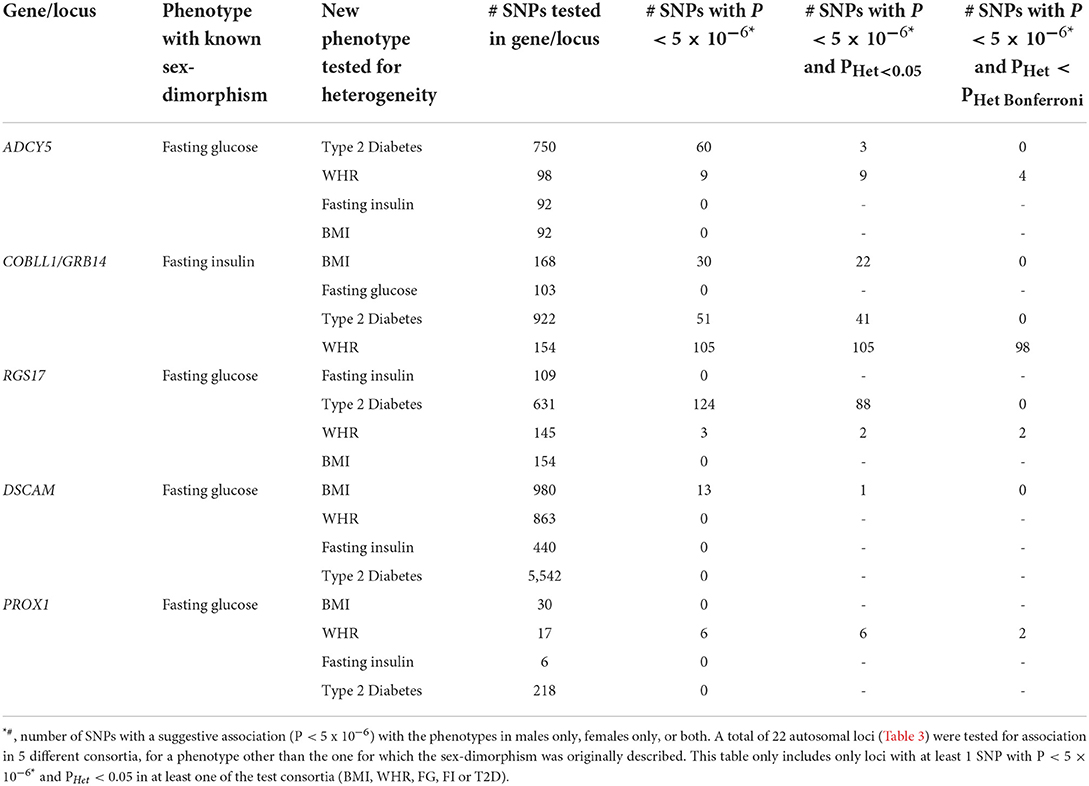
Table 2. Summary of cross-phenotype sex-heterogeneity tests in BMI, WHR, T2D, FG, and FI consortium data at genetic loci with previously known sex-dimorphism.
Genes identified in childbearing women
Autosomal genes
COBLL1/GRB14 locus
The locus which includes growth factor receptor bound protein 14 (GRB14) and cordon-bleu WH2 repeat protein like 1 (COBLL1) genes, has the strongest evidence of sexual dimorphism across multiple traits. GRB14 encodes for a protein that binds to insulin, inhibiting its signaling activity (24). The biological function of COBLL1's protein is unclear beyond its possible involvement in the Wnt/PCP pathway regulation in mammals (that regulates crucial aspects of cell fate determination, cell migration, cell polarity, neural patterning and organogenesis during embryonic development) (25), and its association to multiple metabolic traits and tumor types. SNPs in this gene displayed nominal evidence of sex-differences, with an association with FI observed in females only (23). The FI increasing allele of the lead SNP described by Lagou et al. (23) (rs10195252) was also significantly associated with a lower BMI and WHR in women only (effect of the same SNP in men was weaker and nominal) (Table 1). The same FI increasing allele (T) was associated with an increased risk of T2D in a female-specific manner (Table 1). Sex-differences influencing WHR (23–25), triglycerides (26) and T2D (27) at this locus have also been described in the literature. Finally, gene expression of COBLL1 was higher in women's gluteal fat, abdominal fat and whole blood, while men had a higher expression than women in the liver (23). Levels of GRB14 were nominally higher in women's gluteal fat. Levels of GRB14 in abdominal fat were similar between men and women (23). Given all these results, it is possible that an effect of variants within/near to COBLL1 and GRB14 could influence glucose metabolism via adipose tissue and body fat distribution differences between men and women. More studies are needed in order to establish this link.
ADCY5 gene
In sex-combined studies, SNPs in the adenylate cyclase 5 (ADCY5) gene are associated with multiple T2D-related traits including FG (23, 28–32), FI (21), glycated hemoglobin (33), HOMA-B (i.e., index of insulin secretion) and T2D (34–43), anthropometric and body fat distribution traits, such as WHR (44, 45), body fat percentage (46), BMI (47), inflammation phenotypes such as C-reactive protein (48), early life factors including gestation duration (49), birth weight (50–56), blood lipid levels, including total cholesterol (57, 58), HDL-cholesterol, apolipoprotein A1 (59), and blood pressure measures (60). SNPs in the vicinity of ADCY5 showed sex-dimorphic effects in association to FG. The lead SNP associated with FG described by Lagou et al. (23) (rs11708067) did not show evidence for sexual dimorphism when tested for FI, T2D, WHR or BMI (Table 1). Nevertheless, another variant in this gene likely independent from the aforementioned SNP (rs3934729), shows nominal sex-dimorphic effect with a stronger effect in women (Table 1). The sex-dimorphic effect of rs11708067 on T2D risk was also previously described in the literature (27). In a gene expression analysis, ADCY5 SNPs were associated with levels of sex hormone binding protein (SHBG) (61), which provides a possible clue as to the mechanisms and pathways involved in ADCY5's sex-specific effects.
PROX1 gene
The prospero homeobox 1 (PROX1), which encodes for the Prospero homeobox protein 1 transcription factor plays a key role in embryonic cellular development and differentiation in multiple organs of complex organisms including Drosophila, mice and humans (62, 63). On a molecular level, PROX1 was mostly studied for its prominent role in lymphatic endothelial cell fate determination of which it is generally considered as the master regulator (64). Mutations in PROX1 have been associated with multiple forms of cancer. In human adult GWASs, PROX1 genetic variants have been overwhelmingly associated with glycemic traits overall (65), blood glucose levels (23, 30, 31, 66, 67), glycated hemoglobin levels, HOMA-B, T2D (32, 34–37, 39, 43), and to a lesser extent to birth weight (51), and other cardiometabolic traits such as triglycerides (68) or WHR (suggestive association only) (45). As an established FG locus, Lagou et al. (23) tested and observed nominal evidence for sex heterogeneity in multiple PROX1 genetic variants on FG, where the associations were stronger in women. This potentially sex-dimorphic FG SNP described by Lagou et al. (23) (rs340874) did not show evidence for association with BMI but nominal association with FI in both sexes, with no significant sex-related heterogeneity. However, the FG increasing allele (T) was associated with higher WHR in women only, with a significant heterogeneity. Multiple other SNPs located next to this locus showed nominal sex-dimorphic effects on WHR (Table 1). Possible clues as to how PROX1 could influence glycemic traits in a heterogenous manner between men and women come from other GWASs. Indeed, SNPs in the vicinity of PROX1 and its neighboring non-protein coding PROX1 Antisense RNA 1 (PROX1-AS1) genes have been shown to be associated with SHBG levels in both men and women (61, 69). However, the sex-dimorphic SNP in PROX1 (rs1281962) described by Lagou et al. (23) was not associated with SHBG levels and more investigations are required in order to establish a connection between sexual dimorphism for SNPs near PROX1, SHBG, and FG. PROX1 SNPs were also associated with testosterone levels at a GWAS-significance level in men, but not in women, which could suggest a sex-dimorphic effect (61). Whether the PROX1 plays a differential role in glucose related traits through its regulation of SHBG and testosterone levels remains to be determined.
RGS17 gene
The regulator of G protein signaling 17 (RGS17) gene and its corresponding RGS17 protein are involved in the regulation of multiple G protein-coupled receptor signaling cascades (70). SNPs in RGS17 displayed strong associations with HDL-cholesterol (32, 57–59), triglycerides, apolipoprotein A1, FG (21, 23, 31), glycated hemoglobin, T2D (34), WHR (45), BMI (39, 44, 47, 71, 72), diastolic blood pressure (60), and C-reactive protein. Multiple SNPs in the RGS17 gene revealed larger effects on FG in women at nominal significance (23). The FG-increasing allele in the lead SNP was associated with higher BMI in a GWAS meta-analysis with larger effects in women (45). The lead SNP (rs1281962) did not show evidence for sexual dimorphism on BMI, T2D, FI or WHR (Table 1). However, two other SNPs in RGS17 gene (rs3910736, rs514784) showed a weak association with WHR in women only (Table 1). However, these sex-dimorphic effects were not significant after correction for multiple hypothesis testing. No significant interaction was detected at this locus (72). A nominally significant heterogeneity effect was observed against T2D (Table 3). Given its pleiotropic role on cardio-metabolic traits, the mechanisms by which RGS17 might differentially affect phenotypes remains unclear.
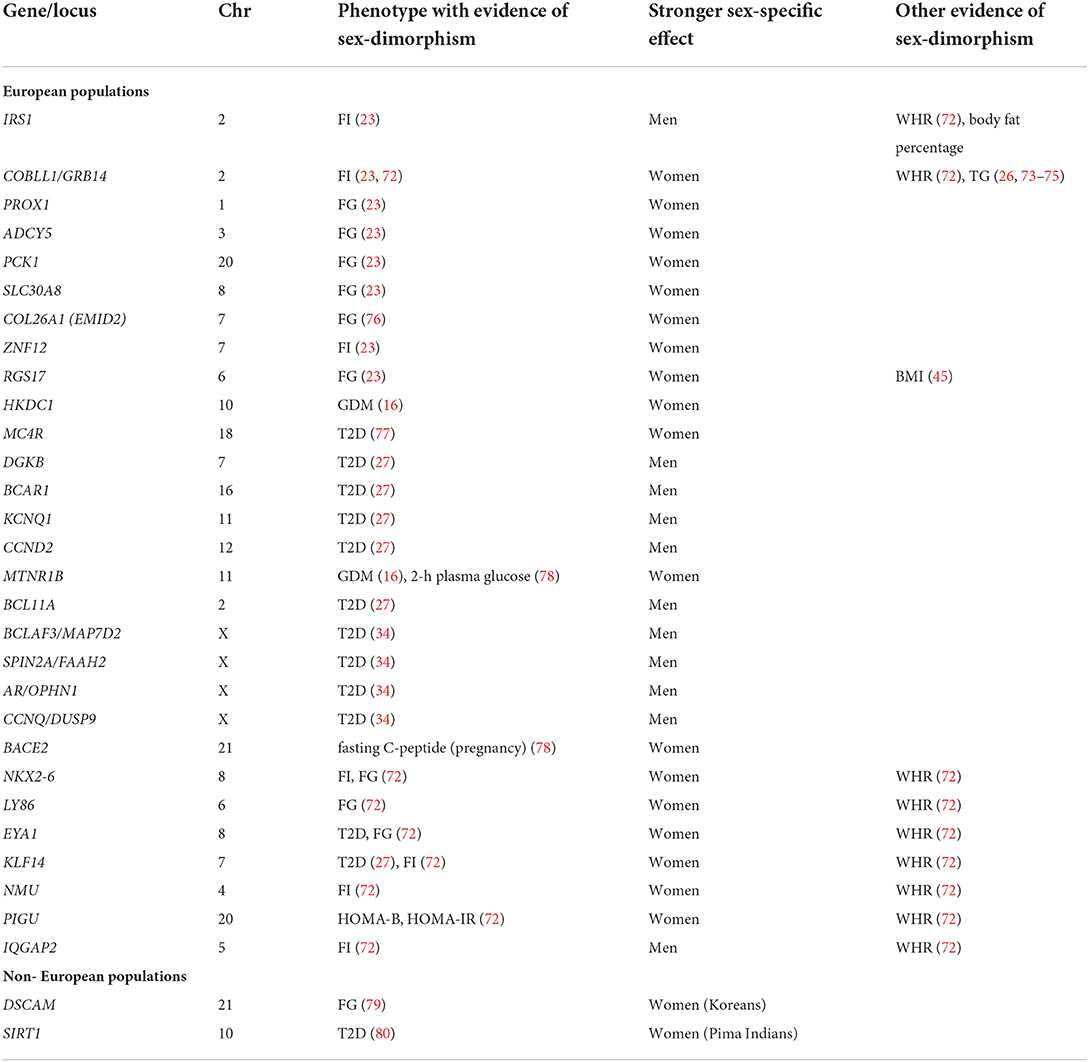
Table 3. Genes/loci with suggestive evidence of sexual dimorphism on type 2 diabetes and related phenotypes.
Genes on chromosome X
Only few GWASs of glucose- and T2D-related traits have analyzed and identified chromosome X genetic variants (32, 34, 43, 81–83). This is partially due to the difference in the number of copies of sex chromosomes between men and women, which requires them to be analyzed separately from the autosomes. However, given the male heterogamety nature of sex determination in humans, and that the genes coding for some major regulators of sex steroids levels are located on this chromosome [e.g., sex hormone binding globulin (SHBG) and androgen receptor (AR)], loci on Chr X are candidates for gene × sex interactions. Among the studies that identified and validated the largest number of chromosome X variants directly associated with T2D, there was an analysis lead by Vujkovic et al. (34). This study included participants from multiple ancestries and identified a total of ten T2D loci on chromosome X (34). Among these, SNPs at the AR/OPHN1 locus which displayed male-specific effects (non-significant in females) on T2D risk (Table 3). AR is an interesting biological candidate since this codes for the steroid hormone and transcription factor androgen receptor implicated in the expression of multiple male sexual development and differentiation genes under the control of testosterone (84). SNPs in AR have been associated with fasting insulin levels, and with baldness in males (34). The mechanism by which the AR gene indirectly influences results in sex-dimorphic phenotypes in relation to glucose metabolism still needs to be investigated.
Genes identified during pregnancy
Given the female-specific aspect of pregnancies, genes associated with GDM during the unique physiological state of pregnancy can be considered as sex-dimorphic. GDM is thought to be closely related to T2D from a genetic perspective. T2D polygenic risk score has been strongly associated with risk of GDM, although only a few GDM GWASs have been performed (16, 78, 85). The following genes have been identified.
HKDC1 gene
Although the majority of GDM associated genetic loci were previously known for their association with T2D or glycemic traits (86, 87), two GWASs reported hexokinase domain containing 1 (HKDC1) SNPs to be associated with GDM (78), and 2h-post load glucose test (16). HKDC1 also appears to influence other early life factors, including maternal genetic effect on birth weight (51). This locus was not known for association with glycemic traits or T2D in non-gravid populations except for glycated hemoglobin (31). However, SNPs in HKDC1 were significantly associated with SHBG levels in sex-combined and women only groups, but not in males (61). Other traits associated with HKDC1 are mostly related to multiple blood cell count phenotypes (32, 88–91) and liver function [e.g., alanine aminotransferase (31, 92, 93), and aspartate aminotransferase].
MTNR1B gene
The melatonin receptor 1B (MTNR1B) gene is a well-established T2D locus with significant associations with multiple glucose-related traits including FG (21, 23, 28–30, 67, 94–101), glycated hemoglobin (31–33, 102, 103), insulin levels, insulin disposition index and insulin secretion rates (104, 105), acute insulin response, HOMA-B (21), and T2D (27, 34, 35, 37–40, 42, 43, 106, 107). The gene is also associated with various sleep and circadian rhythm-related phenotypes (108, 109). Given the strong association with T2D, it is not surprising that this gene has also been associated with GDM in several GWASs (16, 110). Despite the absence of evidence of sex-dimorphic effects in MTNR1B, this locus is of particular interest given that the effect size of MTNR1B SNPs are higher in GDM than T2D (16), which suggests a female-specific effect of this locus during pregnancy.
Insights from genetic studies in rodents
The use of rodent models in the study of human disease is an important component of translational knowledge. It has the dual function of either confirming what was observed in human genetic studies or provide more information on the mechanisms by which specific genes can be associated with the development of T2D. Although wild type rodent models do not spontaneously develop diabetes, the condition can be induced genetically or chemically. Examples of human genes whose functional implication in T2D has been validated in mice are numerous. For instance, a human GWAS analysis showed that SLC30A8's SNPs are associated with susceptibility to T2D. Subsequent studies showed that the deletion its mouse homolog Slc30a8 induced defects in insulin secretion and an overall impairment in glucose homeostasis (111).
The implication of genetics factors in diabetes-related sex-dimorphic effects in rodents is evidenced by the fact that different strains, with different genetic backgrounds, display different sex-dimorphic phenotypes in otherwise similar environments. The characteristics of the different models that display sex-dimorphism phenotypes are provided in Table 4. Models with monogenic forms of diabetes provide direct evidence of the involvement of a gene in these sex-dimorphic outcomes. A clear illustration of this is the Zucker Diabetic Fatty (ZDF) rats, a widely used model of obesity caused by the mutation of the leptin receptor gene (Lepr, also known as Fa) (123). Male ZDF develop hyperglycemia, hyperinsulinemia, impaired glucose tolerance, while females are normoglycemic (124) (Table 4). This highlights the differential involvement of the Lepr gene and the Leptin/Melanocortin pathway in the development of diabetes between males and females. Another interesting rodent model is the aromatase-knockout (Arko) mice, which result from a targeted disruption of Cyp19A1, a gene that encodes for the aromatase, an enzyme involved in the production of endogenous estrogen (113). Cyp19A1 knock down mice display a range of sex-dimorphic phenotypes as a result. Among these, male ArKO mice show signs of insulin resistance and impaired glucose homeostasis, whereas females develop glucose intolerance, but not insulin resistance (113). The importance of estrogen and all actors involved in its metabolism will be discussed below.
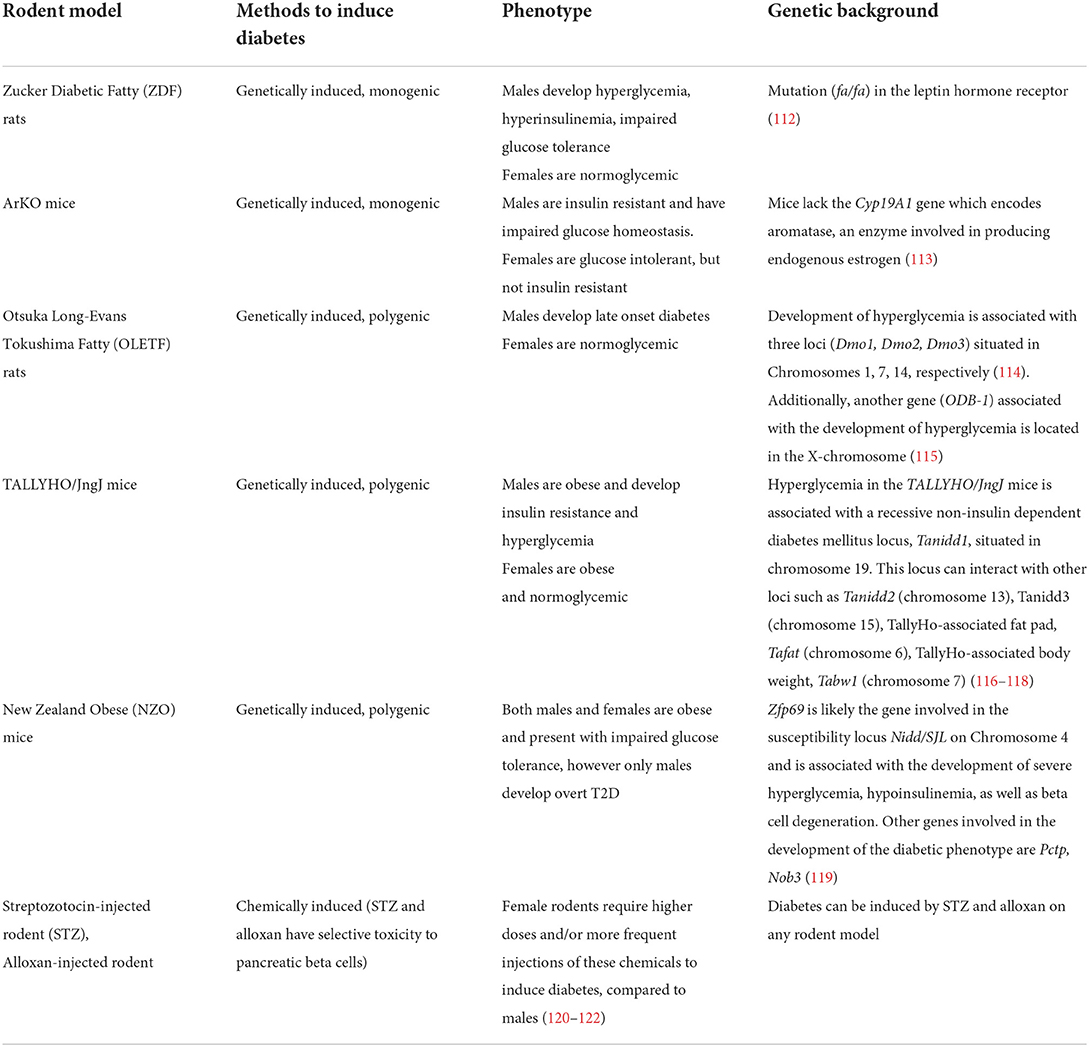
Table 4. Genetically and chemically induced rodent models of T2D showing sexual dimorphism in glucose homeostasis.
Rodent models of polygenic forms of diabetes also exist, with some models displaying more sex-dimorphic traits than others (Table 4). However, like human studies, the identification of the genes involved in sex-dimorphic phenotypes in these polygenic and more complex forms of diabetes is more difficult and has been poorly studied in rodents. However, given the recent advances in gene editing and other functional genomic tools, several new models have been specifically developed for the study the genetic causes of sex-dimorphic traits (124, 125). Among the most interesting candidates is a mouse for which the sex determining region of Chr Y (Sry locus) involved in male sex determination is transferred from chromosome Y to chromosome 3 in a male mouse, therefore detaching gonadal development from sex chromosomes (126). Male mice with this manipulation are then crossed with female mice carrying two X chromosomes. The resulting offspring can be an XY or XX carrying Sry in chromosome 3 (corresponding to a model where the effects of sex hormones are independent from the gonadal status), or XY and XX without this manipulation (126). In a recent study, these mice underwent gonadectomy and were subsequently supplemented with either estrogen, testosterone, or a blank control (126). It was observed that in these mice, estrogen and testosterone decrease the gonadal regulation of gene expression in the liver, whereas they enhance it in the adipose tissue. Furthermore, the effect of estrogen is more prominent than that of testosterone. It was also observed that sex chromosomes seem to regulate the expression of the Hccs gene, which is involved in the regulation of multiple metabolic pathways. Additionally, the study showed that genes affected by sex hormones in the adipose tissue are highly enriched with variants associated with cardiometabolic diseases and traits (126).
The study just described is an example of how rodent models can be used to decipher the mechanisms by which various sex-related components can individually affect gene expression at the basis of sexual dimorphism observed in glucose-related traits.
Effects of sex hormones on glucose and T2D-related traits
In humans
Although there is no study conducted in humans to investigate the interaction of circulating sex hormone levels with genetic factors on T2D risk, overall evidence shows that circulating levels of sex-hormones directly or indirectly modulate the effects of the genetic susceptibility for T2D, through different pathways and mechanisms. First, estrogen regulates body composition and fat distribution. In women, high estrogen level is usually associated with less ectopic fat deposition, more favorable lipid profile, and less insulin resistance than in men (6). Premenopausal women store fat primarily in gluteofemoral depots, which are considered benign or metabolically beneficial, whereas men tend to store fat in abdominal depots (6). In addition, estradiol has a beneficial impact to decrease visceral adipose tissue and increase brown adipose tissue (127). During female-specific age-related transitions, estrogen loss leads to decreased physical activity, increased adiposity with redistribution of fat to abdominal depots (128), and decreased muscle mass, whereas estrogen replacement reverses these changes (6). Second, excess of androgens and lower levels of SHBG have a direct effect on insulin resistance and T2D (129). Interestingly, sex hormones differentially modulate glycemic status and risk of T2D in men and women. High testosterone levels are associated with higher risk of T2D in women but with lower risk in men; the inverse association of SHBG with risk seems to be stronger in women than in men (130). Moreover, in women, low SHBG levels predict higher T2D risk, regardless of BMI and age (130). However, the causal relationships between low SHBG levels and T2D risk have been reported similarly in both sexes (131), though an effect on insulin resistance (132). Interestingly, lower estradiol level in men predicts lower risk of T2D (133).
Evidence on the effects of sex hormones on overall genetic susceptibility for T2D also comes from the usage of hormone replacing therapies (HRT) administered to prevent consequences of menopause including vasomotor/genitourinary symptoms and osteoporosis (134). Several clinical studies have demonstrated that HRT is beneficial for glucose homeostasis (135–138). Evidence provided by the North American Menopause Society/American College of Cardiology/American Heart Association, suggest that in women of < 60 y-old, within 10 years after menopause onset, menopausal hormone therapy with estrogens (combined or not with progestogen) may be beneficial for the prevention of coronary disease and may also reduce the incidence of T2D (139). In post-menopausal women with T2D, menopausal hormone therapy improves glycemic control, and insulin sensitivity (139), by improving β-cell insulin secretion and insulin sensitivity (137).
The study of transgender individuals also provides a unique opportunity to determine which metabolic functions are modulated by the prevailing milieu of sex steroids, because the chromosomal configuration remains unchanged (140). Usage of estrogen therapy in transgender women, as a feminizing hormonal therapy for individuals assigned male sex at birth, showed a significant effect on body composition within 12 months and these changes persist over the time, such as transgender women on estrogen lost lean mass and gained fat mass (141). Estrogen and antiandrogen therapy seemed to be associated with an increase in the absolute amount of visceral fat and subcutaneous fat, but a reduction in the ratio of visceral to subcutaneous fat (142). Effects on insulin sensitivity are controversial. Although transfeminine people may be at higher risk for T2D compared with cisgender women, the corresponding difference relative to cisgender men was not discernable in the STRONG cohort (143). There was little evidence that T2D occurrence in either transgender women or transgender men was attributable to gender-affirming hormone therapy use (143). Data from another large gender identity study suggested that both transgender men and women exhibited higher incidence of T2D than the general population (144), with a higher CV mortality rate among transwomen but not among transgender men. Despite receiving similar estrogen therapy, transgender women who elected orchiectomy had improved metabolic health compared with transgender women who retained their testes. Furthermore, data suggest that suppression of endogenous testosterone in transgender women appears to improve insulin sensitivity and reduce hepatic steatosis (145). Thus, the implications for long-term T2D incidence or cardiovascular health are still unclear, due to a paucity of long term prospective controlled studies. It should also be noted that most of the participants in these studies are of white European ethnicity, which limits the generalizability of the findings to transgender individuals of other ethnic groups.
In rodents
A wide variety of interventions have been used to study the impact of hormonal changes on sex differences in the presence of T2D in rats and mice, with overall results that validate and strengthen the observations made from human studies. Both chemical and surgical approaches can be used to mimic menopause in any rodent model of choice (146). Chemically induced menopause can be attained by exposing rodents to the chemical 4-vinylcyclohexene diepoxide (VCD), which gradually depletes ovarian follicles and therefore mimics the perimenopausal and menopausal stages (147). It has been shown that the loss of ovarian function is associated with an increase in insulin resistance, the development of metabolic syndrome, and T2D (148). Interestingly, another study in VCD mice showed that hyperglycemia was significantly more severe in VCD female mice post-ovarian failure, compared to cycling females (149). Surgical menopause through ovariectomy, which induces immediate estrogen depletion, is the most used models to induce menopause in rodents. The detrimental effects of ovariectomy on glucose homeostasis have been analyzed in several mouse models of diabetes. For instance, female normoglycemic ZDF rats, showed impaired glucose homeostasis after ovariectomy (123). Ovariectomized female New Zealand obese (NZO) mice, who otherwise rarely develop diabetes (150), display severe hyperglycemia with a significantly higher prevalence compared to sham operated controls (151). Similar observations can be made in Wistar rats and C57BL/6J mice, two other rodent models that are typically normoglycemic, but where ovariectomy can impair their glucose homeostasis.
Estrogen supplementation, another commonly used chemical procedure in rodents, has been shown to restore glucose homoeostasis in ovariectomized female ZDF rats (123), and improve glucose tolerance and fasting blood glucose levels in males (152). Estrogen replacement reversed insulin resistance and visceral fat accumulation, and improved insulin sensitivity in the skeletal muscle (when combined with high fat diet) in ovariectomized female Wistar rats (153, 154). Estrogen supplementation in ovariectomized female C57BL/6J mice significantly improved blood glucose levels, glucose-stimulated insulin secretion as well as insulin content in pancreatic beta cells (155) and improved insulin sensitivity in the hepatic tissue (156). Finally, in the ArKO mice, estrogen replacement also significantly improved glucose tolerance and insulin sensitivity in both males and females, further indicating the protective role of estrogen in glucose homeostasis (113).
Of note, rodent studies show that the impact of sex hormones, combined to the exposure to environmental risk factors can lead to sexual dimorphic phenotypes as early as in utero. For example, in Sprague-Dawley rats, a multipurpose rodent model typically used in studies of metabolism and diabetes, male offspring born to mothers with diet-induced GDM had altered expression of genes associated with pancreatic growth, reduced beta cell expansion and differentiation, and impaired insulin secretion (157). Sex differences in glucose homeostasis of offspring born to C57BL/6J mice with diet-induced GDM have also been observed (158). Specifically, male offspring were more insulin resistant, had higher plasma insulin levels, and had smaller pancreatic islets compared to female offspring. Additionally, the expression of PDX-1 which is involved in pancreatic beta cell maturation was significantly reduced in males, compared to female offspring. These data show that estradiol might exert protective effects in the metabolic homeostasis of female offspring.
Taken together, these studies illustrate how rodent models can help to recapitulate and pinpoint the mechanistic effects at the basis of sexual dimorphism as well as how they can be a valuable and complemental tool to inform genetic analysis on this specific area of research.
Gender-specific genetic determinants for T2D risk
Gender-specific lifestyle behaviors including unhealthy diet, low physical activity and smoking are well-recognized risk factors for T2D that differ between gender (4), yet studies on interaction between gender-specific lifestyle behaviors and genetic determinants on T2D risk are still lacking, for the main reason that such studies need a very large sample size to reach the power required to detect significant effects. It has been shown that men are more likely to smoke than women (159, 160); women are more likely to consume a healthy diet (161, 162), but are less active than men [global average 32% for inactive women vs. 23% for inactive men (163, 164)]. Diets enriched in ultra-processed foods and sugar-sweetened beverages are associated with an increased risk of developing T2D (165), while diets enriched in fruits, whole grains, and dairy products are associated with a decreased risk of T2D (166). Moreover, the influence of lifestyle factors may exert their effects differently along the life stages.
Knowledge gaps and conclusions
Most of GWASs studying the sex-dimorphic effects of genes on T2D risk have been conducted on populations of European ancestry. To date, the list of genes displaying sex dimorphic effects in non-European populations is extremely limited and includes SIRT1, identified in a population of Pima Indians (80), and DSCAM in Koreans (Table 3). However, the replication of sex-dimorphic effects at these loci is still needed, and larger, more diverse studies are still needed in order to identify more sex-dimorphic loci and increase our understanding on the interplay between genes, sex and the risk of diabetes.
Sex and gender consideration in research studies has improved over the last 20 years, but preclinical research is still primarily done using male rodent models and male-derived cells, with the result that many conclusions are made based on incomplete and sex-biased data (167). Although sex-specific data can improve disease prevention, diagnosis, and treatment as well as reduce inequities, research to address the important goal of understanding key sex differences in cardiometabolic disease across the lifetime is still lacking. More studies are required to identify the mechanisms responsible for the sex-specific increase in cardiometabolic risk and to develop therapies that are safe and effective in women. Such research should take into account biological and behavioral factors that differ between women and men, including unique exposures in women, such as hormonal fluctuations across the life time from conception through aging (167) (Figure 1). Critical gaps and research priorities should include elucidation of the mechanisms whereby sex hormones regulate body composition, fat distribution and how they interact with diet and age, how are sex differences and race/ethnicity interrelated with T2D what are the mechanisms underlying the apparent paradox of effects of sex hormones in premenopausal women vs. postmenopausal women vs. men, what are the sex differences in therapeutic treatments and effects of T2D drugs, cardiovascular disease outcomes, and what are the metabolic impacts of hormonal replacement therapy, and of androgen and estrogen use in the context of biologically different sex. Previously published reviews on T2D genetics did not address the sex-specific differences on T2D genetic susceptibility. Our review is the first to synthesize the data on sex differences in relation to its effects with genetic variants on T2D and related traits. These offer a solid basis for future research in this field. Ultimately, expanding our understanding on why risk of T2D differs between men and women during life course could lead to the identification of new therapeutic targets to prevent T2D more effectively in both women and men.
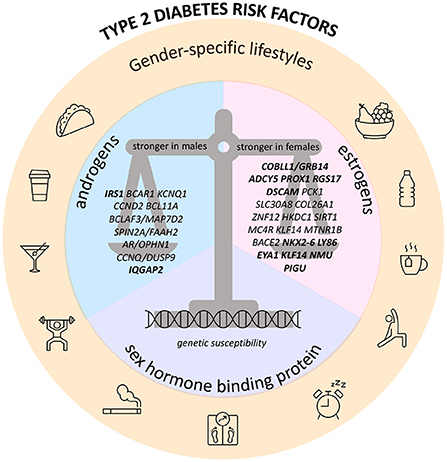
Figure 1. Recap figure depicting sex-specific genetic determinants of type 2 diabetes and glucose-related traits, and their interaction with endogenous/exogenous sex hormones, sex hormone binding protein, and gender-specific lifestyle factors. Hereby are presented the genes with sex-dimorphic effect on risk of T2D and/or GDM and/or FG and/or FI; genes in bold also have sex-dimorphic effect on BMI or WHR.
Author contributions
AL, MD, and MP wrote the manuscript. RD, GW, and SA provided guidance and edited the manuscript. All authors have approved the manuscript submission.
Conflict of interest
The authors declare that the research was conducted in the absence of any commercial or financial relationships that could be construed as a potential conflict of interest.
Publisher's note
All claims expressed in this article are solely those of the authors and do not necessarily represent those of their affiliated organizations, or those of the publisher, the editors and the reviewers. Any product that may be evaluated in this article, or claim that may be made by its manufacturer, is not guaranteed or endorsed by the publisher.
References
1. DeFronzo RA, Ferrannini E, Groop L, Henry RR, Herman WH, Holst JJ, et al. Type 2 diabetes mellitus. Nat Rev Dis Primer. (2015) 1:1–22. doi: 10.1038/nrdp.2015.19
2. Bellou V, Belbasis L, Tzoulaki I, Evangelou E. Risk factors for type 2 diabetes mellitus: an exposure-wide umbrella review of meta-analyses. PLoS ONE. (2018) 13:e0194127. doi: 10.1371/journal.pone.0194127
3. Diabetes. Available online at: https://www.who.int/health-topics/diabetes (accessed April 28, 2022).
4. Kautzky-Willer A, Harreiter J, Pacini G. Sex and gender differences in risk, pathophysiology and complications of type 2 diabetes mellitus. Endocr Rev. (2016) 37:278–316. doi: 10.1210/er.2015-1137
5. Logue J, Walker JJ, Colhoun HM, Leese GP, Lindsay RS, McKnight JA, et al. Do men develop type 2 diabetes at lower body mass indices than women? Diabetologia. (2011) 54:3003–6. doi: 10.1007/s00125-011-2313-3
6. Tramunt B, Smati S, Grandgeorge N, Lenfant F, Arnal J-F, Montagner A, et al. Sex differences in metabolic regulation and diabetes susceptibility. Diabetologia. (2020) 63:453–61. doi: 10.1007/s00125-019-05040-3
7. Asllanaj E, Bano A, Glisic M, Jaspers L, Ikram M, Laven J, et al. Age at natural menopause and life expectancy with and without type 2 diabetes. Menopause. (2019) 26:387–94. doi: 10.1097/GME.0000000000001246
8. Ren Y, Zhang M, Liu Y, Sun X, Wang B, Zhao Y, et al. Association of menopause and type 2 diabetes mellitus. Menopause. (2019) 26:325–30. doi: 10.1097/GME.0000000000001200
9. Visser JA. The importance of metabolic dysfunction in polycystic ovary syndrome. Nat Rev Endocrinol. (2021) 17:77–8. doi: 10.1038/s41574-020-00456-z
10. Aroda VR, Christophi CA, Edelstein SL, Zhang P, Herman WH, Barrett-Connor E, et al. The effect of lifestyle intervention and metformin on preventing or delaying diabetes among women with and without gestational diabetes: the diabetes prevention program outcomes study 10-year follow-up. J Clin Endocrinol Metab. (2015) 100:1646–53. doi: 10.1210/jc.2014-3761
11. Brand JS, van der Schouw YT, Onland-Moret NC, Sharp SJ, Ong KK, Khaw K-T, et al. Age at menopause, reproductive life span, and type 2 diabetes risk: results from the EPIC-InterAct study. Diabetes Care. (2013) 36:1012–9. doi: 10.2337/dc12-1020
12. Regensteiner JG, Golden S, Huebschmann AG, Barrett-Connor E, Chang AY, Chyun D, et al. Sex differences in the cardiovascular consequences of diabetes mellitus: a scientific statement from the American heart association. Circulation. (2015) 132:2424–47. doi: 10.1161/CIR.0000000000000343
13. Iorga A, Cunningham CM, Moazeni S, Ruffenach G, Umar S, Eghbali M. The protective role of estrogen and estrogen receptors in cardiovascular disease and the controversial use of estrogen therapy. Biol Sex Differ. (2017) 8:33. doi: 10.1186/s13293-017-0152-8
14. Fuchsberger C, Flannick J, Teslovich TM, Mahajan A, Agarwala V, Gaulton KJ, et al. The genetic architecture of type 2 diabetes. Nature. (2016) 536:41–7. doi: 10.1038/nature18642
15. Tian Y. PCOS-GWAS susceptibility variants in THADA, INSR, TOX3, and DENND1A are associated with metabolic syndrome or insulin resistance in women with PCOS. Front Endocrinol Lausanne. (2020) 11:274. doi: 10.3389/fendo.2020.00274
16. Pervjakova N. Multi-ancestry genome-wide association study of gestational diabetes mellitus highlights genetic links with type 2 diabetes. Hum Mol Genet. (2022) 31:3377–91. doi: 10.1093/hmg/ddac050
17. Yang J, Zeng J, Goddard ME, Wray NR, Visscher PM. Concepts, estimation and interpretation of SNP-based heritability. Nat Genet. (2017) 49:1304–10. doi: 10.1038/ng.3941
18. Bernabeu E. Sex differences in genetic architecture in the UK Biobank. Nat Genet. (2021) 53:1283–9. doi: 10.1038/s41588-021-00912-0
19. Scott LJ, Mohlke KL, Bonnycastle LL, Willer CJ Li Y, Duren WL, Erdos MR, et al. A genome-wide association study of type 2 diabetes in Finns detects multiple susceptibility variants. Science. (2007) 316:1341–5. doi: 10.1126/science.1142382
20. Mahajan A, Spracklen CN, Zhang W, Ng MCY, Petty LE, Kitajima H, et al. Multi-ancestry genetic study of type 2 diabetes highlights the power of diverse populations for discovery and translation. Nat Genet. (2022) 54:560–72. doi: 10.1038/s41588-022-01058-3
21. Chen J. The trans-ancestral genomic architecture of glycemic traits. Nat Genet. (2021) 53:840–60. doi: 10.1038/s41588-021-00852-9
22. Magi R, Lindgren CM, Morris AP. Meta-analysis of sex-specific genome-wide association studies. Genet Epidemiol. (2010) 34:846–53. doi: 10.1002/gepi.20540
23. Lagou V. Sex-dimorphic genetic effects and novel loci for fasting glucose and insulin variability. Nat Commun. (2021) 12:24. doi: 10.1038/s41467-020-19366-9
24. Goenaga D, Hampe C, Carré N, Cailliau K, Browaeys-Poly E, Perdereau D, et al. Molecular determinants of Grb14-mediated inhibition of insulin signaling. Mol Endocrinol Baltim Md. (2009) 23:1043–51. doi: 10.1210/me.2008-0360
25. Plešingerová H, Janovská P, Mishra A, Smyčková L, Poppová L, Libra A, et al. Expression of COBLL1 encoding novel ROR1 binding partner is robust predictor of survival in chronic lymphocytic leukemia. Haematologica. (2018) 103:313–24. doi: 10.3324/haematol.2017.178699
26. Teslovich TM. Biological, clinical and population relevance of 95 loci for blood lipids. Nature. (2010) 466:707–13. doi: 10.1038/nature09270
27. Morris AP. Large-scale association analysis provides insights into the genetic architecture and pathophysiology of type 2 diabetes. Nat Genet. (2012) 44:981–90. doi: 10.1038/ng.2383
28. Downie CG. Multi-ethnic GWAS and fine-mapping of glycaemic traits identify novel loci in the PAGE Study. Diabetologia. (2022) 65:477–89. doi: 10.1007/s00125-021-05635-9
29. Wessel J. Low-frequency and rare exome chip variants associate with fasting glucose and type 2 diabetes susceptibility. Nat Commun. (2015) 6:5897. doi: 10.1038/ncomms6897
30. Dupuis J. New genetic loci implicated in fasting glucose homeostasis and their impact on type 2 diabetes risk. Nat Genet. (2010) 42:105–16. doi: 10.1038/ng.520
31. Sinnott-Armstrong N. Genetics of 35 blood and urine biomarkers in the UK Biobank. Nat Genet. (2021) 53:185–94. doi: 10.1038/s41588-020-00757-z
32. Sakaue S. A cross-population atlas of genetic associations for 220 human phenotypes. Nat Genet. (2021) 53:1415–24. doi: 10.1038/s41588-021-00931-x
33. Wheeler E. Impact of common genetic determinants of Hemoglobin A1c on type 2 diabetes risk and diagnosis in ancestrally diverse populations: A transethnic genome-wide meta-analysis. PLoS Med. (2017) 14:1002383. doi: 10.1371/journal.pmed.1002383
34. Vujkovic M. Discovery of 318 new risk loci for type 2 diabetes and related vascular outcomes among 1.4 million participants in a multi-ancestry meta-analysis. Nat Genet. (2020) 52:680–91. doi: 10.1101/19012690
35. Xue A. Genome-wide association analyses identify 143 risk variants and putative regulatory mechanisms for type 2 diabetes. Nat Commun. (2018) 9:2941. doi: 10.1038/s41467-018-04951-w
36. Mahajan A. Fine-mapping type 2 diabetes loci to single-variant resolution using high-density imputation and islet-specific epigenome maps. Nat Genet. (2018) 50:1505–13. doi: 10.1038/s41588-018-0241-6
37. Zhao W. Identification of new susceptibility loci for type 2 diabetes and shared etiological pathways with coronary heart disease. Nat Genet. (2017) 49:1450–7. doi: 10.1038/ng.3943
38. Replication DIG. Genome-wide trans-ancestry meta-analysis provides insight into the genetic architecture of type 2 diabetes susceptibility. Nat Genet. (2014) 46:234–44. doi: 10.1038/ng.2897
39. Kichaev G. Leveraging polygenic functional enrichment to improve GWAS power. Am J Hum Genet. (2019) 104:65–75. doi: 10.1016/j.ajhg.2018.11.008
40. Spracklen CN. Identification of type 2 diabetes loci in 433,540 East Asian individuals. Nature. (2020) 582:240–5. doi: 10.1038/s41586-020-2263-3
41. Imamura M. Genome-wide association studies in the Japanese population identify seven novel loci for type 2 diabetes. Nat Commun. (2016) 7:10531. doi: 10.1038/ncomms10531
42. Qi Q. Genetics of type 2 diabetes in U.S. hispanic/latino individuals: results from the hispanic community health study/study of latinos (HCHS/SOL. Diabetes. (2017) 66:1419–25. doi: 10.2337/db16-1150
43. Bonas-Guarch S. Re-analysis of public genetic data reveals a rare X-chromosomal variant associated with type 2 diabetes. Nat Commun. (2018) 9:321. doi: 10.1038/s41467-017-02380-9
44. Christakoudi S, Evangelou E, Riboli E, Tsilidis KK. GWAS of allometric body-shape indices in UK Biobank identifies loci suggesting associations with morphogenesis, organogenesis, adrenal cell renewal and cancer. Sci Rep. (2021) 11:10688. doi: 10.1038/s41598-021-89176-6
45. Pulit SL. Meta-analysis of genome-wide association studies for body fat distribution in 694 649 individuals of European ancestry. Hum Mol Genet. (2019) 28:166–74. doi: 10.1093/hmg/ddy327
46. Martin S. Genetic evidence for different adiposity phenotypes and their opposing influences on ectopic fat and risk of cardiometabolic disease. Diabetes. (2021) 70:1843–56. doi: 10.2337/db21-0129
47. Zhu Z. Shared genetic and experimental links between obesity-related traits and asthma subtypes in UK Biobank. J Allergy Clin Immunol. (2020) 145:537–49. doi: 10.1016/j.jaci.2019.09.035
48. Han X. Using Mendelian randomization to evaluate the causal relationship between serum C-reactive protein levels and age-related macular degeneration. Eur J Epidemiol. (2020) 35:139–46. doi: 10.1007/s10654-019-00598-z
49. Zhang G. Genetic associations with gestational duration and spontaneous preterm birth. N Engl J Med. (2017) 377:1156–67. doi: 10.1056/NEJMc1713902
50. Yang XL. Three novel loci for infant head circumference identified by a joint association analysis. Front Genet. (2019) 10:947. doi: 10.3389/fgene.2019.00947
51. Warrington NM. Maternal and fetal genetic effects on birth weight and their relevance to cardio-metabolic risk factors. Nat Genet. (2019) 51:804–14. doi: 10.1038/s41588-019-0403-1
52. Freathy RM. Variants in ADCY5 and near CCNL1 are associated with fetal growth and birth weight. Nat Genet. (2010) 42:430–5. doi: 10.1038/ng.567
53. Plotnikov D, Williams C, Guggenheim JA. Association between birth weight and refractive error in adulthood: a mendelian randomisation study. Br J Ophthalmol. (2020) 104:214–9. doi: 10.1136/bjophthalmol-2018-313640
54. Horikoshi M. Genome-wide associations for birth weight and correlations with adult disease. Nature. (2016) 538:248–52. doi: 10.1038/nature19806
55. Horikoshi M. Discovery and fine-mapping of glycaemic and obesity-related trait loci using high-density imputation. PLoS Genet. (2015) 11:e1005230. doi: 10.1371/journal.pgen.1005230
56. Beck JJ. Genetic meta-analysis of twin birth weight shows high genetic correlation with singleton birth weight. Hum Mol Genet. (2021) 30:1894–905. doi: 10.1093/hmg/ddab121
57. Liu DJ. Exome-wide association study of plasma lipids in >300,000 individuals. Nat Genet. (2017) 49:1758–66. doi: 10.1038/ng.3977
58. Hoffmann TJ. A large electronic-health-record-based genome-wide study of serum lipids. Nat Genet. (2018) 50:401–13. doi: 10.1038/s41588-018-0064-5
59. Richardson TG. Evaluating the relationship between circulating lipoprotein lipids and apolipoproteins with risk of coronary heart disease: a multivariable Mendelian randomisation analysis. PLoS Med. (2020) 17:e1003062. doi: 10.1371/journal.pmed.1003062
60. Evangelou E. Genetic analysis of over 1 million people identifies 535 new loci associated with blood pressure traits. Nat Genet. (2018) 50:1412–25. doi: 10.1038/s41588-018-0205-x
61. Ruth KS. Using human genetics to understand the disease impacts of testosterone in men and women. Nat Med. (2020) 26:252–8. doi: 10.1038/s41591-020-0751-5
62. Elsir T, Smits A, Lindstrom MS, Nister M. Transcription factor PROX1: its role in development and cancer. Cancer Metastasis Rev. (2012) 31:793–805. doi: 10.1007/s10555-012-9390-8
63. Mansour Aly D. Genome-wide association analyses highlight etiological differences underlying newly defined subtypes of diabetes. Nat Genet. (2021) 53:1534–42. doi: 10.1038/s41588-021-00948-2
64. Ducoli L, Detmar M. Beyond PROX1: transcriptional, epigenetic, and noncoding RNA regulation of lymphatic identity and function. Dev Cell. (2021) 56:406–26. doi: 10.1016/j.devcel.2021.01.018
65. Masotti M, Guo B, Wu B. Pleiotropy informed adaptive association test of multiple traits using genome-wide association study summary data. Biometrics. (2019) 75:1076–85. doi: 10.1111/biom.13076
66. Lind L. Genetic determinants of clustering of cardiometabolic risk factors in U.K. Biobank Metab Syndr Relat Disord. (2020) 18:121–7. doi: 10.1089/met.2019.0096
67. Manning AK. A genome-wide approach accounting for body mass index identifies genetic variants influencing fasting glycemic traits and insulin resistance. Nat Genet. (2012) 44:659–69. doi: 10.1038/ng.2274
68. Surakka I. The impact of low-frequency and rare variants on lipid levels. Nat Genet. (2015) 47:589–97. doi: 10.1038/ng.3300
69. Barton AR, Sherman MA, Mukamel RE, Loh PR. Whole-exome imputation within UK biobank powers rare coding variant association and fine-mapping analyses. Nat Genet. (2021) 53:1260–9. doi: 10.1038/s41588-021-00892-1
70. Mao H. RGS17/RGSZ2, a novel regulator of Gi/o, Gz, and Gq signaling. J Biol Chem. (2004) 279:26314–22. doi: 10.1074/jbc.M401800200
71. Akiyama M. Genome-wide association study identifies 112 new loci for body mass index in the Japanese population. Nat Genet. (2017) 49:1458–67. doi: 10.1038/ng.3951
72. Winkler TW. The influence of age and sex on genetic associations with adult body size and shape: a large-scale genome-wide interaction study. PLoS Genet. (2015) 11:e1005378. doi: 10.1371/journal.pgen.1005378
73. Randall JC. Sex-stratified genome-wide association studies including 270,000 individuals show sexual dimorphism in genetic loci for anthropometric traits. PLoS Genet. (2013) 9:e1003500. doi: 10.1371/journal.pgen.1003500
74. Heid IM. Meta-analysis identifies 13 new loci associated with waist-hip ratio and reveals sexual dimorphism in the genetic basis of fat distribution. Nat Genet. (2010) 42:949–60. doi: 10.1038/ng.685
75. Shungin D. New genetic loci link adipose and insulin biology to body fat distribution. Nature. (2015) 518:187–96. doi: 10.1038/nature14132
76. Kilpelainen TO. Genetic variation near IRS1 associates with reduced adiposity and an impaired metabolic profile. Nat Genet. (2011) 43:753–60. doi: 10.1038/ng.866
77. Vazquez-Moreno M. Sex/gender modifies the association between the MC4R p.Ile269Asn mutation and type 2 diabetes in the Mexican population. J Clin Endocrinol Metab. (2021) 106:112–7. doi: 10.1210/clinem/dgaa726
78. Hayes MG. Identification of HKDC1 and BACE2 as genes influencing glycemic traits during pregnancy through genome-wide association studies. Diabetes. (2013) 62:3282–91. doi: 10.2337/db12-1692
79. Kong S, Cho YS. Identification of female-specific genetic variants for metabolic syndrome and its component traits to improve the prediction of metabolic syndrome in females. BMC Med Genet. (2019) 20:99. doi: 10.1186/s12881-019-0830-y
80. Dong Y. SIRT1 is associated with a decrease in acute insulin secretion and a sex specific increase in risk for type 2 diabetes in Pima Indians. Mol Genet Metab. (2011) 104:661–5. doi: 10.1016/j.ymgme.2011.08.001
81. Dominguez-Cruz MG. Pilot genome-wide association study identifying novel risk loci for type 2 diabetes in a Maya population. Gene. (2018) 677:324–31. doi: 10.1016/j.gene.2018.08.041
82. Hara K. Genome-wide association study identifies three novel loci for type 2 diabetes. Hum Mol Genet. (2014) 23:239–46. doi: 10.1093/hmg/ddt399
83. Bloomer LD. Male-specific region of the Y chromosome and cardiovascular risk: phylogenetic analysis and gene expression studies. Arter Thromb Vasc Biol. (2013) 33:1722–7. doi: 10.1161/ATVBAHA.113.301608
84. Tan MHE, Li J, Xu E, Melcher K, Yong E-l. Androgen receptor: structure, role in prostate cancer and drug discovery. Acta Pharmacol Sin. (2015).
85. Wu NN. A genome-wide association study of gestational diabetes mellitus in Chinese women. J Matern Fetal Neonatal Med. (2021) 34:1557–64. doi: 10.1080/14767058.2019.1640205
86. Powe CE, Kwak SH. Genetic studies of gestational diabetes and glucose metabolism in pregnancy. Curr Diab Rep. (2020) 20:69. doi: 10.1007/s11892-020-01355-3
87. Wei W. Gestational diabetes mellitus: the genetic susceptibility behind the disease. Horm Metab Res. (2021) 53:489–98. doi: 10.1055/a-1546-1652
88. Vuckovic D. The polygenic and monogenic basis of blood traits and diseases. Cell. (2020) 182:1214–31. doi: 10.1016/j.cell.2020.08.008
89. Chen MH. Trans-ethnic and ancestry-specific blood-cell genetics in 746,667 individuals from 5 global populations. Cell. (2020) 182:1198–213. doi: 10.1016/j.cell.2020.06.045
90. Astle WJ. The allelic landscape of human blood cell trait variation and links to common complex disease. Cell. (2016) 167:1415–29. doi: 10.1016/j.cell.2016.10.042
91. Kachuri L. Genetic determinants of blood-cell traits influence susceptibility to childhood acute lymphoblastic leukemia. Am J Hum Genet. (2021) 108:1823–35. doi: 10.1016/j.ajhg.2021.08.004
92. Ward LD. GWAS of serum ALT and AST reveals an association of SLC30A10 Thr95Ile with hypermanganesemia symptoms. Nat Commun. (2021) 12:4571. doi: 10.1101/2020.05.19.104570
93. Chen VL. Genome-wide association study of serum liver enzymes implicates diverse metabolic and liver pathology. Nat Commun. (2021) 12:816. doi: 10.1038/s41467-020-20870-1
94. Wojcik GL. Genetic analyses of diverse populations improves discovery for complex traits. Nature. (2019) 570:514–8. doi: 10.1038/s41586-019-1310-4
95. Loomis SJ. Genome-wide association study of serum fructosamine and glycated albumin in adults without diagnosed diabetes: results from the atherosclerosis risk in communities study. Diabetes. (2018) 67:1684–96. doi: 10.2337/db17-1362
96. Chung RH. Multi-omics analysis identifies CpGs near G6PC2 mediating the effects of genetic variants on fasting glucose. Diabetologia. (2021) 64:1613–25. doi: 10.1007/s00125-021-05449-9
97. Li-Gao R. Genetic studies of metabolomics change after a liquid meal illuminate novel pathways for glucose and lipid metabolism. Diabetes. (2021) 70:2932–46. doi: 10.2337/db21-0397
98. Rasmussen-Torvik LJ. Fasting glucose GWAS candidate region analysis across ethnic groups in the multiethnic study of atherosclerosis (MESA. Genet Epidemiol. (2012) 36:384–91. doi: 10.1002/gepi.21632
99. Prokopenko I. Variants in MTNR1B influence fasting glucose levels. Nat Genet. (2009) 41:77–81. doi: 10.1038/ng.290
100. Chambers JC. Common genetic variation near melatonin receptor MTNR1B contributes to raised plasma glucose and increased risk of type 2 diabetes among Indian Asians and European Caucasians. Diabetes. (2009) 58:2703–8. doi: 10.2337/db08-1805
101. Bouatia-Naji N. A variant near MTNR1B is associated with increased fasting plasma glucose levels and type 2 diabetes risk. Nat Genet. (2009) 41:89–94. doi: 10.1038/ng.277
102. Soranzo N. Common variants at 10 genomic loci influence hemoglobin A(1)(C) levels via glycemic and nonglycemic pathways. Diabetes. (2010) 59:3229–39. doi: 10.2337/db10-0502
103. Kanai M. Genetic analysis of quantitative traits in the Japanese population links cell types to complex human diseases. Nat Genet. (2018) 50:390–400. doi: 10.1038/s41588-018-0047-6
104. Wood AR. A genome-wide association study of IVGTT-based measures of first-phase insulin secretion refines the underlying physiology of type 2 diabetes variants. Diabetes. (2017) 66:2296–309. doi: 10.2337/db16-1452
105. Prokopenko I. A central role for GRB10 in regulation of islet function in man. PLoS Genet. (2014) 10:1004235. doi: 10.1371/journal.pgen.1004235
106. Voight BF. Twelve type 2 diabetes susceptibility loci identified through large-scale association analysis. Nat Genet. (2010) 42:579–89. doi: 10.1038/ng.609
107. Keaton JM. Genome-wide interaction with the insulin secretion locus MTNR1B reveals CMIP as a novel type 2 diabetes susceptibility gene in African Americans. Genet Epidemiol. (2018) 42:559–70. doi: 10.1002/gepi.22126
108. Jones SE. Genome-wide association analyses of chronotype in 697,828 individuals provides insights into circadian rhythms. Nat Commun. (2019) 10:343. doi: 10.1038/s41467-018-08259-7
109. Dashti HS. Genetic determinants of daytime napping and effects on cardiometabolic health. Nat Commun. (2021) 12:900. doi: 10.1038/s41467-020-20585-3
110. Kwak SH. A genome-wide association study of gestational diabetes mellitus in Korean women. Diabetes. (2012) 61:531–41. doi: 10.2337/db11-1034
111. Cox RD, Church CD. Mouse models and the interpretation of human GWAS in type 2 diabetes and obesity. Dis Model Mech. (2011) 4:155–64. doi: 10.1242/dmm.000414
112. Pugsley MK, Brooks MB, Fishman CE, Katavolos P, Chiang AY, Parish ST, et al. Use of the ZDF rat to model dietary fat induced hypercoagulability is limited by progressive and fatal nephropathy. J Pharmacol Toxicol Methods. (2021) 107:106933. doi: 10.1016/j.vascn.2020.106933
113. Van Sinderen M, Steinberg G, Jorgensen SB, Honeyman J, Chow JDY, Simpson ER, et al. Sexual dimorphism in the glucose homeostasis phenotype of the aromatase knockout (ArKO) mice. J Steroid Biochem Mol Biol. (2017) 170:39–48. doi: 10.1016/j.jsbmb.2016.05.013
114. Kanemoto N, Hishigaki H, Miyakita A, Oga K, Okuno S, Tsuji A, et al. Genetic dissection of “OLETF”, a rat model for non-insulin-dependent diabetes mellitus. Mamm Genome. (1998) 9:419–25. doi: 10.1007/s003359900789
115. Hirashima T, Kawano K, Mori S, Matsumoto K, Natori T. A diabetogenic gene (ODB-1) assigned to the X-chromosome in OLETF rats. Diabetes Res Clin Pract. (1995) 27:91–6. doi: 10.1016/0168-8227(95)01028-C
116. Kim JH, Stewart TP, Soltani-Bejnood M, Wang L, Fortuna JM, Mostafa OA, et al. Phenotypic characterization of polygenic type 2 diabetes in TALLYHO/JngJ mice. J Endocrinol. (2006) 191:437–46. doi: 10.1677/joe.1.06647
117. Leiter EH, Strobel M, O'Neill A, Schultz D, Schile A, Reifsnyder PC. Comparison of two new mouse models of polygenic type 2 diabetes at the jackson laboratory, NONcNZO10Lt/J and TALLYHO/JngJ. J Diabetes Res. (2013) 2013:165327. doi: 10.1155/2013/165327
118. Kim JH, Sen S, Avery CS, Simpson E, Chandler P, Nishina PM, et al. Genetic analysis of a new mouse model for non-insulin-dependent diabetes. Genomics. (2001) 74:273–86. doi: 10.1006/geno.2001.6569
119. Kluge R, Scherneck S, Schürmann A, Joost H-G. Pathophysiology and genetics of obesity and diabetes in the New Zealand obese mouse: a model of the human metabolic syndrome. Methods Mol Biol Clifton NJ. (2012) 933:59–73. doi: 10.1007/978-1-62703-068-7_5
120. Lenzen S. The mechanisms of alloxan- and streptozotocin-induced diabetes. Diabetologia. (2008) 51:216–26. doi: 10.1007/s00125-007-0886-7
121. Paik SG, Michelis MA, Kim YT, Shin S. Induction of insulin-dependent diabetes by streptozotocin. inhibition by estrogens and potentiation by androgens. Diabetes. (1982) 31:724–9. doi: 10.2337/diabetes.31.8.724
122. Li Y, Huang J, Yan Y, Liang J, Liang Q, Lu Y, et al. Preventative effects of resveratrol and estradiol on streptozotocin-induced diabetes in ovariectomized mice and the related mechanisms. PLoS ONE. (2018) 13:e0204499. doi: 10.1371/journal.pone.0204499
123. Weigt C, Hertrampf T, Flenker U, Hülsemann F, Kurnaz P, Fritzemeier KH, et al. Effects of estradiol, estrogen receptor subtype-selective agonists and genistein on glucose metabolism in leptin resistant female Zucker diabetic fatty (ZDF) rats. J Steroid Biochem Mol Biol. (2015) 154:12–22. doi: 10.1016/j.jsbmb.2015.06.002
124. Burgoyne PS, Arnold AP. A primer on the use of mouse models for identifying direct sex chromosome effects that cause sex differences in non-gonadal tissues. Biol Sex Differ. (2016) 7:68. doi: 10.1186/s13293-016-0115-5
125. De Vries GJ, Rissman EF, Simerly RB, Yang L-Y, Scordalakes EM, Auger CJ, et al. model system for study of sex chromosome effects on sexually dimorphic neural and behavioral traits. J Neurosci Off J Soc Neurosci. (2002) 22:9005–14. doi: 10.1523/JNEUROSCI.22-20-09005.2002
126. Blencowe M, Chen X, Zhao Y, Itoh Y, McQuillen CN, Han Y, et al. Relative contributions of sex hormones, sex chromosomes, and gonads to sex differences in tissue gene regulation. Genome Res. (2022) 32:807–24. doi: 10.1101/gr.275965.121
127. Martínez de Morentin PB, González-García I, Martins L, Lage R, Fernández-Mallo D, Martínez-Sánchez N, et al. Estradiol regulates brown adipose tissue thermogenesis via hypothalamic AMPK. Cell Metab. (2014) 20:41–53. doi: 10.1016/j.cmet.2014.03.031
128. Moreira-Pais A, Ferreira R, Neves JS, Vitorino R, Moreira-Gonçalves D, Nogueira-Ferreira R. Sex differences on adipose tissue remodeling: from molecular mechanisms to therapeutic interventions. J Mol Med Berl Ger. (2020) 98:483–93. doi: 10.1007/s00109-020-01890-2
129. Ding EL, Song Y, Manson JE, Hunter DJ, Lee CC, Rifai N, et al. Sex hormone-binding globulin and risk of type 2 diabetes in women and men. N Engl J Med. (2009) 361:1152–63. doi: 10.1056/NEJMoa0804381
130. Ding EL, Song Y, Malik VS, Liu S. Sex differences of endogenous sex hormones and risk of type 2 diabetes: a systematic review and meta-analysis. JAMA. (2006) 295:1288–99. doi: 10.1001/jama.295.11.1288
131. Perry JRB, Weedon MN, Langenberg C, Jackson AU, Lyssenko V, Sparsø T, et al. Genetic evidence that raised sex hormone binding globulin (SHBG) levels reduce the risk of type 2 diabetes. Hum Mol Genet. (2010) 19:535–44. doi: 10.1093/hmg/ddp522
132. Wallace IR, McKinley MC, Bell PM, Hunter SJ. Sex hormone binding globulin and insulin resistance. Clin Endocrinol. (2013) 78:321–9. doi: 10.1111/cen.12086
133. Li J, Lai H, Chen S, Zhu H, Lai S. Interaction of sex steroid hormones and obesity on insulin resistance and type 2 diabetes in men: the third national health and nutrition examination survey. J Diabetes Complications. (2017) 31:318–27. doi: 10.1016/j.jdiacomp.2016.10.022
134. Harper-Harrison G, Shanahan MM. “Hormone Replacement Therapy” StatPearls. Treasure Island, FL: StatPearls Publishing (2022). Available online at: http://www.ncbi.nlm.nih.gov/books/NBK493191/ (accessed March 15, 2022).
135. Bitoska I, Krstevska B, Milenkovic T, Subeska-Stratrova S, Petrovski G, Mishevska SJ, et al. Effects of hormone replacement therapy on insulin resistance in postmenopausal diabetic women. Open Access Maced J Med Sci. (2016) 4:83–8. doi: 10.3889/oamjms.2016.024
136. Kanaya AM, Herrington D, Vittinghoff E, Lin F, Grady D, Bittner V, et al. Glycemic effects of postmenopausal hormone therapy: the heart and estrogen/progestin replacement study: a randomized, double-blind, placebo-controlled trial. Ann Intern Med. (2003) 138:1–9. doi: 10.7326/0003-4819-138-1-200301070-00005
137. Salpeter SR, Walsh JME, Ormiston TM, Greyber E, Buckley NS, Salpeter EE. Meta-analysis: effect of hormone-replacement therapy on components of the metabolic syndrome in postmenopausal women. Diabetes Obes Metab. (2006) 8:538–54. doi: 10.1111/j.1463-1326.2005.00545.x
138. Mauvais-Jarvis F, Manson JE, Stevenson JC, Fonseca VA. Menopausal hormone therapy and type 2 diabetes prevention: evidence, mechanisms, and clinical implications. Endocr Rev. (2017) 38:173–88. doi: 10.1210/er.2016-1146
139. Manson JE, Chlebowski RT, Stefanick ML, Aragaki AK, Rossouw JE, Prentice RL, et al. Menopausal hormone therapy and health outcomes during the intervention and extended poststopping phases of the Women's Health Initiative randomized trials. JAMA. (2013) 310:1353–68. doi: 10.1001/jama.2013.278040
140. Gooren LJ, Kreukels B, Lapauw B, Giltay EJ. (Patho)physiology of cross-sex hormone administration to transsexual people: the potential impact of male-female genetic differences. Andrologia. (2015) 47:5–19. doi: 10.1111/and.12389
141. Spanos C, Bretherton I, Zajac JD, Cheung AS. Effects of gender-affirming hormone therapy on insulin resistance and body composition in transgender individuals: a systematic review. World J Diabetes. (2020) 11:66–77. doi: 10.4239/wjd.v11.i3.66
142. Elbers JMH, Giltay EJ, Teerlink T, Scheffer PG, Asscheman H, Seidell JC, et al. Effects of sex steroids on components of the insulin resistance syndrome in transsexual subjects. Clin Endocrinol. (2003) 58:562–71. doi: 10.1046/j.1365-2265.2003.01753.x
143. Islam N, Nash R, Zhang Q, Panagiotakopoulos L, Daley T, Bhasin S, et al. Is There a link between hormone use and diabetes incidence in transgender people? data from the STRONG cohort. J Clin Endocrinol Metab. (2022) 107:e1549–57. doi: 10.1210/clinem/dgab832
144. Wierckx K, Elaut E, Declercq E, Heylens G, De Cuypere G, Taes Y, et al. Prevalence of cardiovascular disease and cancer during cross-sex hormone therapy in a large cohort of trans persons: a case-control study. Eur J Endocrinol. (2013) 169:471–8. doi: 10.1530/EJE-13-0493
145. Morselli E, Santos RS, Criollo A, Nelson MD, Palmer BF, Clegg DJ. The effects of oestrogens and their receptors on cardiometabolic health. Nat Rev Endocrinol. (2017) 13:352–64. doi: 10.1038/nrendo.2017.12
146. Rettberg JR, Yao J, Brinton RD. Estrogen: a master regulator of bioenergetic systems in the brain and body. Front Neuroendocrinol. (2014) 35:8–30. doi: 10.1016/j.yfrne.2013.08.001
147. Brooks HL, Pollow DP, Hoyer PB. The VCD mouse model of menopause and perimenopause for the study of sex differences in cardiovascular disease and the metabolic syndrome. Physiology. (2016) 31:250–7. doi: 10.1152/physiol.00057.2014
148. Romero-Aleshire MJ, Diamond-Stanic MK, Hasty AH, Hoyer PB, Brooks HL. Loss of ovarian function in the VCD mouse-model of menopause leads to insulin resistance and a rapid progression into the metabolic syndrome. Am J Physiol Regul Integr Comp Physiol. (2009) 297:R587–92. doi: 10.1152/ajpregu.90762.2008
149. Keck M, Romero-Aleshire MJ, Cai Q, Hoyer PB, Brooks HL. Hormonal status affects the progression of STZ-induced diabetes and diabetic renal damage in the VCD mouse model of menopause. Am J Physiol-Ren Physiol. (2007) 293:F193–9. doi: 10.1152/ajprenal.00022.2007
150. Veroni MC, Proietto J, Larkins RG. Evolution of insulin resistance in New Zealand obese mice. Diabetes. (1991) 40:1480–7. doi: 10.2337/diabetes.40.11.1480
151. Vogel H, Mirhashemi F, Liehl B, Taugner F, Kluth O, Kluge R, et al. Estrogen deficiency aggravates insulin resistance and induces β-cell loss and diabetes in female New Zealand obese mice. Horm Metab Res. (2013) 45:430–5. doi: 10.1055/s-0032-1331700
152. Tiano JP, Delghingaro-Augusto V, Le May C, Liu S, Kaw MK, Khuder SS, et al. Estrogen receptor activation reduces lipid synthesis in pancreatic islets and prevents β cell failure in rodent models of type 2 diabetes. J Clin Invest. (2011) 121:3331–42. doi: 10.1172/JCI44564
153. Kawakami M, Yokota-Nakagi N, Uji M, Yoshida K, Tazumi S, Takamata A, et al. Estrogen replacement enhances insulin-induced AS160 activation and improves insulin sensitivity in ovariectomized rats. Am J Physiol-Endocrinol Metab. (2018) 315:E1296–304. doi: 10.1152/ajpendo.00131.2018
154. Yokota-Nakagi N, Omoto S, Tazumi S, Kawakami M, Takamata A, Morimoto K. Estradiol replacement improves high-fat diet-induced insulin resistance in ovariectomized rats. Physiol Rep. (2022) 10:e15193. doi: 10.14814/phy2.15193
155. Santos RS, Batista TM, Camargo RL, Morato PN, Borck PC, Leite NC, et al. Lacking of estradiol reduces insulin exocytosis from pancreatic β-cells and increases hepatic insulin degradation. Steroids. (2016) 114:16–24. doi: 10.1016/j.steroids.2016.05.002
156. Zhu L, Brown WC, Cai Q, Krust A, Chambon P, McGuinness OP, et al. Estrogen treatment after ovariectomy protects against fatty liver and may improve pathway-selective insulin resistance. Diabetes. (2013) 62:424–34. doi: 10.2337/db11-1718
157. Agarwal P, Brar N, Morriseau TS, Kereliuk SM, Fonseca MA, Cole LK, et al. Gestational diabetes adversely affects pancreatic islet architecture and function in the male rat offspring. Endocrinology. (2019) 160:1907–25. doi: 10.1210/en.2019-00232
158. Yokomizo H, Inoguchi T, Sonoda N, Sakaki Y, Maeda Y, Inoue T, et al. Maternal high-fat diet induces insulin resistance and deterioration of pancreatic β-cell function in adult offspring with sex differences in mice. Am J Physiol-Endocrinol Metab. (2014) 306:E1163–75. doi: 10.1152/ajpendo.00688.2013
159. Who Smokes More, Men or Women? Our World Data. Available online at: https://ourworldindata.org/who-smokes-more-men-or-women (accessed June 8, 2022).
160. Reitsma MB, Kendrick PJ, Ababneh E, Abbafati C, Abbasi-Kangevari M, Abdoli A, et al. Spatial, temporal, and demographic patterns in prevalence of smoking tobacco use and attributable disease burden in 204 countries and territories, 1990–2019: a systematic analysis from the global burden of disease study 2019. Lancet. (2021) 397:2337–60. doi: 10.1016/S0140-6736(21)01169-7
161. Imamura F, Micha R, Khatibzadeh S, Fahimi S, Shi P, Powles J, et al. Dietary quality among men and women in 187 countries in 1990 and 2010: a systematic assessment. Lancet Glob Health. (2015) 3:e132–42. doi: 10.1016/S2214-109X(14)70381-X
162. Wardle J, Haase AM, Steptoe A, Nillapun M, Jonwutiwes K, Bellisle F. Gender differences in food choice: the contribution of health beliefs and dieting. Ann Behav Med Publ Soc Behav Med. (2004) 27:107–16. doi: 10.1207/s15324796abm2702_5
163. Guthold R, Stevens GA, Riley LM, Bull FC. Worldwide trends in insufficient physical activity from 2001 to 2016: a pooled analysis of 358 population-based surveys with 1·9 million participants. Lancet Glob Health. (2018) 6:e1077–86. doi: 10.1016/S2214-109X(18)30357-7
164. Gerovasili V, Agaku IT, Vardavas CI, Filippidis FT. Levels of physical activity among adults 18-64 years old in 28 European countries. Prev Med. (2015) 81:87–91. doi: 10.1016/j.ypmed.2015.08.005
165. Levy RB, Rauber F, Chang K, Louzada ML da C, Monteiro CA, Millett C, et al. Ultra-processed food consumption and type 2 diabetes incidence: a prospective cohort study. Clin Nutr Edinb Scotl. (2021) 40:3608–14. doi: 10.1016/j.clnu.2020.12.018
166. Gao M, Jebb SA, Aveyard P, Ambrosini GL, Perez-Cornago A, Papier K, et al. Associations between dietary patterns and incident type 2 diabetes: prospective cohort study of 120,343 UK biobank participants. Diabetes Care. (2022) 45:1315–25. doi: 10.2337/figshare.19209750
Keywords: genetics, type 2 diabetes, sex-specific effect, sex-dimorphism, gestational diabetes, genome wide association studies, animal models
Citation: Lamri A, De Paoli M, De Souza R, Werstuck G, Anand S and Pigeyre M (2022) Insight into genetic, biological, and environmental determinants of sexual-dimorphism in type 2 diabetes and glucose-related traits. Front. Cardiovasc. Med. 9:964743. doi: 10.3389/fcvm.2022.964743
Received: 08 June 2022; Accepted: 21 October 2022;
Published: 24 November 2022.
Edited by:
Mete Civelek, University of Virginia, United StatesReviewed by:
Sander W. van der Laan, University Medical Center Utrecht, NetherlandsMichael C. Mahaney, The University of Texas Rio Grande Valley, United States
Copyright © 2022 Lamri, De Paoli, De Souza, Werstuck, Anand and Pigeyre. This is an open-access article distributed under the terms of the Creative Commons Attribution License (CC BY). The use, distribution or reproduction in other forums is permitted, provided the original author(s) and the copyright owner(s) are credited and that the original publication in this journal is cited, in accordance with accepted academic practice. No use, distribution or reproduction is permitted which does not comply with these terms.
*Correspondence: Marie Pigeyre, cGlnZXlyZW0mI3gwMDA0MDttY21hc3Rlci5jYQ==