- 1Brain-Heart Axis: Cellular and Molecular Mechanisms Unit, Centro Cardiologico Monzino Istituti di Ricovero e Cura a Carattere Scientifico (IRCCS), Milan, Italy
- 2Department of Biomolecular and Pharmacological Sciences, University of Milan, Milan, Italy
- 3EPIGET LAB, Department of Clinical Sciences and Community Health, University of Milan, Milan, Italy
- 4Occupational Health Unit, Fondazione Istituti di Ricovero e Cura a Carattere Scientifico (IRCCS) Ca' Granda Ospedale Maggiore Policlinico, Milan, Italy
- 5Department of Neurosciences and Mental Health, Fondazione Istituti di Ricovero e Cura a Carattere Scientifico (IRCCS) Ca'Granda Ospedale, Maggiore Policlinico, Milan, Italy
- 6Department of Pathophysiology and Transplantation, University of Milan, Milan, Italy
- 7Department of Pharmaceutical Sciences, University of Milan, Milan, Italy
Background: Obesity and depression are intertwined diseases often associated with an increased risk of cardiovascular (CV) complications. Brain-Derived Neurotrophic Factor (BDNF), altered in the brain both of subjects with depression and obesity, provides a potential link between depression and thrombosis. Since the relationship among peripheral BDNF, depression and obesity is not well-defined, the aim of the present report has been to address this issue taking advantage of the contribution played by extracellular vesicle (EV)-derived miRNAs.
Research Process: Associations among circulating BDNF, depression and EV-derived miRNAs related to atherothrombosis have been evaluated in a large Italian cohort of obese individuals (n = 743), characterized by the Beck Depression Inventory (BDI-II) score.
Results: BDI-II was negatively associated with BDNF levels without a significant impact of the rs6265 BDNF polymorphism; this association was modified by raised levels of IFN-γ. BDNF levels were linked to an increase of 80 EV-derived miRNAs and a decrease of 59 miRNAs related to atherosclerosis and thrombosis. Network analysis identified at least 18 genes targeted by these miRNAs, 7 of which involved in depression and CV risk. The observation of a possible link among BDNF, depression, and miRNAs related to atherothrombosis and depression in obesity is novel and may lead to a wider use of BDNF as a CV risk biomarker in this specific subject group.
Introduction
Obesity prevalence has increased exponentially over the last few decades reaching epidemic proportions. Between 2017 and 2018, 42.4% of adult Americans were found to have a body mass index (BMI) exceeding 30 (1). Obesity is a complex condition, with serious social and psychological facets. It may affect virtually all age and socioeconomic groups and it may threaten to overwhelm the health systems of both developed and developing countries. Contrary to conventional wisdom, the obesity epidemic is not restricted to industrialized societies; in developing countries, it is estimated that over 115 million people suffer from obesity-related health problems (2).
Although in men generally there is a higher rate of overweight, women have instead higher rates of obesity, linked to numerous health consequences, including diabetes and diseases of the cardiovascular (CV) system, such as hypertension, stroke, atherosclerosis, and thrombosis (3). In addition, systematic reviews and meta-analyses have confirmed the relationship between obesity and depression, both indicating that obesity raises the risk of depression, and that depression predisposes to obesity (4). This reciprocal link establishes a potential vicious circle that can lead to or aggravate the development of metabolic disorders. In particular, it is well-known that depression increases markedly the risk to develop CV complications in obese patients (5). Understanding the biochemical bases of obesity/depression, is thus crucial in order to identify new potential biomarkers of CV diseases (CVDs).
In this complex area of research, the investigations on circulating extracellular vesicles (EVs)-derived miRNAs have achieved a great interest. In particular, changes in circulating EV-derived miRNAs have been described in atherosclerosis, thrombosis and obesity, suggesting that EVs might play a role in the crosstalk between metabolic homeostasis and CV complications (6, 7).
Genome-wide association studies (GWAS) have identified 24 potential pleiotropic genes, overlapping between mood disorders and cardiometabolic diseases (8). Among these genes, brain derived neurotrophic factor (BDNF) has been recently found in both obesity and depression (9). BDNF is a neurotrophin playing a pivotal role in several physiological and pathological conditions, including neuroplasticity, energy homeostasis, and cardiovascular function (10). It is involved in the regulation of neurogenesis and neuroprotection, food intake, atherosclerotic plaque variability and morphology, and it has been related to inflammation, fibrin clotting stability, platelet and monocyte activation, and adipocyte maturation (11–14). Altogether, the hypothesis that altered levels of this neurotrophin may predispose to a raised CV risk is supported by many Authors (15).
Polymorphisms in the BDNF gene, among these the rs6265, have been associated with depression (16) and acute coronary syndromes (17, 18); low circulating BDNF levels have been reported in coronary artery disease (12, 15, 19) and in depressed patients (20). In contrast, the relationship between peripheral BDNF and obesity is not well-defined (21). Indeed, owing to small sample sizes of clinical studies (<100 individuals), bias in subjects' recruitment, procedures of sampling/storage and BDNF measurement, as well as the lack of adjustment for confounders (e.g., platelet number, physical exercise) it is difficult to interpret results in literature (22).
Aim of the present study was, therefore, to investigate the association among BDNF, depression and EV-derived miRNAs related to atherothrombosis in a cohort of 743 obese individuals. In addition, the impact of the rs6265 BDNF polymorphism (23) on these associations has been explored.
Materials and Methods
Study Design and Patient Population
Seventy hundred and forty-three individuals of the cross-sectional Susceptibility to Particle Health Effects, miRNAs and Exosomes (SPHERE) study were randomly selected (24). These individuals were recruited at the Center for Obesity and Work-Activity (Fondazione IRCCS Ca' Granda Ospedale Maggiore Policlinico in Milan, Lombardy, Italy). The eligibility criteria of the SPHERE study were: (a) older than 18 years at enrolment; (b) overweight/obese according to body mass index (BMI): overweight, BMI between 25 and 30 kg/m2; obese: BMI of 30 kg/m2 or more; (c) resident in the Lombardy Region at the time of recruitment. Exclusion criteria were: diagnosis of cancer, heart diseases, stroke, other chronic diseases in the last 5 years. The study conformed to the Declaration of Helsinki and each participant provided written informed consent approved by the Ethics Committee of Fondazione IRCCS Cà Granda Ospedale Maggiore Policlinico (approval number 1425).
Samples Collection
All patients underwent fasting blood sampling around 9 a.m. Peripheral venous blood samples were collected into vacutainer tubes containing EDTA (ethylenediaminetetraacetic acid) disodium salt (9.3 mM; Vacutainer System, Becton Dickinson, Franklin Lakes, NJ, US.); they were centrifuged at 1,200 g for 15 min. Plasma and buffy coat fractions was transferred to separate cryovials, aliquoted and immediately frozen at −80°C until use. Only one aliquot of 1.5 ml of fresh plasma was centrifuged to prepare EV pellets at 1,000, 2,000, and 3,000 g for 15 min at 4°C. The pellet was discarded to remove cell debris.
Laboratory Measurements and Beck Depression Inventory II (BDI-II) Evaluation
Demographic, clinical and biochemical data were obtained in all subjects as previously described (25). All participants were evaluated according to the Beck Depression Inventory II (BDI-II), considered as an appropriate tool to evaluate depressive symptoms in subjects with medical comorbidities such as obesity (26). The following scores correspond to the different severity of depressive symptoms: minimal changes = 0–13, mild depression = 14–19, moderate depression = 20–28, and severe depression = 29–63.
BDNF and PCSK9 Evaluation
Plasma BDNF and PCSK9 concentrations were measured by commercial ELISA kits (Biosensis, South Australia and R&D Systems, MN, respectively) as previously described (12, 25).
BDNF Polymorphism
DNA was extracted from each buffy coat by the Wizard Genomic DNA purification kit (Promega, Madison, WI, USA), according to the manufacturer's instructions. BDNF polymorphism rs6265 (C/T) was selected for the current study, and analyzed using TaqMan® SNP Genotyping Assay (C_11582758_10, Applied Biosystems, San Diego, CA, USA). In a volume of 7 μl, containing 15 ng gDNA sample (3 μl), 0.35 μl TaqMan® Assay (20X), 3.5 μl TaqPath™ ProAmp™ Master Mix and 0.15 μl Nuclease-Free Water, the Polymerase Chain Reaction (PCR) was carried out as follows: pre-read 60°C for 30 s, initial denaturation 95°C for 5 min, followed by 40 cycles of denaturing at 95°C for 15 s and annealing/extension at 60°C for 60 s; a final step post-read 60°C for 30 s.The reactions and analysis were conducted on Quant Studio 12KFlex Real-Time PCR System (Applied Biosystems, San Diego, CA, USA).
Extracellular Vesicles-miRNAs Isolation and Analysis
To prepare the EV pellets for miRNA extraction, fresh plasma deprived of cell debris was transferred to a 10.4-ml polycarbonate ultracentrifuge tube (Beckman Coulter), filled with PBS. Plasma was ultracentrifuged (BeckmanCoulter Optima-MAX-XP) at 110,000 g for 75 min at 4°C and decanted. The EV pellet was stored at −80°C until use. Isolation of miRNAs from plasma extracellular vesicles (EVs) was performed with the combination of miRNeasy kit and RNeasy Cleanup Kit (Qiagen), according to the manufacturer's protocol. MiRNAs were eluted in 20 μL of Nuclease-Free Water and stored at −80°C, until use. MiRNAs reverse transcription (RT) and preamplification reactions, followed by real-time RT-PCR analysis with the QuantStudio™ 12K Flex OpenArray® Platform (Applied Biosystems), were previously described (27). Gene Expression Suite Software (Applied Biosystems) was used to process miRNA expression data from the “TaqMan™ OpenArray™ Human MicroRNA panel” (ThermoFisher) analysis. To elucidate the possible role of the EV-miRNAs, we performed a miRNA target analysis using SpidermiR by R software (v 4.0.4). Then, we compared genes obtained by the miRNA target analysis, with genes associated with thrombosis, atherosclerosis, atherothrombosis, cardiovascular disease, and depression downloaded from DisGeNET v 7.0. Finally, genes targeted by identified miRNAs and associated with atherothrombosis and depression were used to create a network on STRING v 11.5.
Statistical Analysis
Descriptive statistics were performed on all variables. Continuous data were expressed as the mean ± standard deviation (SD) or as the median and interquartile range (Q1–Q3), as appropriate. Categorical data were presented as frequencies and percentages. We applied univariate and multivariable negative binomial regression models for over-dispersed count observations to evaluate the relationship between BDI-II score and BDNF levels. We tested the presence of over-dispersion based upon the Lagrange Multiplier (LM) test. Continuous variables were tested for normality and linearity. Multivariable analyses were adjusted for variables significantly related to the outcome in univariate analysis (p < 0.05). Given the existence of multicollinearity among predictor variables (e.g., HOMA-IR, QUICKI) the variance inflation factor (VIF) statistic was calculated. To determine the best-performing model, we ran several regression equations separately, that included one or more significant explanatory variables used to predict BDI-II score. For each model, we separately estimated the respective β coefficient and standard error (SE) for each variable in the model, p-value, VIF statistics, as well as goodness of fit of the model (R2). Finally, the best model selected to predict the association between BDI-II score and BDNF levels was adjusted for gender, BMI, education, occupation, lifestyle, BDNF rs6265, use of antidepressant drugs, PCSK9, MAP, glycated hemoglobin, HOMA-IR, use of diabetes medications, fibrinogen, and platelets. Estimated effects were described as a percent variation associated with an increase of 1 pg/mL in BDNF concentration. The percent variation was defined as [incidence rate ratio (IRR)-1]*100, IRR correspond to exp(β).
To examine the potential effect modification by IFN-γ, we added the interaction term BDNF*IFN-γ to the multivariable selected model. We evaluated whether the effect of BDNF on depression score differs depending on IFN-γ concentrations. Linear regression models were used to evaluate the associations between BDNF levels and demographics and clinical characteristics of participants.
Multivariable linear regression models were applied to verify the association between miRNA expression and BDNF levels. miRNA expression values were log2-transformed to achieve a normal distribution. The regression models were adjusted for age, gender, BMI, smoking habits. Due to the high number of comparisons, we applied multiple comparison correction methods based on the Benjamini-Hochberg False Discovery Rate (FDR) to calculate the FDR P-value. A volcano plot of Δ% vs. –log10 P-values was used to display results. All statistical analyses were performed with SAS software (version 9.4; SAS Institute Inc., Cary, North Carolina, USA). A two-sided p-value of 0.05 was considered as statistically significant.
Results
Study Population
As shown in Table 1, the majority of the 743 individuals (aged 50.8 ± 13.5) were females (73.6 vs. 26.4%). Mean BMI was 33.3 ± 5.4 kg/m2 and the waist circumference (WC) was 100.7 ± 13.2 cm. Those not exercising physically were 60.2%, compared to 37.7% physically active. Blood pressure was in the normal range, 36.6% of participants being on hypotensive medications. The mean values of total cholesterol (TC), low-density lipoprotein cholesterol (LDL-C) and non-high-density lipoprotein cholesterol (non-HDL-C) were in the upper range of normal (TC 212.6 ± 39.8 mg/dL, LDL-C 133.4 ± 35.3 mg/dL, and non-HDL-C 153.8 ± 40.1 mg/dL). Only 10.1% of subjects were on statin medications. HDL-C and triglyceride (TG) levels were also in the normal range: mean 58.8 ± 14.9 mg/dL and median 102.2 (75.0, 144) mg/dL, respectively. Mean levels of PCSK9 were 276.3 ± 102.6 ng/mL.
Complete blood count (CBC), white blood cell formula, and platelets were in the normal range. Mean thyroid-stimulating hormone (TSH) levels were normal: 1.8 (1.2, 2.4) μU/ml and no participants were on thyroid substitution/suppression therapies. 16.7% were current smokers and C-reactive protein (CRP) median levels were 0.3 (0.1, 0.6) mg/L. The profile of inflammatory cytokines, namely, IFN-γ (10.4 ± 5.4 pg/mL), IL-8 (9.7 ± 9.9 pg/mL), IL-10 (4.2 [3.1;5.6] pg/mL), IL-18 (276.5 [208.5;356] pg/mL), macrophage inflammatory protein-1 α (MIP-1α) (87 [58.5;120] pg/mL), macrophage inflammatory protein-1β (MIP-1β) (186.5 [147;234] pg/mL), monocyte chemoattractant protein-1 (MCP-1) (174 [142;215.5] pg/mL), and TNF-α (5.7 [3.2;8.5] pg/mL) is reported in Supplementary Table 1.
Concerning the glycaemic control, median basal fasting plasma glucose (FPG) was 91.0 (86, 100) mg/dL, median insulin was 12.4 (8.5, 17.8) UI/ml and median glycated hemoglobin (HbA1C) 38.7 (29, 30) mmol/mol; 7.5% were on antidiabetic medications. There were no significant abnormalities in the standard laboratory tests including liver enzymes (AST, ALT, GGT).
Average BDNF plasma concentration was 9.4 ± 5.6 pg/mL (Figure 1 and Table 1). To dissect the impact of the BDNF Val66Met variant, the entire cohort was genotyped for the rs6265 BDNF SNP. Carriers of BDNFVal/Val were 62.6%, of BDNFVal/Met 32.2%, and of BDNFMet/Met 5.2%. The presence of the Met allele did not affect circulating levels of BDNF. These were 10.5 ± 7.2 (BDNFMet/Met), 9.1 ± 5.1 (BDNFVal/Met), and 9.4 ± 5.7 (BDNFVal/Val) pg/mL (p = 0.3503).
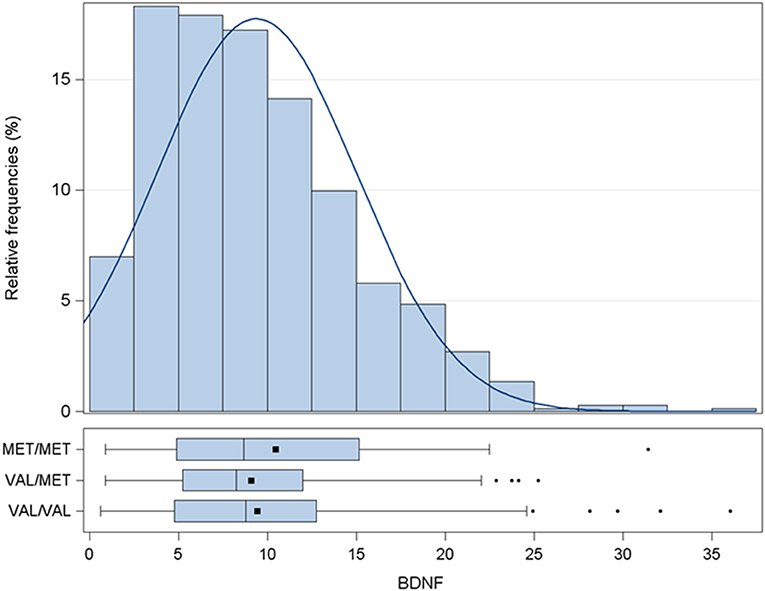
Figure 1. Distribution of BDNF levels (pg/mL). Data are presented as histogram and box-plot grouped by BDNF rs6265 SNP. MET/MET stands for carriers of BDNFMet/Met; VAL/MET stands for carriers of BDNFVal/Met; VAL/VAL stands for carriers of BDNFVal/Val. BDNF, brain derived neurotrophic factor; SNP, Single Nucleotide Polymorphism.
The median BDI-II total score was 9 (5, 14), 1.5% of participants showing severe depression. BDI-II values were higher in women compared to men, namely, 11.9 ± 7.9 and 6.8 ± 5.8, respectively (p < 0.0001). Less than 13% of the whole cohort was treated with antidepressant medications, namely, selective serotonin or norepinephrine/serotonin reuptake inhibitors. Antidepressant treatment was generally prescribed to subjects with moderate and severe disease, the therapeutic approach being quite homogeneously distributed among the different clusters of depression severity (Table 1).
Relation Between BDI-II Score and Selected Study Variables
In univariate negative binomial regression analysis (Supplementary Table 2), BMI, fibrinogen, glycated hemoglobin, HOMA-IR score, QUICKI-score, PCSK9, platelets, employment status, use of antidepressant medications, were positively associated with the BDI-II score. A negative association was found for education, lifestyle, blood pressure, pre-diabetes, use of antidepressant medications and diabetes medications (Supplementary Table 2).
In the multivariate regression analysis, other variables were positively associated with the severity of depression: the HOMA-IR (β = 0.0293, SE = 0.125, p = 0.019), PCSK9 (β = 0.0007, SE = 0.0003, p = 0.0159) and platelet count (β = 0.0017, SE = 0.0005, p = 0.0018) were positively associated with the severity of depression (BDI-II score) (Table 2). In the univariate analysis (Supplementary Table 2), the severity of depression (BDI-II score) did not significantly associate with plasma BDNF levels (β = −0.009, SE = 0.005, p = 0.087), whereas this association became significant in multivariate analysis. For every one-unit increase of BDI-II, BDNF levels decreased by 1.93% (β = −0.0195, SE = 0.0056, p = 0.0004) (Table 3). No relationship between BDI-II score and BDNFVal66Met polymorphism was found (Supplementary Table 2).
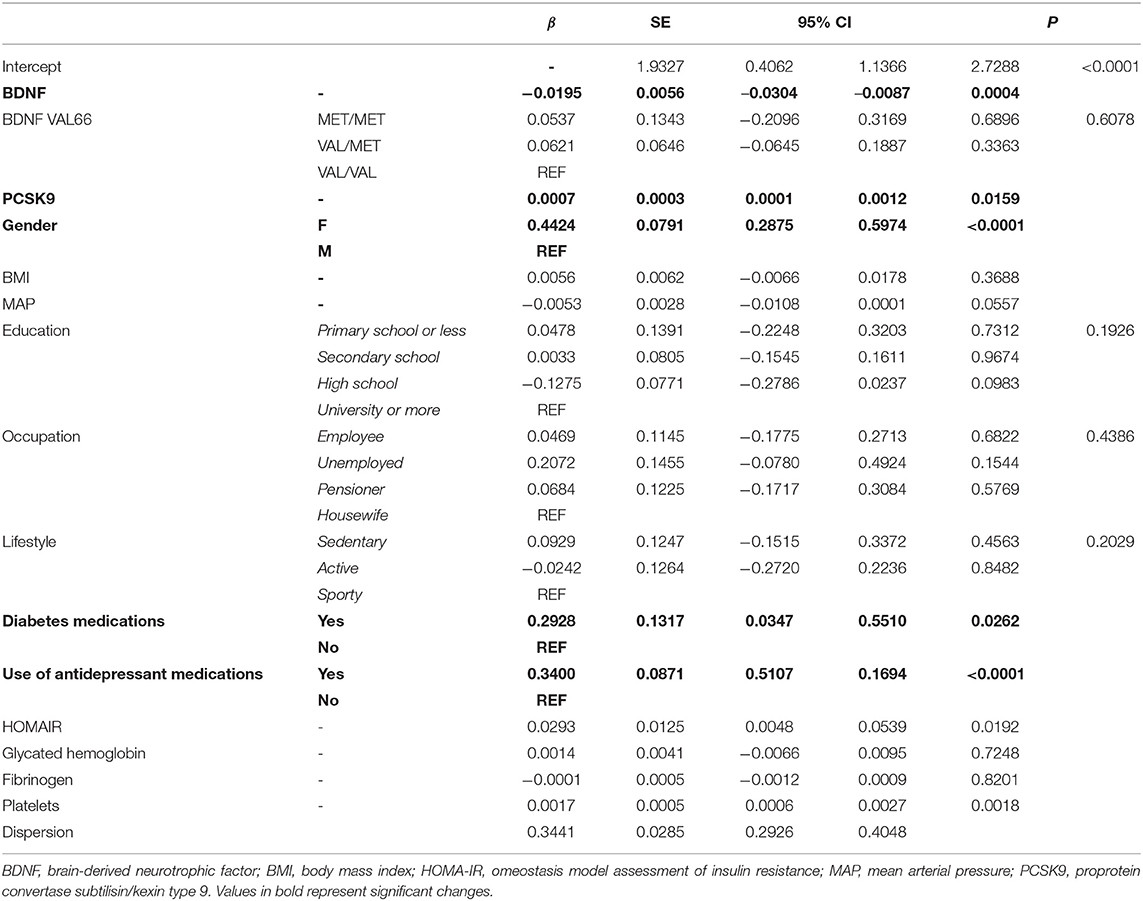
Table 2. Negative binomial regression model evaluating the association between BDI-II score and BDNF adjusted for participant's characteristics.
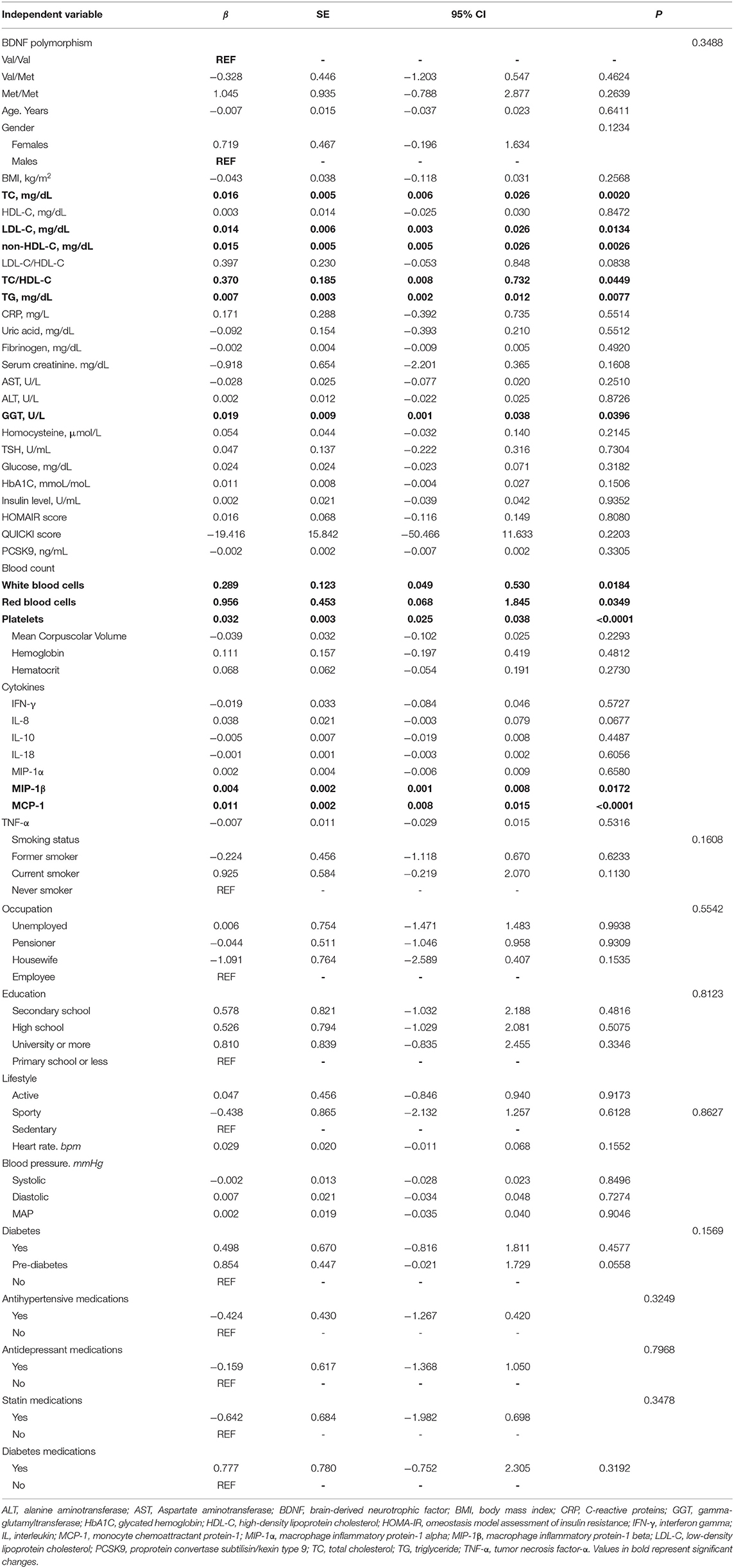
Table 3. Slope coefficients from univariate linear regression models to evaluate association between BDNF and demographics and clinical characteristics of participants.
Similarly, the association between BDI-II score and the use of antidepressants and of diabetes medications were robust even after adjusting for the relevant covariates (Table 2).
Role of Inflammatory Markers in the Association Between Plasma BDNF Levels and BDI-II Score
Since inflammation has been linked to depression (28), the impact of the inflammatory environment on the previous associations was evaluated. Among the different tested cytokines (Supplementary Table 1), IFN-γ significantly modified the association between BDNF and BDI-II (interaction term p-value = 0.011). The strength of the association was modified in different IFN-γ strata. Each unit rise in BDNF led to an incidence rate ration (IRR) of 0.963 (95% CI 0.948–0.978), of 0.973 (95% CI 0.962–0.984), of 0.986 (95% CI 0.975–0.0997), and of 0.999 (95% CI 0.983–1.014), respectively, at IFN-γ levels of 0.65, 5, 10.4 and 15.8 pg/mL (Figure 2). No significant association between the different tested cytokines and BDI-II score was found (Supplementary Table 2).
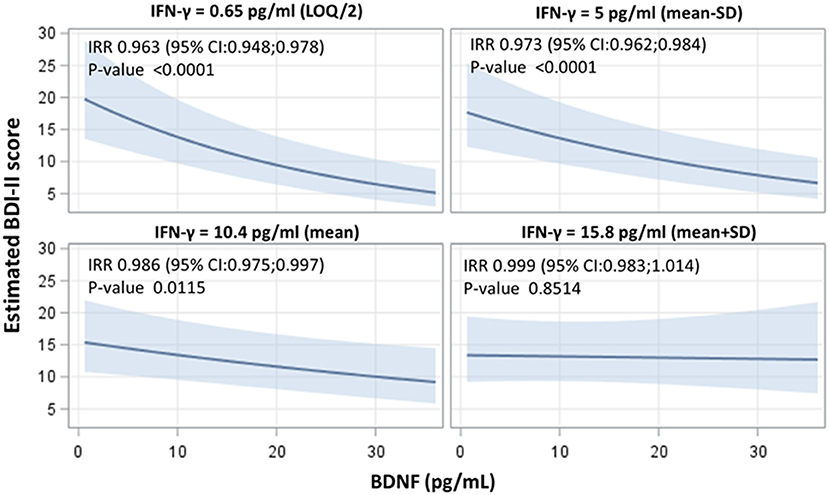
Figure 2. Interactive effects of BDNF and IFN-γ on the BDI-II score, indicating the strength of association between BDNF concentrations and BDI-II score at four selected levels of IFN-γ (LOQ/2), mean - standard deviation (SD), mean and mean ± SD value). Adjusted incidence rate ratios (IRR) were reported for one unit increase in BDNF concentrations, at each IFN-γ level. IRR correspond to exp(β); BDNF, Brain-Derived Neurotrophic Factor; BDI-II, Beck Depression Inventory-II; IFN-γ, interferon gamma.
Association Between BDNF and CV Risk Factors
BDNF levels were positively associated with CV parameters: TC (β = 0.016, SE 0.005, p = 0.0020), LDL-C (β = 0.014, SE = 0.006, p = 0.0134), non-HDL-C (β = 0.015, SE = 0.005, p = 0.0026), and TG (β = 0.007, SE = 0.005, p = 0.0077). In addition, a positive association between BDNF and platelets (β = 0.032, SE = 0.003, p < 0.0001), white (β = 0.289, SE = 0.123, p = 0.0184), and red (β = 0.956, SE = 0.453, p = 0.0349) blood cells were found. Among the tested proinflammatory cytokines, MIP-1β (β= 0.004, SE = 0.002, p = 0.0172) and MCP-1 (β= 0.011, SE = 0.002, p < 0.0001) were positively associated with plasma BDNF levels (Table 3).
EVs-Derived miRNA, Atherothrombosis, and Depression
To further dissect the possible involvement of BDNF in CV disease, we analyzed EV-derived miRNAs isolated from the same individuals. After data cleaning, 508 miRNAs were expressed in at least one subject. In a model adjusted for age, gender, BMI, and smoking habit, BDNF levels were associated with a significant increase of 80 miRNAs (the up-regulated group) and a significant decrease of 59 miRNAs (the down-regulated group), after FDR adjustment for multiple comparisons (FDR p < 0.05; Figure 3 and Supplementary Table 3).
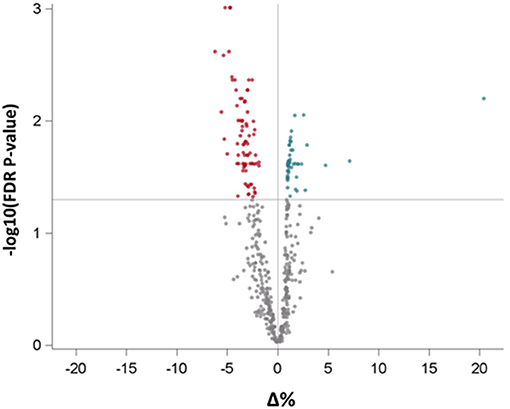
Figure 3. Volcano plot reporting the association of BDNF levels with all measured miRNAs in extracellular vesicles. Red and green dots represent miRNAs, respectively, with negative and positive statistically significant association with BDNF. BDNF, Brain-Derived Neurotrophic Factor.
By using the DisGeNET database, we identified the genes targeted by these 139 miRNAs specifically related to thrombosis, atherosclerosis, atherothrombosis and CV disease. Among the group of up-regulated miRNAs targeted genes (11,890), 1,091 were associated with CV disease, 1,253 with atherosclerosis, 60 with thrombosis, and 69 with atherothrombosis (Supplementary Figure 1). In particular, they represented more than 50% of total genes included in the databases of atherothrombosis and more than 60% of those included in the databases of thrombosis, atherosclerosis and CV disease.
Among the group of down-regulated miRNAs targeted genes (11,121), 1,008 were associated with CV disease, 1,180 with atherosclerosis, 50 with thrombosis, and 63 with atherothrombosis (Supplementary Figure 2). In particular, they represented between 50 and 57% of total genes included in the four datasets. Among all the identified target genes, 18 were reported in all four datasets (thrombosis, atherosclerosis, atherothrombosis, and cardiovascular disease). Specifically, 15 target genes were associated with the group of up-regulated miRNAs and 13 target genes with the down-regulated group. The following 10 target genes CYP2C19, F3, IL6, MTHFR, PLAT, PTGS 2, SERPINE 1, TFP1, THBD, and TNF were shared between the up- and down-regulated groups (Supplementary Table 4).
In silico multiple-protein interaction analysis (STRING) revealed that all 18 target genes (AC2, PTGS2, CYP2C19, IL18, IL6, TNF, PTGS1, MTHFR, LPA, PLAT, SERPINE1, SELP, F3, VWF, THBD, CPB2, TFPI, and PROC) directly or indirectly interacted with BDNF (Figure 4). Overall, these data support the important relationship between circulating BDNF levels and increased CV risk in this population.
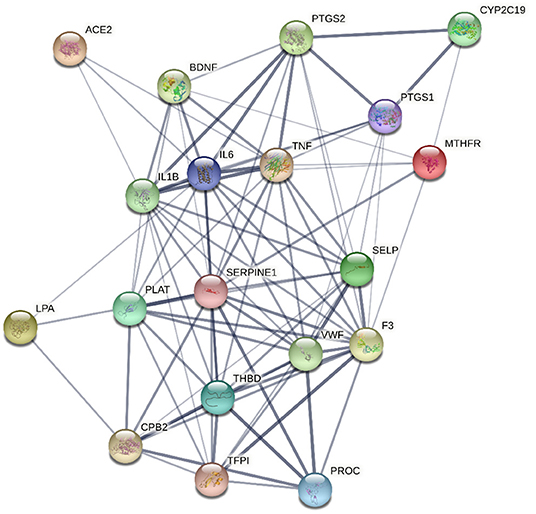
Figure 4. Network, constructed by the STRING protein-protein interaction network tool, of the 18 genes targeted by miRNAs associated with Brain-Derived Neurotrophic Factor and related to thrombosis, atherosclerosis, atherothrombosis, and cardiovascular disease. The line thickness represents strength of the interaction.
Finally, in order to dissect the potential relationship among BDNF, obesity, atherothrombosis and depression, we performed a further analysis by using specific datasets of the DisGeNET pertaining to depression, i.e., chronic depression, clinical depression, mild depression, recurrent depression and severe depression. We found that 51 out of 11,890 genes targeted by up-regulated miRNAs were associated with depression, of which 8 were reported also in the atherothrombosis database (Supplementary Table 5). Regarding the genes targeted by down-regulated miRNAs, 52 out of 11,121 were related to depression, 7 of which were also found in the atherothrombosis dataset (Supplementary Table 6). Interestingly, BDNF emerged among the genes shared by depression and atherothrombosis, and it was well-connected to all the genes identified in both databases, namely, IL-1β, IL-6, IL-18, TNF, APOE, ACE, MTR, MTHFR genes (Figure 5).
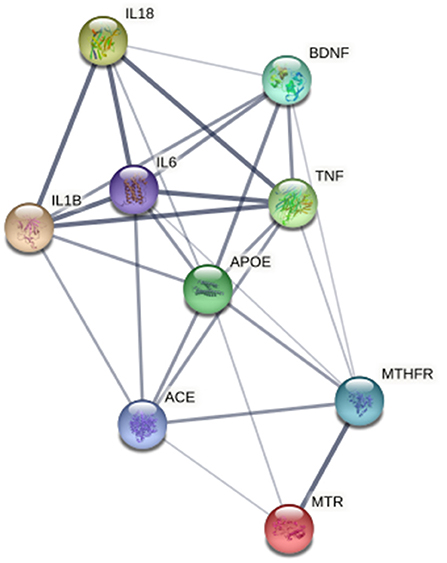
Figure 5. Network, constructed by the STRING Protein–Protein interaction network tool, depict interactions, among the 7 genes targeted by miRNA associated with Brain-Derived Neurotrophic Factor and related to both atherothrombosis and depression. The line thickness represents strength of the interaction.
Discussion
In the present study by using data from an Italian cohort of obese individuals, we identified a significant negative association between depression scores and BDNF plasma levels, a finding that was modified by elevated inflammatory levels of IFN-γ. In addition, we provided evidence that the amount of circulating BDNF can reflect the EV-derived miRNAs related to CV disease and depression, supporting the hypothesis that BDNF may be the link between mood disorders and cardiometabolic diseases. Depression development is linked to several factors among which obesity most likely has a causal role. Mendelian randomization studies have provided clear evidence that high BMI is causally linked to the likelihood to develop depression (31).
Among central nervous system (CNS) determinants of obesity, BDNF could exert a potentially significant role. The relationship of BDNF with obesity and depression has been the subject of many investigations which collectively described a reduction of brain BDNF levels in both obesity and depression. Indeed, by contributing to the regulation of both synaptic plasticity and energy metabolism, including feeding behavior, BDNF has been recognized as a key target in the relationship between metabolic syndrome and psychiatric diseases. BDNF by activation of tyrosine kinase receptor-B (Trk-B) promotes neuronal survival, neuroplasticity, synaptogenesis, and survival and differentiation of serotonergic neurons through different signaling, such as PLC/DAG/IP3, PI3K/Akt, and Ras/MAPK-ERK pathways. BDNF can modulate the release of several neurotransmitters by interacting with other neuropeptides. Specifically, BDNF may regulate serotonin release (32), and modulates dopamine release via PLC-γ/IP3 pathway (33). Additionally, it may facilitate glutamate release through the MAPK/SynI activation (34), and controls GABAergic synaptic transmission influencing neuronal excitability. Reduction of BDNF levels affects formation of synaptic spine density, and decreases excitatory neurons leading to depression (35). BDNF can also interact with melanocortin, leptin, corticotrophin-releasing hormone (CRH), and thyrotropin-releasing hormone (TRH) playing critical physiopathological roles. BDNF and TrkB play a significant role in the neural circuitry triggering food intake and body weight, regulating appetite through the modulation of melanocortin signaling and hypothalamic serotonergic mechanisms. Pre-clinical data have shown that central alterations of BDNF signaling in mice results in severe hyperphagia and obesity (14, 36). On the other hand, high-fat diet promotes obesity, depressive-like phenotypes and reduction of BDNF in the hippocampus, a brain region important in controlling the mood. Moreover, hippocampal reductions of BDNF have been also reported in animal models of depression and in the postmortem brain samples of depressed patients (35). Interestingly, BDNF is potentially associated with obesity, depression and coronary syndromes (22). Being a versatile regulator of food intake as well as of inflammatory adipocyte maturation, it has been considered a critical mediator of obesity (14). In addition, compared to healthy subjects, reduced levels of BDNF have been found in patients with coronary syndromes (11, 12), and elevated BDNF appears to exert a protective effect (15). However, an open question remains as to whether BDNF has a fundamental role in CV complications, or it is just a bystander.
Waiting for the understanding of whether “came first the chicken or the egg,” several mechanisms have been proposed, starting from the knowledge that BDNF plays a cardio-protective role thanks to its antioxidant, pro-survivor and pro-angiogenic properties. It promotes the activation of reactive oxygen species detoxifying enzymes, increases cardiomyocyte survival under hypoxic conditions, stimulates new vessel assembly, and affects the structure, polymerization and viscoelastic properties of the fibrin clot, thus influencing the anticoagulation process (12, 37), without changing circulating fibrinogen levels (12, 29). According to these data (12, 29), in the SPHERE population no association between BDNF and fibrinogen levels was found. However, the ability of BDNF to modify fibrin clot structure suggests its clinical relevance as a potential biomarker of thrombosis including acute coronary syndrome (ACS). Indeed, as emerged from the PLATO study, fibrin clot properties independently predict adverse clinical outcomes after ACS (38). Future studies in depressed obese subjects should be performed to dissect out the potential relationship between BDNF and fibrin clot properties.
Interestingly, in a human study, Wu et al. found a positive correlation between severity of coronary artery stenosis and depression scores, and reported that lower serum BDNF levels were closely related to the development of depression in patients with stable coronary artery disease (39). Since it is well-established that reduced BDNF levels in the brain are linked to obesity, BDNF may represent the bridge among obesity, depression, and CV diseases. However, whether obesity itself may influence circulating BDNF levels is not well-defined as yet (22). In the present study, plasma BDNF levels did not correlate with BMI, whereas an evident relationship with BDI-II emerged, overall suggesting the strong impact of BDNF on depression. The lack of follow-up of the SPHERE study did not allow to assess whether antidepressant medications were able to raise plasma BDNF levels. We did not find any interaction between the antidepressant medications and BDNF levels (p = 0.9013) as well as between antidepressant medications and BDNF rs6265 (p = 0.0603) on BDI-II score.
Alterations in GABAergic, catecholaminergic and serotonergic systems as well as an aberrant cytokine production related to dysregulation of both innate and adaptive immune systems have been reported in people suffering from depression when compared to healthy individuals (40, 41). However, no significant correlations between catecholamine metabolites and BDNF in the blood of subjects with major depression have been reported (42), and platelet serotonin levels do not seem to go hand in hand with BDNF levels. Platelet serotonin levels do not differ between depressed and control subjects, whereas both reduced platelet BDNF content and plasma BDNF levels were found in subjects with depression (20, 30, 43). Then, it has been hypothesized that the altered BDNF levels observed in patients with depression are due to a reduced release from platelets which are considered one of the major circulation sources of BDNF. This latter is released by platelets upon their activation, raising to 100-fold higher levels in serum vs plasma (44) and correlating positively with platelets number (45). However, in people with depression, the reduction in serum and plasma BDNF levels seem to be independent of platelet reactivity (20), suggesting that other cells are involved in this modulation.
Recently, awareness has been raised regarding the relationship among obesity, depression and diet-related inflammation (46, 47). Gialluisi et al. (46) showed that the low-grade inflammation (INFLA)-score, based on four circulating inflammatory biomarkers (C-reactive protein, granulocyte-to-lymphocyte ratio, platelet and white blood cell counts), could explain the association between depressive symptoms and the dietary inflammatory index.
Considering that the studied cohort predominantly comprises overweight individuals, our findings are two fold: depression is directly related to a higher number of platelets, as in normal weight people (48); and the positive association between platelet number and depression score was mitigated by BDNF (Supplementary Figure 3). Altogether, this suggests that other cell populations (49, 50) could actively affect circulating BDNF levels. On this matter, we found that BDNF was positively correlated with white and red blood cells. This correlation can be explained, at least in part, by the ability of peripheral blood mononuclear (PBMC) to produce/secret BDNF (51, 52) that in turn can promote cell proliferation and survival. Indeed, BDNF induces the maturation, activation, proliferation and survival of T and B lymphocytes (49, 50). Since TrkB receptor was found in several bone marrow cells including osteoblasts, eosinophils, mastocytes, megakaryocytes and erythroblasts (49), it is possible to hypothesize that BDNF can affect also the behavior of these cell types.
Accumulating evidence shows that chronic inflammation is associated with depressive symptoms, and pre-clinical evidence supports the critical involvement of IFN-γ in the pathogenesis of this disease (53). In the SPHERE population, we did not measure levels of catecholamines, acetylcholine, serotonin, or of other neurotransmitters, but several cytokines have been evaluated. Interestingly, we did not find evidence of an association between BDI-II score and the measured cytokines, although IFN-γ affected the association BDNF-depression. The evidence that modulation of the BDNF-depression association occurs primarily at low IFN-γ levels suggests that under elevated inflammatory conditions, as found, e.g., in severe obesity (54), the ability of BDNF to impact on depressive symptoms is potentially negligible. In vitro data showed that T cells co-secreted larger amounts of BDNF and IFN-γ upon CD40 activation, whereas activated monocytes produced BDNF but not IFN-γ (55). Interestingly, obese patients displayed high levels of CD40 Ligand (56) suggesting that in this clinical condition activation of PBMC, rather than platelets, could be an important source of circulating BDNF.
The increased circulating levels of BDNF paralleled by the enhanced expression of the inflammatory markers MCP-1 and MIP1β, might be a consequence of ongoing pathological events. Abundant BDNF is present in the atherosclerotic lesions localizing in macrophages, vascular smooth muscle cells, as well as in perivascular adipose tissue (13, 37, 57). It may be secreted by activated monocytes in response to inflammatory cytokines (58) to exert anti-inflammatory and anti-apoptotic effects. However, when BDNF is chronically expressed in the presence of severe inflammatory conditions, it could have deleterious effects. BDNF enhances oxidative stress and promotes tissue factor (TF) activity in monocyte/macrophages (13, 51) and down regulates TrkB (57). Reduction in TrkB expression can contribute to atherosclerosis progression by affecting endothelial and smooth muscle cells survival and function, as well as heart development (37). Interestingly, our group showed that despite circulating levels of BDNF being lower in CV patients compared to healthy controls, a positive relationship between plasma BDNF and coronary plaque vulnerability, also including macrophage infiltration, appears to be present (11).
The relationship between the BDNFVal66Met polymorphism and depression is currently under debate. Specifically, the systematic review and meta-analyses performed by Kishi et al. (59) did not found any association between this polymorphism and depression, while noting that plasma BDNF levels were reduced in depressed patient. On the contrary, a positive association between this polymorphism and depression only in men, but not in women, was found by Verhagen (60). Similarly, the genetic association studies of the BDNFVal66Met polymorphism in obesity and cardiometabolic diseases provided inconsistent results. BDNF polymorphism could not differentiate carriers of Val vs. Met based on BMI (61, 62), or with CNS BDNF levels in suicidal depressed patients (63). However, this polymorphism may increase the risk of developing obesity when associated with the other polymorphisms related to the metabolic pathway (62). By contrast, other reports showed both a positive and an inverse association between obesity and the Met allele (64, 65). Finally, a greater adiposity based on BMI percentiles or BMI-for-age z-scores has been also described in Met allele carriers (61). In agreement with these studies, in the SPHERE cohort we did not find differences in BDNF allele frequency in relation with BMI, depression and circulating BDNF levels. Specific additional studies, including also subjects with a normal weight, may be needed in order to provide more definite conclusions.
Finally, by analyzing the EV-miRNA profiles we found that 139 miRNAs, known to target genes involved in atherothrombotic and CV processes, were associated with BDNF levels. Even if evidence on the ability of BDNF to directly modulate miRNAs expression is scanty, BDNF alters the expression of miR-433, miR-19b-1, miR-432, and miR-181c in human endothelial progenitor cells (66), and upregulates miR-214 in mouse embryonic stem cells (67). These findings provide evidence that some of the miRNAs here identified may be affected by BDNF.
Interestingly, the 139 miRNAs here identified target 10 genes (CYP2C19, F3, IL6, MTHFR, PLAT, PTGS2, SERPINE1, TFPI, THBD, TNF) present in all datasets analyzed. Remarkably, the protein-protein interaction analyses showed that BDNF could represent a critical hub among all these genes.
In addition, when data were analyzed focusing on depression state, 9 genes (ACE, APOE, BDNF, IL18, IL1B, IL6, MTHFR, MTR, and TNF) were shared with the atherothrombotic dataset. All these 9 genes were closely interlinked, and BDNF was directly related to 6 of them. Altogether these observations support the hypothesis that BDNF may be a common link among obesity, CV diseases and depression.
Remarkably, ACE has been identified as the target gene for both atherothrombotic complications (68) and management of depression (69). Overexpression of ACE2 in a transgenic animal model attenuated plaque vulnerability and inflammation (70) and reduced the propensity to develop an anxiety phenotype (29). Recently, it has been demonstrated that down regulation of ACE2 by SARS-CoV-2 exacerbates the thrombotic COVID-19 severity and predisposes to depression (71). Changes in the levels of serum BDNF were observed in post-infected-COVID-19 symptomatic patients (72), predicting an intensive care admission and a worse prognosis (73).
In this scenario, Lorkiewicz et al. (74) proposed to include BDNF among biomarkers of COVID-19-derived depression, whereas De Sousa et al. (75) underlined how the rise of BDNF levels, induced by physical activity, was crucial for maintaining good mental health after COVID-19 syndrome.
Of note, alterations in the ACE signaling-pathway affected the release of modulators of fibrinolytic processes, such as Serpin1 and Tissue-type plasminogen activator (PLAT). Although Serpin1 and PLAT were not co-shared between the depression and atherothrombotic phenotypes, it has been hypothesized that PLAT and Serpin1, by modulating the proteolytic ability of plasmin, may regulate the cleavage of pro-BDNF precursor to the mature form (76). All these findings clearly summarize the strong connections of these genes in obesity, depression, and CV disease.
Our analysis also identified the possible involvement of prostaglandin-endoperoxide synthase 2 (PTGS2), a key enzyme in the production of prostanoids. Increased PTGS2 levels have been detected in atherosclerotic plaques (77) as well as in the rat brain showing depression-like phenotypes (78). The imbalance in PTGS2-BDNF signaling pathway has been suggested to be one of the pathogenetic mechanisms of depression (79).
Although P-selectin, thromboglobulin, and MTHFR, a key modulator of purine and thymidylate biosynthesis, play a critical role in thrombosis (80, 81), and their alterations have been observed in subjects with depression (82, 83), definitive potential relationship with BDNF is actually missing.
The present study presents some limitations. Firstly, this is a monocentric study, and the questionnaire was self-administered, without final psychiatric interview diagnosing the depressive state. Moreover, the BDI-II questionnaire was administered only once at the recruitment of patients. BDI-II has been widely applied including earlier studies in cardiac patients (84); having been successfully administered both to adolescents and adults, as a tool of both medical assistance and research, it represents an established self-rating scale. A large portion of subjects classified with depression has subclinical or mild symptomatology, only a fraction of those taking psychotropic medications. Although we collected extensive information on habitual physical activity and education, many other factors may influence BDNF levels, and not all potential confounders could be taken into consideration here. The follow-up of these subjects is not available at present, limiting the potential prognostic value of BDNF for cardiovascular events at this moment. No other traditional markers of predisposition to thrombosis have been measured. Finally, the molecular mechanisms underlying the relation between BDNF and the emerged miRNAs affecting obesity, depression and CVD have not been investigated as yet.
Conclusions
The described link among BDNF and obesity, classical and non-classical CV risk factors in individuals with obesity and different degrees of depression provide one more explanatory variable (i.e., BDNF) as a culprit. In the present report, there was clear evidence of a negative association between BDNF and major clinical depression scores, with no impact of BDNF genetic polymorphisms. Although the association between BDNF and depression risk factors was lost in the presence of elevated levels of a major inflammatory marker (IFN-γ), the detection of this link is novel as was the association with miRNAs related to atherothrombosis and depression. This last analysis detected a large number of up-regulated and down-regulated genes, eventually allowing to create a network analysis of potential use in the molecular characterization of both CV and depression in obese individuals.
Data Availability Statement
The original contributions presented in the study are included in the article/Supplementary Material, further inquiries can be directed to the corresponding authors.
Ethics Statement
The studies involving human participants were reviewed and approved by Ethics Committee of Fondazione IRCCS Cà Granda OspedaleMaggiore Policlinico (approval 1425).
Author Contributions
PA, CM, MZ, and MG performed the experiments. CF performed the statistical analyses. LD isolated the EV-derived miRNAs. GS performed the bioinformatic analyses of miRNAs. LV was responsible for the recruitment of SPHERE cohort. MB was responsible for the evaluation of BDI-II score. LS, AI, AP, and CS critically reviewed the manuscript. SB, MR, and VB conceived the study and wrote the first draft of the manuscript. All authors contributed to the article and approved the submitted version.
Funding
This work was partially supported by Fondazione Cariplo (2018-0511 to MR and 2018-0525 to SB), Banca di Credito Cooperativa di Milano (donation to MR), European Research Council (ERC-2011-StG 282,413 to VB), and Italian Ministry of Health, Rome, Italy (Ricerca Corrente RC 2021, and 5 × 1000 2018).
Conflict of Interest
The authors declare that the research was conducted in the absence of any commercial or financial relationships that could be construed as a potential conflict of interest.
Publisher's Note
All claims expressed in this article are solely those of the authors and do not necessarily represent those of their affiliated organizations, or those of the publisher, the editors and the reviewers. Any product that may be evaluated in this article, or claim that may be made by its manufacturer, is not guaranteed or endorsed by the publisher.
Supplementary Material
The Supplementary Material for this article can be found online at: https://www.frontiersin.org/articles/10.3389/fcvm.2022.906483/full#supplementary-material
References
1. Hales CM, Fryar CD, Carroll MD, Freedman DS, Ogden CL. Trends in obesity and severe obesity prevalence in US youth and adults by sex and age, 2007-2008 to 2015–2016. JAMA. (2018) 319:1723–5. doi: 10.1001/jama.2018.3060
2. Organization WH. Obesity (2021). Available online at: https://www.who.int/health-topics/obesity#tab=tab_1.
3. Powell-Wiley TM, Poirier P, Burke LE, Despres JP, Gordon-Larsen P, Lavie CJ, et al. Obesity and cardiovascular disease: a scientific statement from the American Heart Association. Circulation. (2021) 143:e984–1010. doi: 10.1161/CIR.0000000000000973
4. Luppino FS, de Wit LM, Bouvy PF, Stijnen T, Cuijpers P, Penninx BW, et al. Overweight, obesity, and depression: a systematic review and meta-analysis of longitudinal studies. Arch Gen Psychiatry. (2010) 67:220–9. doi: 10.1001/archgenpsychiatry.2010.2
5. Singh C WM, Solanki RK. A study of cardiovascular risk among depressed obese patients. J Ment Health Hum Behav. (2020) 21:5–9. doi: 10.4103/jmhhb.jmhhb_19_18
6. Macchi C, Greco MF, Favero C, Dioni L, Cantone L, Hoxha M, et al. Associations among Pcsk9 levels, atherosclerosis-derived extracellular vesicles, and their mirna content in adults with obesity. Front Cardiovasc Med. (2021) 8:785250. doi: 10.3389/fcvm.2021.785250
7. Zara M, Guidetti GF, Camera M, Canobbio I, Amadio P, Torti M, et al. Biology and role of Extracellular Vesicles (Evs) in the pathogenesis of thrombosis. Int J Mol Sci. (2019) 20:2840. doi: 10.3390/ijms20112840
8. Amare AT, Schubert KO, Klingler-Hoffmann M, Cohen-Woods S, Baune BT. The genetic overlap between mood disorders and cardiometabolic diseases: a systematic review of genome wide and candidate gene studies. Transl Psychiatry. (2017) 7:e1007. doi: 10.1038/tp.2016.261
9. Gharipour M, Barekatain M, Sung J, Emami N, Sadeghian L, Dianatkhah M, et al. The epigenetic overlap between obesity and mood disorders: a systematic review. Int J Mol Sci. (2020) 21:6758. doi: 10.3390/ijms21186758
10. Colucci-D'Amato L, Speranza L, Volpicelli F. Neurotrophic factor BDNF, physiological functions and therapeutic potential in depression, neurodegeneration and brain cancer. Int J Mol Sci. (2020) 21:7777. doi: 10.3390/ijms21207777
11. Amadio P, Cosentino N, Eligini S, Barbieri S, Tedesco CC, Sandrini L, et al. Potential relation between plasma bdnf levels and human coronary plaque morphology. Diagnostics. (2021) 11:1010. doi: 10.3390/diagnostics11061010
12. Amadio P, Porro B, Sandrini L, Fiorelli S, Bonomi A, Cavalca V, et al. Patho- physiological role of BDNF in fibrin clotting. Sci Rep. (2019) 9:389. doi: 10.1038/s41598-018-37117-1
13. Ejiri J, Inoue N, Kobayashi S, Shiraki R, Otsui K, Honjo T, et al. Possible role of Brain-Derived Neurotrophic Factor in the pathogenesis of coronary artery disease. Circulation. (2005) 112:2114–20. doi: 10.1161/CIRCULATIONAHA.104.476903
14. Rios M. BDNF and the central control of feeding: accidental bystander or essential player? Trends Neurosci. (2013) 36:83–90. doi: 10.1016/j.tins.2012.12.009
15. Kaess BM, Preis SR, Lieb W, Beiser AS, Yang Q, Chen TC, et al. Circulating Brain-Derived Neurotrophic Factor concentrations and the risk of cardiovascular disease in the community. J Am Heart Assoc. (2015) 4:e001544. doi: 10.1161/JAHA.114.001544
16. Rawson KS, Dixon D, Nowotny P, Ricci WM, Binder EF, Rodebaugh TL, et al. Association of functional polymorphisms from brain-derived neurotrophic factor and serotonin-related genes with depressive symptoms after a medical stressor in older adults. PLoS ONE. (2015) 10:e0120685. doi: 10.1371/journal.pone.0120685
17. Amadio P, Colombo GI, Tarantino E, Gianellini S, Ieraci A, Brioschi M, et al. Bdnfval66met polymorphism: a potential bridge between depression and thrombosis. Eur Heart J. (2017) 38:1426–35. doi: 10.1093/eurheartj/ehv655
18. Sandrini L, Castiglioni L, Amadio P, Werba JP, Eligini S, Fiorelli S, et al. Impact of BDNF Val66met polymorphism on myocardial infarction: exploring the macrophage phenotype. Cells. (2020) 9:1084. doi: 10.3390/cells9051084
19. Jiang H, Liu Y, Zhang Y, Chen ZY. Association of plasma brain-derived neurotrophic factor and cardiovascular risk factors and prognosis in angina pectoris. Biochem Biophys Res Commun. (2011) 415:99–103. doi: 10.1016/j.bbrc.2011.10.020
20. Karege F, Bondolfi G, Gervasoni N, Schwald M, Aubry JM, Bertschy G. Low Brain-Derived Neurotrophic Factor (BDNF) levels in serum of depressed patients probably results from lowered platelet BDNF release unrelated to platelet reactivity. Biol Psychiatry. (2005) 57:1068–72. doi: 10.1016/j.biopsych.2005.01.008
21. Sandrini L, Ieraci A, Amadio P, Zara M, Mitro N, Lee FS, et al. Physical exercise affects adipose tissue profile and prevents arterial thrombosis in BDNF Val66met mice. Cells. (2019) 8:875. doi: 10.3390/cells8080875
22. Sandrini L, Di Minno A, Amadio P, Ieraci A, Tremoli E, Barbieri SS. Association between Obesity and Circulating Brain-Derived Neurotrophic Factor (BDNF) levels: systematic review of literature and meta-analysis. Int J Mol Sci. (2018) 19:2281. doi: 10.3390/ijms19082281
23. Losenkov IS, Mulder NJV, Levchuk LA, Vyalova NM, Loonen AJM, Bosker FJ, et al. Association between BDNF gene variant Rs6265 and the severity of depression in antidepressant treatment-free depressed patients. Front Psychiatry. (2020) 11:38. doi: 10.3389/fpsyt.2020.00038
24. Bollati V, Iodice S, Favero C, Angelici L, Albetti B, Cacace R, et al. Susceptibility to particle health effects, mirna and exosomes: rationale and study protocol of the sphere study. BMC Public Health. (2014) 14:1137. doi: 10.1186/1471-2458-14-1137
25. Macchi C, Favero C, Ceresa A, Vigna L, Conti DM, Pesatori AC, et al. Depression and cardiovascular risk-association among beck depression inventory, Pcsk9 levels and insulin resistance. Cardiovasc Diabetol. (2020) 19:187. doi: 10.1186/s12933-020-01158-6
26. Schneider KL, Busch AM, Whited MC, Appelhans BM, Waring ME, Pagoto SL. Assessing depression in obese women: an examination of two commonly-used measures. J Psychosom Res. (2013) 75:425–30. doi: 10.1016/j.jpsychores.2013.08.015
27. Pergoli L, Cantone L, Favero C, Angelici L, Iodice S, Pinatel E, et al. Extracellular vesicle-packaged mirna release after short-term exposure to particulate matter is associated with increased coagulation. Part Fiber Toxicol. (2017) 14:32. doi: 10.1186/s12989-017-0214-4
28. Miller AH, Raison CL. The role of inflammation in depression: from evolutionary imperative to modern treatment target. Nat Rev Immunol. (2016) 16:22–34. doi: 10.1038/nri.2015.5
29. Wang L, de Kloet AD, Pati D, Hiller H, Smith JA, Pioquinto DJ, et al. Increasing brain angiotensin converting enzyme 2 activity decreases anxiety-like behavior in male mice by activating central mas receptors. Neuropharmacology. (2016) 105:114–23. doi: 10.1016/j.neuropharm.2015.12.026
30. Sagud M, Nikolac Perkovic M, Vuksan-Cusa B, Maravic A, Svob Strac D, Mihaljevic Peles A, et al. A prospective, longitudinal study of platelet serotonin and plasma brain-derived neurotrophic factor concentrations in major depression: effects of vortioxetine treatment. Psychopharmacology. (2016) 233:3259–67. doi: 10.1007/s00213-016-4364-0
31. Tyrrell J, Mulugeta A, Wood AR, Zhou A, Beaumont RN, Tuke MA, et al. Using genetics to understand the causal influence of higher BMI on depression. Int J Epidemiol. (2019) 48:834–48. doi: 10.1093/ije/dyy223
32. Homberg JR, Molteni R, Calabrese F, Riva MA. The serotonin-BDNF duo: developmental implications for the vulnerability to psychopathology. Neurosci Biobehav Rev. (2014) 43:35–47. doi: 10.1016/j.neubiorev.2014.03.012
33. Neal M, Cunningham J, Lever I, Pezet S, Malcangio M. Mechanism by which brain-derived neurotrophic factor increases dopamine release from the rabbit retina. Invest Ophthalmol Vis Sci. (2003) 44:791–8. doi: 10.1167/iovs.02-0557
34. Jovanovic JN, Czernik AJ, Fienberg AA, Greengard P, Sihra TS. Synapsins as mediators of BDNF-enhanced neurotransmitter release. Nat Neurosci. (2000) 3:323–9. doi: 10.1038/73888
35. Yang T, Nie Z, Shu H, Kuang Y, Chen X, Cheng J, et al. The role of BDNF on neural plasticity in depression. Front Cell Neurosci. (2020) 14:82. doi: 10.3389/fncel.2020.00082
36. Ieraci A, Barbieri SS, Macchi C, Amadio P, Sandrini L, Magni P, et al. BDNF Val66met polymorphism alters food intake and hypothalamic BDNF expression in mice. J Cell Physiol. (2020) 235:9667–75. doi: 10.1002/jcp.29778
37. Hang PZ, Zhu H, Li PF, Liu J, Ge FQ, Zhao J, et al. The emerging role of BDNF/TrkB signaling in cardiovascular diseases. Life. (2021) 11. doi: 10.3390/life11010070
38. Sumaya W, Wallentin L, James SK, Siegbahn A, Gabrysch K, Bertilsson M, et al. Fibrin clot properties independently predict adverse clinical outcome following acute coronary syndrome: a plato substudy. Eur Heart J. (2018) 39:1078–85. doi: 10.1093/eurheartj/ehy013
39. Wu Y, Chen Z, Duan J, Huang K, Zhu B, Yang L, et al. Serum levels of FGF21, β-Klotho, and BDNF in stable coronary artery disease patients with depressive symptoms: a cross-sectional single-center study. Front Psychiatry. (2020) 11:587492. doi: 10.3389/fpsyt.2020.587492
40. Beurel E, Toups M, Nemeroff CB. The bidirectional relationship of depression and inflammation: double trouble. Neuron. (2020) 107:234–56. doi: 10.1016/j.neuron.2020.06.002
41. Pan JX, Xia JJ, Deng FL, Liang WW, Wu J, Yin BM, et al. Diagnosis of major depressive disorder based on changes in multiple plasma neurotransmitters: a targeted metabolomics study. Transl Psychiatry. (2018) 8:130. doi: 10.1038/s41398-018-0183-x
42. Yoshimura R, Kishi T, Atake K, Katsuki A, Iwata N. Serum brain-derived neurotrophic factor, and plasma catecholamine metabolites in people with major depression: preliminary cross-sectional study. Front Psychiatry. (2018) 9:52. doi: 10.3389/fpsyt.2018.00052
43. Lee BH, Kim YK. Reduced platelet BDNF level in patients with major depression. Prog Neuropsychopharmacol Biol Psychiatry. (2009) 33:849–53. doi: 10.1016/j.pnpbp.2009.04.002
44. Fujimura H, Altar CA, Chen R, Nakamura T, Nakahashi T, Kambayashi J, et al. Brain-Derived Neurotrophic Factor is stored in human platelets and released by agonist stimulation. Thromb Haemost. (2002) 87:728–34. doi: 10.1055/s-0037-1613072
45. Naegelin Y, Dingsdale H, Sauberli K, Schadelin S, Kappos L, Barde YA. Measuring and validating the levels of Brain-Derived Neurotrophic Factor in human serum. eNeuro. (2018) 5:ENEURO.0419-17.2018. doi: 10.1523/ENEURO.0419-17.2018
46. Gialluisi A, Santonastaso F, Bonaccio M, Bracone F, Shivappa N, Hebert JR, et al. Circulating inflammation markers partly explain the link between the dietary inflammatory index and depressive symptoms. J Inflamm Res. (2021) 14:4955–68. doi: 10.2147/JIR.S312925
47. Vahid F, Bourbour F, Gholamalizadeh M, Shivappa N, Hebert JR, Babakhani K, et al. A pro-inflammatory diet increases the likelihood of obesity and overweight in adolescent boys: a case-control study. Diabetol Metab Syndr. (2020) 12:29. doi: 10.1186/s13098-020-00536-0
48. Gialluisi A, Izzi B, Di Castelnuovo A, Cerletti C, Donati MB, de Gaetano G, et al. Revisiting the link between platelets and depression through genetic epidemiology: new insights from platelet distribution width. Haematologica. (2020) 105:e246–8. doi: 10.3324/haematol.2019.222513
49. Azoulay D, Horowitz NA. Brain-derived neurotrophic factor in hematological malignancies: from detrimental to potentially beneficial. Blood Rev. (2022) 51:100871. doi: 10.1016/j.blre.2021.100871
50. Wang N, Tian B. Brain-derived neurotrophic factor in autoimmune inflammatory diseases (review). Exp Ther Med. (2021) 22:1292. doi: 10.3892/etm.2021.10727
51. Amadio P, Baldassarre D, Sandrini L, Weksler BB, Tremoli E, Barbieri SS. Effect of cigarette smoke on monocyte procoagulant activity: focus on Platelet-Derived Brain-Derived Neurotrophic Factor (BDNF). Platelets. (2017) 28:60–5. doi: 10.1080/09537104.2016.1203403
52. Azoulay D, Vachapova V, Shihman B, Miler A, Karni A. Lower Brain-derived neurotrophic factor in serum of relapsing remitting MS: reversal by glatiramer acetate. J Neuroimmunol. (2005) 167:215–8. doi: 10.1016/j.jneuroim.2005.07.001
53. Inserra A, Mastronardi CA, Rogers G, Licinio J, Wong ML. Neuroimmunomodulation in major depressive disorder: focus on caspase 1, inducible nitric oxide synthase, and interferon-gamma. Mol Neurobiol. (2019) 56:4288–305. doi: 10.1007/s12035-018-1359-3
54. Saltiel AR, Olefsky JM. Inflammatory mechanisms linking obesity and metabolic disease. J Clin Invest. (2017) 127:1–4. doi: 10.1172/JCI92035
55. Azoulay D, Urshansky N, Karni A. Low and dysregulated BDNF secretion from immune cells of MS patients is related to reduced neuroprotection. J Neuroimmunol. (2008) 195:186–93. doi: 10.1016/j.jneuroim.2008.01.010
56. Rocha VZ, Folco EJ, Sukhova G, Shimizu K, Gotsman I, Vernon AH, et al. Interferon-gamma, a Th1 cytokine, regulates fat inflammation: a role for adaptive immunity in obesity. Circ Res. (2008) 103:467–76. doi: 10.1161/CIRCRESAHA.108.177105
57. Zierold S, Buschmann K, Gachkar S, Bochenek ML, Velmeden D, Hobohm L, et al. Brain-derived neurotrophic factor expression and signaling in different perivascular adipose tissue depots of patients with coronary artery disease. J Am Heart Assoc. (2021) 10:e018322. doi: 10.1161/JAHA.120.018322
58. Schulte-Herbruggen O, Nassenstein C, Lommatzsch M, Quarcoo D, Renz H, Braun A. Tumor necrosis factor-alpha and interleukin-6 regulate secretion of brain-derived neurotrophic factor in human monocytes. J Neuroimmunol. (2005) 160:204–9. doi: 10.1016/j.jneuroim.2004.10.026
59. Kishi T, Yoshimura R, Ikuta T, Iwata N. Brain-derived neurotrophic factor and major depressive disorder: evidence from meta-analyses. Front Psychiatry. (2017) 8:308. doi: 10.3389/fpsyt.2017.00308
60. Verhagen M, van der Meij A, van Deurzen PA, Janzing JG, Arias-Vasquez A, Buitelaar JK, et al. Meta-analysis of the BDNF Val66met polymorphism in major depressive disorder: effects of gender and ethnicity. Mol Psychiatry. (2010) 15:260–71. doi: 10.1038/mp.2008.109
61. Goldfield GS, Walsh J, Sigal RJ, Kenny GP, Hadjiyannakis S, De Lisio M, et al. Associations of the BDNF Val66met polymorphism with body composition, cardiometabolic risk factors, and energy intake in youth with obesity: findings from the hearty study. Front Neurosci. (2021) 15:715330. doi: 10.3389/fnins.2021.715330
62. Ricci C, Marzocchi C, Riolo G, Ciuoli C, Benenati N, Bufano A, et al. The impact of CPT1B rs470117, LEPR rs1137101 and BDNF rs6265 polymorphisms on the risk of developing obesity in an Italian population. Obes Res Clin Pract. (2021) 15:327–33. doi: 10.1016/j.orcp.2021.06.008
63. Youssef MM, Underwood MD, Huang YY, Hsiung SC, Liu Y, Simpson NR, et al. Association of BDNF Val66met polymorphism and brain BDNF levels with major depression and suicide. Int J Neuropsychopharmacol. (2018) 21:528–38. doi: 10.1093/ijnp/pyy008
64. Gunstad J, Schofield P, Paul RH, Spitznagel MB, Cohen RA, Williams LM, et al. BDNF Val66met polymorphism is associated with body mass index in healthy adults. Neuropsychobiology. (2006) 53:153–6. doi: 10.1159/000093341
65. Leon-Mimila P, Villamil-Ramirez H, Villalobos-Comparan M, Villarreal-Molina T, Romero-Hidalgo S, Lopez-Contreras B, et al. Contribution of common genetic variants to obesity and obesity-related traits in mexican children and adults. PLoS ONE. (2013) 8:e70640. doi: 10.1371/journal.pone.0070640
66. He T, Sun R, Li Y, Katusic ZS. Effects of Brain-derived neurotrophic factor on microrna expression profile in human endothelial progenitor cells. Cell Transplant. (2018) 27:1005–9. doi: 10.1177/0963689718761658
67. Descamps B, Saif J, Benest AV, Biglino G, Bates DO, Chamorro-Jorganes A, et al. BDNF (Brain-Derived Neurotrophic Factor) promotes embryonic stem cells differentiation to endothelial cells via a molecular pathway, including MicroRNA-214, EZH2 (Enhancer of Zeste Homolog 2), and eNOS (Endothelial Nitric Oxide Synthase). Arterioscler Thromb Vasc Biol. (2018) 38:2117–25. doi: 10.1161/ATVBAHA.118.311400
68. Ceconi C, Fox KM, Remme WJ, Simoons ML, Deckers JW, Bertrand M, et al. Ace inhibition with perindopril and biomarkers of atherosclerosis and thrombosis: results from the pertinent study. Atherosclerosis. (2009) 204:273–5. doi: 10.1016/j.atherosclerosis.2008.08.042
69. de Melo LA, Almeida-Santos AF. Neuropsychiatric properties of the Ace2/Ang-(1-7)/Mas pathway: a brief review. Protein Pept Lett. (2020) 27:476–83. doi: 10.2174/0929866527666191223143230
70. Dong B, Zhang C, Feng JB, Zhao YX, Li SY, Yang YP, et al. Overexpression of ACE2 enhances plaque stability in a rabbit model of atherosclerosis. Arterioscler Thromb Vasc Biol. (2008) 28:1270–6. doi: 10.1161/ATVBAHA.108.164715
71. Okechukwu CE. The downregulation of angiotensin-converting enzyme 2 expression may be associated with depression and anxiety among patients with severe acute respiratory syndrome Coronavirus 2. Curr Med Issues. (2021) 19:218–9. doi: 10.4103/cmi.cmi_47_21
72. Minuzzi LG, Seelaender M, Silva BSA, Cunha E, Deus MC, Vasconcellos FTF, et al. COVID-19 outcome relates with circulating BDNF, according to patient adiposity and age. Front Nutr. (2021) 8:784429. doi: 10.3389/fnut.2021.784429
73. Petrella C, Nenna R, Petrarca L, Tarani F, Paparella R, Mancino E, et al. Serum NGF and BDNF in Long-Covid-19 adolescents: a pilot study. Diagnostics. (2022) 12:1162. doi: 10.3390/diagnostics12051162
74. Lorkiewicz P, Waszkiewicz N. Biomarkers of Post-COVID depression. J Clin Med. (2021) 10:4142. doi: 10.3390/jcm10184142
75. De Sousa RAL, Improta-Caria AC, Aras-Junior R, de Oliveira EM, Soci UPR, Cassilhas RC. Physical exercise effects on the brain during COVID-19 pandemic: links between mental and cardiovascular health. Neurol Sci. (2021) 42:1325–34. doi: 10.1007/s10072-021-05082-9
76. Tsai SJ. Role of tissue-type plasminogen activator and plasminogen activator inhibitor-1 in psychological stress and depression. Oncotarget. (2017) 8:113258–68. doi: 10.18632/oncotarget.19935
77. Santovito D, Mezzetti A, Cipollone F. Cyclooxygenase and prostaglandin synthases: roles in plaque stability and instability in humans. Curr Opin Lipidol. (2009) 20:402–8. doi: 10.1097/MOL.0b013e32832fa22c
78. Guo JY, Li CY, Ruan YP, Sun M, Qi XL, Zhao BS, et al. Chronic treatment with celecoxib reverses chronic unpredictable stress-induced depressive-like behavior via reducing cyclooxygenase-2 expression in rat brain. Eur J Pharmacol. (2009) 612:54–60. doi: 10.1016/j.ejphar.2009.03.076
79. Chen Q, Luo Y, Kuang S, Yang Y, Tian X, Ma J, et al. Cyclooxygenase-2 signalling pathway in the cortex is involved in the pathophysiological mechanisms in the rat model of depression. Sci Rep. (2017) 7:488. doi: 10.1038/s41598-017-00609-7
80. Ferroni P, Riondino S, Vazzana N, Santoro N, Guadagni F, Davi G. Biomarkers of platelet activation in acute coronary syndromes. Thromb Haemost. (2012) 108:1109–23. doi: 10.1160/TH12-08-0550
81. Santilli F, Davi G, Patrono C. Homocysteine, methylenetetrahydrofolate reductase, folate status and atherothrombosis: a mechanistic and clinical perspective. Vascul Pharmacol. (2016) 78:1–9. doi: 10.1016/j.vph.2015.06.009
82. Amadio P, Zara M, Sandrini L, Ieraci A, Barbieri SS. Depression and cardiovascular disease: the viewpoint of platelets. Int J Mol Sci. (2020) 21:7560. doi: 10.3390/ijms21207560
83. Mesirow MSC, Roberts S, Cecil CAM, Maughan B, Jacka FN, Relton C, et al. Serum cholesterol, MTHFR methylation, and symptoms of depression in children. Dev Psychol. (2019) 55:2575–86. doi: 10.1037/dev0000831
84. Rubin RR, Peyrot M, Gaussoin SA, Espeland MA, Williamson D, Faulconbridge LF, et al. Four-year analysis of cardiovascular disease risk factors, depression symptoms, and antidepressant medicine use in the look ahead (action for health in diabetes) clinical trial of weight loss in diabetes. Diabetes Care. (2013) 36:1088–94. doi: 10.2337/dc12-1871
Keywords: BDNF, obesity, depression, atherothrombosis, EV-miRNA, cardiovascular disease
Citation: Amadio P, Macchi C, Favero C, Zarà M, Solazzo G, Dioni L, Sandrini L, Vigna L, Greco MF, Buoli M, Sirtori CR, Pesatori AC, Ieraci A, Ruscica M, Barbieri SS and Bollati V (2022) Brain-Derived Neurotrophic Factor and Extracellular Vesicle-Derived miRNAs in an Italian Cohort of Individuals With Obesity: A Key to Explain the Link Between Depression and Atherothrombosis. Front. Cardiovasc. Med. 9:906483. doi: 10.3389/fcvm.2022.906483
Received: 28 March 2022; Accepted: 16 June 2022;
Published: 13 July 2022.
Edited by:
Jinwei Tian, The Second Affiliated Hospital of Harbin Medical University, ChinaReviewed by:
Undurti Narasimha Das, UND Life Sciences LLC, United StatesJahaira Lopez-Pastrana, Jefferson University Hospitals, United States
David Azoulay, Galilee Medical Center, Israel
Copyright © 2022 Amadio, Macchi, Favero, Zarà, Solazzo, Dioni, Sandrini, Vigna, Greco, Buoli, Sirtori, Pesatori, Ieraci, Ruscica, Barbieri and Bollati. This is an open-access article distributed under the terms of the Creative Commons Attribution License (CC BY). The use, distribution or reproduction in other forums is permitted, provided the original author(s) and the copyright owner(s) are credited and that the original publication in this journal is cited, in accordance with accepted academic practice. No use, distribution or reproduction is permitted which does not comply with these terms.
*Correspondence: Massimiliano Ruscica, bWFzc2ltaWxpYW5vLnJ1c2NpY2FAdW5pbWkuaXQ=; Silvia Stella Barbieri, c2lsdmlhLmJhcmJpZXJpQGNjZm0uaXQ=
†These authors have contributed equally to this work and share last authorship