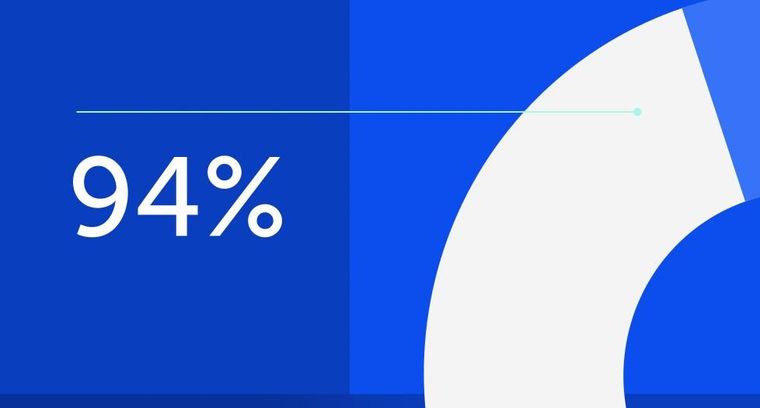
94% of researchers rate our articles as excellent or good
Learn more about the work of our research integrity team to safeguard the quality of each article we publish.
Find out more
ORIGINAL RESEARCH article
Front. Cardiovasc. Med., 17 June 2022
Sec. Cardiovascular Biologics and Regenerative Medicine
Volume 9 - 2022 | https://doi.org/10.3389/fcvm.2022.877450
This article is part of the Research TopicModeling of Cardiovascular Cell BarriersView all 4 articles
Background: Constant supply of oxygen is crucial for multicellular tissue homeostasis and energy metabolism in cardiac tissue. As a first response to acute hypoxia, endothelial cells (ECs) promote recruitment and adherence of immune cells to the dysbalanced EC barrier by releasing inflammatory mediators and growth factors, whereas chronic hypoxia leads to the activation of a transcription factor (TF) battery, that potently induces expression of growth factors and cytokines including platelet-derived growth factor (PDGF) and vascular endothelial growth factor (VEGF). We report a hypoxia-minded, targeted bioinformatics approach aiming to identify and validate TFs that regulate angiogenic signaling.
Results: A comprehensive RNA-Seq dataset derived from human ECs subjected to normoxic or hypoxic conditions was selected to identify significantly regulated genes based on (i) fold change (normoxia vs. hypoxia) and (ii) relative abundancy. Transcriptional regulation of this gene set was confirmed via qPCR in validation experiments where HUVECs were subjected to hypoxic conditions for 24 h. Screening the promoter and upstream regulatory elements of these genes identified two TFs, KLF5 and SP1, both with a potential binding site within these regions of selected target genes. In vitro, siRNA experiments confirmed SP1- and KLF5-mediated regulation of identified hypoxia-sensitive endothelial genes. Next to angiogenic signaling, we also validated the impact of TFs on inflammatory signaling, both key events in hypoxic sensing. Both TFs impacted on inflammatory signaling since endogenous repression led to increased NF-κB signaling. Additionally, SP1 silencing eventuated decreased angiogenic properties in terms of proliferation and tube formation.
Conclusion: By detailed in silico analysis of promoter region and upstream regulatory elements for a list of hypoxia-sensitive genes, our bioinformatics approach identified putative binding sites for TFs of SP or KLF family in vitro. This strategy helped to identify TFs functionally involved in human angiogenic signaling and therefore serves as a base for identifying novel RNA-based drug entities in a therapeutic setting of vascularization.
Constant supply of oxygen is crucial for cellular and tissue homeostasis regulating energy metabolism (1). In contrast, hypoxia is defined as the state that describes an impaired oxygen partial pressure loss in blood and tissue, eventually leading to a shortage of oxygen and thus detrimental for organ function. Besides physiological oxygen levels, also the oxygen concentration at which endogenous hypoxia gene responses are activated vary between different tissues and developmental stages (1). Of note, hypoxia itself can occur naturally, for example at high altitudes (2) or in a variety of disease models such as stroke (3), cardiovascular disease (4), and cancer (5). Cellular or tissue hypoxia is known to regulate gene expression on multiple levels: at DNA level transcription factors (TFs) are present, which are activated under hypoxic conditions (e.g., HIF-1α), and additionally the activity of histone-modifying enzymes that modulate chromosomal organization is oxygen-dependent (6, 7). This complex molecular interplay is resulting in dynamic chromatin structures leading to active or repressed genomic sites as shown in earlier studies investigating angiogenic hypoxic gene signature (8). Hypoxic signaling is causally linked to inflammation as hypoxia-inducible factor 1 (HIF-1α) stimulates the expression of NF-κB, which subsequently triggers the expression of inflammatory genes (9). In a feedback-loop manner, NF-κB signaling was also shown to transcriptionally activate HIF-1α and basal NF-κB activity was demonstrated to be required for HIF-1α protein accumulation under hypoxia (10). Furthermore, hypoxic stress stimulates the induction of autophagy in order to maintain cellular survival (11, 12). In cancer, the reciprocal crosstalk between NF-κB and autophagy can either repress or promote tumorigenesis. Here, stimulus and context determine the fate of tumorigenesis (13). Taken together, these information link hypoxia with autophagy, innate immunity and inflammation via multi-lateral interactions. ECs form the inner layer of blood vessels and thus act a vital part in a variety of biological processes such as angiogenesis (14), inflammation (15), and nutrient supply via the circulatory system (16). The response of ECs to changes in oxygen availability crucially depends on how the underlying condition manifests in a time-dependent manner. As a first response to acute hypoxia, ECs promote recruitment and adherence of immune cells to the dysbalanced endothelium by expressing and releasing inflammatory mediators and growth factors, whereas long-term hypoxia causes the activation of a TF battery (e.g., HIF-1α), that potently induces expression of growth factors and cytokines including platelet-derived growth factor (PDGF) and vascular endothelial growth factor (VEGF) (17). Abundant expression of those cytokines initiates proliferation and migration of ECs (14) which ultimately supports the formation of fresh blood vessels in poorly perfused tissue areas. Understanding the molecular pathways of angiogenic gene patterns can thus highlight novel therapeutic approaches in the aforementioned organ disorders.
Herein, we apply a hypoxia-minded, targeted bioinformatics promoter screening approach followed by experimental validation of hypoxia-sensitive endothelial genes regulated by the TFs SP1 and KLF5 including functional hypoxia-regulated events. Our approach is not limited to a specific biological setting, thus serves as a general base for identifying novel drug entities either supporting or inhibiting these networks in a therapeutic setting.
Initially, a previously reported RNA-Seq dataset (GSE70335) derived from human ECs subjected to normoxic or hypoxic conditions (8) was selected to identify significantly regulated genes based on (i) fold change (normoxia vs. hypoxia) and (ii) relative abundancy. Transcriptional regulation of this gene set was confirmed in vitro via qPCR in validation experiments. In line with the experimental setup of the reported RNA-Seq dataset, HUVECs were incubated for 24 h at low oxygen conditions to validate differential gene expression in qPCR experiments (Figure 1A). Combining upstream regulatory elements of this gene set with TF binding matrices in our efficient analysis pipeline allowed high throughput screening of our gene set for multiple TF binding sites (Figure 1B). Screening the promoter and upstream regulatory elements of genes, whose dysregulation could be validated in qPCR experiments, identified the TFs KLF5 and SP1 harboring a possible binding site within these regions in 8 out of the 10 differentially expressed genes. As the top two TFs in our results they were selected for further validation experiments (Figure 1C). All results from the bioinformatics analysis can be found in Supplementary Material (Supplementary Table 1). Given this prediction, we examined if hypoxia regulates endogenous SP1 and KLF5 expression in ECs by monitoring their mRNA levels after various periods of hypoxia. Both, SP1 as well as KLF5 mRNA levels remained constant after hypoxia treatment for 24 h (Figure 1D). In line, time lapse hypoxia experiments in HUVECs revealed that mRNA levels of both, SP1 and KLF5 remain constant during the first 24 h, whereas after 48 h, mRNA levels are significantly elevated compared to normoxic cells (Supplementary Figure 1). Thus, we hypothesize that mainly SP1 and KLF5 localization but not their expression levels act as a potential driver of hypoxic gene expression in human ECs in vitro.
Figure 1. Transcription factors SP1 and KLF5 are potential regulators of EC transcriptional response to hypoxia. (A) mRNA levels of selected genes from a previously reported RNA-Seq dataset were validated in HUVECs after 24 h of hypoxia via qPCR (n = 3). (B) Bioinformatic pipeline for the selection of transcription factors. (C) In silico prediction of potential binding sites for SP1 and KLF5 in hypoxia-sensitive geneset. (D) qPCR data of SP1 and KLF5 mRNA levels in HUVECs after 24 h of hypoxia (n = 3). Each dot resembles the mean value of all technical replicates from an independent experiment. *p ≤ 0.05, **p ≤ 0.01, ***p ≤ 0.001.
Next, we aimed to investigate the effect of TF modulation on hypoxia-induced endothelial gene transcription. Silencing of endogenous SP1 with a specific siRNA resulted in a robust knockdown of SP1 on protein but not on mRNA level (Figures 2A,B). To examine whether SP1 could influence expression of selected hypoxia-sensitive genes in ECs, we quantified relative mRNA levels after siRNA application during hypoxic conditions in vitro. We observed that six out of eight genes with a suspected SP1 binding site within their upstream regulatory elements show altered mRNA levels after SP1 knockdown under hypoxia (Figures 2C,D).
Figure 2. SP1 differentially regulates hypoxic gene expression in ECs in vitro. (A) SP1 mRNA levels in HUVECs after 24 h of hypoxia were detected via qPCR (n = 4). (B) SP1 protein levels in HUVECs after 24 h after siRNA transfection were detected by Western blot (n = 3). For each sample, 20 μg of protein were loaded onto polyacrylamide gels. Chemiluminescence with an exposure time of 390 s (SP1) or 120 s (GAPDH). (C) mRNA levels of genes, which were upregulated under hypoxia, were detected using qPCR after siRNA-mediated SP1-knockdown in combination with hypoxia (n = 4). (D) mRNA levels of genes, which were downregulated under hypoxia, were detected using qPCR after siRNA-mediated SP1-knockdown in combination with hypoxia (n = 4). Each dot resembles the mean value of all technical replicates from an independent experiment. *p ≤ 0.05, **p ≤ 0.01, ***p ≤ 0.001.
For four (ANPTL4, DNAH11, INHBA, and BGN) of these five upregulated genes with a predicted binding site the hypoxia-driven upregulation was augmented after SP1-knockdown, even though the knockdown did not affect expression of these mRNAs under normoxic conditions, indicating a selective mechanism of SP1 between normoxia and hypoxia (Figure 2C). In contrast, out of the three downregulated genes with a predicted binding site, two (LRP8 and MCM4) of these were shown to be unaffected on mRNA levels after SP1 repression in hypoxic environment. Transcriptomic repression as a result of SP1-knockdown at normoxic conditions was abolished in hypoxic HUVECs (Figure 2D). In conclusion, SP1 shows a bilateral function under hypoxic signaling where it can either repress the upregulation of hypoxia-sensitive genes under hypoxic conditions or induce the gene expression under normoxic conditions. The latter only seems to occur if respective genes are downregulated as a result of hypoxic signaling. Combining these observations with our finding that SP1 mRNA levels remain unchanged after 24 h of hypoxia in ECs (Figure 1D), regulation of hypoxic gene expression via SP1 appears to be mediated via modulation of its activity but not its quantity. Collectively, we hypothesize that SP1 activity is altered in human ECs under hypoxemia as transcriptomic regulation patterns after SP1-knockdown differ between hypoxia and normoxia. We observed SP1-mediated regulation in both conditions, thus we claim that not only SP1 but on top chromatin dynamics and hence, accessibility of binding sites possess a major role in the regulation of SP1-driven transcription in ECs under hypoxia.
In parallel, our proof of concept experiments revealed that KLF5 mRNA levels were successfully downregulated by siRNA after 24 h (Figure 3A). In line, Western blot experiments confirmed the knockdown of KLF5 also on protein level (Figure 3B). Assessing mRNA levels of preselected genes after a combinatory treatment of hypoxia and siRNA transfection, we observed that KLF5-knockdown was only able to augment DNAH11 and INHBA mRNA levels under hypoxia, whereas mRNA levels of the other predicted genes remain unchanged (Figures 3C,D). But similar to SP1, a KLF5-knockdown only affected DNAH11 and INHBA mRNA levels under hypoxic conditions and not under regular culture conditions (Figures 2C, 3C), further strengthening our hypothesis of a selective regulatory mechanism induced by either a normoxic or hypoxic environment.
Figure 3. KLF5 does not regulate hypoxic gene expression in ECs. (A) KLF5 mRNA levels in HUVECs after 24 h of hypoxia were detected via qPCR (n = 4). (B) KLF5 protein levels in HUVECs after 24 h after siRNA transfection were detected by Western blot (n = 4). For each sample, 30 μg of protein were loaded onto polyacrylamide gels. Chemiluminescence with an exposure time of 398 s (KLF5) or 13 s (GAPDH). (C) mRNA levels of genes, which were upregulated under hypoxia, were detected using qPCR after siRNA-mediated KLF5-knockdown in combination with hypoxia (n = 4). (D) mRNA levels of genes, which were downregulated under hypoxia, were detected using qPCR after siRNA-mediated KLF5-knockdown in combination with hypoxia (n = 4). Each dot resembles the mean value of all technical replicates from an independent experiment. *p ≤ 0.05, **p ≤ 0.01, ***p ≤ 0.001.
Besides the control of hypoxic gene expression, we were wondering if KLF5 and SP1 actions also affect EC function. As stated earlier, low oxygen supply and inflammation are interconnected by HIF-1α and NF-κB (18, 19). Using a Luciferase-based reporter assay, we investigated if TFs could not only influence hypoxic gene transcription but also inflammatory response. Applying this construct, we were able to monitor NF-κB promoter activity dependent on pro-inflammatory conditions. By stimulating HEK293FT cells with a pro-inflammatory stimulus in terms of Poly I:C we observed an enhanced NF-κB signaling. The increased inflammatory response was attenuated after knockdown of both TFs, SP1 (Figure 4A) and KLF5 (Supplementary Figure 2A). A hallmark of ECs is the initiation of angiogenesis. Therefore, we tested whether siRNA-mediated knockdown of SP1 and KLF5 could interfere with angiogenic attributes of HUVECs such as proliferation, migration and tube formation under hypoxic conditions. By measuring the integration of BrdU into the DNA of dividing HUVECs, SP1-knockdown cells – similar to hypoxic ECs – exhibit decreased proliferation under normoxia, but not under hypoxia (Figure 4B), whereas KLF5-knockdown did not affect EC proliferation at all (Supplementary Figure 2B). This pattern appears to be similar to how SP1 seems to control hypoxic gene expression (Figure 2C). As opposed to this, EC migration was not affected by neither SP1- (Figure 4C) nor KLF5-knockdown in vitro (Supplementary Figure 2C). Eventually, both, EC proliferation and migration result in the formation of new capillary structures. By culturing HUVECs on a three-dimensional matrix, hypoxic ECs exhibit less tube formation compared to normoxic ECs (Figures 4D,E). A knockdown of SP1 mRNA levels resulted in reduced tube formation after normoxia as well as after hypoxia (Figures 4D,E). Similar to EC proliferation and migration a KLF5-knockdown had no effect on EC tube formation, neither under normoxia nor hypoxia (Supplementary Figures 2D,E). In conclusion, SP1 might act as a mediator of inflammatory response via stimulating NF-κB signaling as well as angiogenic signaling in ECs by initiating EC proliferation and tube formation.
Figure 4. SP1 inhibits endothelial function. (A) Luciferase reporter assay was used to determine NF-κB signaling in HEK293FT cells after siRNA-mediated knockdown of SP1 (n = 3). (B) Proliferation of HUVECs after SP1-knockdown and hypoxia was determined by BrdU-incorporation (n = 4). (C) Migration of HUVECs after SP1-knockdown and hypoxia was determined by scratch wound healing assay (n = 3). (D) Tube formation of HUVECs after SP1-knockdown and hypoxia was determined by tube formation assay (n = 3). (E) Representative images of tube formation assay in HUVECs after SP1-knockdown and hypoxia. Scale bar, 1,000 μm. Each dot resembles the mean value of all technical replicates from an independent experiment. *p ≤ 0.05, **p ≤ 0.01, ***p ≤ 0.001.
In addition we analyzed a RNA-Seq data set (GSE116250) of human left ventricular tissue from donors with ischemic cardiomyopathy. Five out of the ten genes of interest were also significantly deregulated between ischemic heart tissue and non-failing hearts (Figure 5).
Figure 5. Volcano plot showing results of differential gene expression analysis of human heart tissue from ischemic heart failure in comparison with healthy controls. Red dots show significantly deregulated genes with logFC > | 0.585| and adjusted p-value < 0.05. Genes of interest are labeled.
The epigenetic landscape of hypoxia is complex (20, 21). Multiple TFs such as HIF-1α and EPAS1 have a dynamic functional pattern in orchestrating gene expression in hypoxia. However, the interaction of these pioneering TFs with the existing epigenetic architecture may be key for the fine-tuning of biological outcomes. Our bioinformatics screening identified SP1 and KLF5 binding sites as common features of deregulated genes in a dataset from hypoxic HUVECs (8), indicating a broad supporting involvement of these TFs as intermediaries in oxygen-deficient conditions. Indeed, the knockdown of SP1 and KLF5 led to a strong de-repression of multiple predicted hypoxic factors, implying their roles as likely intermediaries in the process.
This study reports the key contribution of endothelial TFs SP1 and KLF5 during human EC hypoxic gene response. To our knowledge, this is the first study linking in silico and outcome analysis for identified TFs underlining the importance of herein presented results. Despite a potential false positive rate of putative promoter binding predictions, we were able to identify SP1 and KLF5 as highly relevant biological factors and model their role within the epigenetic regulation of hypoxia. SP1 and KLF5 were indeed independently found in other studies as major players in low-oxygen conditions (22–26). Therefore our in silico analysis approach depicts a very efficient way to narrow down a broad range of results coming from high throughput experiments such as RNA-sequencing to gain precise insights into the regulatory biology behind a certain setting. This allows systematic planning of validation experiments underlining a hypothesis without cost- and time-intensive experiments such as ChiP-Seq. Comparing our approach with other available methods (27, 28) it is noteworthy, that our method is meant to be an upstream step for experimental validation not a stand-alone method. Also we focus more on identifying major regulatory key molecules than discovery of novel binding motifs. The library used in our approach (TFBStools) also contains a wrapper function for a MEME analysis, which, in line with other tools, focuses more on “de novo” discovery of motifs. We preferred to establish a method to efficiently deliver an overview over regulatory effects in a NGS data set of interest. In the method proposed by Srivastava et al. (29), for example, the user needs to create and compile sequence files of the regions of interest as well as manually select the TFs. Our approach combines all steps in an easy to use R script where users only need to enter the organism of interest and a list of genes with identifiers as comma separated text file.
Since physiological processes such as angiogenesis and inflammation take part in multiple disease models, there is an interest in developing drugs that modulate SP1 signaling in ECs under hypoxia. Thus, investigating the modulation of KLF5 and SP1 signaling could give new insights into the treatment of various disease models. Targeting highly interconnected intermediaries such as SP1 and KLF5 may have clinical relevance. In the context of recovery from myocardial ischemia, hypoxia was reported to have both beneficial and detrimental effects (22). In line, clinical translation of our findings to a dataset of human ischemic heart failure samples (GSE116250) revealed significant deregulation of five endothelial-cell related genes (Figure 5). Speculatively, the positive aspects could be directly extracted on a molecular level by maintaining SP1 or KLF5 levels, as SP1 supported the angiogenic TNF-α thymidine phosphorylase pathway (23) and VEGF (24). On the other hand, SP1 (25) and KLF5 inhibition slowed angiogenesis (26), which may be a useful strategy in oncotherapy. Moreover, we previously showed that modular SP1 and EN1 transcriptional binding mediates pro-fibrotic effects of TGFβ signaling, in which EN1 knockout reverses experimental skin fibrosis (30). Our study showed impaired proliferation, migration, and consequently tube formation in hypoxic ECs, which furthermore was intensified after TF knockdown. This is contrast to in vivo observations where hypoxia induces aforementioned features. These findings originate in the different environmental conditions between in vitro and in vivo studies. ECs in vivo are predominantly quiescent before they become activated by hypoxic sensing and subsequently initiate angiogenesis via proliferation and migration. In vitro, ECs are constantly activated which is underlined by their constant proliferation.
In summary, we have developed an intuitive in silico approach to screen for TF regulation, allowing the modeling of significant biological processes including, but not limited to, hypoxia. A potential mediator by which SP1 affects EC function could be Activin A – an INHBA homodimer – and ANGPTL4, as these could already be shown to inhibit tube formation and proliferation of normoxic as well as hypoxic ECs (31–34). This could explain the attenuated tube formation ability and proliferation of ECs after SP1-knockdown.
The whole TF-analysis is presented as an easy-to-use script for the R programming language. Except for initially setting organism of choice; no advanced programming skill are necessary and the script runs automatically. All user inputs are described as comments within the R-script. After termination, CSV-files containing the results from promoter screening as well as the actual gene-TF prediction are saved to the current working directory. The session info is also saved as a txt-file.
The computation was performed on a Windows 10 machine with Intel i5 CPU and 32 GB memory, but is expected to also work on smaller systems with a longer but reasonable timespan for computation. Analysis follows our previously applied promoter profiling method (30). Upstream (−800 bp from TSS) sequences for genes of interest were retrieved using the bioMart package (v 2.48.3) in R (v. 4.1.1). Position weight matrices for human TFs were retrieved using JASPAR2018 package (v 1.1.1). Sequence scanning was performed using searchSequence function provided by TFBStools package (v. 1.30.0). All resulting hits were ranked by calculated binding score in a decreasing order and the top one percent was kept. Using the built-in function TFMPvalue, p-values for the related binding scores are calculated. Since we select only scores within the 1%-quantile, p-values are always significant, but can be stored for further inspection. Multiple hits for a TF-gene combination were eliminated. Unique hits were counted and divided by the number of overall genes of interest to obtain the enrichment ratio.
Raw reads were obtained from NCBI Sequence Read Archive (SRA) using fastq-dump function implemented in sratoolkit.1 Alignment to reference genome (GRCh38) and read mapping was performed using Rsubread (35). Differential gene expression was performed using our previously published tool tRomics (36). Genes with logFC > | 0.585| and adjusted p-value < 0.05 were considered differentially expressed.
HUVECs (Lonza, Switzerland) were cultured in EBM™-2 Endothelial Cell Growth Basal Medium-2 (Lonza, Switzerland), supplemented with Hydrocortisone, hFGF-B, VEGF, R3-IGF-1, Ascorbic Acid, hEGF, GA-1000 (Lonza, Switzerland) and 10% FBS (Gibco, Ireland). The cells were incubated in a humidified incubator (Binder, Germany) at 37°C and 5% CO2. For hypoxia studies, HUVECs were incubated at 0.2% O2 for 24 h 1 day after seeding the cells. Transfection of siRNAs (Table 1) was performed 1 day after seeding the cells. The siRNAs were diluted in Opti-MEM™ I Reduced Serum Medium (Gibco, Ireland) to a final concentration of 10 μM. Separately, Lipofectamine 2000 (Invitrogen, United States) was diluted 1:125 in Opti-MEM™ I Reduced Serum Medium. Both mixes were combined after an incubation time of 5 min at room temperature and incubated for another 20 min at room temperature. HUVECs were incubated in the transfection mix for 4 h at 37°C and 5% CO2. Afterward, medium was changed to regular culture medium and HUVECs were cultured for 24 h either at normal (21% O2) or hypoxic conditions (0.2% O2). All experiments were performed in passage five to nine cells.
HEK293FT were cultured in DMEM, high Glucose (Gibco, Ireland), supplemented with 10% FBS (Gibco, Ireland) and 1% Penicillin/Streptomycin (Gibco, Ireland). For plasmid transfection, 100 ng of DNA was diluted in Opti-MEM™ I Reduced Serum Medium. In parallel, Lipofectamine 2000 was diluted 1:125 in Opti-MEM™ I Reduced Serum Medium and combined with the diluted DNA after 5 min incubation time at room temperature. The mix was incubated for further 20 min at room temperature and added to the cells afterward. After 4 h incubation time at 37°C and 5% CO2, medium was changed.
RNA was isolated via QIAzol Lysis Reagent (Qiagen, Germany) according to the manufacturers instructions. Subsequently, 600–1,000 ng of RNA were transcribed using an Oligo-dT cDNA synthesis protocol (Bio-Rad, United States). For each qPCR reaction 20 ng cDNA were used and mRNA levels were assessed by using the ABsolute™ Blue QPCR Mix, SYBR Green, Low ROX (Thermo Fisher, United States) in a QuantStudio™ 7 Flex Real-Time PCR system (Applied Biosystems, United States). Data were analyzed via standard curve method and mRNA levels of every gene were normalized to the relative levels of HPRT1 mRNA. All primers used in these experiments are listed in Table 2 [INHBA (QT00201586) and KLF5 (QT00074676) primers were purchased from Qiagen (Germany)].
Protein extraction and Western blotting was performed similar to our previous work (37). Cells were washed with PBS, centrifuged, resuspended in cell lysis buffer (Bio-Rad, United States) and sonicated by a Bioruptor® Pico sonication device (Diagenode, Belgium). From each sample either 20 or 30 μg of protein were loaded onto polyacrylamide gels for electrophoretic band separation and transferred to a nitrocellulose membrane (Bio-Rad, United States). Blocking of membranes was performed by incubation for 1 h in 5% blocking milk solution. The membranes were incubated together with the primary antibody overnight at 4°C on a rotator. After rinsing, the membranes were exposed to secondary IgG-HRP antibodies, ultimately being washed again and visualized with luminol-containing substrate solution or Clarity Western ECL Substrate (Bio-Rad, United States). Protein levels were normalized to GAPDH protein levels. Precision Plus Protein™ WesternC™ Blotting Standard (Bio-Rad) was utilized as a protein standard. Antibodies used for detection can be found in Table 3.
A Luciferase Reporter Assay in HEK293FT cells was used to determine the activity of inflammatory NF-κB signaling as previously described (38). In a 48-well format, 175,000 HEK293FT cells were co-transfected with the pSGNluc plasmid, a β-galactosidase control plasmid (Promega, United States) and respective siRNA as described earlier. The pSGNluc plasmid contains multiple NF-κB binding sites and therefore, enables evaluating NF-κB activity via detection of Luciferase activity. After transfection, medium was changed to serum-free medium containing 300 ng/ml Poly I:C incubated on HEK293FT cells for 24 h at 37°C and 5% CO2. Luminescence was detected using a Synergy HT Multi-Detection Microplate Reader (BioTek, United States) and in the end, Luciferase activity was normalized to β-galactosidase activity.
Wells of a 96-well plate were coated with 40 μl Matrigel Basement Membrane Matrix, LDEV-free (Corning, United States) and incubated for 30 min at 37°C prior to seeding the cells. At the end of the treatment, 10,000 HUVECs were transferred onto the Matrigel Matrix and incubated at 37°C and 5% CO2. Brightfield images were captured 24 h after seeding using a Cytation 1 Cell Imaging Multi-Mode Reader (BioTek, United States). Total tube length was determined with ImageJ by the Angiogenesis Analyzer tool (39).
A two-dimensional Scratch Wound Healing Assay was used to determine migration ability of HUVECs. In a 96-well plate, 30,000 HUVECs were seeded per well and treated on the next day as described. After the treatment, cells were stained with 5 μg/ml Hoechst 33342 (Thermo Fisher, United States) for 15 min at 37°C and 5% CO2. Next, a wound was scratched into the cell layer using a 20 μl pipette tip (Sarstedt, Germany). To remove dead cells, the plate was washed once with PBS and fresh medium was added. Subsequently, images were captured every 2 h for 16 h using a Cytation 1 Cell Imaging Multi-Mode Reader (BioTek, United States) with the first images being taken directly at the start. For the duration of the imaging process, the cells were incubated in a BioSpa 8 Automated Incubator (BioTek, United States) at 37°C, 21% O2 and 5% CO2. Migration was analyzed with ImageJ by measuring the covered area for every timepoint and calculating the migration index.
Proliferation of HUVECs was assessed by Cell Proliferation ELISA, BrdU (colorimetric) (Roche, Switzerland). A total of 5,000 HUVECs were seeded in a 96-well plate 1 day prior to the transfection and hypoxia treatment. After 24 h incubation at either normoxic or hypoxic conditions, medium was changed to medium containing BrdU (1:1,000) and incubated for further 24 h at 37°C, 21% O2 and 5% CO2. After the incubation time, the assay was performed according to the manufacturers manual and absorbance was measured using a Synergy HT Multi-Detection Microplate Reader (BioTek, United States).
All statistics were performed with GraphPad Prism (v 8.3.1) (GraphPad, United States). Statistical significance between two groups was determined either by Student’s t-test or Welch’s t-test. Statistical significance among three or more groups was determined by ANOVA. All values are displayed as means ± SEM. Statistical significance was considered for p ≤ 0.05.
All data generated in this study are available in manuscript and as supplement online. The RNASeq datasets are available at GEO with accession numbers: GSE30775 and GSE116250. The R script is available at https://github.com/max-fuchs/tf_screen.
AS, MF, JF, TT, and MK: conceptualization. AS, MF, JF, and MK: methodology, investigation, and visualization. AS, SS, KS, JW, AJ, AP, and JF: experiment. MF, CL, JD, TD, and MK: software. AS, MF, CL, KS, MJ, KX, JF, and MK: formal analysis. TT and MK: resources and funding acquisition. AS, MF, CL, KX, and MK: data curation. AS and MK: writing—original draft and preparation. AS, MF, SS, MJ, JD, TD, JF, and TT: writing—review and editing. JF, TT, and MK: supervision. JF and MK: project administration. All authors have read and agreed to the final version of the manuscript.
Open Access funding enabled by the Friedrich-Alexander-Universität Erlangen-Nürnberg within the funding programme “Open Access Publication Funding”. This research was funded by the German Federal Ministry of Education and Research (BMBF), CompLS program grant 031L0262C (to MK), Era-Net grant 01KT1801 (to MF, TD, and MK), the EU project Cardioregenix (TT), the Deutsche Forschungsgemeinschaft (KFO311-2, TH903/20-2 to TT; DI 1537/17-1, SFB CRC1181 project C01 (to JD), SFB TR221/project number 324392634 (B04 to JD, INF to TD and MK). This work was supported by the Fraunhofer Cluster of Excellence Immune-Mediated Diseases (CIMD) and REBIRTH Research Center for Translational Regenerative Medicine, Hanover.
TT has filed and licensed patents regarding non-coding RNAs in CVD. TT is founder and shareholder of Cardior Pharmaceuticals GmbH.
The remaining authors declare that the research was conducted in the absence of any commercial or financial relationships that could be construed as a potential conflict of interest.
All claims expressed in this article are solely those of the authors and do not necessarily represent those of their affiliated organizations, or those of the publisher, the editors and the reviewers. Any product that may be evaluated in this article, or claim that may be made by its manufacturer, is not guaranteed or endorsed by the publisher.
We would like to thank Ortwin Naujok (Hannover Medical School) for providing HEK293FT cells and Baubak Bajoghli (University Hospital Tübingen) for providing the pSGNluc NF-κB reporter plasmid.
The Supplementary Material for this article can be found online at: https://www.frontiersin.org/articles/10.3389/fcvm.2022.877450/full#supplementary-material
1. Batie M, Rocha S. Gene transcription and chromatin regulation in hypoxia. Biochem Soc Trans. (2020) 48:1121–8. doi: 10.1042/BST20191106
2. Xin J, Zhang H, He Y, Duren Z, Bai C, Chen L, et al. Chromatin accessibility landscape and regulatory network of high-altitude hypoxia adaptation. Nat Commun. (2020) 11:4928. doi: 10.1038/s41467-020-18638-8
3. Ferdinand P, Roffe C. Hypoxia after stroke: a review of experimental and clinical evidence. Exp Transl Stroke Med. (2016) 8:9. doi: 10.1186/s13231-016-0023-0
4. Abe H, Semba H, Takeda N. The roles of hypoxia signaling in the pathogenesis of cardiovascular diseases. J Atheroscler Thromb. (2017) 24:884–94. doi: 10.5551/jat.RV17009
5. Brahimi-Horn MC, Chiche J, Pouysségur J. Hypoxia and cancer. J Mol Med. (2007) 85:1301–7. doi: 10.1007/s00109-007-0281-3
6. Hsu K-F, Wilkins SE, Hopkinson RJ, Sekirnik R, Flashman E, Kawamura A, et al. Hypoxia and hypoxia mimetics differentially modulate histone post-translational modifications. Epigenetics. (2021) 16:14–27. doi: 10.1080/15592294.2020.1786305
7. Melvin A, Rocha S. Chromatin as an oxygen sensor and active player in the hypoxia response. Cell Signal. (2012) 24:35–43. doi: 10.1016/j.cellsig.2011.08.019
8. Fiedler J, Breckwoldt K, Remmele CW, Hartmann D, Dittrich M, Pfanne A, et al. Development of long noncoding RNA-based strategies to modulate tissue vascularization. J Am Coll Cardiol. (2015) 66:2005–15. doi: 10.1016/j.jacc.2015.07.081
9. Cummins EP, Taylor CT. Hypoxia and inflammation. Biochemist. (2017) 39:34–6. doi: 10.1042/bio03904034
10. Rius J, Guma M, Schachtrup C, Akassoglou K, Zinkernagel AS, Nizet V, et al. NF-kappaB links innate immunity to the hypoxic response through transcriptional regulation of HIF-1alpha. Nature. (2008) 453:807–11. doi: 10.1038/nature06905
11. Jiang F. Autophagy in vascular endothelial cells. Clin Exp Pharmacol Physiol. (2016) 43:1021–8. doi: 10.1111/1440-1681.12649
12. Tan Q, Wang M, Yu M, Zhang J, Bristow RG, Hill RP, et al. Role of autophagy as a survival mechanism for hypoxic cells in tumors. Neoplasia. (2016) 18:347–55. doi: 10.1016/j.neo.2016.04.003
13. Verzella D, Pescatore A, Capece D, Vecchiotti D, Ursini MV, Franzoso G, et al. Life, death, and autophagy in cancer: NF-κB turns up everywhere. Cell Death Dis. (2020) 11:210. doi: 10.1038/s41419-020-2399-y
14. Wong BW, Marsch E, Treps L, Baes M, Carmeliet P. Endothelial cell metabolism in health and disease: impact of hypoxia. EMBO J. (2017) 36:2187–203. doi: 10.15252/embj.201696150
15. Pober JS, Sessa WC. Evolving functions of endothelial cells in inflammation. Nat Rev Immunol. (2007) 7:803–15. doi: 10.1038/nri2171
16. Son N-H, Basu D, Samovski D, Pietka TA, Peche VS, Willecke F, et al. Endothelial cell CD36 optimizes tissue fatty acid uptake. J Clin Invest. (2018) 128:4329–42. doi: 10.1172/JCI99315
17. Michiels C, Arnould T, Remacle J. Endothelial cell responses to hypoxia: initiation of a cascade of cellular interactions. Biochim Biophys Acta. (2000) 1497:1–10. doi: 10.1016/S0167-4889(00)00041-0
18. van Uden P, Kenneth NS, Rocha S. Regulation of hypoxia-inducible factor-1alpha by NF-kappaB. Biochem J. (2008) 412:477–84. doi: 10.1042/BJ20080476
19. Okada K, Mori D, Makii Y, Nakamoto H, Murahashi Y, Yano F, et al. Hypoxia-inducible factor-1 alpha maintains mouse articular cartilage through suppression of NF-κB signaling. Sci Rep. (2020) 10:5425. doi: 10.1038/s41598-020-62463-4
20. Kietzmann T, Petry A, Shvetsova A, Gerhold JM, Görlach A. The epigenetic landscape related to reactive oxygen species formation in the cardiovascular system. Br J Pharmacol. (2017) 174:1533–54. doi: 10.1111/bph.13792
21. Kindrick JD, Mole DR. Hypoxic regulation of gene transcription and chromatin: cause and effect. Int J Mol Sci. (2020) 21:8320. doi: 10.3390/ijms21218320
22. Pang B, Zhao F, Zhou Y, He B, Huang W, Zhang F, et al. Systematic review and meta-analysis of the impact of hypoxia on infarcted myocardium: better or worse?. Cell Physiol Biochem. (2018) 51:949–60. doi: 10.1159/000495397
23. Zhu GH, Lenzi M, Schwartz EL. The Sp1 transcription factor contributes to the tumor necrosis factor-induced expression of the angiogenic factor thymidine phosphorylase in human colon carcinoma cells. Oncogene. (2002) 21:8477–85. doi: 10.1038/sj.onc.1206030
24. Ding A, Bian Y-Y, Zhang Z-H. SP1/TGF-β1/SMAD2 pathway is involved in angiogenesis during osteogenesis. Mol Med Rep. (2020) 21:1581–9. doi: 10.3892/mmr.2020.10965
25. Su F, Geng J, Li X, Qiao C, Luo L, Feng J, et al. SP1 promotes tumor angiogenesis and invasion by activating VEGF expression in an acquired trastuzumab-resistant ovarian cancer model. Oncol Rep. (2017) 38:2677–84. doi: 10.3892/or.2017.5998
26. Gao Y, Wu K, Chen Y, Zhou J, Du C, Shi Q, et al. Beyond proliferation: KLF5 promotes angiogenesis of bladder cancer through directly regulating VEGFA transcription. Oncotarget. (2015) 6:43791–805. doi: 10.18632/oncotarget.6101
27. Dang LT, Tondl M, Chiu MHH, Revote J, Paten B, Tano V, et al. TrawlerWeb: an online de novo motif discovery tool for next-generation sequencing datasets. BMC Genomics. (2018) 19:238. doi: 10.1186/s12864-018-4630-0
28. Heinz S, Benner C, Spann N, Bertolino E, Lin YC, Laslo P, et al. Simple combinations of lineage-determining transcription factors prime cis-regulatory elements required for macrophage and B cell identities. Mol Cell. (2010) 38:576–89. doi: 10.1016/j.molcel.2010.05.004
29. Srivastava R, Zhang Y, Xiong X, Zhang X, Pan X, Dong XC, et al. Prediction and validation of transcription factors modulating the expression of sestrin3 gene using an integrated computational and experimental approach. PLoS One. (2016) 11:e0160228. doi: 10.1371/journal.pone.0160228
30. Györfi A-H, Matei A-E, Fuchs M, Liang C, Rigau AR, Hong X, et al. Engrailed 1 coordinates cytoskeletal reorganization to induce myofibroblast differentiation. J Exp Med. (2021) 218:e20201916. doi: 10.1084/jem.20201916
31. Ito Y, Oike Y, Yasunaga K, Hamada K, Miyata K, Matsumoto S-I, et al. Inhibition of angiogenesis and vascular leakiness by angiopoietin-related protein 4. Cancer Res. (2003) 63:6651–7.
32. Okochi-Takada E, Hattori N, Tsukamoto T, Miyamoto K, Ando T, Ito S, et al. ANGPTL4 is a secreted tumor suppressor that inhibits angiogenesis. Oncogene. (2014) 33:2273–8. doi: 10.1038/onc.2013.174
33. Cazes A, Galaup A, Chomel C, Bignon M, Bréchot N, Le Jan S, et al. Extracellular matrix-bound angiopoietin-like 4 inhibits endothelial cell adhesion, migration, and sprouting and alters actin cytoskeleton. Circ Res. (2006) 99:1207–15. doi: 10.1161/01.RES.0000250758.63358.91
34. Kaneda H, Arao T, Matsumoto K, de Velasco MA, Tamura D, Aomatsu K, et al. Activin A inhibits vascular endothelial cell growth and suppresses tumour angiogenesis in gastric cancer. Br J Cancer. (2011) 105:1210–7. doi: 10.1038/bjc.2011.348
35. Liao Y, Smyth GK, Shi W. The R package Rsubread is easier, faster, cheaper and better for alignment and quantification of RNA sequencing reads. Nucleic Acids Res. (2019) 47:e47. doi: 10.1093/nar/gkz114
36. Fuchs M, Kreutzer FP, Kapsner LA, Mitzka S, Just A, Perbellini F, et al. Integrative bioinformatic analyses of global transcriptome data decipher novel molecular insights into cardiac anti-fibrotic therapies. Int J Mol Sci. (2020) 21:4727. doi: 10.3390/ijms21134727
37. Stojanović SD, Fuchs M, Fiedler J, Xiao K, Meinecke A, Just A, et al. Comprehensive bioinformatics identifies key microRNA players in ATG7-deficient lung fibroblasts. Int J Mol Sci. (2020) 21:4126. doi: 10.3390/ijms21114126
38. Kuri P, Ellwanger K, Kufer TA, Leptin M, Bajoghli B. A high-sensitivity bi-directional reporter to monitor NF-κB activity in cell culture and zebrafish in real time. J Cell Sci. (2017) 130:648–57. doi: 10.1242/jcs.196485
Keywords: hypoxia, transcription factor, endothelial, angiogenesis, promoter profiling, signaling
Citation: Schmidt A, Fuchs M, Stojanović SD, Liang C, Schmidt K, Jung M, Xiao K, Weusthoff J, Just A, Pfanne A, Distler JHW, Dandekar T, Fiedler J, Thum T and Kunz M (2022) Deciphering Pro-angiogenic Transcription Factor Profiles in Hypoxic Human Endothelial Cells by Combined Bioinformatics and in vitro Modeling. Front. Cardiovasc. Med. 9:877450. doi: 10.3389/fcvm.2022.877450
Received: 16 February 2022; Accepted: 23 May 2022;
Published: 17 June 2022.
Edited by:
Eoghan Martin Cunnane, University of Pittsburgh, United StatesCopyright © 2022 Schmidt, Fuchs, Stojanović, Liang, Schmidt, Jung, Xiao, Weusthoff, Just, Pfanne, Distler, Dandekar, Fiedler, Thum and Kunz. This is an open-access article distributed under the terms of the Creative Commons Attribution License (CC BY). The use, distribution or reproduction in other forums is permitted, provided the original author(s) and the copyright owner(s) are credited and that the original publication in this journal is cited, in accordance with accepted academic practice. No use, distribution or reproduction is permitted which does not comply with these terms.
*Correspondence: Meik Kunz, bWVpay5rdW56QGZhdS5kZQ==; Thomas Thum, VGh1bS5UaG9tYXNAbWgtaGFubm92ZXIuZGU=; Jan Fiedler, SmFuLkZpZWRsZXJAaXRlbS5mcmF1bmhvZmVyLmRl
†These authors share first authorship
‡These authors share last authorship
Disclaimer: All claims expressed in this article are solely those of the authors and do not necessarily represent those of their affiliated organizations, or those of the publisher, the editors and the reviewers. Any product that may be evaluated in this article or claim that may be made by its manufacturer is not guaranteed or endorsed by the publisher.
Research integrity at Frontiers
Learn more about the work of our research integrity team to safeguard the quality of each article we publish.