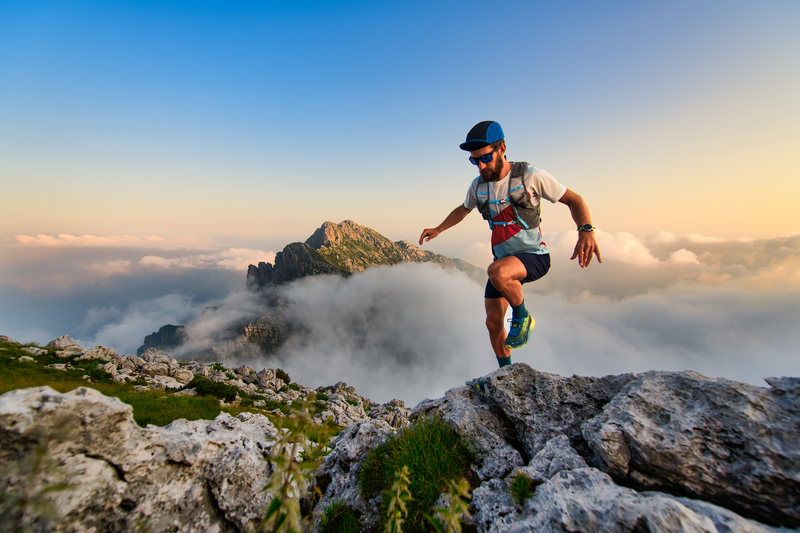
95% of researchers rate our articles as excellent or good
Learn more about the work of our research integrity team to safeguard the quality of each article we publish.
Find out more
ORIGINAL RESEARCH article
Front. Cardiovasc. Med. , 03 June 2022
Sec. Pediatric Cardiology
Volume 9 - 2022 | https://doi.org/10.3389/fcvm.2022.860600
Objective: Congenital heart disease (CHD) is complex in its etiology. Its genetic causes have been investigated, whereas the non-genetic factor related studies are still limited. We aimed to identify dominant parental predictors and develop a predictive model and nomogram for the risk of offspring CHD.
Methods: This was a retrospective study from November 2017 to December 2021 covering 44,578 participants, of which those from 4 hospitals in eastern China were assigned to the development cohort and those from 5 hospitals in central and western China were used as the external validation cohort. Univariable and multivariable analyses were used to select the dominant predictors of CHD among demographic characteristics, lifestyle behaviors, environmental pollution, maternal disease history, and the current pregnancy information. Multivariable logistic regression analysis was used to construct the model and nomogram using the selected predictors. The predictive model and the nomogram were both validated internally and externally. A web-based nomogram was developed to predict patient-specific probability for CHD.
Results: Dominant risk factors for offspring CHD included increased maternal age [odds ratio (OR): 1.14, 95% CI: 1.10–1.19], increased paternal age (1.05, 95% CI: 1.02–1.09), maternal secondhand smoke exposure (2.89, 95% CI: 2.22–3.76), paternal drinking (1.41, 95% CI: 1.08–1.84), maternal pre-pregnancy diabetes (3.39, 95% CI: 1.95–5.87), maternal fever (3.35, 95% CI: 2.49–4.50), assisted reproductive technology (2.89, 95% CI: 2.13–3.94), and environmental pollution (1.61, 95% CI: 1.18–2.20). A higher household annual income (100,000–400,000 CNY: 0.47, 95% CI: 0.34–0.63; > 400,000 CNY: 0.23, 95% CI: 0.15–0.36), higher maternal education level (13–16 years: 0.68, 95% CI: 0.50–0.93; ≥ 17 years: 0.87, 95% CI: 0.55–1.37), maternal folic acid (0.21, 95% CI: 0.16–0.27), and multivitamin supplementation (0.33, 95% CI: 0.26–0.42) were protective factors. The nomogram showed good discrimination in both internal [area under the receiver-operating-characteristic curve (AUC): 0.843] and external validations (development cohort AUC: 0.849, external validation cohort AUC: 0.837). The calibration curves showed good agreement between the nomogram-predicted probability and actual presence of CHD.
Conclusion: We revealed dominant parental predictors and presented a web-based nomogram for the risk of offspring CHD, which could be utilized as an effective tool for quantifying the individual risk of CHD and promptly identifying high-risk population.
Congenital heart disease (CHD) is the most common structural birth defect with an incidence rate of 6–12 per 1,000 newborns (1–4), and its estimated prevalence is about 19–20 per 1,000 newborns when the bicuspid aortic valve is included (5, 6). In China, a national study reported an overall CHD prevalence of 9 per 1,000 newborns (7), and it remains the leading non-infectious cause of death in infancy and childhood despite tremendous progress in surgical therapies (8). CHD imposes a substantial burden on survivors, families, and societies. It is of critical importance to identify dominant risk factors for CHD, and to predict the individual risk of CHD, in order to provide more exhaustive examinations and preventive interventions to pregnant women with higher-risk fetuses for CHD.
Congenital heart disease is complex in its etiology, although much evidence revealed its genetic causes (9, 10), and genetic defects were not detected in many CHD patients. Therefore, non-genetic factors should still be well considered. In recent years, substantial epidemiological evidence has demonstrated that non-genetic factors such as social determinants of health and adverse healthy lifestyle also contribute to abnormal cardiovascular development in fetuses, leading to cardiovascular malformation. The dominant factors of CHD could be divided into six main categories, namely (1) sociodemographic factors, including parental age (11, 12), household annual income and education level (13); (2) adverse lifestyle (14); (3) maternal diseases, including pre-pregnancy diabetes (15) and fever (16); (4) environmental factors such as secondhand smoke exposure (17) and environmental pollution (18); (5) supplements such as folic acid and multivitamin (19); and (6) mode of conception (20). Previous research mainly focused on the association between limited predictors and the risk of CHD, and some conclusions involving maternal fever (16, 21), maternal folic acid, and multivitamin supplementation (19, 22, 23) have been inconsistent. Moreover, the assessment in most of the prior studies was based on data from a single or limited number of medical institutions and was not internally or externally validated, which may have influenced the generalizability and robustness of the results. To the best of our knowledge, no study has been reported on the combined effect of the above factors on the risk of offspring CHD till present. Multicenter collaboration, rigorous study design, and adequate validation are important elements in the effort to develop a reliable prediction tool for offspring CHD based on multiple factors.
Therefore, the aim of this study was to (a) identify and evaluate the dominant predictors of offspring CHD and (b) develop a predictive model and web-based nomogram to directly indicate the probability of offspring CHD occurrence of each pregnant woman.
This was a retrospective study and we used data from the China Birth Cohort Study (CBCS), which is a population-based birth defect surveillance system in China from November 2017 to December 2021 (24). The CBCS was conducted in 38 research sites in 17 provinces, cities, autonomous regions, and municipalities covering most areas of China. We obtained data access permissions for 9 hospitals among them. Data of each participant including birth outcome, demographic characteristics, lifestyle behaviors, environmental pollution, maternal disease history, and the current pregnancy information were required to be reported by trained obstetricians, sonographers, and pediatricians for the same CBCS system. To build a robust nomogram, participants from four hospitals in eastern China, including Beijing Obstetrics and Gynecology Hospital, were used as a development cohort. Participants from five hospitals in central and western China were used as an external validation cohort. All participants’ data of the above nine hospitals were collected. For the development cohort, 29,733 participants were initially recruited. Of these, 25 participants were excluded because of significant neurological or psychiatric illness of pregnant women (the accuracy of questionnaires could not be guaranteed), 494 multiple pregnancies were excluded since etiology of CHD may be different between singleton and multiple pregnancies (18), and a further 113 participants were excluded due to outliers or missing data in any variable of interest. Thus, 29,101 participants (28,798 normal + 303 with CHD) were retrieved for inclusion in the development cohort. At the same time, 15,477 participants (15,324 normal + 153 with CHD) were used as the external validation cohort using the same criteria as for the development cohort (Figure 1 and Supplementary Table 1). The sensitivity analysis that excluded participants with missing data yielded results similar to those presented (data not shown). This study was guided by the Transparent Reporting of a Multivariable Prediction Model for Individual Prognosis or Diagnosis (TRIPOD) criteria (Supplementary Table 2) (25).
Our primary end point was the presence or absence of offspring CHD. CHD cases included livebirths, stillbirths, and late abortions who were diagnosed as CHD for more than 17 weeks of gestation. All cases were evaluated by a trained obstetrician, pediatrician, or cardiologist before hospital discharge or within 3 days after birth. Two echocardiography experts independently examined the echocardiographic images of each CHD case, and they were blinded to the outcome of each other. If there was still a dispute, computed tomography, cardiac catheterization, or autopsy were used to further determine a CHD case. All CHD cases were classified and recorded based on the International Classification of Diseases, Tenth Revision Modification (ICD-10-CM).
We identified potential predictors using a literature search. The following candidate predictors were selected from our standard and structured questionnaires: parental age, parental ethnicity (Han or Minority), parental first-trimester body mass index (BMI), household annual income (< 100,000 CNY or 100,000–400,000 CNY or > 400,000 CNY), parental education level (≤ 12 years or 13–16 years or ≥ 17 years), parental smoking (yes or no), maternal secondhand smoke exposure (yes or no), parental drinking (yes or no), maternal pre-pregnancy diabetes (yes or no), maternal fever (yes or no), maternal folic acid supplementation (yes or no), maternal multivitamin supplementation (yes or no), mode of conception (natural conceived or assisted reproductive technology), and environmental pollution (yes or no).
The height and weight of the participants were obtained through an accurate measurement. The standing height was measured to the nearest 0.1 cm using a stadiometer (26). The value of weight was accurately measured using an electronic scale (BW-150; UWE, Beijing, China) with participants wearing light clothes, no shoes, and empty pockets (27). The BMI was calculated as the weight in kilograms divided by the square of the height in meters. Parental smoking or drinking was defined as mothers or fathers who smoked at least one cigarette per day or drank alcohol once a week for over 6 months (28). Maternal secondhand smoke exposure was defined as non-smokers being exposed to another person’s tobacco smoke for at least 15 min daily for more than 1 day per week (29). Pre-pregnancy diabetes of pregnant women was defined as type 1 or type 2 diabetes diagnosed before pregnancy (15). Maternal fever was defined as body temperature exceeding 38°C or self-reported fever experience in the first trimester (16). Environmental pollution of this study was defined as the presence of smelly water ditches, garbage stations, or coal-fired factories within 100 m of the residential area.
Data were given as a median (interquartile range) or n (%). The independent t-test and Mann–Whitney U test were used to analyze differences in continuous variables between groups, while the chi-square test and the Fisher’s exact test were used for categorical variables. The univariable and multivariable analyses were used to select the dominant predictors. The multivariable logistic regression analysis was used to construct a risk prediction model for offspring CHD, based on the selected predictors of the development cohort. The variables in the final multivariable model were selected by the bidirectional stepwise regression with the Akaike information criterion. The results were described as the odds ratio (OR) and 95% confidence interval (CI). In addition, the outliers and collinearity of multivariable regression model were assessed. A nomogram consisting of the dominant predictors was created to translate the prediction model into a visual scoring system to calculate the estimated probability of offspring CHD. Each predicator was assigned a “points” on the nomogram, based on its predictive ability for offspring CHD. High total points based on the sum of the assigned points for each predicator in the nomogram were associated with a high CHD probability. To validate the performance of the nomogram, both internal and external validations were conducted. For both of them, the discriminatory ability of the nomogram was assessed using the receiver-operating-characteristic curve (AUC); if the AUC was closer to 1, the nomogram was seen as having a good discriminatory ability (30); the calibration of the nomogram was assessed using the calibration curves. To assess possible overfitting, the Delong test was implemented to compare the AUCs. Besides, a web-based nomogram was created to directly indicate the patient-specific probability of offspring CHD occurrence. All statistical tests were performed using the R statistical software version 4.1.11. The statistical significance was assumed at P < 0.05.
The characteristics of participants in the development and external validation cohorts are presented in Table 1. In the development and external validation cohorts, the median [interquartile range (IQR)] age of maternal age was 31.0 (29.0–34.0) and 31.0 (28.0–34.0) years, respectively. The paternal age was 33.0 (30.0–36.0) and 33.0 (30.0–37.0) years, respectively. The development cohort had a similar parental first-trimester BMI, parental ethnicity, household annual income, parental smoking, maternal secondhand smoke exposure, parental drinking, maternal pre-pregnancy diabetes, mode of conception, and environmental pollution status compared with the external validation cohort. A total of 456 CHD cases were included in this study, of which the three most types were ventricular septal defect (35.75%), multiple congenital heart disease (26.10%), and Tetralogy of Fallot (13.38%) (Supplementary Table 3).
In univariable analysis based on the data of the development cohort, the predictors associated with offspring CHD were maternal age (1.19, 95% CI: 1.15–1.22), paternal age (1.13, 95% CI: 1.11–1.15), household annual income (100,000–400,000 CNY, 0.29, 95% CI: 0.22–0.37; > 400,000 CNY, 0.16, 95% CI: 0.11–0.23), maternal education (13–16 years, 0.40, 95% CI: 0.31–0.52; ≥ 17 years, 0.38, 95% CI: 0.27–0.54), paternal education (13–16 years, 0.51, 95% CI: 0.40–0.65; ≥ 17 years, 0.38, 95% CI: 0.26–0.54), maternal secondhand smoke exposure (2.44, 95% CI: 1.93–3.09), paternal smoking (2.00, 95% CI: 1.59–2.51), maternal drinking (1.95, 95% CI: 1.27–3.00), paternal drinking (2.36, 95% CI: 1.88–2.96), maternal pre-pregnancy diabetes (3.38, 95% CI: 2.08–5.48), maternal fever (3.49, 95% CI: 2.71–4.50), maternal folic acid supplementation (0.16, 95% CI: 0.13–0.21), maternal multivitamin supplementation (0.31, 95% CI: 0.25–0.39), mode of conception (5.24, 95% CI: 4.07–6.75), and environmental pollution (2.73, 95% CI: 2.12–3.53). In contrast, parental ethnicity, parental first-trimester BMI, and maternal smoking did not show any association with offspring CHD (Table 2).
Multivariable logistic regression analysis led us to exclude paternal education, paternal smoking, and maternal drinking with P values higher than 0.05. Dominant risk factors for offspring CHD included increased maternal age (1.14, 95% CI: 1.10–1.19), increased paternal age (1.05, 95% CI: 1.02–1.09), maternal secondhand smoke exposure (2.89, 95% CI: 2.22–3.76), paternal drinking (1.41, 95% CI: 1.08–1.84), maternal pre-pregnancy diabetes (3.39, 95% CI: 1.95–5.87), maternal fever (3.35, 95% CI: 2.49–4.50), assisted reproductive technology (2.89, 95% CI: 2.13–3.94), and environmental pollution (1.61, 95% CI: 1.18–2.20). A higher household annual income (100,000–400,000 CNY, 0.47, 95% CI: 0.34–0.63; > 400,000 CNY, 0.23, 95% CI: 0.15–0.36), higher maternal education level (13–16 years, 0.68, 95% CI: 0.50–0.93; ≥ 17 years, 0.87, 95% CI: 0.55–1.37), maternal folic acid supplementation (0.21, 95% CI: 0.16–0.27), and maternal multivitamin supplementation (0.33, 95% CI: 0.26–0.42) were found to be protective factors for offspring CHD (Table 2).
Twelve dominant predictors, including maternal age, paternal age, household annual income, maternal education, maternal secondhand smoke exposure, paternal drinking, maternal pre-pregnancy diabetes, maternal fever, maternal folic acid supplementation, maternal multivitamin supplementation, mode of conception, and environmental pollution, were used to construct the prediction model and nomogram. There was no outlier with a model covariate of approximately ± 0.05 (Supplementary Figure 1). The variance inflation factor values of the twelve covariates ranged from 1.10 to 2.06, indicating no multicollinearity in the model (Supplementary Table 4). The predictive ability of each independent predictor of the model for CHD is reflected in the nomogram (Figure 2).
Figure 2. Nomogram for predicting the risk of offspring congenital heart diseases. CNY, china yuan; CHD, congenital heart disease; Modifiable, modifiable factors; Non-modifiable, non-modifiable factors.
Internal validation of the nomogram based on 10-fold cross-validation method showed the AUC of 0.843, indicating the adequate overall risk discrimination power of the nomogram. The nomogram calibration curve showed good agreement between the nomogram-predicted probability of CHD and actual presence of CHD (Figure 3). As for external validation, the nomogram showed good discriminatory power, with AUCs of 0.849 and 0.837 in the development and the external validation cohorts, respectively (Figure 4). The Delong test revealed that the difference was not statistically significant between the AUCs of the nomogram (D = 0.627 and P = 0.531), indicating the absence of potential overfitting. The nomogram also showed good calibration in both the development and the external validation cohorts (Figure 5).
Figure 3. The calibration curve of internal validation. The 45-degree long gray solid line represents an ideal prediction, the brown dotted line represents the current nomogram we constructed, and the blue solid line is the bias-corrected fitted line of the nomogram using a 10-fold cross-validation method. The closer the blue solid line is to the ideal line, the better the calibration of the nomogram is.
Figure 4. Receiver-operating-characteristic curves for the prediction of congenital heart disease by nomogram in the development cohort (A) and the external validation cohort (B).
Figure 5. Calibration curves of nomogram for predicting offspring congenital heart disease, in the development cohort (A) and the external validation cohort (B). The 45-degree long gray solid line represents an ideal prediction, and the blue solid line represents the predictive performance of the nomogram. The closer the blue solid line is to the ideal line, the better the predictive performance of the nomogram is.
The web-based nomogram provided a user-friendly access, which is available at https://postdocxiaoman.shinyapps.io/DynNomCHDapp/ or the QR code (Supplementary Figure 2), and then the users could self-evaluate the risk of offspring CHD by simply selecting or filling the values of predictors on the user interface. For example, in case of a pregnant woman, aged 36 years and her husband aged 40 years, annual household income of over 400,000 (CNY), was educated for ≥ 17 years, exposed to secondhand smoke, alcoholic husband, maternal pre-pregnancy diabetes, maternal fever, maternal folic acid supplementation, maternal multivitamin supplementation, conceived through assisted reproductive technology, and not exposed to environmental pollution, corresponding to points of 64 + 36 + 0 + 5 + 30 + 12 + 38 + 33 + 0 + 0 + 30 + 0 = 248, the risk probability for offspring CHD was 0.226. The screenshots of the web-based nomogram are presented in Supplementary Figures 3–5.
The results of this study further revealed the non-genetic causes of offspring CHD. The web-based nomogram could potentially be utilized as a convenient and effective tool for quantifying the individual risk of CHD in clinical practice.
We found that certain sociodemographic factors were dominant predictors for offspring CHD, of which the increased maternal and paternal age were risk factors (11), and a higher household annual income and maternal education level were protective factors. Joinau et al. (12) found that the increased paternal age had a higher risk of offspring CHD (summary OR, 1.16; 95% CI, 1.07–1.25) based on a meta-analysis of 9,917,011 participants, which is consistent with our study. The same study also explained that the increased paternal age has been associated with the decreased quality of semen, and increased percentage of short telomeres, especially among those aged over 34–35 years. The study of Peyvandi et al. (31) supported the inverse association between the higher household annual income and offspring CHD risk. Poverty and a low education level limited the access to comprehensive health care service, which may be related to higher CHD risk in offspring. Qu et al. (13) reported 48% and 17% higher risks of CHD in offspring whose mothers have been educated for less than 12 years, and household income is less than 3,000 CNY/person/month. Interestingly, mothers who have been educated for 13–16 years showed a stronger protective effect against offspring CHD compared with those who have been educated for more than 17 years in this study, which may be attributed to excessive stress such as work-related stress during pregnancy of highly educated mothers (32).
Besides, we also found that adverse lifestyle behaviors and environments including maternal secondhand smoke exposure (17), paternal drinking, and environmental pollution increased the risk of offspring CHD. The association between secondhand smoke exposure and offspring CHD may be explained by the fact that carbon monoxide and nicotine induce maternal and fetal circulatory endothelial dysfunction, reduced blood flow to the placenta, and interruption of cardiac angiogenesis, affecting the development of fetal heart and the function of cardiomyocytes and aortic muscle cells (33). Nie et al. (14) confirmed that paternal drinking was associated with an increased risk of CHD (adjusted OR, 2.87; 95% CI: 2.25–3.65). Alcohol may cause damage to cardiac neural crest cells, and increase apoptosis and/or hamper migration, which are critical stages of cardiac early development (34). Yang et al. (18) proved that environmental pollution was associated with the increased CHD risk in offspring. Pregnant women who were exposed to environmental pollution showed increased markers of oxidative stress, which have been reported to be related to outflow tract defects (35). Bove et al. (35) indicated that ambient particulates in the maternal residential area could be transported to the fetuses (36).
In addition, we revealed that maternal pre-pregnancy diseases and the current pregnancy information were also associated with offspring CHD. Specifically, maternal pre-pregnancy diabetes, maternal fever, and assisted reproductive technology served as risk factors, and maternal folic acid supplementation and maternal multivitamin supplementation were protective factors. Wu et al. (15) indicated that maternal pre-pregnancy diabetes was associated with a greater risk of cyanotic CHD (adjusted RRs, 4.61; 95% CI: 4.28–4.96), which was in agreement with our results. These findings highlight the important role of counseling and the management of pre-pregnancy diabetes in the prevention of CHD. Previous studies on the association between maternal fever and CHD were controversial. Yang et al. (16) systematically reviewed 16 studies involving 183,563 participants and reported an association between the maternal fever during pregnancy and greater risk of CHD in offspring (OR, 1.45; 95% CI: 1.21–1.73), whereas a recent cohort study of 77,344 pregnant women failed to conclude such a relationship (21). In this study, maternal fever was a risk factor for offspring CHD, the possible explanation may be that fever could activate temperature-sensitive ion channels of neural crest cells in fetuses, resulting in cardiac defects (37). The association between maternal folic acid as well as multivitamin supplementation and the risk of offspring CHD was controversial (19, 22, 23). The protective effect of maternal folic acid and multivitamin supplementation against offspring CHD may be because folic acid and multivitamin have antagonistic effects on the process of vascular disruption, and have a rescue effect on apoptotic cells with folic acid deficiency (6). Offspring of our study conceived through assisted reproductive technology was more likely to develop CHD compared with those conceived spontaneously (20). Wang et al. supported our view and explained that fetuses conceived through assisted reproductive technology carried more germline de novo mutations than those conceived spontaneously, which could affect protein-coding genes and further result in a higher risk of CHD (38).
Previous studies on the prediction model of CHD were mainly focused on predicting the risk of adverse maternal or neonatal outcomes in pregnant women with CHD (39, 40). There are few studies on the development of the offspring CHD prediction model based on parental non-genetic and environmental factors. Liang et al. (6) built an offspring CHD prediction model using respiratory infections, water pollution, adverse emotions, and nutrition supplementation based on data from a single center involving 654 participants and reported an AUC of 0.72 (95% CI: 0.68–0.76). Our nomogram showed better discriminatory power with AUCs of 0.849 and 0.837 in the development and the external validation cohorts, respectively. Besides, the web-based nomogram developed in this study could quantify the individual risk of CHD and is freely available to users; it could provide women with affected fetuses reproductive choices (i.e., termination of pregnancy, continuation of pregnancy, or give up for adoption) at the earliest opportunity.
In China, the current recommendation is to offer second-trimester ultrasound examination to screen for CHD and other malformations. An effective screening method for CHD in the first trimester is still lacking. Our study has demonstrated that the nomogram achieves good performance in the development and external validation cohorts. This implies that, if the nomogram is used, pregnant women with high-risk of offspring CHD could be detected in the early stage of pregnancy, and more preventive interventions could be provided to them as early as possible. Therefore, screening of pregnant women during routine first-trimester prenatal examination to identify those with high risk of offspring CHD should be encouraged. In addition, most of the predictors included in our nomogram are easily accessible and modifiable. Healthcare workers and expectant parents should be educated about the risk and protective factors of CHD, and the corresponding study should also be conducted to promote the awareness of influences from non-genetic factors in social communities.
This study has several strengths. First, our study has a large sample size based on a nationwide multicenter study, which enabled us to build a nomogram with a small expected relative bias. Second, detailed information of participants was recorded, allowing us to analyze potential predictors as many as possible, including demographic characteristics, lifestyle behaviors, environmental pollution, maternal disease history, and the current pregnancy information of the study population. Third, the nomogram established shows good performance, the web-based nomogram could be conveniently and effectively applied to the prediction of CHD, especially in developing countries that lack well-developed pregnancy monitoring systems. Fourth, we conducted extensive internal and external validations using population from different centers based on TRIPOD criteria, which increases the generalizability of the findings.
However, we acknowledge that several limitations cannot be neglected. First, although we included as many predictors as possible in this study, we cannot rule out the possibility of some residual effects related to other factors such as the adverse emotion of pregnant women during pregnancy (32). Second, participants with outliers or missing data in any variable of interest were excluded, which may have contributed to bias in our findings, but we believe that our final conclusion is robust since the total rate of outliers and missing data is only 0.35% (160 of 45,504). Third, maternal/paternal data such as drinking, smoking, and environmental pollution were from self-administered questionnaire, which may decrease the reliability of our findings. However, the questions are presented in simple language and have been piloted, and the forms completed by participants of the study and medical staff have shown high concordance. Fourth, our participants were predominantly from a single ethnicity and tertiary hospitals, and the nomogram requires prospective validation in other ethnicities and general community populations. Fifth, the future use of our nomogram may be limited by the annual increase in household income like in 10 years when people have much higher income, so updating periodically is needed. The nomogram may not be applicable to individuals with factors lying outside the range of the nomogram such as younger or older pregnant women.
In this study, we have revealed dominant parental predictors and presented a web-based nomogram, which could potentially be utilized as a convenient and effective tool for quantifying the individual risk of CHD and promptly identifying high-risk population. Healthcare workers and expectant parents should be educated about the risk and protective factors of CHD, and the corresponding study should also be conducted for government to improve their education and restrict the number of smelly water ditches, garbage stations, or coal-fired factories near the residential area.
The original contributions presented in the study are included in the article/Supplementary Material, further inquiries can be directed to the corresponding authors.
The studies involving human participants were reviewed and approved by the Ethical Committee of Beijing Obstetrics and Gynecology Hospital, Capital Medical University (number: 2018-KY-003-01). The patients/participants provided their written informed consent to participate in this study.
WY had full access to all the data of the study and took responsibility for the integrity and accuracy of the data analysis. WY, CY, G-HD, WZ, MZ, and YS contributed to concept and design. MZ, YS, WZ, WY, CY, G-HD, B-YY, GC, XZ, and RL contributed to acquisition, analysis, or interpretation of data. MZ, YS, WY, CY, G-HD, and WZ contributed in drafting the manuscript. MZ, YS, and WZ contributed to statistical analysis. WY, CY, MZ, and YS obtained funding. WY, CY, G-HD, and WZ contributed to supervision. All authors contributed to administrative, technical, and material support and contributed to critical revisions of the manuscript for important intellectual contents.
This study was supported by the National Key Research and Development Program of China (Grant Numbers 2016YFC1000101 and 2019YFC1005100), the China Postdoctoral Science Foundation (Grant Number 2020TQ0207), and the Postdoctoral Foundation provide by Beijing Obstetrics and Gynecology Hospital, Capital Medical University.
The authors declare that the research was conducted in the absence of any commercial or financial relationships that could be construed as a potential conflict of interest.
All claims expressed in this article are solely those of the authors and do not necessarily represent those of their affiliated organizations, or those of the publisher, the editors and the reviewers. Any product that may be evaluated in this article, or claim that may be made by its manufacturer, is not guaranteed or endorsed by the publisher.
The Supplementary Material for this article can be found online at: https://www.frontiersin.org/articles/10.3389/fcvm.2022.860600/full#supplementary-material
1. Moons P, Bratt EL, De Backer J, Goossens E, Hornung T, Tutarel O, et al. Transition to adulthood and transfer to adult care of adolescents with congenital heart disease: a global consensus statement of the ESC association of cardiovascular nursing and allied professions (ACNAP), the ESC working group on adult congenital heart disease (WG ACHD), the association for European paediatric and congenital cardiology (AEPC), the Pan-African society of cardiology (PASCAR), the Asia-Pacific pediatric cardiac society (APPCS), the inter-American society of cardiology (IASC), the cardiac society of Australia and New Zealand (CSANZ), the international society for adult congenital heart disease (ISACHD), the world heart federation (WHF), the European congenital heart disease organisation (ECHDO), and the global alliance for rheumatic and congenital hearts (Global ARCH). Eur Heart J. (2021) 42:4213–23. doi: 10.1093/eurheartj/ehab388
2. Fedchenko M, Mandalenakis Z, Giang KW, Rosengren A, Eriksson P, Dellborg M. Long-term outcomes after myocardial infarction in middle-aged and older patients with congenital heart disease-a nationwide study. Eur Heart J. (2021) 42:2577–86. doi: 10.1093/eurheartj/ehaa874
3. Fa J, Zhang X, Zhang X, Qi M, Zhang X, Fu Q, et al. Long noncoding RNA lnc-TSSK2-8 activates canonical Wnt/beta-catenin signaling through small heat shock proteins HSPA6 and CRYAB. Front Cell Dev Biol. (2021) 9:660576. doi: 10.3389/fcell.2021.660576
4. Pennig L, Wagner A, Weiss K, Lennartz S, Grunz JP, Maintz D, et al. Imaging of the pulmonary vasculature in congenital heart disease without gadolinium contrast: intraindividual comparison of a novel compressed SENSE accelerated 3D modified REACT with 4D contrast-enhanced magnetic resonance angiography. J Cardiovasc Magn Reson. (2020) 22:8. doi: 10.1186/s12968-019-0591-y
5. Basu M, Garg V. Maternal hyperglycemia and fetal cardiac development: clinical impact and underlying mechanisms. Birth Defects Res. (2018) 110:1504–16. doi: 10.1002/bdr2.1435
6. Liang Y, Li X, Hu X, Wen B, Wang L, Wang C. A predictive model of offspring congenital heart disease based on maternal risk factors during pregnancy: a hospital based case-control study in Nanchong City. Int J Med Sci. (2020) 17:3091–7. doi: 10.7150/ijms.48046
7. Zhao QM, Liu F, Wu L, Ma XJ, Niu C, Huang GY. Prevalence of congenital heart disease at live birth in China. J Pediatr. (2019) 204:53–8. doi: 10.1016/j.jpeds.2018.08.040
8. Chu Q, Jiang H, Zhang L, Zhu D, Yin Q, Zhang H, et al. CACCT: an automated tool of detecting complicated cardiac malformations in mouse models. Adv Sci (Weinh). (2020) 7:1903592. doi: 10.1002/advs.201903592
9. Morton SU, Quiat D, Seidman JG, Seidman CE. Genomic frontiers in congenital heart disease. Nat Rev Cardiol. (2022) 19:26–42. doi: 10.1038/s41569-021-00587-4
10. Majumdar U, Yasuhara J, Garg V. In vivo and in vitro genetic models of congenital heart disease. Cold Spring Harb Perspect Biol. (2021) 13:a036764. doi: 10.1101/cshperspect.a036764
11. Schulkey CE, Regmi SD, Magnan RA, Danzo MT, Luther H, Hutchinson AK, et al. The maternal-age-associated risk of congenital heart disease is modifiable. Nature. (2015) 520:230–3. doi: 10.1038/nature14361
12. Joinau-Zoulovits F, Bertille N, Cohen JF, Khoshnood B. Association between advanced paternal age and congenital heart defects: a systematic review and meta-analysis. Hum Reprod. (2020) 35:2113. doi: 10.1093/humrep/deaa105
13. Qu Y, Lin S, Bloom MS, Wang X, Ye B, Nie Z, et al. Maternal folic acid supplementation mediates the associations between maternal socioeconomic status and congenital heart diseases in offspring. Prev Med. (2021) 143:106319. doi: 10.1016/j.ypmed.2020.106319
14. Nie Z, Qu Y, Han F, Bell EM, Zhuang J, Chen J, et al. Evaluation of interactive effects between paternal alcohol consumption and paternal socioeconomic status and environmental exposures on congenital heart defects. Birth Defects Res. (2020) 112:1273–86. doi: 10.1002/bdr2.1759
15. Wu Y, Liu B, Sun Y, Du Y, Santillan MK, Santillan DA, et al. Association of maternal prepregnancy diabetes and gestational diabetes mellitus with congenital anomalies of the newborn. Diabetes Care. (2020) 43:2983–90. doi: 10.2337/dc20-0261
16. Yang G, Deng X, Xiao J, Huang P, Zhang K, Li Y. Maternal fever during preconception and conception is associated with congenital heart diseases in offspring: an updated meta-analysis of observational studies. Medicine (Baltimore). (2021) 100:e24899. doi: 10.1097/MD.0000000000024899
17. Liu X, Nie Z, Chen J, Guo X, Ou Y, Chen G, et al. Does maternal environmental tobacco smoke interact with social-demographics and environmental factors on congenital heart defects? Environ Pollut. (2018) 234:214–22. doi: 10.1016/j.envpol.2017.11.023
18. Yang BY, Qu Y, Guo Y, Markevych I, Heinrich J, Bloom MS, et al. Maternal exposure to ambient air pollution and congenital heart defects in China. Environ Int. (2021) 153:106548. doi: 10.1016/j.envint.2021.106548
19. Feng Y, Cai J, Tong X, Chen R, Zhu Y, Xu B, et al. Non-inheritable risk factors during pregnancy for congenital heart defects in offspring: a matched case-control study. Int J Cardiol. (2018) 264:45–52. doi: 10.1016/j.ijcard.2018.04.003
20. Hautala J, Gissler M, Ritvanen A, Tekay A, Pitkanen-Argillander O, Stefanovic V, et al. The implementation of a nationwide anomaly screening programme improves prenatal detection of major cardiac defects: an 11-year national population-based cohort study. BJOG. (2019) 126:864–73. doi: 10.1111/1471-0528.15589
21. Sass L, Urhoj SK, Kjaergaard J, Dreier JW, Strandberg-Larsen K, Nybo AA. Fever in pregnancy and the risk of congenital malformations: a cohort study. BMC Pregnancy Childbirth. (2017) 17:413. doi: 10.1186/s12884-017-1585-0
22. Oyen N, Olsen SF, Basit S, Leirgul E, Strom M, Carstensen L, et al. Association between maternal folic acid supplementation and congenital heart defects in offspring in birth cohorts from Denmark and Norway. J Am Heart Assoc. (2019) 8:e11615. doi: 10.1161/JAHA.118.011615
23. Gildestad T, Bjorge T, Haaland OA, Klungsoyr K, Vollset SE, Oyen N. Maternal use of folic acid and multivitamin supplements and infant risk of birth defects in Norway, 1999-2013. Br J Nutr. (2020) 124:316–29. doi: 10.1017/S0007114520001178
24. Yue W, Zhang E, Liu R, Zhang Y, Wang C, Gao S, et al. The China birth cohort study (CBCS). Eur J Epidemiol. (2022) 37:295–304. doi: 10.1007/s10654-021-00831-8
25. Patzer RE, Kaji AH, Fong Y. TRIPOD reporting guidelines for diagnostic and prognostic studies. JAMA Surg. (2021) 156:675–6. doi: 10.1001/jamasurg.2021.0537
26. Bragg F, Tang K, Guo Y, Iona A, Du H, Holmes MV, et al. Associations of general and central adiposity with incident diabetes in Chinese men and women. Diabetes Care. (2018) 41:494–502. doi: 10.2337/dc17-1852
27. Hunt K, Wyke S, Gray CM, Anderson AS, Brady A, Bunn C, et al. A gender-sensitised weight loss and healthy living programme for overweight and obese men delivered by Scottish premier league football clubs (FFIT): a pragmatic randomised controlled trial. Lancet. (2014) 383:1211–21. doi: 10.1016/S0140-6736(13)62420-4
28. Liu Y, Zhu Y, Jia W, Sun D, Zhao L, Zhang C, et al. Association between lipid profiles and presence of carotid plaque. Sci Rep. (2019) 9:18011. doi: 10.1038/s41598-019-54285-w
29. Carroll X, Liang X, Zhang W, Zhang W, Liu G, Turner N, et al. Socioeconomic, environmental and lifestyle factors associated with gestational diabetes mellitus: a matched case-control study in Beijing, China. Sci Rep. (2018) 8:8103. doi: 10.1038/s41598-018-26412-6
30. Connolly RM, Leal JP, Solnes L, Huang CY, Carpenter A, Gaffney K, et al. TBCRC026: phase II trial correlating standardized uptake value with pathologic complete response to pertuzumab and trastuzumab in breast cancer. J Clin Oncol. (2019) 37:714–22. doi: 10.1200/JCO.2018.78.7986
31. Peyvandi S, Baer RJ, Chambers CD, Norton ME, Rajagopal S, Ryckman KK, et al. Environmental and socioeconomic factors influence the live-born incidence of congenital heart disease: a population-based study in California. J Am Heart Assoc. (2020) 9:e15255. doi: 10.1161/JAHA.119.015255
32. Gu J, Guan HB. Maternal psychological stress during pregnancy and risk of congenital heart disease in offspring: a systematic review and meta-analysis. J Affect Disord. (2021) 291:32–8. doi: 10.1016/j.jad.2021.05.002
33. Zhao L, Chen L, Yang T, Wang L, Wang T, Zhang S, et al. Parental smoking and the risk of congenital heart defects in offspring: an updated meta-analysis of observational studies. Eur J Prev Cardiol. (2020) 27:1284–93. doi: 10.1177/2047487319831367
34. Kalisch-Smith JI, Ved N, Sparrow DB. Environmental risk factors for congenital heart disease. Cold Spring Harb Perspect Biol. (2020) 12:a037234. doi: 10.1101/cshperspect.a037234
35. Qi W, Bi J, Zhang X, Wang J, Wang J, Liu P, et al. Damaging effects of multi-walled carbon nanotubes on pregnant mice with different pregnancy times. Sci Rep. (2014) 4:4352. doi: 10.1038/srep04352
36. Bove H, Bongaerts E, Slenders E, Bijnens EM, Saenen ND, Gyselaers W, et al. Ambient black carbon particles reach the fetal side of human placenta. Nat Commun. (2019) 10:3866. doi: 10.1038/s41467-019-11654-3
37. Hutson MR, Keyte AL, Hernandez-Morales M, Gibbs E, Kupchinsky ZA, Argyridis I, et al. Temperature-activated ion channels in neural crest cells confer maternal fever-associated birth defects. Sci Signal. (2017) 10:eaal4055. doi: 10.1126/scisignal.aal4055
38. Wang C, Lv H, Ling X, Li H, Diao F, Dai J, et al. Association of assisted reproductive technology, germline de novo mutations and congenital heart defects in a prospective birth cohort study. Cell Res. (2021) 31:919–28. doi: 10.1038/s41422-021-00521-w
39. Chu R, Chen W, Song G, Yao S, Xie L, Song L, et al. Predicting the risk of adverse events in pregnant women with congenital heart disease. J Am Heart Assoc. (2020) 9:e16371. doi: 10.1161/JAHA.120.016371
Keywords: congenital heart disease, risk factors, web-based nomogram, prediction, China birth cohort
Citation: Zhang M, Sun Y, Zhao X, Liu R, Yang B-Y, Chen G, Zhang W, Dong G-H, Yin C and Yue W (2022) How Parental Predictors Jointly Affect the Risk of Offspring Congenital Heart Disease: A Nationwide Multicenter Study Based on the China Birth Cohort. Front. Cardiovasc. Med. 9:860600. doi: 10.3389/fcvm.2022.860600
Received: 23 January 2022; Accepted: 10 May 2022;
Published: 03 June 2022.
Edited by:
Andrew Landstrom, Duke University, United StatesReviewed by:
Sam Li, University of Tennessee Health Science Center (UTHSC), United StatesCopyright © 2022 Zhang, Sun, Zhao, Liu, Yang, Chen, Zhang, Dong, Yin and Yue. This is an open-access article distributed under the terms of the Creative Commons Attribution License (CC BY). The use, distribution or reproduction in other forums is permitted, provided the original author(s) and the copyright owner(s) are credited and that the original publication in this journal is cited, in accordance with accepted academic practice. No use, distribution or reproduction is permitted which does not comply with these terms.
*Correspondence: Wangjian Zhang, Wmhhbmd3ajIyN0BtYWlsLnN5c3UuZWR1LmNu; Guang-Hui Dong, ZG9uZ2doNUBtYWlsLnN5c3UuZWR1LmNu; Chenghong Yin, eWluY2hoQGNjbXUuZWR1LmNu; Wentao Yue, eXVld3RAY2NtdS5lZHUuY24=
†These authors have contributed equally to this work and share first authorship
Disclaimer: All claims expressed in this article are solely those of the authors and do not necessarily represent those of their affiliated organizations, or those of the publisher, the editors and the reviewers. Any product that may be evaluated in this article or claim that may be made by its manufacturer is not guaranteed or endorsed by the publisher.
Research integrity at Frontiers
Learn more about the work of our research integrity team to safeguard the quality of each article we publish.