- 1Institute of Applied Dynamics, Friedrich-Alexander-Universität Erlangen-Nürnberg, Erlangen, Germany
- 2Department of Cardiac Surgery, Friedrich-Alexander-Universität Erlangen-Nürnberg, Erlangen, Germany
The present computational study investigates the effects of an epicardial support pressure mimicking a heart support system without direct blood contact. We chose restrictive cardiomyopathy as a model for a diseased heart. By changing one parameter representing the amount of fibrosis, this model allows us to investigate the impairment in a diseased left ventricle, both during diastole and systole. The aim of the study is to determine the temporal course and value of the support pressure that leads to a normalization of the cardiac parameters in diseased hearts. These are quantified via the end-diastolic pressure, end-diastolic volume, end-systolic volume, and ejection fraction. First, the amount of fibrosis is increased to model diseased hearts at different stages. Second, we determine the difference in the left ventricular pressure between a healthy and diseased heart during a cardiac cycle and apply for the epicardial support as the respective pressure difference. Third, an epicardial support pressure is applied in form of a piecewise constant step function. The support is provided only during diastole, only during systole, or during both phases. Finally, the support pressure is adjusted to reach the corresponding parameters in a healthy rat. Parameter normalization is not possible to achieve with solely diastolic or solely systolic support; for the modeled case with 50% fibrosis, the ejection fraction can be increased by 5% with purely diastolic support and 14% with purely systolic support. However, the ejection fraction reaches the value of the modeled healthy left ventricle (65.6%) using a combination of diastolic and systolic support. The end-diastolic pressure of 13.5 mmHg cannot be decreased with purely systolic support. However, the end-diastolic pressure reaches the value of the modeled healthy left ventricle (7.5 mmHg) with diastolic support as well as with the combination of the diastolic and systolic support. The resulting negative diastolic support pressure is −4.5 mmHg, and the positive systolic support pressure is 90 mmHg. We, thereby, conclude that ventricular support during both diastole and systole is beneficial for normalizing the left ventricular ejection fraction and the end-diastolic pressure, and thus it is a potentially interesting therapy for cardiac insufficiency.
1. Introduction
Cardiovascular diseases remain the leading cause of death worldwide (1). If conservative therapy is inadequate due to the pathological condition of the heart, there are usually only two options remaining to treat this condition: cardiac assist devices or heart transplantation. However, there is a chronic shortage of donors. For example, in Germany, 320 patients received a donor heart in 2020, while the remaining 700 patients were still on the waiting list at the end of that year (2). While heart transplantation is a superior option in terms of survival and functional capacity, significant improvements in the field of cardiac assist devices have resulted in promising solutions that close the gap between availability and demand for donor's hearts. Over the last decades, various types of mechanical pumping systems, known as left ventricular (LV) assist devices, have been developed (3). They are used as an invasive form of therapy to directly support blood circulation. In principle, these systems mostly work to bypass the weakened heart. Such cardiac assist devices can relieve the load on the heart and become either a short- to medium-term bridge to treat cardiac insufficiency until possible transplantation (bridge-to-transplantation) or a permanent solution (destination therapy). Despite the rapid development of new devices and improvements in post-surgery survival and functional capacity nearing those of heart transplantation, there are still many problems to solve, including bleeding, thrombosis, strokes, and infections (3, 4).
For the most part, these problems are caused by the direct contact of blood with the cardiac assist device. Several approaches to avoiding direct blood contact, commonly referred to as direct cardiac compression devices, have been proposed to overcome such difficulties. The first bridge-to-transplantation based on a pneumatic compression cup (5) was followed by several other innovative approaches, e.g., (6–9). Review articles provide a more detailed overview of available direct cardiac compression devices (3, 10). For example, systems from AdjuCor GmbH (8) and CorInnova Inc. (9), currently in the preclinical testing phase, provide support during systole and are minimally invasive implants. The common principle of these devices is that they develop a pressure (force per area) that acts on the epicardial surface. Theoretically, a diseased heart can be thereby supported solely in the diastole, solely in the systole, or in both cardiac phases. However, most direct cardiac compression devices only work during systole. The objective of our computational study is to investigate (1) if an application of support pressure on the LV during both the diastolic and systolic phases is able to normalize the ejection fraction (EF) and left ventricular end-diastolic pressure (EDP) and (2) how much pressure is needed. The current study does not focus on the modeling of a particular cardiac assist device.
In general, the functionality of a direct cardiac compression device is mainly determined by the improvement in the pump function of a diseased heart. In the present study, it is quantified via the EF and EDP. However, the influence of a direct cardiac compression device on cardiac performance is not straightforward to compute, due to, e.g., the varying mechanical properties of active biological cardiac tissue that is undergoing complex deformations. The complex orthotropic tissue structure of the healthy myocardium, which can be modeled with different approaches (11–13), plays an important role. Furthermore, the amount of fibrosis in the ventricular wall strongly influences cardiac performance. Over the course of many heart diseases, there is a remodeling process that leads to an increase in fibrosis (14, 15). This is often independent of the triggering disease and can occur after cardiac volumetric pressure loading or ischemia (16). Various studies indicate a correlation between diastolic function and the amount of myocardial fibrosis (17, 18). Regardless of the functional impact of primary cardiomyopathy, the fibrosis progressively limits the diastolic function of the ventricle (19). As long as the condition can be systolically compensated, there is no reduction in the overall myocardial function (heart failure with preserved ejection fraction) (20). However, if the amount of fibrosis exceeds a certain level, the reduction in the diastolic function can no longer be systolically compensated, and the overall myocardial function is reduced (19). It has been shown that ventricular fibrosis inversely correlates with the ejection fraction, both in rats (21) and humans (17). Since the functional impairment resulting from the diastolic dysfunction due to myocardial fibrosis is the terminal stage of most cardiac diseases (17), a better understanding of the role of diastolic function and its relationship with the amount of fibrosis is important. We, therefore, calculated the effects of increasing fibrosis on cardiac function in a computational model of restrictive cardiomyopathy. Studies using postmortem mechanical testing in animal models after myocardial infarction have shown that the fibrosis leads to the stiffening of the cardiac tissue (22–25).
To optimally support a diseased heart via a direct cardiac compression device, two main factors play an important role: the time evolution of the force generated by the device and its maximum and minimum values. To date, how these factors influence cardiac function has not been investigated in detail. In this early research study, a computer simulation offers many advantages. First, it saves time compared to experimental testing. Second, there are beneficial synergies between computational modeling and simulation and experimental testing; for example, various parameters can be predicted that cannot be directly measured. Third, modeling and simulation can eventually be used to improve the adaptivity of such a system at a patient-specific level, making it a fundamental instrument in modern and future medicine. So far, a few finite element-based computational models that account for the coupling between a cardiac assist device and the heart have been developed. The existing work presents simulations for the ventricular pumps that are coupled with a univentricular (26) or biventricular heart models (27) via a cannula. A computational model for the innovative support system from AdjuCor GmbH is presented in Hirschvogel et al. (28). Recently, Chavanne (29) presented a simulation of a dielectric elastomer actuator-based aortic plaster interacting with a lumped parameter model for the heart.
The present study uses a finite element-based computer simulation. The study models an actively contracting rat LV with different amounts of fibrosis (30) and investigates the influence of direct cardiac compression devices supporting the LV on cardiac performance during both diastolic and systolic phases. As the current study does not focus on modeling a particular cardiac assist device, for simplicity, the model represents a support pressure acting on the outer surface of the modeled LV, as depicted in Figure 2A. During diastole, a negative support pressure is modeled to facilitate the ventricular filling, whereas a positive support pressure in systole is applied to eventually support the blood outflow from the LV. In particular, we investigate whether a support pressure calculated as a difference between the modeled ventricular pressure in a fibrotic and healthy LV would lead to a normalization of cardiac function parameters. Subsequently, the maximum positive systolic and negative diastolic support pressures are optimized such that the EF, EDP, and left ventricular end-diastolic volume (EDV) of a control healthy rat LV are restored. In the present study, a simplified rat LV ventricle is computationally modeled.
2. Methods
This section describes the computational model of the contraction of a rat LV and the associated numerical experiments.
2.1. Modeling
2.1.1. Balance Equations and Support Pressure Boundary Conditions
The field equation governing the state of the material point X∈Ω0 at time t, t∈[t0, tf] (t0 and tf are the initial and final times, respectively) can be formulated. The mechanical field equation is the balance of linear momentum together with the boundary conditions on the boundaries Γφ, Γ1, and Γ2:
where F is the deformation gradient with its determinant J = detF, S is the second Piola Kirchhoff stress (PK2), Fφ is the external mechanical body force, φ is the displacement with prescribed value on the boundary Γφ, T is the surface traction vector in the reference configuration, N is the outer unit normal in the reference configuration and pi are the prescribed values of the pressures acting on the boundaries Γi, i∈{1, 2}. The pressure p1 in the LV, obtained from the three-element Windkessel model (representing the interaction between the LV, aorta, and peripheral arteries), serves as a Neumann boundary condition on the endocardial surface Γ1 whereas the support pressure p2 serves as a Neumann boundary condition on the epicardial surface Γ2. The basis of the LV (Γφ) is fixed in the longitudinal direction; additionally, the nodes on the outer basis are fixed in all directions (31).
2.1.2. Constitutive Equations
In the present study, the total PK2 is additively decomposed into the passive part Spas and the active part Sact (31–35), namely
Based on Martonová et al. (23), we model the LV as a mixture of the intact myocardium and fibrotic scar structure. The amount of fibrosis, fib, serves as a scaling factor. Furthermore, we assume that only the intact muscle tissue is able to contract (30, 36), and therefore the active part of the stress tensor is as well-scaled by the amount of fibrosis. The resulting PK2 reads as
where the superscripts s and m correspond to the scar and intact myocardium and the subscripts pas and act to the passive and active parts of the PK2, respectively. In particular, by setting fib = 0, only the intact cardiac tissue is modeled. As proposed in Martonová et al. (23) for the passive part, the scar structure is modeled as a transversely isotropic material and the intact myocardium as an orthotropic material, according to Holzapfel and Ogden (37). The active contraction of the intact myocardium is modeled following the simple time-dependent approach from Pfaller et al. (38):
The temporal evolution of the active tension T depicted in Figure 1 is obtained by using the parameters shown in Table A1. For the equations describing T(t), we refer to the Appendix or the original study (38).
Based on the experimental evidence (39) and our previous study (30), in addition to the contraction in the fiber direction f0, reduced active stress along the cross-fiber direction n0 is added and scaled by ν in Equation (5). We note that the electromechanical coupling is omitted in this study as it would introduce further complexity and variability. However, the model can be coupled with a model for electrical excitation (38). The constitutive model is applied to the generic ellipsoidal rat LV based on the data from echocardiography. For more details regarding the geometry, fiber orientation, compressibility, and the Windkessel model serving as a boundary condition in Equation (1), we refer to previous study (35).
2.1.3. Diastolic Filling
The blood flow I between the left atrium and LV is given as
where Δp is the pressure difference between the left atrium and LV, and Rv1 is the resistance of the atrioventricular valve. The pressure in the atrium pa is modeled according to the following equation:
where pa1 and pa2 are the minimum and maximum atrial pressures, and ta, ted model the onset of the atrial and ventricular contraction, respectively. The resulting curve is shown in Figure 1. We note that the atrial pressure is as well-scaled by the amount of fibrosis, allowing us to model the higher EDP observed in rats with different amounts of fibrosis after myocardial infarction (40). To avoid an unlimited blood inflow from the atrium to the LV, the maximal EDV is restricted to that of the control rat.
2.2. Numerical Experiments
In the following, p(t) defines the support pressure acting on the epicardial surface of the LV. Three numerical experiments are performed.
First, the amount of fibrosis is varied from 0% to 60% in order to compare the cardiac performance represented by the EF in the fibrotic rat LV at different fibrosis stages without a support pressure.
Second, a support pressure is applied in order to increase the diastolic and systolic performance of the diseased LV at different fibrosis stages. The support pressure is computed as a difference between the left ventricular pressure in the control and diseased LV at each time point during the cardiac cycle (Figure 2B), namely
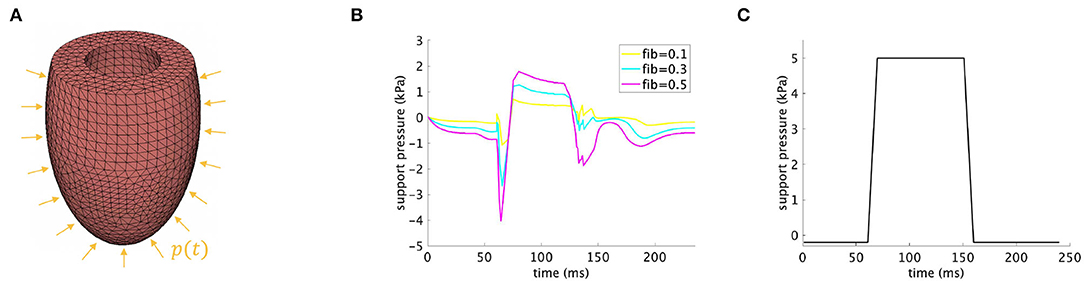
Figure 2. (A) Discretized model of the rat LV with applied support pressure p(t) on the epicardial surface. (B) Time-dependent support pressure according to Equation (8) for fib∈{0.1, 0.3, 0.5}. (C) Time-dependent support pressure according to Equation (9).
where is the pressure in the control LV (fib = 0), and is the left ventricular pressure in a fibrotic LV for different amounts of fibrosis. In these examples, we consider fib∈{0.1, 0.3, 0.5}.
Third, the support pressure displayed in Figure 2C and obeying the following equation is applied on exemplary fibrotic LVs, namely fib∈{0.3, 0.5}.
As mentioned in the Introduction, three possibilities for supporting a weakened heart can be distinguished: pure diastolic, pure systolic, and combined diastolic and systolic support. For the first possibility, we aim to regain EDP* and EDV* of the healthy control LV (within given tolerances tol1, tol2). For the second and third possibilities, we additionally aim to nearly reach ESV* of the healthy control LV. Note that EDP and EDV depend on the value of pmin only, i.e., EDP(pmin), EDV(pmin), while ESV depends on both values pmin and pmax, i.e., ESV(pmin, pmax). We started with the determination of the optimal negative support pressure during the diastole in Equation (9). In a second step, only systolic support is considered, i.e., pmin in Equation (9) is set to zero, and the maximal positive support pressure is determined. In the last step, a combination of diastolic and systolic support is assumed. Therefore, from the first step is fixed, and the optimal systolic support is to be found. We note that hold in general, as the end-diastolic states are different in both cases. For these three steps, the following algorithm is performed:
1. Diastolic support: Find so that and are fulfilled
2. Systolic support: Set pmin = 0 kPa and find so that is fulfilled
3. Diastolic and systolic support: Set and find so that is fulfilled
where the optimal support pressure p*(t) from Equation (9) is determined via the negative diastolic support pressure and positive systolic support pressures , for the purely systolic support and systolic support combined with diastolic support, respectively.
3. Results
Different quantities characterizing the cardiac performance are plotted for all simulations introduced in Section 2.2, including pressure volume loop, EF, EDV, ESV, EDP, and averaged end-diastolic hydrostatic stress over the domain, that is , where are the diagonal components of the Cauchy stress in the i-th finite element and nel = 22846 is the total number of the tetrahedral finite elements in the computational domain representing the LV; refer to Figure 2A. We note that EDP is the fluid pressure inside the cavity of the modeled LV, whereas the end-diastolic hydrostatic stress, computed according to the above formula, depends on the myocardial tissue structure and its volume change during the deformation (41). The latter can be interpreted as a measure of the force that drives fluid out of the myocardium and into the surrounding tissues. Positive hydrostatic stress means that the tissue is under extension and there is an increase in its volume (fluid flows into the myocardium), whereas negative hydrostatic stress implies that the myocardial tissue is compressed (fluid flows out of the myocardium).
3.1. Different Amounts of Fibrosis Without Support
Figure 3 shows the simulation results for scenarios with different amounts of fibrosis without any support pressure. Clearly, by increasing the amount of fibrosis, EF and EDV decrease, whereas ESV and EDP increase. For example, EF and EDP in the healthy model are 65.6% and 1 kPa (7.5 mmHg), respectively. These are close to the experimentally reported values in rats, which are slightly above the normal values in humans (35, 42, 43). By increasing the amount of fibrosis to 30% and 50%, EF reduces to 56.1% and 46.1%, whereas EDP increases to 1.6 kPa (12 mmHg) and 1.8 kPa (13.5 mmHg), respectively; refer to Figures 3B,E.
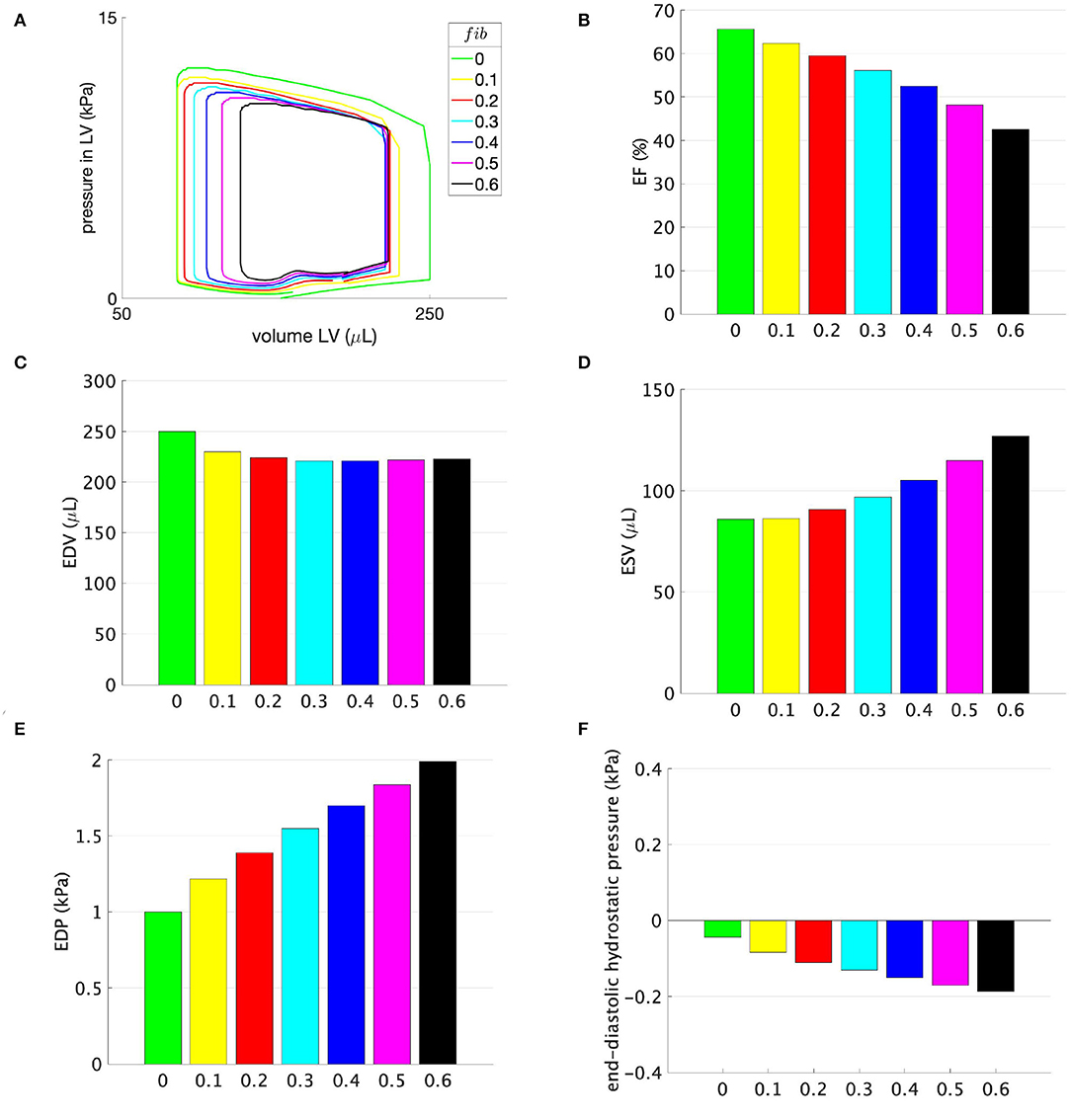
Figure 3. (A) Pressure-volume loops, (B) ejection fraction (EF), (C) EDV, (D) ESV, (E) EDP, and (F) end-diastolic hydrostatic stress for models with different amounts of fibrosis fib displayed on the horizontal axis. No support pressure is applied.
3.2. Support Pressure as the Difference With Respect to the Control Rat (fib = 0)
In Figure 4, changes in the cardiac function parameters are displayed for the rats with 10%, 30%, and 50% fibrosis in the LV. By applying a support pressure, computed according to Equation (8) and displayed in Figure 2B, EF, EDV, EDP, and end-diastolic hydrostatic pressure are at least partially improved, refer to Figures 4B,C,E,F, respectively. ESV remains nearly unchanged, refer to Figure 4D.
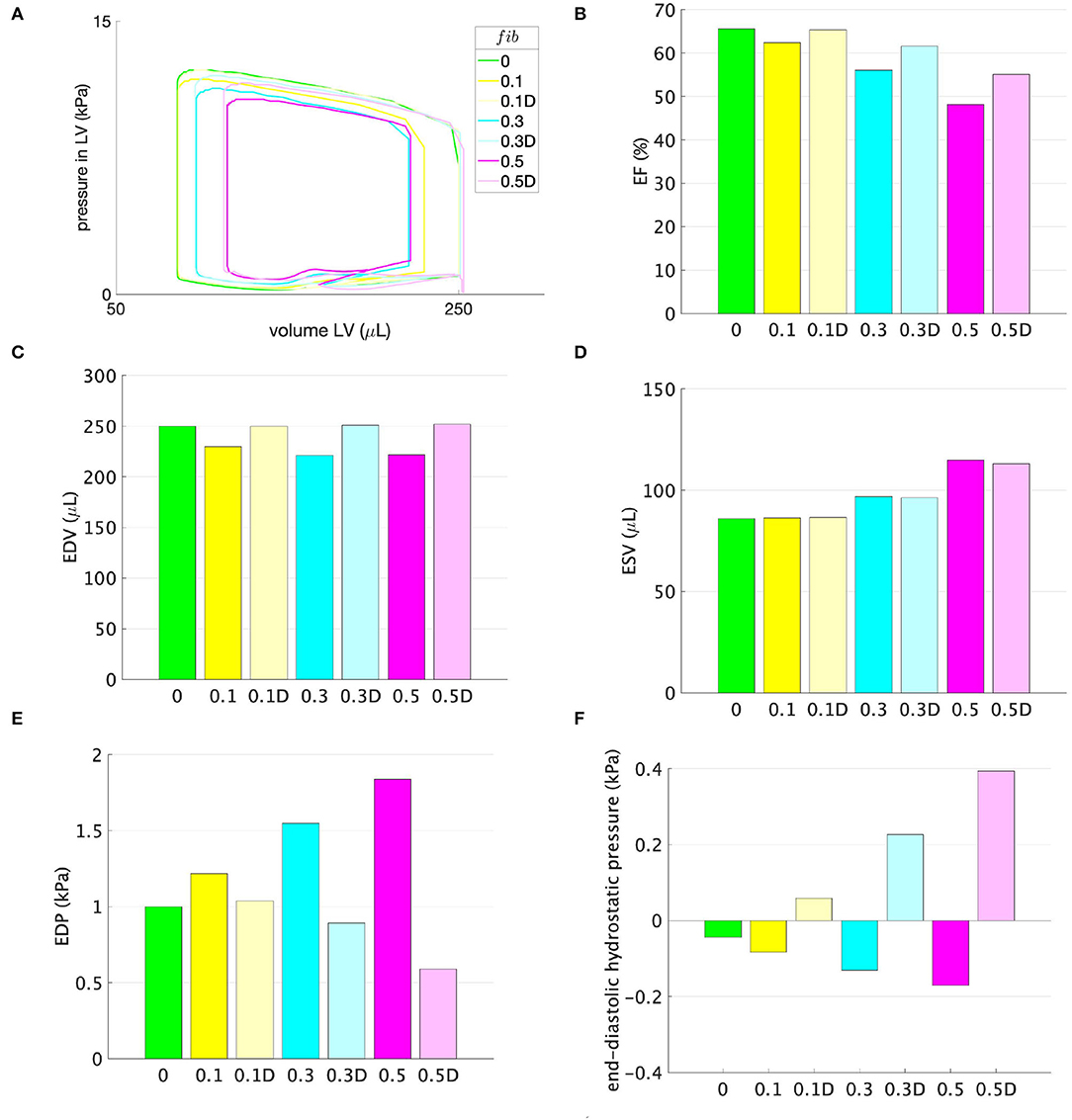
Figure 4. (A) Pressure-volume loops, (B) EF, (C) EDV, (D) ESV, (E) EDP, and (F) end-diastolic hydrostatic stress for models with different amounts of fibrosis fib displayed on the horizontal axis. No support pressure is applied for models marked with the corresponding amount of fibrosis fib and displayed in opaque colors, whereas support pressure according to the function shown in Figure 2B, is applied for models with the suffix D displayed in transparent colors.
3.3. Constant Minimal and Maximal Support Pressure
Figures 5, 6 illustrate how the stepwise increase in the negative diastolic and positive systolic support pressure influences cardiac performance. The support pressure is increased until the EDP, EDV, and ESV of the control rat are reached up to tolerance. The resulting optimal values of the support pressure are kPa (3.8 mmHg) and kPa (45 mmHg) for fib = 0.3 and kPa (4.5 mmHg), kPa (90 mmHg) for fib = 0.5. For example, for the modeled case with 50% fibrosis in comparison with the control LV, the EF can be increased by 5% with only diastolic support, increased by 14% with only systolic support, and completely reach the value of the modeled control LV (65.6%) with the combination of the diastolic and systolic support. The end-diastolic pressure of 1.8 kPa (13.5 mmHg) cannot be decreased with only systolic support and can completely reach the value of the modeled control LV (7.5 mmHg) with only diastolic support as well as with the combination of the diastolic and systolic support. By increasing the value of the diastolic support, the end-diastolic hydrostatic stress becomes positive. This means that the myocardial tissue is extended and volumetric increase (possibly via a fluid inflow) is present.
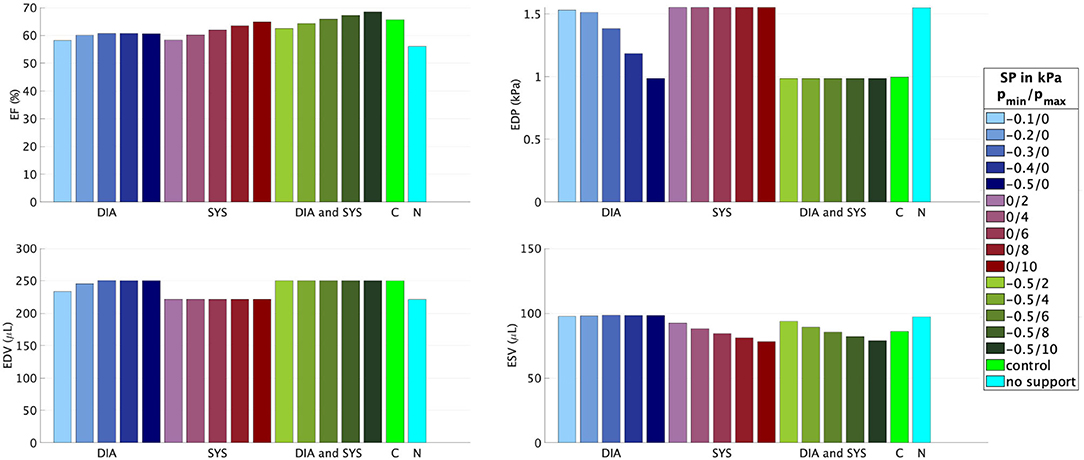
Figure 5. Ejection fraction, EDP, EDV, and ESV for different combinations of the minimal pmin and maximal pmax values of the support pressure (SP) applied to the epicardial surface of the model with fib = 0.3. DIA, diastolic support (blue); SYS, systolic support (red); DIA and SYS, combined support (green); C, control rat LV (light green); N, rat LV with fib = 0.3 without any support (blue-green).
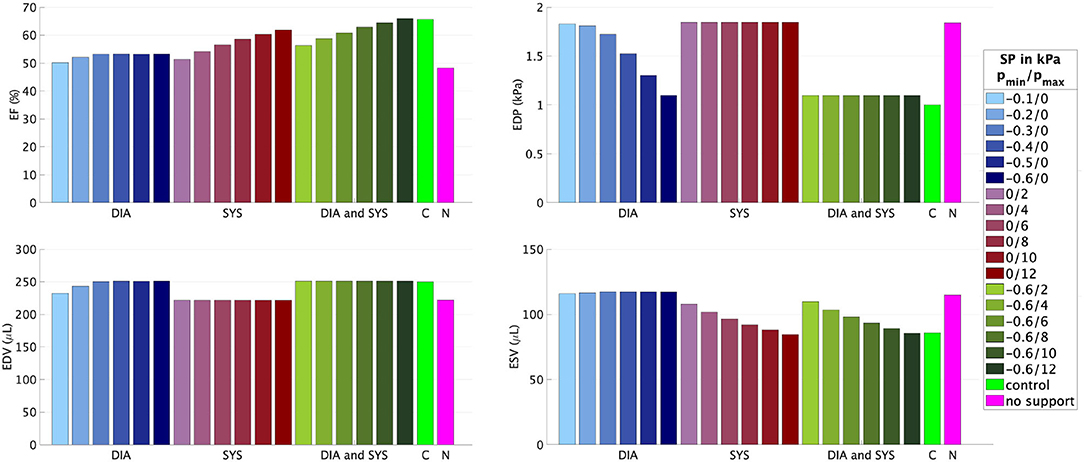
Figure 6. Ejection fraction, EDP, EDV, and ESV for different combinations of the minimal pmin and maximal pmax values of the support pressure (SP) applied to the epicardial surface of the model with fib = 0.5. DIA, diastolic support (blue); SYS, systolic support (red); DIA and SYS, combined support (green); C, control rat LV (light green); N, rat LV with fib = 0.5 without any support (pink).
4. Discussion
The simulation results show that, with an increased amount of fibrosis, the cardiac performance is reduced; specifically, a reduction in EF is accompanied by increases in ESV and EDP, as shown in Figures 3B,D,E. These results mirror the experimental studies in rats after myocardial infarction, where the infarct size was determined as the percentage of the fibrotic scar in the LV (21, 40). As depicted in Figure 3C, for an amount of fibrosis of 20%, EDV decreases by 11%. With a further increase in the amount of fibrosis, EDV remains nearly constant due to the combination of the stiffer myocardium and a higher EDP, as shown in Figure 3E.
It is worth noting that the alterations in EDP and EDV are caused solely by changes in the passive material properties, whereas the value of ESV is influenced by both the change in the stiffness as well as the reduced maximum active tension, i.e., contractility, which is in Equation (5) scaled by the amount of fibrosis. The absolute value of the hydrostatic stress depicted in Figure 3F increases nearly linearly with the amount of fibrosis. Its negative value represents a mechanical compression in the cardiac tissue modeled as a continuum. Theoretically, a high negative hydrostatic stress together with a high EDP might lead to compression and the closure of arterioles supplying the heart and then eventually to an under-perfusion. A more elaborate computational model accounting for the heart perfusion is needed to interpret the results quantitatively.
Considering the support pressure resulting from the difference between the ventricular pressure in a healthy and diseased LV, Figure 4C shows that the support pressure is sufficient for reaching the control EDV. We note that the maximum possible EDV was limited to that of the healthy rat. Therefore, even if the negative support pressure is higher than necessary, a normalization of the EDV is accompanied by a reduction in the EDP as depicted in Figures 4C,E. Due to the reduction in the EDP and enlargement of the LV, the hydrostatic stress becomes positive for all three fibrosis stages. This means that the tissue is under tension and better perfusion is expected. However, the observed decrease in ESV (Figure 4D) is marginal and only sufficient for the case fib = 0.1. Therefore, higher systolic support pressure is needed in order to reduce the ESV and eventually increase the EF, which is significantly below that of the control rat for higher amounts of fibrosis. For example, the resulting EFs are 61.1%, 55.2% for fib = 0.3, 0.5, respectively, compared to the control rat with EF = 65.6%.
In the numerical test, that applied a constant minimum and maximum support pressure during the diastole and systole, respectively, the algorithm described in Section 2.2 is exemplarily performed for two fibrosis stages, namely fib = 0.3, 0.5. We started with supporting only the diastolic phase such that the negative value of the support pressure is increased until the desired EDV and EDP are reached up to a given tolerance. The EF can be increased by approximately 5% for both fibrosis stages, resulting in EF = 60.7%, 53.3% for fib = 0.3, 0.5, respectively. Even for the case with 50% fibrotic tissue, a relatively small support pressure of −0.6 kPa (−5.3 mmHg) is sufficient for regaining the desired EDV and EDP. Furthermore, as depicted in Figure 7, the compressive hydrostatic stress in the stiff fibrotic myocardium can be reduced and even changed into positive hydrostatic stress. We believe that this phenomenon could improve myocardial perfusion. However, as discussed above, this is currently only speculative and needs to be investigated. When only the systolic phase is supported, significantly higher positive support pressures are needed to normalize the systolic function, 6 kPa (45 mmHg) and 12 kPa (90 mmHg) for fib = 0.3, 0.5, respectively. Nevertheless, in this case, the desired EF of the control rat (65.6%) is not reached due to insufficient diastolic filling caused by the stiff fibrotic tissue. When using only the systolic support, a further increase in the support pressure would theoretically lead to the desired EF. However, the EDP would remain elevated, which has been identified as a potential predictor of heart failure (44–46). Whether solely the reduction in EDP would eventually lower the risk needs to be investigated further. The best option for normalizing EF and EDP turns out to be a combination of both, diastolic and systolic support. As demonstrated in Figures 5, 6, complete normalization of the functional parameters can be reached.
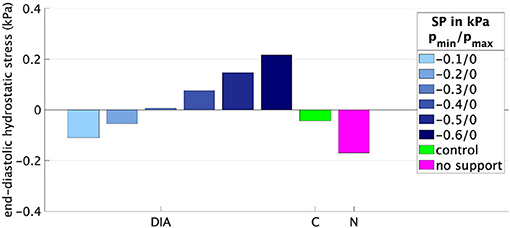
Figure 7. End-diastolic hydrostatic stress for different support pressures (SPs) applied to the rat LV with fib = 0.5. DIA, diastolic support; C, control rat LV; N, rat LV with fib = 0.5 without any support.
The present study aimed to explore the potential usefulness of diastolic support during the cardiac cycle of a rat LV suffering from restrictive cardiac disease. A simple computational model based on an investigation of mechanical support acting on the outer surface of the LV was chosen. However, there are some limitations and possibilities for future model development. First, the present study investigated the rat heart model and, to date, is not clinically applicable. However, as pointed out in the Introduction, some innovative approaches have investigated possible direct compression assist devices for clinical use in humans. Second, since it is difficult to develop a model that accounts for all influencing factors causing heart insufficiency, we initially chose a model in which we can change both the diastolic and the systolic function of a ventricle by changing one parameter, namely the amount of fibrosis. Besides this, various other factors must be considered in the future to more realistically mimic the remodeling process. These include the effects of geometric changes (in particular those due to ventricular dilatation or hypertrophy), the fact that most failing hearts are rather dilated than restrictive (especially in Paediatrics), the fact that myocardial infarction and ischaemic heart disease are only one among multiple causes of heart failure, the influence of arrhythmias and synchronization, the ever-changing metabolic needs, the fluid status of the patient impacting the preload of the heart, and much more. Third, for a better understanding of the interaction between the heart and a direct cardiac compression device, instead of the prescribed support pressure, a complete direct cardiac compression device should be modeled. Here, one possibility would be to use mechano-active materials, such as biocompatible dielectric elastomer actuators (47) that compress and expand when a voltage is applied. Their relatively large (more than 40%) expandability would be beneficial for generating the support pressure needed during the diastole (48). Fourth, in future development, electromechanical coupling and in particular electromechanical feedback would possibly play a role with respect to the interaction of the heart and the direct cardiac compression device.
5. Conclusion
A computational model for different amounts of fibrosis in the rat LV is presented. Based on this model, we investigate how a support pressure acting on the outer surface of the diseased LV influences the cardiac performance quantified via the EF, EDP, and ESV, as well as the hydrostatic stress in the cardiac tissue. We conclude that a negative support pressure during diastole combined with a positive support pressure during systole can normalize the modeled diastolic and systolic function of the rat LV at different fibrosis stages. Although not investigated in this study, it is tempting to assume that the negative diastolic support pressure could potentially improve cardiac perfusion. Furthermore, we adjusted the value of the support pressure so that functional parameters of the healthy rat LV are restored. We conclude that cardiac assist devices without direct blood contact and with a simultaneous diastolic and systolic support functionality present a potentially interesting therapy for heart failure in restrictive LV physiology, as that is resulting from myocardial scars after ischaemic insults.
Data Availability Statement
The raw data supporting the conclusions of this article will be made available by the authors, without undue reservation.
Author Contributions
DM mainly contributed to this study concerning the writing of the original manuscript, deriving the equations, modeling, simulation, and post-processing and visualization of the results. DH contributed to the fiber modeling and the revision of the manuscript. DB contributed to initial simulations and post-processing of simulation results. MW contributed to the acquisition of the publication fee support and advice for the medical part. SL and MA contributed to the conception and design of the study and the revision of the manuscript and provided the leadership responsibility for the technical and medical parts, respectively. MA contributed by writing and improving the medical parts of the manuscript. All authors contributed to the article and approved the submitted version.
Funding
This study was funded by the Klaus Tschira Stiftung Grant 00.289.2016. The authors acknowledge financial support by Deutsche Forschungsgemeinschaft and Friedrich-Alexander-Universität Erlangen-Nürnberg within the funding programme “Open Access Publication Funding”.
Conflict of Interest
The authors declare that the research was conducted in the absence of any commercial or financial relationships that could be construed as a potential conflict of interest.
Publisher's Note
All claims expressed in this article are solely those of the authors and do not necessarily represent those of their affiliated organizations, or those of the publisher, the editors and the reviewers. Any product that may be evaluated in this article, or claim that may be made by its manufacturer, is not guaranteed or endorsed by the publisher.
References
1. WHO. Global Health Estimates 2019: Disease Burden By Cause, Age, Sex, By Country And By Region, 2000-2019. (2020). Available online at: https://www.who.int/data/gho/data/themes/mortality-and-global-health-estimates (accessed May 25, 2022).
2. Eurotransplant. Annual Report 2020. (2021). Available online at: https://www.eurotransplant.org/statistics/annual-report/ (accessed May 25, 2022).
3. Han J, Trumble DR. Cardiac assist devices: early concepts, current technologies, and future innovations. Bioengineering. (2019) 6:18. doi: 10.3390/bioengineering6010018
4. Lima B, Bansal A, Abraham J, Rich JD, Lee SS, Soleimani B, et al. Controversies and challenges of ventricular assist device therapy. Am J Cardiol. (2018) 121:1219–24. doi: 10.1016/j.amjcard.2018.01.034
5. Lowe JE, Anstadt MP, Van Trigt P, Smith PK, Hendry PJ, Plunkett MD, et al. First successful bridge to cardiac transplantation using direct mechanical ventricular actuation. Ann Thorac Surg. (1991) 52:1237–45. doi: 10.1016/0003-4975(91)90007-D
6. Artrip JH, Yi GH, Levin HR, Burkhoff D, Wang J. Physiological and hemodynamic evaluation of nonuniform direct cardiac compression. Circulation. (1999) 100:II236–43. doi: 10.1161/01.CIR.100.suppl_2.II-236
7. Roche ET, Horvath MA, Wamala I, Alazmani A, Song SE, Whyte W, et al. Soft robotic sleeve supports heart function. Sci Transl Med. (2017) 9:eaaf3925. doi: 10.1126/scitranslmed.aaf3925
8. Jagschies L, Hirschvogel M, Matallo J, Maier A, Mild K, Brunner H, et al. Individualized biventricular epicardial augmentation technology in a drug-induced porcine failing heart model. ASAIO J. (2018) 64:480–8. doi: 10.1097/MAT.0000000000000686
9. Hord EC, Bolch CM, Tuzun E, Cohn WE, Leschinsky B, Criscione JC. Evaluation of the corinnova heart assist device in an acute heart failure model. J Cardiovasc Transl Res. (2019) 12:155–63. doi: 10.1007/s12265-018-9854-5
10. Oz MC, Artrip JH, Burkhoff D. Direct cardiac compression devices. J Heart Lung Transpl. (2002) 21:1049–55. doi: 10.1016/S1053-2498(02)00482-5
11. Bayer JD, Blake RC, Plank G, Trayanova NA. A novel rule-based algorithm for assigning myocardial fiber orientation to computational heart models. Ann Biomed Eng. (2012) 40:2243–54. doi: 10.1007/s10439-012-0593-5
12. Wong J, Kuhl E. Generating fibre orientation maps in human heart models using Poisson interpolation. Comput Methods Biomech Biomed Eng. (2014) 17:1217–26. doi: 10.1080/10255842.2012.739167
13. Holz D, Duong MT, Martonová D, Alkassar M, Dittrich S, Leyendecker S. Transmural path model improves definition of orthotropic tissue structure in heart simulation. J Biomech Eng. (2022) 144:031002. doi: 10.1115/1.4052219
14. Heusch G, Libby P, Gersh B, Yellon D, Böhm M, Lopaschuk G, et al. Lancet seminar: cardiovascular remodelling in coronary artery disease and heart failure. Lancet. (2014) 383:1933–43. doi: 10.1016/S0140-6736(14)60107-0
15. Liu T, Song D, Dong J, Zhu P, Liu J, Liu W, et al. Current understanding of the pathophysiology of myocardial fibrosis and its quantitative assessment in heart failure. Front Physiol. (2017) 8:238. doi: 10.3389/fphys.2017.00238
16. Humeres C, Frangogiannis NG. Fibroblasts in the infarcted, remodeling, and failing heart. JACC. Basic Transl Sci. (2019) 4:449–467. doi: 10.1016/j.jacbts.2019.02.006
17. Moreo A, Ambrosio G, De Chiara B, Pu M, Tran T, Mauri F, et al. Influence of myocardial fibrosis on left ventricular diastolic function: noninvasive assessment by cardiac magnetic resonance and echo. Circ Cardiovasc Imaging. (2009) 2:437–43. doi: 10.1161/CIRCIMAGING.108.838367
18. Zile MR, Baicu CF, S Ikonomidis J, Stroud RE, Nietert PJ, Bradshaw AD, et al. Myocardial stiffness in patients with heart failure and a preserved ejection fraction: contributions of collagen and titin. Circulation. (2015) 131:1247–59. doi: 10.1161/CIRCULATIONAHA.114.013215
19. Kane GC, Karon BL, Mahoney DW, Redfield MM, Roger VL, Burnett JC, et al. Progression of left ventricular diastolic dysfunction and risk of heart failure. JAMA. (2011) 306:856–63. doi: 10.1001/jama.2011.1201
20. Sweeney M, Corden B, Cook SA. Targeting cardiac fibrosis in heart failure with preserved ejection fraction: mirage or miracle? EMBO Mol Med. (2020) 12:e10865. doi: 10.15252/emmm.201910865
21. Fletcher PJ, Pfeffer JM, Pfeffer MA, Braunwald E. Left ventricular diastolic pressure-volume relations in rats with healed myocardial infarction. Effects on systolic function. Circ Res. (1981) 49:618–26. doi: 10.1161/01.RES.49.3.618
22. Fomovsky GM, Holmes JW. Evolution of scar structure, mechanics, and ventricular function after myocardial infarction in the rat. Am J Physiol Heart Circ Physiol. (2010) 298:H221–8. doi: 10.1152/ajpheart.00495.2009
23. Martonová D, Alkassar M, Seufert J, Holz D, Duong MT, Reischl B, et al. Passive mechanical properties in healthy and infarcted rat left ventricle characterised via a mixture model. J Mech Behav Biomed Mater. (2021) 119:104430. doi: 10.1016/j.jmbbm.2021.104430
24. Gupta KB, Ratcliffe MB, Fallert MA, Edmunds LH, Bogen DK. Changes in passive mechanical stiffness of myocardial tissue with aneurysm formation. Circulation. (1994) 89:2315–26. doi: 10.1161/01.CIR.89.5.2315
25. Morita M, Eckert CE, Matsuzaki K, Noma M, Ryan LP, Burdick JA, et al. Modification of infarct material properties limits adverse ventricular remodeling. Ann Thorac Surg. (2011) 92:617–24. doi: 10.1016/j.athoracsur.2011.04.051
26. McCormick M, Nordsletten DA, Kay D, Smith NP. Simulating left ventricular fluid-solid mechanics through the cardiac cycle under LVAD support. J Comput Phys. (2013) 244:80–96. doi: 10.1016/j.jcp.2012.08.008
27. Ahmad Bakir A, Al Abed A, Stevens MC, Lovell NH, Dokos S. A multiphysics biventricular cardiac model: simulations with a left-ventricular assist device. Front Physiol. (2018) 9:1259. doi: 10.3389/fphys.2018.01259
28. Hirschvogel M, Jagschies L, Maier A, Wildhirt SM, Gee MW. An in silico twin for epicardial augmentation of the failing heart. IInt J Numer Method Biomed Eng. (2019) 35:1–23. doi: 10.1002/cnm.3233
29. Chavanne JAJM. Cylindrical dielectric elastomer actuator for cardiac assist device. Ph.D. thesis. Lausanne: Integrated Actuators Laboratory (2019).
30. Martonová D, Alkassar M, Seufert J, Holz D, Tuan Duong M, Reischl B, et al. Influence of passive mechanical properties in healthy and infarcted rat myocardium on the cardiac cycle. PAMM. (2021) 21:e202100054. doi: 10.1002/pamm.202100054
31. Eriksson TSE, Prassl AJ, Plank G, Holzapfel GA. Modeling the dispersion in electromechanically coupled myocardium. Int J Num Methods Biomed Eng. (2013) 29:1267–84. doi: 10.1002/cnm.2575
32. Göktepe S, Kuhl E. Electromechanics of the heart: a unified approach to the strongly coupled excitation–contraction problem. Comput Mech. (2010) 45:227–43. doi: 10.1007/s00466-009-0434-z
33. Sack KL, Dabiri Y, Franz T, Solomon SD, Burkhoff D, Guccione JM. Investigating the role of interventricular interdependence in development of right heart dysfunction during LVAD support: a patient-specific methods-based approach. Front Physiol. (2018) 9:524. doi: 10.3389/fphys.2018.00520
34. Duong MT, Holz D, Alkassar M, Dittrich S, Leyendecker S. Interaction of the mechano-electrical feedback with passive mechanical models on a 3D rat left ventricle: a computational study. Front Physiol. (2019) 10:1041. doi: 10.3389/fphys.2019.01041
35. Martonová D, Holz D, Seufert J, Duong MT, Alkassar M, Leyendecker S. Comparison of stress and stress-strain approaches for the active contraction in a rat cardiac cycle model. J Biomech. (2022) 134:110980. doi: 10.1016/j.jbiomech.2022.110980
36. Berberog~lu E, Göktepe S. Computational modeling of myocardial infarction. Proc IUTAM. (2015) 12:52–61. doi: 10.1016/j.piutam.2014.12.007
37. Holzapfel GA, Ogden RW. Constitutive modelling of passive myocardium: a structurally based framework for material characterization. Philos Trans R Soc A Math Phys Eng Sci. (2009) 367:3445–75. doi: 10.1098/rsta.2009.0091
38. Pfaller MR, Hörmann JM, Weigl M, Nagler A, Chabiniok R, Bertoglio C, et al. The importance of the pericardium for cardiac biomechanics: from physiology to computational modeling. Biomech Model Mechanobiol. (2019) 18:503–29. doi: 10.1007/s10237-018-1098-4
39. Lin DHS, Yin FCP. A multiaxial constitutive law for mammalian left ventricular myocardium in steady-state barium contracture or tetanus. J Biomech Eng. (1998) 120:504–17. doi: 10.1115/1.2798021
40. Pfeffer MA, Pfeffer JM, Fishbein MC, Fletcher PJ, Spadaro J, Kloner RA, et al. Myocardial infarct size and ventricular function in rats. Circ Res. (1979) 44:503–12. doi: 10.1161/01.RES.44.4.503
42. Pacher P, Mabley JG, Liaudet L, Evgenov OV, Marton A, Haskó G, et al. Left ventricular pressure-volume relationship in a rat model of advanced aging-associated heart failure. Am J Physiol Heart Circ Physiol. (2004) 287:H2132–7. doi: 10.1152/ajpheart.00405.2004
43. Jegger D, Jeanrenaud X, Nasratullah M, Chassot PG, Mallik A, Tevaearai H, et al. Noninvasive Doppler-derived myocardial performance index in rats with myocardial infarction: validation and correlation by conductance catheter. Am J Physiol Heart Circ Physiol. (2006) 290:H1540–8. doi: 10.1152/ajpheart.00935.2005
44. Mielniczuk LM, Lamas GA, Flaker GC, Mitchell G, Smith SC, Gersh BJ, et al. Left ventricular end-diastolic pressure and risk of subsequent heart failure in patients following an acute myocardial infarction. Congest Heart Fail. (2007) 13:209–14. doi: 10.1111/j.1527-5299.2007.06624.x
45. Planer D, Mehran R, Witzenbichler B, Guagliumi G, Peruga JZ, Brodie BR, et al. Prognostic utility of left ventricular end-diastolic pressure in patients with ST-segment elevation myocardial infarction undergoing primary percutaneous coronary intervention. Am J Cardiol. (2011) 108:1068–74. doi: 10.1016/j.amjcard.2011.06.007
46. Khan AA, Al-Omary MS, Collins NJ, Attia J, Boyle AJ. Natural history and prognostic implications of left ventricular end-diastolic pressure in reperfused ST-segment elevation myocardial infarction: An analysis of the thrombolysis in myocardial infarction (TIMI) II randomized controlled trial. BMC Cardiovasc Disord. (2021) 21:243. doi: 10.1186/s12872-021-02046-x
47. Ponniah JK, Chen H, Adetiba O, Verduzco R, Jacot JG. Mechanoactive materials in cardiac science. J Mater Chem B. (2016) 4:7350–62. doi: 10.1039/C6TB00069J
48. Alkassar M. Circulatory Assistance Device. European patent No. EP3503938 B1. Munich, Germany. European Patent Office (2021).
Appendix
Simulation Parameters
Active Tension
Based on the study by Pfaller et al. (38), the evolution of the active tension T reads as
with activation function a, the maximum value of the active stress T, and the function |a(t)|+ = max(a(t);0). The activation function a(t) is modeled by
with maximum and minimum activation rates αmax and αmin, respectively, and functions
with steepness γ and descending and ascending sigmoid functions S+ and S−, respectively. The indicator function f∈(0, 1) indicates systole. The times ted and tps model the end-diastolic and the peak-systolic times, respectively.
Keywords: cardiac assist device, cardiomyopathy, epicardial heart support, left ventricle, support pressure, fibrosis
Citation: Martonová D, Holz D, Brackenhammer D, Weyand M, Leyendecker S and Alkassar M (2022) Support Pressure Acting on the Epicardial Surface of a Rat Left Ventricle—A Computational Study. Front. Cardiovasc. Med. 9:850274. doi: 10.3389/fcvm.2022.850274
Received: 07 January 2022; Accepted: 31 May 2022;
Published: 06 July 2022.
Edited by:
Sebastiano A. G. Lava, Centre Hospitalier Universitaire Vaudois (CHUV), SwitzerlandReviewed by:
Ender Ödemiş, Koç University Hospital, TurkeyMichał Ma̧czewski, Medical Centre for Postgraduate Education, Poland
Copyright © 2022 Martonová, Holz, Brackenhammer, Weyand, Leyendecker and Alkassar. This is an open-access article distributed under the terms of the Creative Commons Attribution License (CC BY). The use, distribution or reproduction in other forums is permitted, provided the original author(s) and the copyright owner(s) are credited and that the original publication in this journal is cited, in accordance with accepted academic practice. No use, distribution or reproduction is permitted which does not comply with these terms.
*Correspondence: Denisa Martonová, ZGVuaXNhLm1hcnRvbm92YUBmYXUuZGU=