Commentary: “Multimodality advanced cardiovascular and molecular imaging for early detection and monitoring of cancer therapy-associated cardiotoxicity and the role of artificial intelligence and big data”
- 1Section of Cardiovascular Medicine, Yale University School of Medicine, New Haven, CT, United States
- 2Geisel School of Medicine at Dartmouth, Hanover, NH, United States
- 3Department of Radiology and Biomedical Imaging, Yale University School of Medicine, New Haven, CT, United States
- 4Department of Biomedical Engineering, Yale University, New Haven, CT, United States
Cancer mortality has improved due to earlier detection via screening, as well as due to novel cancer therapies such as tyrosine kinase inhibitors and immune checkpoint inhibitions. However, similarly to older cancer therapies such as anthracyclines, these therapies have also been documented to cause cardiotoxic events including cardiomyopathy, myocardial infarction, myocarditis, arrhythmia, hypertension, and thrombosis. Imaging modalities such as echocardiography and magnetic resonance imaging (MRI) are critical in monitoring and evaluating for cardiotoxicity from these treatments, as well as in providing information for the assessment of function and wall motion abnormalities. MRI also allows for additional tissue characterization using T1, T2, extracellular volume (ECV), and delayed gadolinium enhancement (DGE) assessment. Furthermore, emerging technologies may be able to assist with these efforts. Nuclear imaging using targeted radiotracers, some of which are already clinically used, may have more specificity and help provide information on the mechanisms of cardiotoxicity, including in anthracycline mediated cardiomyopathy and checkpoint inhibitor myocarditis. Hyperpolarized MRI may be used to evaluate the effects of oncologic therapy on cardiac metabolism. Lastly, artificial intelligence and big data of imaging modalities may help predict and detect early signs of cardiotoxicity and response to cardioprotective medications as well as provide insights on the added value of molecular imaging and correlations with cardiovascular outcomes. In this review, the current imaging modalities used to assess for cardiotoxicity from cancer treatments are discussed, in addition to ongoing research on targeted molecular radiotracers, hyperpolarized MRI, as well as the role of artificial intelligence (AI) and big data in imaging that would help improve the detection and prognostication of cancer-treatment cardiotoxicity.
Introduction
Cancer incidence is expected to increase by 50% by 2050, but over the past two decades, cancer mortality has improved in part due to earlier detection via screening and the advent of novel therapies such as tyrosine kinase inhibitors (TKI) for cancers like chronic myelogenous leukemia (CML), liver, gastrointestinal and lung cancers, as well as immunotherapy, such as checkpoint inhibitors, for metastatic disease and an expanding list of indications including triple negative breast cancer, lung cancer, melanoma, bladder cancer, and renal cell cancer (1–6).
However, with the rise of newer oncologic therapies, there have been a spectrum of adverse cardiovascular toxicities including cardiomyopathy (CM), myocardial infarction, myocarditis, arrhythmia, hypertension (HTN) and thrombosis that have been associated with these agents. More traditional cardiotoxic agents like anthracyclines (i.e., doxorubicin), one of the most widely used class of chemotherapeutics due to improved overall cancer and survival outcomes has been shown to alter myocardial energetics, promote mitochondrial dysfunction, increase reactive oxygen species levels leading to activation of matrix metalloproteases, inhibit topoisomerase IIb and cause DNA strand breaks, thereby promoting cardiomyopathy (7–9).
HER2 inhibitors like trastuzumab has also been shown to increase risk of CM via antagonizing important pro survival as well as other important signal transduction pathways for metabolism in the heart (10). Platinum agents like cisplatin have been shown to increase oxidative stress and increased apoptosis and has been associated with cardiomyopathy in rare instances (11). Alkylating agents like cyclophosphamide, which can cause oxidative damage and direct endothelial cell damage have been linked to myocarditis and cardiomyopathy (12). Antimetabolites like 5 fluorouracil (5FU), which is commonly used in head and neck cancers as well as gastrointestinal cancers has been shown to increase risk of coronary vasospasm and myocardial infarction (13, 14). Multiple myeloma therapies (bortezomib, lenalidomide) and vascular endothelial growth factor (VEGF) inhibitors like bevacizumab have been associated with thrombosis and hypertension by promoting endothelial cell dysfunction (15–18). TKIs like ibrutinib has been associated with atrial fibrillation, while other TKIs such as ponatinib, sorafenib, sunitinib have been associated with CM and myocardial infarction (MI) (19–21).
Of the close to 2 million patients diagnosed with cancer in 2019, it is estimated that 38.5% are eligible for ICI therapy (22, 23). In addition to increased risk of myocarditis, pericarditis and vasculitis, immune checkpoint inhibitors (ICI) have been associated with increased risk of plaque rupture/acceleration of atherosclerosis and thrombosis (24). ICI myocarditis is characterized by lymphocytic infiltration with CD4 and CD8 cells and mortality is high if not identified and if left untreated (25).
Newer immunotherapies may also increase risk of myocarditis, such as cellular therapies like CART and molecular inhibitors such as CCR4 antagonist, mogamulizumab, which is used to treat T cell lymphomas (26–28). However, evaluation of the earliest signs of immune cell infiltration in the myocarditis process is limited (Table 1; Figure 1). Imaging modalities like echocardiography (echo) and magnetic resonance imaging (MRI) are routinely used to monitor and evaluate for the aforementioned oncologic therapy related cardiotoxicity, with both allowing for assessment of function and wall motion abnormalities and MRI allowing for additional tissue characterization using T1, T2, extracellular volume (ECV) and delayed gadolinium enhancement (DGE) assessment. While nuclear studies like multi-gated acquisition (MUGA) scans have fallen out of favor for the evaluation of cardiomyopathy mediated by oncologic therapy due to the higher sensitivity, and availability of echo and MRI, emerging nuclear imaging using molecularly targeted radiotracers may confer more specificity and help elucidate the mechanisms of cardiotoxicity, many of which are already in clinical use for oncology purposes and thus can be adapted to evaluate their signal/role in cardiotoxicity (Table 1). In addition to molecular targets, hyperpolarized MRI has emerged as a potential imaging modality to evaluate effects of oncologic therapy on cardiac metabolism and has reached human studies. Finally, artificial intelligence and big data of imaging modalities including electrocardiograms may be able to help predict and detect early signs of cardiotoxicity and response to cardioprotective medications once cardiomyopathy develops but also help provide insights on diagnostic and prognostic value of molecular based imaging. We review current imaging modalities used to assess for cardiovascular toxicities associated with oncologic therapies and highlight ongoing research in the areas of molecular imaging, targeted molecular radiotracers and hyperpolarized MRI as well as the role of artificial intelligence (AI) and big data in imaging that would help improve detection, prognostication of oncologic therapy related cardiotoxicity.
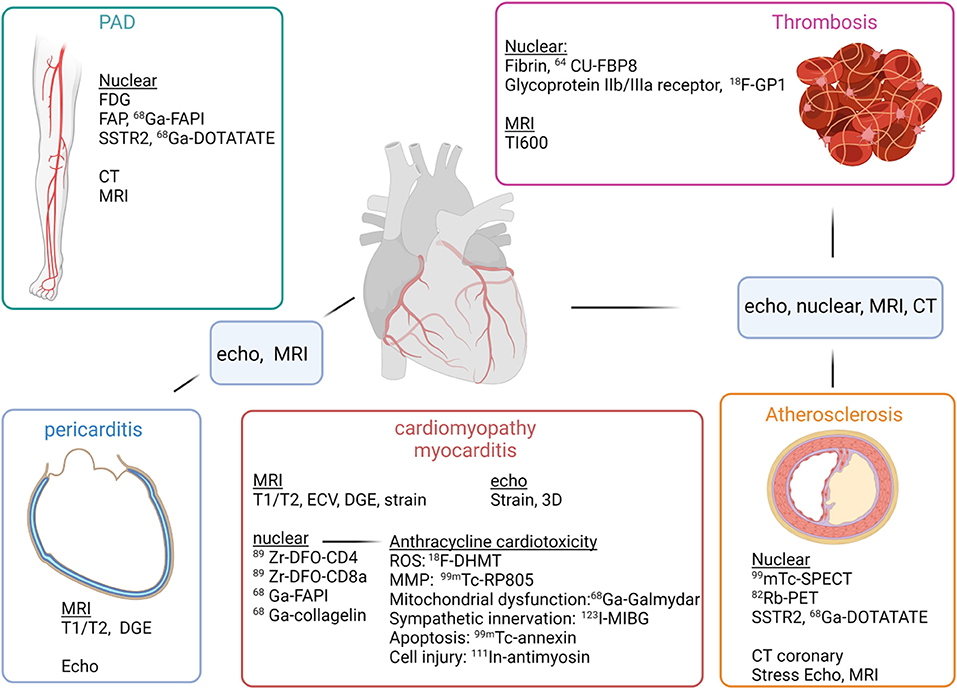
Figure 1. Imaging modalities and evaluation of cardiotoxicities of oncologic therapies. For evaluation of peripheral artery disease (PAD) (top left), FDG, FAP and SSTR2 imaging may be able to identify vulnerable plaque, while CT and MRI can help evaluate degree of stenosis. For evaluation of thrombosis (top right), nuclear imaging may be able to identify early clot formation with radiotracers directed at fibrin or glycoprotine IIb/IIIa, and MRI can use a long inversion time to identify thrombus, as with TI600. For evaluation of cardiomyopathy/myocarditis (middle), echo and MRI can evaluate ejection fraction as well as myocardial strain. For myocarditis, MRI can evaluate tissue characteristics such as T1, T2 and DGE, which are now components of the Lake Louise criteria for myocarditis. Nuclear can evaluate for T cell infiltration using tracers targeting CD4, CD8 cells. Tracers directed against FAP, such as 68Ga-FAPI has been shown to be increased in an animal model of checkpoint inhibitor myocarditis. Evaluation of pericarditis (bottom left), a complication of checkpoint inhibitors can be assessed by echo for detection of pericardial effusion, but with greater specificity MRI can identify edema and DGE. Atherosclerosis (bottom right) can be evaluated by traditional SPECT and PET techniques to evaluate for perfusion with stress and rest. CT coronary is now first line for evaluation of those with intermediate risk chest pain to rule out obstructive disease. Stress MRI or DGE can also be performed to evaluate for prior myocardial infarction as well as myocardial viability.
Current Imaging Modalities Used to Interrogate Oncologic Therapy Cardiotoxicity
Echo and MRI in Evaluation of Cardiotoxicity
Cardiotoxicity due to anthracycline use (often dose dependent, but can occur at any dose) are common, up to 5% with cumulative doses <400 mg/kg, but up to 20% for those treated with 700 mg/kg or more (72). HER2 inhibitor mediated cardiomyopathy can occur in 5–10% of patients and is increased when given in conjunction with anthracyclines up to 27% (73, 74). Oncologic therapy mediated cardiomyopathy can be evaluated by traditional imaging modalities such as echo and MRI, which are able to evaluate wall motion, left and right ventricular function and even early signs of toxicity via changes in strain, namely global longitudinal strain (75, 76). The European Society for Medical Oncology (ESMO) and the American Society of Echo (ASE) recommend 2D/3D echo or MRI for assessing left ventricular function including strain for monitoring of known cardiotoxic therapies such as anthracyclines or anti-Her2 therapies and the American Society of Clinical Oncology (ASCO) recommends echo or MRI as first line imaging modalities with MUGA as a second line if echo/MRI are not available or if not technically feasible for MRI (77–81). Due to reduced variability compared to 2D echo, 3D echo or MRI are recommended for sequential follow up (82).
In addition to being the gold standard for volumetrics and ejection fraction, MRI has additional evaluation capabilities including tissue characterization for injured cells such as changes in ECV and increased native T1 times, shown with anthracycline use and increased T2 relaxation times with anthracycline toxicity (83–86). The presence of DGE post trastuzumab, a HER2 inhibitor, was associated with cardiomyopathy (87).
Strain as a Predictor of Cardiomyopathy
Feature tracking global longitudinal strain (GLS) was first used in echo to show that it could be predictive of future cardiomyopathy in multiple studies of cancer patients undergoing cardiotoxic chemotherapy with anthracycline or trastuzumab. For example, an increase in GLS >12 or 15% was associated with a significant drop in LVEF >10% 6 months after in several studies (88, 89). MRI has subsequently shown that use of tagging, feature tracking strain or fast strain encoded (SENC) assessment are sensitive and highly accurate in detecting subclinical cardiotoxicity as evidenced by an increase in GLS for patients on cardiotoxic chemotherapy such as anthracyclines, with SENC having a higher accuracy that was less dependent on loading conditions (90–94). However, strain assessment in MRI is largely used in a research setting and is not routinely used in the clinical practice yet.
MRI Evaluation of Adverse Immune Related Cardiac Events
ICI myocarditis can occur in 1–2% of patients and has a high mortality of up to 50% if untreated (25, 95). MRI has become a work horse for evaluation of immunotherapy related cardiotoxicities. In addition to T1, and ECV changes, T2 abnormalities allow for assessment of myocardial edema in patients on checkpoint inhibitors with concern for myocarditis or pericarditis and DGE, a marker of myocardial injury or scarring is another tissue characterization parameter that can evaluate for immunotherapy toxicities. MRI is recommended by specialty society guidelines as part of the evaluation and monitoring of ICI myocarditis using the Lake Louise criteria, updated in 2018 to require both increased myocardial signal intensity ratio >2 or increased myocardial relaxation times or visible myocardial edema in T2-weighted images and increased myocardial relaxation times or extracellular volume fraction or DGE in T1-weighted images for the imaging diagnosis of myocarditis (80, 96–100). However, DGE is non-specific and cannot distinguish from cell damage vs. end stage fibrosis and current standard clinical imaging modalities are lacking in assessment of potential molecular correlates, such as collagen deposition and scar. Thus, molecularly targeted imaging tracers may shed light on both mechanism and help increase the specificity of cardiac imaging findings.
Molecular Targeted Nuclear Imaging Modalities to Evaluate Oncologic Therapy Related Adverse Cardiovascular Pathologies
Molecular Nuclear Imaging for Evaluation of Anthracycline Cardiotoxicity
Anthracycline mediated cardiotoxicity has been associated with an increase in reactive oxygen species (ROS) levels in the heart. ROS levels have been shown to confer cardiotoxicity by increased apoptosis, inflammation, mitochondrial dysfunction and activation of matrix metalloproteases (31). Molecular nuclear imaging studies have helped shed light on mechanisms of anthracycline mediated cardiotoxicity. Increased ROS levels in an animal model of doxorubicin cardiotoxicity showed that a novel PET tracer, 18F-labeled radioanalog of dihydroethidium, [18F]-6-(4-((1-(2-fluoroethyl)-1H-1,2,3-triazol-4-yl)methoxy)phenyl)-5-methyl-5,6 dihydrophenanthridine-3, 8-diamine ([18F]·DHMT), which targets superoxide, was able to reveal an elevation in superoxide levels in the heart at least 2 weeks prior to a drop in the left ventricular ejection fraction (35). ROS activation of MMPs downstream can then promote adverse cardiac remodeling (101). Renin-angiotensin-aldosterone system (RAAS) activation has been shown to augment the progression of anthracycline induced cardiotoxicity and inhibition via RAAS inhibitors like angiotensin receptor blockers or angiotensin converting enzyme inhibitors have been able to prevent and treat anthracycline mediated cardiomyopathy (102, 103). Use of a novel angiotensin receptor-neprilysin Inhibitor, sacubitril/valsartan in a rodent model of anthracycline cardiotoxicity was able to attenuate cardiotoxicity. MMP imaging of activated MMPs using SPECT radiotracer 99mTc-RP805 showed that sacubitril/valsartan in conjunction with doxorubicin was able to significantly attenuate MMP activation as well as prevent a decline in LVEF compared to doxorubicin alone vs. doxorubicin and valsartan groups. Myocardial MMP activity as assessed by 99mTc-RP805 uptake was inversely related to left ventricular ejection fraction (31). In addition to MMP activation and adverse remodeling, ROS can also injure endothelial cells. Anthracycline use has been associated with capillary loss in the heart in some rodent models and protection of endothelial cells with vascular endothelial growth factor-B (VEGF-B) treatment led to preservation of capillary mass (104).
ROS has also been shown to confer mitochondrial dysfunction. Disruption of mitochondrial membrane potential in mitochondrial dysfunction mediated by anthracycline can be evaluated by 68Ga-Galmydar. In a rodent model, uptake of 68Ga-Galmydar was reduced by 2-fold with anthracycline treatment compared to control and in H9c2 rat cardiomyoblasts, this was associated with activation of the apoptosis cascade (36).
Early markers of anthracycline cardiotoxicity include an increased uptake of indium-111-labeled antimyosin in the heart, which occurs due to myocyte damage and subsequent association of antimyosin with myosin, which is normally intracellular. Increased uptake of 111In-antimyosin in patients on anthracycline was associated with LV dysfunction (30). Detection of the earliest stages of apoptosis can also signal early toxicity. Annexin V has a high affinity for phosphatidylserine, which gets exposed on the cell surface during apoptosis. Use of annexin V imaging has allowed for detection of cells undergoing apoptosis. In a rodent model of doxorubicin cardiotoxicity, radiolabeled annexin V, 99mTc-annexin was used to visualize apoptosis that corresponded to histological evidence of apoptosis on TUNEL staining (33). Finally, sympathetic nervous innervation of the myocardium has also been shown to be disrupted with anthracycline toxicity. An assessment of myocardial sympathetic innervation impairment was done by evaluating a radiotracer that is an analog of norepinephrine, iodine-123-labeled meta-iodobenzylguanidine (123I-MIBG). A decrease in 123I-MIBG uptake with increasing cumulative doses of anthracyclines in human patients was associated with LV dysfunction. However, it takes higher cumulative doses of anthracycline to see a drop in 123I-MIBG uptake, thus this agent would be less useful if earlier detection of toxicity is desired. However, 123I-MIBG is clinically available and routinely used to evaluate for adrenaline secreting tumors (30) (Figure 2).
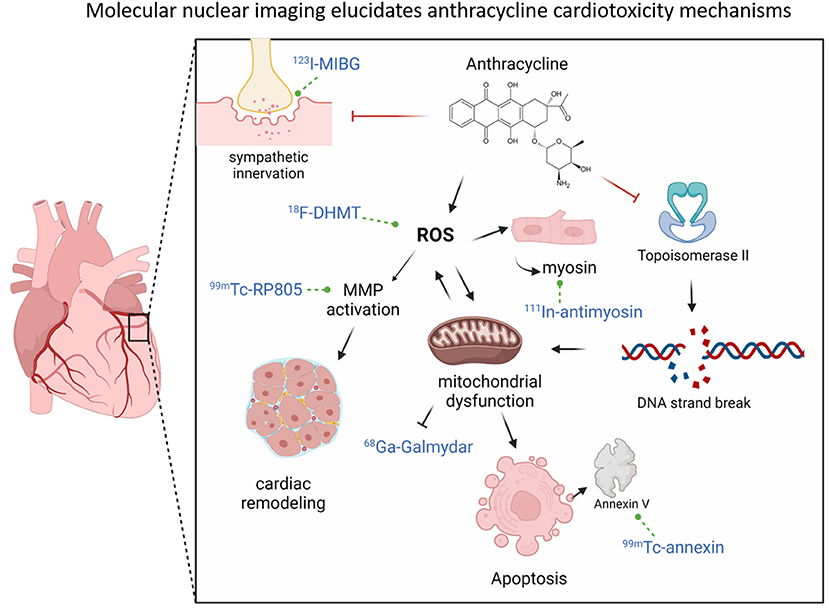
Figure 2. Molecular nuclear imaging elucidates anthracycline cardiotoxicity mechanisms. Anthracyclines can increase ROS levels (which can be assessed by nuclear tracer 18F-DHMT), which can activate MMPs (which can be assessed by 99mTc-RP805) (bottom left), leading to adverse cardiac remodeling. ROS levels can also promote mitochondrial dysfunction, which can disrupt the mitochondrial membrane potential and thereby reduce 68Ga-Galmydar uptake (middle bottom). Mitochondrial damage can lead to apoptosis, which can be detected by Annexin V positivity (detected by 99mTc-Annexin (bottom right). Damage to cardiomyocytes can lead to release of intracellular myosin, which can thereby be assessed by (105). In-myosin (right of ROS). In addition to ROS increase, anthracyclines can also directly bind and inhibit Topoisomerase II, which can lead to double-stranded DNA breaks (right) and cause further mitochondrial dysfunction and prevent mitochondrial regeneration. Finally, anthracyclines can lead to impaired sympathetic innervation over time for mechanisms that are unclear but is associated with cardiac dysfunction and this can be assessed by 123I-MIBG uptake (top left).
CD4, CD8 Imaging in ICI Myocarditis
Molecularly targeted radiotracers in nuclear medicine are emerging to evaluate processes such as fibrosis, inflammation and thrombosis, extending beyond nuclear cardiology's traditional use to evaluate perfusion deficits in ischemic heart disease via single photon emission computed tomography (SPECT) and positron emission tomography (PET), tissue viability or inflammation with PET fluorodeoxyglucose (FDG), which evaluates for glucose uptake predominantly by inflammatory cells, such as myeloid and T cells (106). These processes are common adverse effects of oncologic and immunotherapies.
Detection of the earliest signs of myocardial inflammation in ICI myocarditis, which occurs in 1-2% of patients on these agents remains a clinical challenge (95, 107). The ability to detect the initial infiltration of inflammatory cells such as CD4 or CD8 cells before injury has occurred could help reduce morbidity and high mortality associated with this condition (25). Emerging molecularly targeted probes against CD4, 89Zr-DFO-CD4 and CD8 cells, 89Zr-DFO-CD8a may be a potential avenue to detect inflammation at these earliest of stages, which can prompt more frequent follow ups, biomarker checking and earlier therapy (44). Determining specificity of these findings will also be important as to avoid withholding cancer fighting immunotherapy or treatment with steroids, which may potentially lower the efficacy of the immunotherapy agent (108–110). Checkpoint inhibitors have been shown to accelerate atherosclerosis and increase risk of plaque rupture in addition to the risk for myocarditis and pericarditis by driving increased inflammatory cells, including CD8 T cell infiltration into plaques in animal models and patients on checkpoint inhibitors (43, 111, 112). Thus, evaluation of atherosclerotic lesions with CD8 radiotracers, may be able to identify those at risk for myocardial infarction in patients on checkpoint inhibitor therapy.
Detection of Vulnerable Plaque
Both checkpoint inhibitor use and certain TKIs like ponatinib and sorafenib have been associated with increased risk of myocardial infarction (43, 113). ICIs have also been associated with increased risk of stroke (114). Use of ICIs have been associated with increased infiltration of CD3, CD8 and CD68 cells, markers for T cells and macrophages respectively into atherosclerotic lesions (115). Increased somatostatin receptor 2 (SSTR2) on the cell surface of inflammatory macrophages is a marker of macrophage activation. In a study of symptomatic stroke patients, increased uptake of SSTR2 in culprit vessels assessed by PET tracer 68Ga-DOTATATE was shown to predict plaque rupture (58). Thus, evaluation of SSRT2 levels in patients on ICI therapy may help identify vulnerable plaques and warrants further investigation. The mechanisms for TKI mediated MI on the other hand are attributed to endothelial cell dysfunction and activation of apoptosis pathways, although direct evidence for MI mechanisms are still lacking, thus further research would be needed to see if macrophage activation is involved and whether activated macrophage imaging would help risk stratify patients on these TKIs (113).
FAP Imaging in ICI Myocarditis
Another potential marker of early stages of ICI myocarditis is fibroblast activating protein (FAP), which is a protein that gets significantly upregulated in cancer tissue, atherosclerosis, arthritis and fibrosis. It is emerging as an imaging marker for fibroblast activation and fibrosis (116, 117). A PET radiotracer tracer targeting FAP is 68Ga-FAPI. In a recent study, 68Ga-FAPI was shown to be a potential early marker of ICI myocarditis with median standardized uptake values (SUV) 1.79 (IQR 1.62, 1.85) in myocarditis patients vs. 1.15 (IQR 0.955, 1.52) in non-myocarditis patients (45). FAP has also been used to evaluate post myocardial infarction fibrosis, but its level in the blood vessels and myocardium of patients on checkpoint inhibitors is unclear (118, 119).
PD1 Imaging as a Potential Risk Factor for ICI Myocarditis
Another challenge with checkpoint inhibitor myocarditis is trying to figure out who is at increased risk. Programmed cell death protein 1 (PD1), a target of checkpoint inhibitors like pembrolizumab and its expression on cardiomyocytes warrants additional research as a potential risk factor. PET radiotracer, 64Cu-DOTA-pembrolizumab can detect PD1 in rodent hearts as well as on the surface of human blood cells and may be used in such an investigation (120).
MRI DGE Limitations in Fibrosis Assessment and Collagen Imaging
A higher burden of DGE and presumed scarring in hypertrophic cardiomyopathy is associated with worse cardiovascular and death outcomes (121, 122). In a retrospective study of ICI myocarditis patients who underwent cMRI, DGE evaluation did not correlate with cardiovascular outcomes, nor fibrosis, with only 35% of pathology proven fibrosis cases showing DGE on MRI (96, 121, 123, 124). Further, of the 56 patients with histopathology available either through biopsy or autopsy, 98% had lymphocytic infiltration but only 38% had DGE and 26% with T2 positivity (96). Thus in addition to evaluation of lymphocytic infiltration with targeted radiotracers for CD4 and CD8 cells to identify early stages of myocarditis and increase sensitivity of diagnosis, late stages of myocardial injury that can result in scar and thus collagen deposition can be evaluated by radiotracers targeting collagen. The PET radiotracer 68Ga-collagelin targets collagen, which can help quantify the burden of scarring or end stage fibrosis, which was shown to be able to detect pulmonary fibrosis in a mouse model of bleomycin induced pulmonary fibrosis and correlated with fibrosis on pathology (46) (Figure 3). MRI with DGE is able to evaluate for possible scarring, but it is not able to distinguish between early vs. late stage fibrosis, with the former having potential reversibility and may partially explain the differential outcomes we see between HCM and ICI myocarditis patients when it comes to the differences in the fibrosis processes between the two conditions and correlation of scar burden as quantified by DGE and outcomes (125). There is also a MRI collagen type I targeted probe EP-3533 that is conjugated to gadolinium, which was shown to be able to visualize pulmonary, liver and bowel fibrosis in rodent models, but these have not yet advanced to use in humans (126–128).
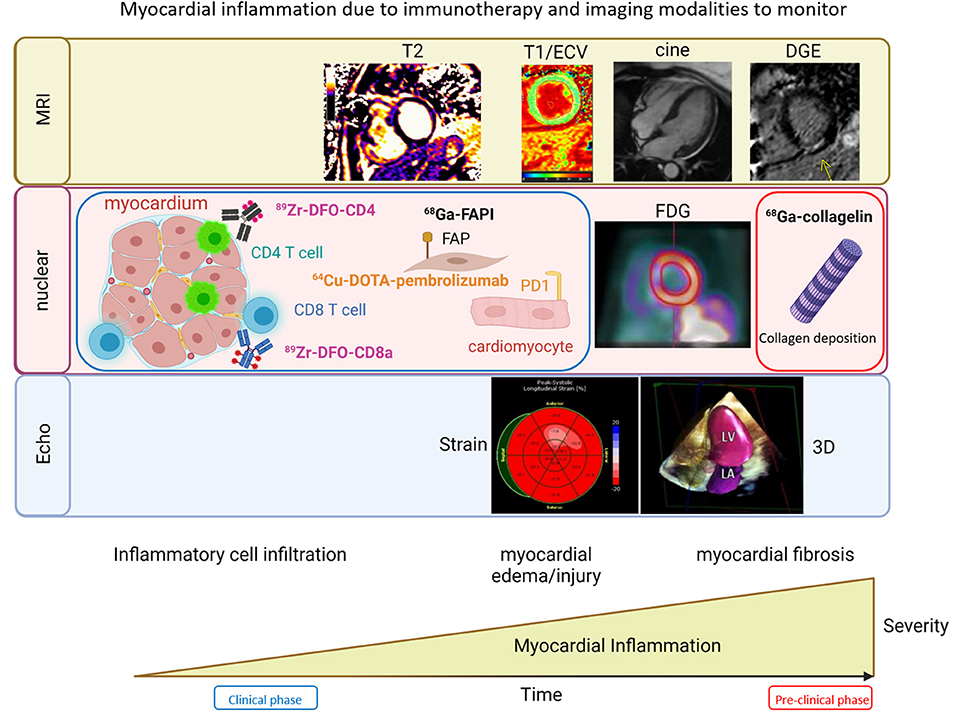
Figure 3. Imaging modalities in the evaluation of immunotherapy related cardiotoxicities. Imaging modalities that can be used to monitor myocardial inflammation due to immunotherapy include: MRI (top) using tissue characterization assessments such as T2, T1/ECV, delayed gadolinium enhancement (DGE) and cine to evaluate wall motion and function; Nuclear Imaging (middle) approaches involving molecularly targeted probes conjugated to radiotracers facilitating evaluation of CD4 cells with 89Zr-DFO-CD4, CD8 cells with 89Zr-DFO-CD8, early signs of fibrosis with fibroblast activation protein (FAP), expression of PD1 on cardiomyocytes, which can be seen with 64Cu-DOTA-pembrolizumab and may reflect increased risk of checkpoint inhibitor myocarditis, FDG that allows for monitoring of inflammation, and the final stages of inflammation with tissue damage and fibrosis and scar deposition assessed with collagen imaging with 68Ga collagelin; Echocardiography (bottom) is able to evaluate regional and global strain to detect signs of chemotherapy related toxicity and myocarditis.
Thrombosis Imaging
Pathologic thromboses like pulmonary embolism (PE), deep vein thrombosis (DVT) carries high morbidity and mortality (129). Cancer patients are at increased risk of thrombosis and some of their oncologic therapies can increase that risk further (130, 131). ICI, VEGF inhibitors and lenalidomide have been associated with increased thrombosis risk. Increasing the sensitivity of diagnosing blood clots so treatment can be timely instigated may help avoid complications and help improve outcomes (132–134). Radiotracers that can target fibrin, a molecular precursor of blood clotting can be useful in detection of blood clots. PET radiotracer 64CU-FBP8 can target fibrin and has been used to identify thrombi in animal models, particularly earlier stages of clots (49). Another PET radiotracer, 18F-GP1 that targets the glycoprotein IIb/IIIa receptors on activated platelets and has been demonstrated to detect venous thrombosis and arterial thromboses (53, 135). A phase 1, first-in-human study of 18F-GP1 positron emission tomography for imaging acute arterial thrombosis is underway (53). These PET thrombosis imaging agents may be of utility for detection of DVTs and PEs in cancer patients, especially for those who may have contraindications to contrast, such as those with chronic kidney disease or those who have an allergy to contrast.
Molecular MRI and MR Spectroscopy
Hyperpolarized MRI for Evaluation of Cardiac Metabolism in vivo
As the human heart failures, it has been shown to shift its metabolism from predominantly fatty acid oxidation to more glucose utilization (136). Changes in oxidative phosphorylation or substrate utilization may reflect early signs of cardiotoxicity, yet in vivo real time detection of cardiac metabolism has been limited to small studies with radioactive tracers using PET. More recently, substrate utilization and metabolism have been evaluated using magnetic resonance (MR) imaging and spectroscopy. Hyperpolarized carbon-13 (13C) labeled pyruvate imaging is different from standard clinical MRI using gadolinium contrast, in that it provides information on how tissue uses carbon-based nutrients (37). In rodent models of anthracycline cardiotoxicity, carbon-13 MR spectroscopy (MRS) was used to assess changes to oxidative phosphorylation and tricarboxylic acid (TCA) cycle flux in vivo. These studies showed that doxorubicin lead to reduced cardiac oxidative phosphorylation in a rat model as evidenced by increased 13C lactate production (38). First in human MRS was used to evaluate tumor metabolism in prostate cancer and ongoing clinical trials are evaluating hyperpolarized MR in tumor metabolism and correlations with outcomes in prostate and pancreatic cancer (137–139). First use of hyperpolarized 13C metabolic MRI in human heart involved evaluation of pyruvate metabolism in healthy individuals (39). Hyperpolarized MR imaging may allow for visualization of changes in cardiac energetics, particularly from fatty acid metabolism to more glucose utilization in an evolving cardiomyopathy in response to cardiotoxic chemotherapy and to evaluate response to cardioprotective medications such as beta blockers and angiotensin converting enzyme inhibitors in real time (140).
Apoptosis Evaluation by MRI
Various chemotherapy agents, most notably anthracyclines are known to increase cardiomyocyte apoptosis. Molecular MRI probes conjugated to superparamagnetic iron oxide (SPIO) and human annexin was shown to be able to visualize apoptosis in real time in a rodent model following ischemia and post doxorubicin exposure, but these MRI molecular probes have not gone beyond animal studies thus far but have the potential to detect early signs of cell death in the myocardium (105, 141).
Inflammation Imaging by MRI
In addition to T1, ECV and T2 signal changes, use of ultrasmall superparamagnetic particles of iron oxide (USPIOs) in MRI may confer insights on inflammation via increased macrophage activity. USPIOs have been shown to be taken up by macrophages and correlates with plaque inflammation in animal studies (142). In a study of patients with severe carotid stenosis, uptake of USPIOs corresponded to inflamed plaques on histology. Uptake of USPIOs induced areas of signal loss on -weighted magnetic resonance imaging within the vessel wall. Whether this can help predict plaque vulnerability in those on checkpoint inhibitors or help identify ICI myocarditis is untested and warrants further investigation (143). However, this has been used clinically and may have potential to distinguish vulnerable plaque from less vulnerable plaque.
Barriers to Advancing Molecular Imaging
For the molecular imaging tracers that are already clinically used, barriers to use include radiation exposure, so deciding who should get the test, when to get it and how often will have to be established. For example, if FAP is associated with ICI myocarditis as a potential early marker, then perhaps it should be obtained when there is suspicion for myocarditis or when troponin becomes positive. Timed with evaluation of this marker for residual disease, it can also help with monitoring of resolution of myocarditis, potentially complementing cardiac MRI or taking place of MRI for those who cannot tolerate MRI, which is usually used for monitoring. Access is another challenge. Access to molecular nuclear studies are often available through large hospital systems and for agents with shorter radioisotope half-lives like Gallium-68 (68Ga) with average half-life of 68 min, an onsite germanium-68/gallium-68 generator is needed along with accompanying nuclear accreditation, thus, more rural hospitals or private practices may have to refer out to larger centers in order to obtain these tests at high volume imaging centers (144). Finally, nuclear studies tend to be more expensive than echo and either on par or more expensive than MRI studies due to the costs associated with radiolabeled probes, thus being able to get these studies approved can also be a challenge for providers even if it is clinically used and indicated. For the molecular tracers that are in the preclinical stage, the usual barriers exist for clinical translation, including establishing safety, a favorable target to noise ratio in humans and correlation with outcomes to achieve FDA approval and ultimately clinical use. For those radiotracers that are already in clinical use for oncology indications, such as FAP, CD4, CD8 and PD1, incidental detection in the heart and correlation with outcomes is possible and can be further explored for future dedicated cardiac imaging and may provide unique clinical value. The power of machine learning, artificial intelligence and big data in evaluation of imaging signals can help unlock patterns that humans may not readily be able to see, such as in a recent evaluation of cardiac fibrosis by T1 imaging by MRI and be able to correlate these imaging findings with outcomes (145).
Role of Artificial Intelligence (AI) and Big Data in Cardio-Oncology and Imaging
Overview of Current AI Applications in Cardio-Oncology
Artificial intelligence (AI), through the training of machine and deep learning models, has shown remarkable potential in the prevention and diagnosis of cancer therapeutics-related cardiac dysfunction (CTRCD). With applications across all stages of the natural history of CTRCD, AI can assist scientists and physicians in screening for molecular interactions between novel therapeutic agents and the cardiovascular system, as well as detecting subclinical cardiovascular effects prior to the development of overt clinical disease (Figure 4).
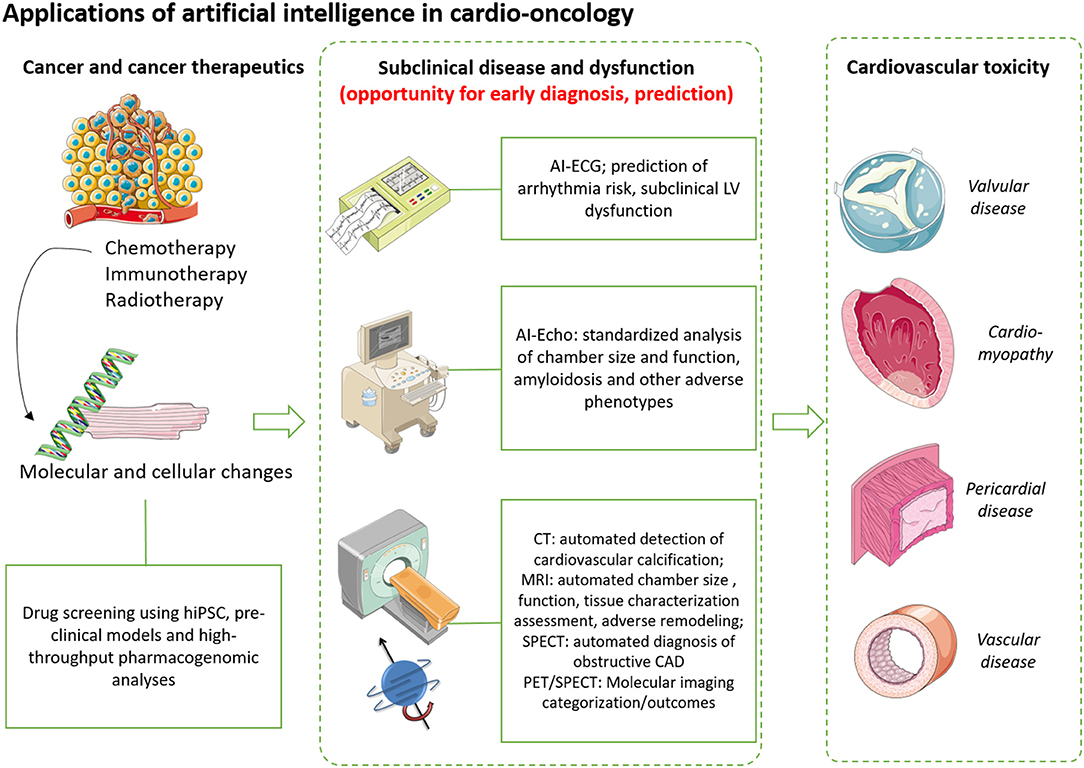
Figure 4. Applications of artificial intelligence, big data in cardio-oncology. Artificial intelligence (AI) can improve our understanding of the early molecular and phenotypic changes that occur prior to the development of clinical cancer therapeutics-related cardiac dysfunction. Machine learning approaches enable high-throughput screening of novel therapeutics using preclinical models, such as induced pluripotent stem cells as well as in silico simulations using libraries of drugs and molecular targets. In the clinical setting, AI can improve risk prediction of left ventricular dysfunction, arrhythmias as well as facilitate accurate and standardized assessment of chamber size, function and coronary calcification, all hallmarks of cardiovascular disease that can be caused or exacerbated by cancer therapeutics. Therefore, AI offers an opportunity for early diagnosis and deployment of strategies to prevent the progression to overt cardiovascular disease. Images have been reproduced under a Creative Commons Attribution 3.0 Unported License from smart.servier.com. CAD, coronary artery disease; CT, computed tomography; ECG, electrocardiography; hiPSC, human induced pluripotent stem cell; LV, left ventricular; MRI, magnetic resonance imaging; SPECT, single photon emission computed tomography.
At the pre-clinical stage, AI techniques have been used for high-throughput screening of cancer agents using a variety of disease models. These range from human induced pluripotent stem cell-derived cardiomyocytes (hiPSC-CMs) exposed to antineoplastic agents, screening of drug libraries to detect agents that interact with channel proteins resulting in QT prolongation, all the way to exome sequencing to identify variants in cardiac injury pathway genes that protect against anthracycline-induced cardiotoxicity and dual transcriptomic and molecular machine learning to predict different types of cardiotoxic response (146–150). Such approaches can de-risk early-stage drug discovery but also contribute to post-marketing surveillance to maximize patient safety. On the same note, pharmacovigilance in cardio-oncology can be assisted by machine learning-guided monitoring of electronic health records that includes patient demographics, echocardiography, laboratory values to detect signals suggestive of increased cardiac risk with specific therapies or practices (151, 152).
For therapies that form the mainstay of cancer therapy, ranging from chemotherapy to immunotherapy and radiation therapy, active surveillance protocols have been proposed and implemented, particularly for therapies with known cardiotoxic effects, such as anthracyclines and HER-2/neu inhibitors. Here, non-invasive cardiac imaging (by means of transthoracic echocardiography and/or magnetic resonance imaging (MRI)) and electrocardiography (ECG) represent the modalities of choice in the screening of conditions, such as anthracycline-induced cardiotoxicity and immune checkpoint-induced myocarditis (78, 153). Whereas AI applications in cardiovascular imaging have traditionally been developed in the general population, shared phenotypes seen in both CTRCD and non-cancer-related cardiac dysfunction, may extend the use of these technologies to cardio-oncology.
An expanding body of research has in fact demonstrated the ability of deep learning-enhanced interpretation of ECG in screening for and improving the diagnosis of left ventricular dysfunction, essentially functioning as a gatekeeper to the use of more advanced imaging modalities (154). It is notable that this tool was tested in a randomized controlled trial and demonstrated effectiveness in increasing the early diagnosis of decreased left ventricular ejection fraction (LVEF) without an increase in the use of echocardiography (155). Similarly, AI-guided ECG assessment can also predict the future incidence of atrial fibrillation (156). In childhood cancer survivors, machine learning algorithms of baseline and follow up ECGs were able to predict future cardiomyopathy (157). However, whether these results generalize to cardio-oncology, such as in the monitoring of anthracycline or Herceptin mediated cardiotoxicity, or ibrutinib-associated atrial fibrillation remains unknown and should be explored in future studies (158, 159).
AI has contributed to a more efficient and standardized interpretation of several non-invasive cardiovascular imaging modalities. For instance, in the field of transthoracic echocardiography, deep learning video-based models now enables fast and automated calculation of LVEF, with variance that is comparable to that or even lower of a human observer (160, 161). Similarly, combined assessment of ECG- and echocardiography-derived AI models has shown good discrimination in detecting cardiac amyloidosis, a rare disorder that is however more prevalent among patients with cancer compared to the general population (162). Similar approaches can be found in the field of computed tomography (CT) imaging, where automated tools enable an accurate assessment of coronary artery calcium burden, which can be generalized to both gated and non-gated CT scans of the chest, with the latter often used in the staging or monitoring of patients (163, 164). Therefore, such tools may refine a patient's baseline cardiovascular risk and inform risk-benefit discussions about the deployment of potentially cardiotoxic therapies. Finally, automated chamber size quantification, tissue characterization parameters such as T1, T2, extracellular volume and functional indices that can be extracted from cardiac MRI images can have the ability to confer insights into cardiotoxicity including the potential to identify early to late cardiotoxicity mediated by chemotherapy or immunotherapy agents via detection of changes in chamber size, abnormal T1, T2 relaxation times and delayed gadolinium enhancement patterns (86, 95, 96, 99, 145, 165–167). Deep learning models have also shown promise in the standardized interpretation of functional nuclear modalities, such as SPECT (single photon emissions computed tomography) myocardial perfusion imaging with good discrimination for the presence of obstructive coronary artery disease (168). However, as these tools become clinically available, prospective validation and possibly recalibration specifically in patients with cancer will be required to ensure their validity and generalizability.
Strengths and Weaknesses of Current Methods and Barriers for Clinical Translation
To better understand the strengths and weaknesses of AI applications in cardio-oncology, one first needs to review key definitions. AI refers to the ability of an automated system to perform tasks that are typically characteristic of human intelligence, such as image and pattern recognition, as well as prediction and classification. Machine learning describes the process by which a system gains the ability to perform such tasks. This learning process can be further divided into supervised and unsupervised learning. The former describes the analysis of labeled datasets with the goal of predicting the label of a given datapoint based on a set of independent predictors. The latter refers to the analysis of unlabeled and unclassified datasets where the algorithm attempts to discover patterns within the data on its own. Algorithms may range from traditional regression models to deep neural networks, consisting of multiple layers of neurons and nodes which operate in a manner similar to the human brain (169, 170). However, independent of the algorithm used, machine learning systems rely on high-quality input to deliver high-quality output. This is where “big data” become relevant, describing the need for datasets that are large enough to ensure adequate variance, remain representative of their original and target populations, enable time-efficient analyses and have been carefully rather opportunistically curated to address a specific question (171).
With those key concepts in mind, some of the limitations of machine learning applications in cardio-oncology become apparent. First, cardiovascular disease is often listed as an exclusion criterion in major cancer trials, thus resulting in under-representation of patients with cardiovascular disease in pivotal cancer trials (172). However, the inclusion of cardiovascular outcomes in cancer trials will be able to help fill this data gap if sufficient baseline and follow up data are acquired (molecular biomarkers, baseline imaging prior to oncologic therapy and follow up that can be used as input). Second, while AI systems can learn patterns in the data, explaining what drives those predictions or establishing causal inference is not a straightforward task (173). Moreover, cancer is a highly heterogeneous condition with multiple molecular, histological, and clinical subtypes that often respond differently to the same therapies (174). Therefore, ensuring generalizability of models across different cancer subtypes, treatments and patient populations may be an insurmountable task without access to vast amounts of accurately labeled data. Third, there is often significant delay in the timing between data collection, model training and the final model deployment. As a result, AI models are often outdated when deployed for clinical use, thus highlighting the need for more efficient pathways that would enable real-time updates. Finally, AI models are bias-prone often reproducing biases that are inherently present in the datasets used for training. Ensuring representation of diverse patient populations is of paramount importance to promote an equitable impact of AI in healthcare delivery and outcomes (175).
Future Applications of AI in Cardio-Oncology and Molecular Imaging
With careful consideration of these limitations, AI has the potential to advance cardio-oncology in many different directions. Radiomic applications, which extract several metrics based on the shape, dimensions, signal density and spatial interrelationship of voxel signals in a given tissue, have been found to be superior to conventional readouts in reflecting tissue composition, as well as metabolic or inflammatory activity (176–178). In fact, some of the most exciting applications of AI lie beyond structural imaging in molecular imaging. In the recent past, deep learning and generative adversarial networks have successfully reconstructed PET images directly from raw sinogram data effectively maximizing image quality (179, 180). In other applications, AI tools have generated full-dose PET images from low-dose images, thus maximizing signal-to-noise ratio at lower radiation levels (181, 182). In another example, convolutional neural networks have enabled the development of cMRI virtual native imaging technologies which generate late gadolinium enhancement-like images in an accurate and reproducible manner without the need for contrast administration (183). Though originally developed in patients with hypertrophic cardiomyopathy, this technology may be of value in cardio-oncology and the monitoring of ICI-myocarditis. Further, for molecular imaging targeting biomarkers like FAP and PD1, these are already used clinically in oncology to monitor for residual disease and assess response to immunotherapy respectively, thus if the heart is captured in existing data sets, AI/ML can help to predict whether the presence of these markers are associated with adverse cardiovascular outcomes. Coupled with improvements in the speed and accuracy of segmentation algorithms, AI can accelerate the clinical deployment of molecular imaging approaches in the timely detection of cardiovascular toxicity (184).
Conclusions
Imaging advances, particularly molecularly targeted imaging modalities may help detect cardiotoxicities at the earliest stages with greater specificity, shed light on mechanism as well as response to cardioprotective medications such as beta blockers, angiotensin converting enzyme inhibitors, etc. Newer MRI metabolic evaluation techniques such as hyperpolarized MRI may allow for a non-invasive approach to evaluate cardiac metabolism in real time. To complement imaging studies, use of AI and big data on imaging parameters and forthcoming molecular imaging datasets, in addition to patient demographics may help predict or detect cardiovascular toxicities at their earliest stages. Inclusion of diverse patient cohorts as well as cardiovascular parameters/biomarkers and imaging in cancer trials can enable AI/Ml to increase accurate categorization as well prediction models in cardio-oncology patients. Additional research in these areas and advancing animal studies toward human studies may further help improve cardiovascular outcomes in cancer patients.
Author Contributions
JK led the development of the manuscript, writing, and generation of figures. EO contributed to writing and generation of figures. MH contributed to writing, assisting with editing and organizing of the manuscript. AJS oversaw the writing, editing, and review of the manuscript. All authors contributed to the article and approved the submitted version.
Funding
This work was funded by NIH R01 HL113352, R01 HL121226, R01 HL123949, and R01 HL154345 associated with AJS.
Conflict of Interest
AJS is a limited partner and consultant of MicroVide, LLC, which holds the license for the use of 99mTc-RP805 in myocardial applications.
The authors declare that the research was conducted in the absence of any commercial or financial relationships that could be construed as a potential conflict of interest.
Publisher's Note
All claims expressed in this article are solely those of the authors and do not necessarily represent those of their affiliated organizations, or those of the publisher, the editors and the reviewers. Any product that may be evaluated in this article, or claim that may be made by its manufacturer, is not guaranteed or endorsed by the publisher.
Acknowledgments
Biorender was used to create Figures 1–3.
Abbreviations
AI, artificial intelligence; CM, cardiomyopathy; CML, chronic myelogenous leukemia; DGE, delayed gadolinium enhancement; DNA, Deoxyribonucleic acid; ECV, extracellular volume; GLS, global longitudinal strain; ICI, checkpoint inhibitors; HER2, human epidermal growth factor receptor 2; HF, heart failure; HTN, hypertension; MI, myocardial infarction; MUGA, multigated acquisition; ROS, reactive oxygen species; TdP, torsades de pointe; TKI, tyrosine kinase inhibitor; VTE, venous thromboembolism.
References
1. Weir HK, Thompson TD, Stewart SL, White MC. Cancer incidence projections in the United States between 2015 and 2050. Prevent Chronic Dis. (2021) 18:E59. doi: 10.5888/pcd18.210006
2. Eckstein N, Röper L, Haas B, Potthast H, Hermes U, Unkrig C, et al. Clinical pharmacology of tyrosine kinase inhibitors becoming generic drugs: the regulatory perspective. J Exp Clin Cancer Res. (2014) 33:1. doi: 10.1186/1756-9966-33-15
3. Vito A, Salem O, El-Sayes N, MacFawn IP, Portillo AL, Milne K, et al. Immune checkpoint blockade in triple negative breast cancer influenced by B cells through myeloid-derived suppressor cells. Commun Biol. (2021) 4:1–19. doi: 10.1038/s42003-021-02375-9
4. Schmid P, Adams S, Rugo HS, Schneeweiss A, Barrios CH, Iwata H, et al. Atezolizumab and nab-paclitaxel in advanced triple-negative breast cancer. New Engl J Med. (2018) 379:2109–21. doi: 10.1056/NEJMoa1809615
5. Siegel RL, Miller KD, Fuchs HE, Jemal A. Cancer statistics, 2021. CA Cancer J Clin. (2021) 71:9–29. doi: 10.3322/caac.21654
6. Vaddepally RK, Kharel P, Pandey R, Garje R, Chandra AB. Review of indications of FDA-approved immune checkpoint inhibitors per NCCN guidelines with the level of evidence. Cancers. (2020) 12:738. doi: 10.3390/cancers12030738
7. Guha A, Caraballo C, Jain P, Miller PE, Owusu-Guha J, Clark KAA, et al. Outcomes in patients with anthracycline-induced cardiomyopathy undergoing left ventricular assist devices implantation. ESC Heart Failure. (2021) 8:2866–75. doi: 10.1002/ehf2.13362
8. Russo M, della Sala A, Tocchetti CG, Porporato PE, Ghigo A. Metabolic aspects of anthracycline cardiotoxicity. Curr Treat Options Oncol. (2021) 22:1–21. doi: 10.1007/s11864-020-00812-1
9. Zhang S, Liu X, Bawa-Khalfe T, Lu LS Lyu YL, Liu LF, et al. Identification of the molecular basis of doxorubicin-induced cardiotoxicity. Nat Med. (2012) 18:1639–42. doi: 10.1038/nm.2919
10. Rosen SD. Trastuzumab induced cardiomyopathy: Wider implications for cardio-oncology. Heart. (2013) 99:599–600. doi: 10.1136/heartjnl-2012-303495
11. Hu Y, Sun B, Zhao B, Mei D, Gu Q, Tian Z. Cisplatin-induced cardiotoxicity with midrange ejection fraction: a case report and review of the literature. Medicine. (2018) 97:e13807. doi: 10.1097/MD.0000000000013807
12. Dhesi S, Chu MP, Blevins G, Paterson I, Larratt L, Oudit GY, et al. Cyclophosphamide-induced cardiomyopathy. J Investig Med High Impact Case Rep. (2013) 1:2324709613480346. doi: 10.1177/2324709613480346
13. Chong JH, Ghosh AK. Coronary artery vasospasm induced by 5-fluorouracil: proposed mechanisms, existing management options and future directions. Interv Cardiol Rev. (2019) 14:89. doi: 10.15420/icr.2019.12
14. Lestuzzi C, Vaccher E, Talamini R, Lleshi A, Meneguzzo N, Viel E, et al. Effort myocardial ischemia during chemotherapy with 5-fluorouracil: an underestimated risk. Ann Oncol. (2014) 25:1059–64. doi: 10.1093/annonc/mdu055
15. Hirsh J. Risk of thrombosis with lenalidomide and its prevention with aspirin. Chest. (2007) 131:275–7. doi: 10.1378/chest.06-2360
16. Li A, Wu Q, Warnick G, Li S, Libby EN, Garcia DA, et al. The incidence of thromboembolism for lenalidomide versus thalidomide in older patients with newly diagnosed multiple myeloma. Ann Hematol. (2020) 99:121–6. doi: 10.1007/s00277-019-03860-2
17. Alahmari AK, Almalki ZS, Alahmari AK, Guo JJ. Thromboembolic events associated with bevacizumab plus chemotherapy for patients with colorectal cancer: a meta-analysis of randomized controlled trials. Am Health Drug Benefits. (2016) 9:221.
18. Syrigos KN, Karapanagiotou E, Boura P, Manegold C, Harrington K. Bevacizumab-induced hypertension: pathogenesis and management. BioDrugs. (2011) 25:159–69. doi: 10.2165/11590180-000000000-00000
19. Khalid S, Yasar S, Khalid A, Spiro T, Haddad A, Daw H. Management of atrial fibrillation in patients on ibrutinib: a cleveland clinic experience. Cureus. (2018) 10:e2701. doi: 10.7759/cureus.2701
20. Uraizee I, Cheng S, Moslehi J. Reversible cardiomyopathy associated with sunitinib and sorafenib. New Engl J Med. (2011) 365:1649–50. doi: 10.1056/NEJMc1108849
21. Chen MH, Kerkelä R, Force T. Mechanisms of cardiac dysfunction associated with tyrosine kinase inhibitor cancer therapeutics. Circulation. (2008) 118:84–95. doi: 10.1161/CIRCULATIONAHA.108.776831
22. Siegel RL, Miller KD, Jemal A. Cancer statistics, 2019. CA Cancer J Clin. (2019) 69:7–34. doi: 10.3322/caac.21551
23. Haslam A, Gill J, Prasad V. Estimation of the percentage of US patients with cancer who are eligible for immune checkpoint inhibitor drugs. JAMA Netw Open. (2020) 3:e200423. doi: 10.1001/jamanetworkopen.2020.0423
24. Bar J, Markel G, Gottfried T, Percik R, Leibowitz-Amit R, Berger R, et al. Acute vascular events as a possibly related adverse event of immunotherapy: a single-institute retrospective study. Eur J Cancer. (2019) 120:122–31. doi: 10.1016/j.ejca.2019.06.021
25. Mahmood SS, Fradley MG, Cohen JV, Nohria A, Reynolds KL, Heinzerling LM, et al. Myocarditis in patients treated with immune checkpoint inhibitors. J Am Coll Cardiol. (2018) 71:1755–64. doi: 10.1016/j.jacc.2018.02.037
26. Alvi RM, Frigault MJ, Fradley MG, Jain MD, Mahmood SS, Awadalla M, et al. Cardiovascular events among adults treated with chimeric antigen receptor T-cells (CAR-T). J Am Coll Cardiol. (2019) 74:3099–108. doi: 10.1016/j.jacc.2019.10.038
27. Kwan JM, Henry ML, Cook K, Higgins A, Cuomo J, Foss F, et al. Adverse cardiovascular events in patients treated with mogamulizumab. Am Heart J Plus Cardiol Res Pract. (2021) 9:100049. doi: 10.1016/j.ahjo.2021.100049
28. Kwan JM, Odanovic N, Arbune A, Higgins A, Henry M, Greif D, et al. Mogamulizumab-associated acute myocarditis in a patient with T-Cell lymphoma. JACC: Case Rep. (2021) 3:1018–23. doi: 10.1016/j.jaccas.2021.04.001
29. Chatterjee K, Zhang J, Honbo N, Karliner JS. Doxorubicin cardiomyopathy. Cardiology. (2010) 115:155–62. doi: 10.1159/000265166
30. Carrio I, Estorch M, Berna L, Lopez-Pousa J, Tabernero J, Torres G. Indium-111-antimyosin and iodine-123-MIBG studies in early assessment of doxorubicin cardiotoxicity. J Nuclear Med. (1995) 36:2044–9.
31. Boutagy NE, Feher A, Pfau D, Liu Z, Guerrera NM, Freeburg LA, et al. Dual angiotensin receptor-neprilysin inhibition with sacubitril/valsartan attenuates systolic dysfunction in experimental doxorubicin-induced cardiotoxicity. JACC: CardioOncol. (2020) 2:774–87. doi: 10.1016/j.jaccao.2020.09.007
32. Bhattacharya S, Liu X, jie Senior R, Jain D, Leppo JA, Lahiri A. 111In antimyosin antibody uptake is related to the age of myocardial infarction. Am Heart J. (1991) 122:1583–7. doi: 10.1016/0002-8703(91)90274-L
33. Bennink RJ, van den Hoff MJ, van Hemert FJ, de Bruin KM, Spijkerboer AL, Vanderheyden JL, et al. Annexin V imaging of acute doxorubicin cardiotoxicity (apoptosis) in rats. J Nuclear Medicine. (2004) 45:842–8. Available online at: https://jnm.snmjournals.org/content/45/5/842/tab-article-info
34. Blankenberg FG, Kalinyak J, Liu L, Koike M, Cheng D, Goris ML, et al. 99mTc-HYNIC-annexin V SPECT imaging of acute stroke and its response to neuroprotective therapy with anti-Fas ligand antibody. Eur J Nucl Med Mol Imag. (2006) 33:566–74. doi: 10.1007/s00259-005-0046-6
35. Boutagy NE, Wu J, Cai Z, Zhang W, Booth CJ, Kyriakides TC, et al. In vivo reactive oxygen species detection with a novel positron emission tomography tracer, 18F-DHMT, allows for early detection of anthracycline-induced cardiotoxicity in rodents. JACC Basic Transl Sci. (2018) 3:378–90. doi: 10.1016/j.jacbts.2018.02.003
36. Sivapackiam J, Kabra S, Speidel S, et al. 68Ga-Galmydar: a PET imaging tracer for noninvasive detection of Doxorubicin-induced cardiotoxicity. PLoS ONE. (2019) 14:e0215579. doi: 10.1371/journal.pone.0215579
37. Rider OJ, Tyler DJ. Clinical implications of cardiac hyperpolarized magnetic resonance imaging. J Cardiovasc Magn Reson. (2013) 15:1–9. doi: 10.1186/1532-429X-15-93
38. Timm KN, Perera C, Ball V, Henry JA, Miller JJ, Kerr M, et al. Early detection of doxorubicin-induced cardiotoxicity in rats by its cardiac metabolic signature assessed with hyperpolarized MRI. Commun Biol. (2020) 3:1–10. doi: 10.1038/s42003-020-01440-z
39. Cunningham CH, Lau JYC, Chen AP, Geraghty BJ, Perks WJ, Roifman I, et al. Hyperpolarized 13C metabolic MRI of the human heart: initial experience. Circ Res. (2016) 119:1177–82. doi: 10.1161/CIRCRESAHA.116.309769
40. Inayat F, Masab M, Gupta S, Ullah W. New drugs and new toxicities: pembrolizumab-induced myocarditis. BMJ Case Reports. (2018) 2018:bcr-2017. doi: 10.1136/bcr-2017-223252
41. Yun S, Vincelette ND, Mansour I, Hariri D, Motamed S. Late onset ipilimumab-induced pericarditis and pericardial effusion: a rare but life threatening complication. Case Rep Oncol Med. (2015) 2015:1–5. doi: 10.1155/2015/794842
42. Chen DY, Huang WK, Wu VC, Chang WC, Chen JS, Chuang CK, et al. Cardiovascular toxicity of immune checkpoint inhibitors in cancer patients: A review when cardiology meets immuno-oncology. J Formosan Med Assoc. (2020) 119:1461–75. doi: 10.1016/j.jfma.2019.07.025
43. Drobni ZD, Alvi RM, Taron J, Zafar A, Murphy SP, Rambarat PK, et al. Association between immune checkpoint inhibitors with cardiovascular events and atherosclerotic plaque. Circulation. (2020) 6:877–85. doi: 10.1161/CIRCULATIONAHA.120.049981
44. Kristensen LK, Fröhlich C, Christensen C, Melander MC, Poulsen TT, Galler GR, et al. CD4+ and CD8a+ PET imaging predicts response to novel PD-1 checkpoint inhibitor: studies of Sym021 in syngeneic mouse cancer models. Theranostics. (2019) 9:8221. doi: 10.7150/thno.37513
45. Finke D, Heckmann MB, Herpel E, Katus HA, Haberkorn U, Leuschner F, et al. Early detection of checkpoint inhibitor-associated myocarditis using 68Ga-FAPI PET/CT. Frontiers in Cardiovasc Med. (2021) 8:54. doi: 10.3389/fcvm.2021.614997
46. Désogère P, Tapias LF, Hariri LP, Rotile NJ, Rietz TA, Probst CK, et al. Type I collagen-Targeted PET probe for pulmonary fibrosis detection and staging in preclinical models. Sci Transl Med. (2017) 9:eaaf4696. doi: 10.1126/scitranslmed.aaf4696
47. The cancer drug imatinib can cause cardiotoxicity. Nat Clin Pract Cardiovasc Med. (2007) 4:8. doi: 10.1038/ncpcardio0699
48. Cortes JE, Jean Khoury H, Kantarjian H, Brümmendorf TH, Mauro MJ, Matczak E, et al. Long-term evaluation of cardiac and vascular toxicity in patients with Philadelphia chromosome-positive leukemias treated with bosutinib. Am J Hematol. (2016) 91:606–16. doi: 10.1002/ajh.24360
49. Blasi F, Oliveira BL, Rietz TA, Rotile NJ, Naha PC, Cormode DP, et al. Multisite thrombus imaging and fibrin content estimation with a single whole-body PET scan in rats. Arterioscler Thromb Vasc Biol. (2015) 35:2114–21. doi: 10.1161/ATVBAHA.115.306055
50. Case Medical Research. PET. Fibrin Imaging of DVT and PE. Available online at: https://wwwclinicaltrialsgov/. (2019).
51. Haguet H, Bouvy C, Delvigne AS, Modaffari E, Wannez A, Sonveaux P, et al. The risk of arterial thrombosis in patients with chronic myeloid leukemia treated with second and third generation BCR-ABL tyrosine kinase inhibitors may be explained by their impact on endothelial cells: an in-vitro study. Front Pharmacol. (2020) 11:1007. doi: 10.3389/fphar.2020.01007
52. Abu Rmilah AA, Lin G, Herrmann J. Risk of QTc interval prolongation among cancer patients treated with tyrosine kinase inhibitors. J Clin Oncol. (2019) 37(15_suppl):3160–7. doi: 10.1200/JCO.2019.37.15_suppl.3033
53. Chae SY, Kwon TW, Jin S, Kwon SU, Sung C, Oh SJ, et al. A phase 1, first-in-human study of 18 F-GP1 positron emission tomography for imaging acute arterial thrombosis. EJNMMI Res. (2019) 9:1–10. doi: 10.1186/s13550-018-0471-8
54. Merkulova A, Mitchell SC, Stavrou EX, Forbes GL, Schmaier AH. Ponatinib treatment promotes arterial thrombosis and hyperactive platelets. Blood Adv. (2019) 3:2312. doi: 10.1182/bloodadvances.2019000034
55. Singh AP, Glennon MS, Umbarkar P, Gupte M, Galindo CL, Zhang Q, et al. Ponatinib-induced cardiotoxicity: delineating the signalling mechanisms and potential rescue strategies. Cardiovasc Res. (2019) 115:966–77. doi: 10.1093/cvr/cvz006
56. Chu TF, Rupnick MA, Kerkela R, Dallabrida SM, Zurakowski D, Nguyen L, et al. Cardiotoxicity associated with tyrosine kinase inhibitor sunitinib. Lancet. (2007) 370:2011–9. doi: 10.1016/S0140-6736(07)61865-0
57. Kloth JS, Pagani A, Verboom MC, Malovini A, Napolitano C, Kruit WH, et al. Incidence and relevance of QTc-interval prolongation caused by tyrosine kinase inhibitors. Br J Cancer. (2015) 112:1011–6. doi: 10.1038/bjc.2015.82
58. Tarkin JM, Joshi FR, Evans NR, Chowdhury MM, Figg NL, Shah AV, et al. Detection of atherosclerotic inflammation by 68Ga-DOTATATE PET compared to [18F]FDG PET imaging. J Am Coll Cardiol. (2017) 69:1774–91. doi: 10.1016/j.jacc.2017.01.060
59. Sanli Y, Garg I, Kandathil A, Kendi T, Zanetti MJ, Kuyumcu S, et al. Neuroendocrine tumor diagnosis and management: 68 Ga-DOTATATE PET/CT. Am J Roentgenol. (2018) 211:267–77. doi: 10.2214/AJR.18.19881
60. Qi WX, Shen Z, Lin F, Sun YJ, Min DL, Tang LN, et al. Incidence and risk of hypertension with vandetanib in cancer patients: A systematic review and meta-analysis of clinical trials. Br J Clin Pharmacol. (2013) 75:919–30. doi: 10.1111/j.1365-2125.2012.04417.x
61. Zang J, Wu S, Tang L, Xu X, Bai J, Ding C, et al. Incidence and risk of QTc interval prolongation among cancer patients treated with vandetanib: a systematic review and meta-analysis. PLoS ONE. (2012) 7:e30353. doi: 10.1371/journal.pone.0030353
62. Ewer MS, Patel K, O'Brien D, Lorence RM. Cardiac safety of afatinib: a review of data from clinical trials. Cardio-Oncology. (2015) 1:1–8. doi: 10.1186/s40959-015-0006-7
63. Ding S, Long F, Jiang S. Acute myocardial infarction following erlotinib treatment for NSCLC: a case report. Oncol Lett. (2016) 11:4240–4. doi: 10.3892/ol.2016.4508
64. Coppola C, Rienzo A, Piscopo G, Barbieri A, Arra C, Maurea N. Management of QT prolongation induced by anti-cancer drugs: target therapy and old agents. Different algorithms for different drugs. Cancer Treatment Rev. (2018) 63:135–43. doi: 10.1016/j.ctrv.2017.11.009
65. Omori S, Oyakawa T, Naito T, Takahashi T. Gefitinib-induced cardiomyopathy in epidermal growth receptor-mutated NSCLC. J Thoracic Oncol. (2018) 13:e207–8. doi: 10.1016/j.jtho.2018.05.020
66. Kadowaki H, Ishida J, Akazawa H, Yagi H, Saga-Kamo A, Umei M, et al. Axitinib induces and aggravates hypertension regardless of prior treatment with tyrosine kinase inhibitors. Circul Rep. (2021) 3:CR21. doi: 10.1253/circrep.CR-21-0008
67. Tougaard RS, Hansen ES, Laustsen C, Lindhardt J, Schroeder M, Bøtker HE, et al. Acute hypertensive stress imaged by cardiac hyperpolarized [1-13C]pyruvate magnetic resonance. Magn Reson Med. (2018) 80:2053–61. doi: 10.1002/mrm.27164
68. Guan J, Zhang M. Cardiotoxicity of anthracycline-free targeted oncological therapies in HER2-positive breast cancer (Review). Oncol Lett. (2021) 21:1. doi: 10.3892/ol.2020.12361
69. Sengupta PP, Northfelt DW, Gentile F, Zamorano JL, Khandheria BK. Trastuzumab-induced cardiotoxicity: heart failure at the crossroads. Mayo Clinic Proc. (2008) 83:197–203. doi: 10.1016/S0025-6196(11)60840-9
70. Lenihan D, Suter T, Brammer M, Neate C, Ross G, Baselga J. Pooled analysis of cardiac safety in patients with cancer treated with pertuzumab. Ann Oncol. (2012) 23:791–800. doi: 10.1093/annonc/mdr294
71. Yildirim M, Parlak C, Sezer C, Eryilmaz R, Kaya C, Yildiz M. Coronary vasospasm secondary to 5-fluorouracil and its management: case report. Eurasian J Med. (2011) 43:54. doi: 10.5152/eajm.2011.11
72. Curigliano G, Cardinale D, Dent S, Criscitiello C, Aseyev O, Lenihan D, et al. Cardiotoxicity of anticancer treatments: epidemiology, detection, and management. CA Cancer J Clin. (2016) 66:309–25. doi: 10.3322/caac.21341
73. Tu CM, Chu KM, Yang SP, Cheng SM, Wang W. Trastuzumab (Herceptin)-associated cardiomyopathy presented as new onset of complete left bundle-branch block mimicking acute coronary syndrome: a case report and literature review. Am J Emerg Med. (2009) 27:903. doi: 10.1016/j.ajem.2008.11.012
74. Mohan N, Jiang J, Dokmanovic M, Wu WJ. Trastuzumab-mediated cardiotoxicity: current understanding, challenges, and frontiers. Antibody Therap. (2018) 1:13–7. doi: 10.1093/abt/tby003
75. Celutkiene J, Pudil R, López-Fernández T, Grapsa J, Nihoyannopoulos P, Bergler-Klein J, et al. Role of cardiovascular imaging in cancer patients receiving cardiotoxic therapies: a position statement on behalf of the Heart Failure Association (HFA), the European Association of Cardiovascular Imaging (EACVI) and the Cardio-Oncology Council of the European Society of Cardiology (ESC). Eur J Heart Fail. (2020) 22:1504–24. doi: 10.1002/ejhf.1957
76. Gripp E de A, de Oliveira GE, Feijó LA, Garcia MI, Xavier SS, de Sousa AS. Global longitudinal strain accuracy for cardiotoxicity prediction in a cohort of breast cancer patients during anthracycline and/or trastuzumab treatment. Arq Bras Cardiol. (2018) 110:140–50. doi: 10.5935/abc.20180021
77. Curigliano G, Cardinale D, Suter T, Plataniotis G, De Azambuja E, Sandri MT, et al. Cardiovascular toxicity induced by chemotherapy, targeted agents and radiotherapy: ESMO clinical practice guidelines. Ann Oncol. (2012) 23(SUPPL. 7):vii155–66. doi: 10.1093/annonc/mds293
78. Plana JC, Galderisi M, Barac A, Ewer MS, Ky B, Scherrer-Crosbie M, et al. Expert consensus for multimodality imaging evaluation of adult patients during and after cancer therapy: a report from the American Society of Echocardiography and the European Association of Cardiovascular Imaging. Eur Heart J Cardiovasc Imaging. (2014) 15:1603–93. doi: 10.1093/ehjci/jeu192
79. Schneider BJ, Naidoo J, Santomasso BD, Lacchetti C, Adkins S, Anadkat M, et al. Management of immune-related adverse events in patients treated with immune checkpoint inhibitor therapy: ASCO guideline update. J Clin Oncol. (2021) 39:4073–126. doi: 10.1200/JCO.21.01440
80. Ferreira VM, Schulz-Menger J, Holmvang G, Kramer CM, Carbone I, Sechtem U, et al. Cardiovascular magnetic resonance in nonischemic myocardial inflammation: expert recommendations. J Am Coll Cardiol. (2018) 72:3158–76. doi: 10.1016/j.jacc.2018.09.072
81. Biersmith MA, Tong MS, Guha A, Simonetti OP, Addison D. Multimodality cardiac imaging in the era of emerging cancer therapies. J Am Heart Assoc. (2020) 9:e013755. doi: 10.1161/JAHA.119.013755
82. Lambert J, Lamacie M, Thampinathan B, Altaha MA, Esmaeilzadeh M, Nolan M, et al. Variability in echocardiography and MRI for detection of cancer therapy cardiotoxicity. Heart. (2020) 106:817–23. doi: 10.1136/heartjnl-2019-316297
83. Salerno M, Sharif B, Arheden H, Kumar A, Axel L, Li D, et al. Recent advances in cardiovascular magnetic resonance techniques and applications. Circ Cardiovasc Imaging. (2017) 47:e003951. doi: 10.1161/CIRCIMAGING.116.003951
84. Neilan TG, Coelho-Filho OR, Shah RV, Feng JH, Pena-Herrera D, Mandry D, et al. Myocardial extracellular volume by cardiac magnetic resonance imaging in patients treated with anthracycline-based chemotherapy. Am J Cardiol. (2013) 111:717–22. doi: 10.1016/j.amjcard.2012.11.022
85. Jordan JH, Vasu S, Morgan TM, D'Agostino RB Jr, Meléndez GC, Hamilton CA, et al. Anthracycline-associated T1 mapping characteristics are elevated independent of the presence of cardiovascular comorbidities in cancer survivors. Circul Cardiovasc Imag. (2016) 9:e004325. doi: 10.1161/CIRCIMAGING.115.004325
86. Lustberg MB, Reinbolt R, Addison D, Ruppert AS, Moore S, Carothers S, et al. Early detection of anthracycline-induced cardiotoxicity in breast cancer survivors with T2 cardiac magnetic resonance. Circul Cardiovasc Imag. (2019) 12:e008777. doi: 10.1161/CIRCIMAGING.118.008777
87. Fallah-Rad N, Lytwyn M, Fang T, Kirkpatrick I, Jassal DS. Delayed contrast enhancement cardiac magnetic resonance imaging in trastuzumab induced cardiomyopathy. J Cardiovasc Magn Reson. (2008) 10:1–4. doi: 10.1186/1532-429X-10-5
88. Thavendiranathan P, Poulin F, Lim KD, Plana JC, Woo A, Marwick TH. Use of myocardial strain imaging by echocardiography for the early detection of cardiotoxicity in patients during and after cancer chemotherapy: a systematic review. J Am College Cardiol. (2014) 63(25 PART A):2751–68. doi: 10.1016/j.jacc.2014.01.073
89. Oikonomou EK, Kokkinidis DG, Kampaktsis PN, Amir EA, Marwick TH, Gupta D, et al. Assessment of prognostic value of left ventricular global longitudinal strain for early prediction of chemotherapy-induced cardiotoxicity: a systematic review and meta-analysis. JAMA Cardiol. (2019) 4:1007–18. doi: 10.1001/jamacardio.2019.2952
90. Löffler AI, Salerno M. Cardiac MRI for the evaluation of oncologic cardiotoxicity. J Nuclear Cardiol. (2018) 25:2148–58. doi: 10.1007/s12350-018-1293-9
91. Drafts BC, Twomley KM, D'Agostino R, Lawrence J, Avis N, Ellis LR, et al. Low to moderate dose anthracycline-based chemotherapy is associated with early noninvasive imaging evidence of subclinical cardiovascular disease. JACC: Cardiovasc Imag. (2013) 6:877–85. doi: 10.1016/j.jcmg.2012.11.017
92. Jordan JH, Sukpraphrute B, Meléndez GC, Jolly MP, D'Agostino RB, Hundley WG. Early myocardial strain changes during potentially cardiotoxic chemotherapy may occur as a result of reductions in left ventricular end-diastolic volume: the need to interpret left ventricular strain with volumes. Circulation. (2017) 135:2575–7. doi: 10.1161/CIRCULATIONAHA.117.027930
93. Giusca S, Korosoglou G, Montenbruck M, Geršak B, Schwarz AK, Esch S, et al. Multiparametric early detection and prediction of cardiotoxicity using myocardial strain, T1 and T2 mapping, and biochemical markers: a longitudinal cardiac resonance imaging study during 2 years of follow-up. Circul Cardiovasc Imag. (2021) 14:e012459. doi: 10.1161/CIRCIMAGING.121.012459
94. Steen H, Montenbruck M, Gersak B, Schwarz AK, Esch S, Kelle S, et al. Intramyocardial fast-SENC is less impacted by compensatory mechanisms while monitoring cardiotoxic effects of chemotherapy than echocardiography and conventional CMR: the PREFECT study. Eur Heart J. (2020) 41(Supplement_2): ehaa946–1222. doi: 10.1093/ehjci/ehaa946.1222
95. D'Souza M, Nielsen D, Svane IM, Iversen K, Rasmussen PV, Madelaire C, et al. The risk of cardiac events in patients receiving immune checkpoint inhibitors: a nationwide Danish study. Eur Heart J. (2021) 42:1621–31. doi: 10.1093/eurheartj/ehaa884
96. Zhang L, Awadalla M, Mahmood SS, Nohria A, Hassan MZ, Thuny F, et al. Cardiovascular magnetic resonance in immune checkpoint inhibitor-associated myocarditis. Eur Heart J. (2020) 41:1733–43. doi: 10.1093/eurheartj/ehaa051
97. Faron A, Isaak A, Mesropyan N, Reinert M, Schwab K, Sirokay J, et al. Cardiac MRI depicts immune checkpoint inhibitor–induced myocarditis: a prospective study. Radiology. (2021) 301:602–9. doi: 10.1148/radiol.2021210814
98. Arbune A, Soufer A, Baldassarre LA. Clinical and advanced MRI techniques for detection of checkpoint inhibitor associated myocarditis. Curr Cardiovasc Imag Rep. (2020) 13:1–10. doi: 10.1007/s12410-019-9521-5
99. Higgins AY, Arbune A, Soufer A, Ragheb E, Kwan JM, Lamy J, et al. Left ventricular myocardial strain and tissue characterization by cardiac magnetic resonance imaging in immune checkpoint inhibitor associated cardiotoxicity. PLoS ONE. (2021) 16:e0246764. doi: 10.1371/journal.pone.0246764
100. Palaskas N, Lopez-Mattei J, Durand JB, Iliescu C, Deswal A. Immune checkpoint inhibitor myocarditis: pathophysiological characteristics, diagnosis, and treatment. J Am Heart Assoc. (2020) 9:e013757. doi: 10.1161/JAHA.119.013757
101. Mukhopadhyay P, Rajesh M, Bátkai S, Kashiwaya Y, Hasko G, Liaudet L, et al. Role of superoxide, nitric oxide, and peroxynitrite in doxorubicin-induced cell death in vivo and in vitro. Am J Physiol Heart Circul Physiol. (2009) 296:H1466–83. doi: 10.1152/ajpheart.00795.2008
102. Guglin M, Krischer J, Tamura R, Fink A, Bello-Matricaria L, McCaskill-Stevens W, et al. Randomized trial of lisinopril versus carvedilol to prevent trastuzumab cardiotoxicity in patients with breast cancer. J Am Coll Cardiol. (2019) 73:2859–68. doi: 10.1016/j.jacc.2019.03.495
103. Sawicki KT, Sala V, Prever L, Hirsch E, Ardehali H, Ghigo A. Preventing and treating anthracycline cardiotoxicity: new insights. Annu Rev Pharmacol Toxicol. (2021) 61:3–8. doi: 10.1146/annurev-pharmtox-030620-104842
104. Räsänen M, Degerman J, Nissinen TA, Miinalainen I, Kerkelä R, Siltanen A, et al. VEGF-B gene therapy inhibits doxorubicin-induced cardiotoxicity by endothelial protection. Proc Natl Acad Sci USA. (2016) 113:13144–9. doi: 10.1073/pnas.1616168113
105. Dash R, Chung J, Chan T, Yamada M, Barral J, Nishimura D, et al. A molecular MRI probe to detect treatment of cardiac apoptosis in vivo. Magn Reson Med. (2011) 66:1152–62. doi: 10.1002/mrm.22876
106. Reinfeld BI, Madden MZ, Wolf MM, Chytil A, Bader JE, Patterson AR, et al. Cell-programmed nutrient partitioning in the tumour microenvironment. Nature. (2021) 593:282–8. doi: 10.1038/s41586-021-03442-1
107. Makunts T, Saunders IM, Cohen I. v., Li M, Moumedjian T, Issa MA, et al. Myocarditis occurrence with cancer immunotherapy across indications in clinical trial and post-marketing data. Sci Rep. (2021) 11:1–15. doi: 10.1038/s41598-021-96467-5
108. Pan EY, Merl MY, Lin K. The impact of corticosteroid use during anti-PD1 treatment. J Oncol Pharm Pract. (2020) 26:814–22. doi: 10.1177/1078155219872786
109. Maslov D, Tawagi K, Kc M, Simenson V, Yuan H, Parent C, et al. Timing of steroid initiation and response rates to immune checkpoint inhibitors in metastatic cancer. J ImmunoTherapy Cancer. (2021) 9:2312–6. doi: 10.1136/jitc-2020-002261
110. Ricciuti B, Dahlberg SE Adeni A, Sholl LM, Nishino M, Awad MM. Immune checkpoint inhibitor outcomes for patients with non-small-cell lung cancer receiving baseline corticosteroids for palliative versus nonpalliative indications. J Clin Oncol. (2019) 37:1927–34. doi: 10.1200/JCO.19.00189
111. Poels K, van Leent MMT, Boutros C, Tissot H, Roy S, Meerwaldt AE, et al. Immune checkpoint inhibitor therapy aggravates T cell–driven plaque inflammation in atherosclerosis. JACC: CardioOncology. (2020) 2:599–610. doi: 10.1016/j.jaccao.2020.08.007
112. Newman JL, Stone JR. Immune checkpoint inhibition alters the inflammatory cell composition of human coronary artery atherosclerosis. Cardiovasc Pathol. (2019) 43:107148. doi: 10.1016/j.carpath.2019.107148
113. Chaar M, Kamta J, Ait-Oudhia S. Mechanisms, monitoring, and management of tyrosine kinase inhibitors–associated cardiovascular toxicities. OncoTargets Therapy. (2018). 11: 6227–37. doi: 10.2147/OTT.S170138
114. Jain P, Gutierrez J, Guha A, Jain C, Patil N, Shen T, et al. 641 incidence and risk factors for stroke associated with immune checkpoint inhibitor therapy in cancer patients using real-world clinical data. J ImmunoTherapy Cancer. (2020) 8(Suppl 3):A677–8. doi: 10.1136/jitc-2020-SITC2020.0641
115. Champion SN, Stone JR. Immune checkpoint inhibitor associated myocarditis occurs in both high-grade and low-grade forms. Modern Pathol. (2020) 33:99–108. doi: 10.1038/s41379-019-0363-0
116. Fitzgerald AA, Weiner LM. The role of fibroblast activation protein in health and malignancy. Cancer Metastasis Rev. (2020) 39:783–803. doi: 10.1007/s10555-020-09909-3
117. Kratochwil C, Flechsig P, Lindner T, Abderrahim L, Altmann A, Mier W, et al. 68Ga-FAPI PET/CT: tracer uptake in 28 different kinds of cancer. J Nuclear Med. (2019) 60:801–5. doi: 10.2967/jnumed.119.227967
118. Varasteh Z, Mohanta S, Robu S, Braeuer M, Li Y, Omidvari N, et al. Molecular imaging of fibroblast activity after myocardial infarction using a 68Ga-labeled fibroblast activation protein inhibitor, FAPI-04. J Nuclear Med. (2019) 60:1743–9. doi: 10.2967/jnumed.119.226993
119. Kessler L, Kupusovic J, Ferdinandus J, Hirmas N, Umutlu L, Zarrad F, et al. Visualization of fibroblast activation after myocardial infarction using 68Ga-FAPI PET. Clin Nucl Med. (2021) 46:810–3. doi: 10.1097/RLU.0000000000003745
120. Natarajan A, Patel CB, Habte F, Gambhir SS. Dosimetry prediction for clinical translation of 64Cu-pembrolizumab ImmunoPET targeting human PD-1 expression. Sci Rep. (2018) 8:1–11. doi: 10.1038/s41598-017-19123-x
121. Dohy Z, Szabo L, Toth A, Czimbalmos C, Horvath R, Horvath V, et al. Prognostic significance of cardiac magnetic resonance-based markers in patients with hypertrophic cardiomyopathy. Int J Cardiovasc Imag. (2021) 37:2027–36. doi: 10.1007/s10554-021-02165-8
122. Kamp NJ, Chery G, Kosinski AS, Desai MY, Wazni O, Schmidler GS, et al. Risk stratification using late gadolinium enhancement on cardiac magnetic resonance imaging in patients with hypertrophic cardiomyopathy: a systematic review and meta-analysis. Prog Cardiovasc Dis. (2021) 66:10–6. doi: 10.1016/j.pcad.2020.11.001
123. Papavassiliu T, Germans T, Flüchter S, Doesch C, Suriyakamar A, Haghi D, et al. CMR findings in patients with hypertrophic cardiomyopathy and atrial fibrillation. J Cardiovasc Magn Reson. (2009) 11:1–9. doi: 10.1186/1532-429X-11-34
124. Jordà P, García-Álvarez A. Hypertrophic cardiomyopathy: sudden cardiac death risk stratification in adults. Glob Cardiol Sci Pract. (2018) 2018:25. doi: 10.21542/gcsp.2018.25
125. Franco A, Javidi S, Ruehm SG. Delayed myocardial enhancement in cardiac magnetic resonance imaging. J Radiol Case Rep. (2015) 9:6. doi: 10.3941/jrcr.v9i6.2328
126. Caravan P, Yang Y, Zachariah R, Schmitt A, Mino-Kenudson M, Chen HH, et al. Molecular magnetic resonance imaging of pulmonary fibrosis in mice. Am J Respir Cell Mol Biol. (2013) 49:1120–6. doi: 10.1165/rcmb.2013-0039OC
127. Farrar CT, Gale EM, Kennan R, Ramsay I, Masia R, Arora G, et al. CM-101: type I collagen-targeted MR imaging probe for detection of liver fibrosis. Radiology. (2018) 287:581–9. doi: 10.1148/radiol.2017170595
128. Li Z, Lu B, Lin J, He S, Huang L, Wang Y, et al. A Type I collagen-targeted mr imaging probe for staging fibrosis in Crohn's disease. Front Mol Biosci. (2021) 8:1–11. doi: 10.3389/fmolb.2021.762355
129. Gupta A, Day JR, Streiff MB, Takemoto C, Jung K, Abro C, et al. Mortality and associated comorbidities among patients hospitalized for deep vein thrombosis and pulmonary embolism in the United States: results from a nationally representative database. Blood. (2020) 136(Supplement 1):39–40. doi: 10.1182/blood-2020-136867
130. Razak NBA, Jones G, Bhandari M, Berndt MC, Metharom P. Cancer-associated thrombosis: An overview of mechanisms, risk factors, and treatment. Cancers. (2018) 10:380. doi: 10.3390/cancers10100380
131. Debbie Jiang MD, Alfred Ian Lee MD. Thrombotic risk from chemotherapy and other cancer therapies. Cancer Treat Res. (2019) 179:87-101. doi: 10.1007/978-3-030-20315-3_6
132. Moik F, Chan WSE, Wiedemann S, Hoeller C, Tuchmann F, Aretin MB, et al. Incidence, risk factors, and outcomes of venous and arterial thromboembolism in immune checkpoint inhibitor therapy. Blood. (2021) 137:1669–78. doi: 10.1182/blood.2020007878
133. Meyer G. Effective diagnosis and treatment of pulmonary embolism: Improving patient outcomes. Arch Cardiovasc Dis. (2014) 107:406–14. doi: 10.1016/j.acvd.2014.05.006
134. Klok FA, Zondag W, van Kralingen KW, van Dijk APJ, Tamsma JT, Heyning FH, et al. Patient outcomes after acute pulmonary embolism: a pooled survival analysis of different adverse events. Am J Respir Crit Care Med. (2010) 181:501–6. doi: 10.1164/rccm.200907-1141OC
135. Kim C, Lee JS, Han Y, Chae SY, Jin S, Sung C, et al. Glycoprotein IIb/IIIa receptor imaging with 18 F-GP1 PET for acute venous thromboembolism: An open-label, nonrandomized, phase 1 study. J Nucl Med. (2019) 60:244–9. doi: 10.2967/jnumed.118.212084
136. Dávila-Román VG, Vedala G, Herrero P, De Las Fuentes L, Rogers JG, Kelly DP, et al. Altered myocardial fatty acid and glucose metabolism in idiopathic dilated cardiomyopathy. J Am College Cardiol. (2002) 40:271–7. 01967-8 doi: 10.1016/S0735-1097(02)01967-8
137. Nelson SJ, Kurhanewicz J, Vigneron DB, Larson PEZ, Harzstark AL, Ferrone M, et al. Metabolic imaging of patients with prostate cancer using hyperpolarized [1-13C]pyruvate. Sci Transl Med. (2013) 5:198ra108. doi: 10.1126/scitranslmed.3006070
138. ClinicalTrials.gov. Magnetic Resonance Imaging (MRI) With Hyperpolarized Pyruvate (13C) as Diagnostic Tool in Advanced Prostate Cancer. San Francisco, CA (2020). Available online at: ClinicalTrials.gov
139. ClinicalTrials.gov. Hyperpolarized 13C Pyruvate MRI for Treatment Response Assessment in Patients With Locally Advanced or Metastatic Pancreatic Cancer. ClinicalTrials.gov.
140. van Bilsen M, van Nieuwenhoven FA, van der Vusse GJ. Metabolic remodelling of the failing heart: Beneficial or detrimental? Cardiovasc Res. (2009). 81:420–8. doi: 10.1093/cvr/cvn282
141. Dash R, Chan T, Yamada M, Paningbatan M, Myagmar B-E, Swigart P, et al. Cardiac magnetic resonance of targeted annexin-iron oxide labeling detects cardiac cell death in vivo after doxorubicin and myocardial infarction. J Cardiovasc Magn Reson. (2009) 11:1–316. doi: 10.1186/1532-429X-11-S1-O8
142. Ruehm SG, Corot C, Vogt P, Kolb S, Debatin JF. Magnetic resonance imaging of atherosclerotic plaque with ultrasmall superparamagnetic particles of iron oxide in hyperlipidemic rabbits. Circulation. (2001) 103:415–22. doi: 10.1161/01.CIR.103.3.415
143. Trivedi RA, Mallawarachi C, U-King-Im JM, Graves MJ, Horsley J, Goddard MJ, et al. Identifying inflamed carotid plaques using in vivo USPIO-enhanced MR imaging to label plaque macrophages. Arterioscl Thromb Vascul Biol. (2006) 26:1601–6. doi: 10.1161/01.ATV.0000222920.59760.df
144. Banerjee SR, Pomper MG. Clinical applications of Gallium-68. Appl Radiat Isotop. (2013) 76:2–13. doi: 10.1016/j.apradiso.2013.01.039
145. Nauffal V, Achille P di, Klarqvist MDR, Cunningham JW, Pirruccello JP, Weng L-C, et al. Genetics of myocardial interstitial fibrosis in the human heart and association with disease running title: genetic and phenotypic associations of myocardial interstitial fibrosis. medRxiv. doi: 10.1101/2021.11.05.21265953
146. Sharma A, Burridge PW, McKeithan WL, Serrano R, Shukla P, Sayed N, et al. High-throughput screening of tyrosine kinase inhibitor cardiotoxicity with human induced pluripotent stem cells. Sci Transl Med. (2017) 9: eaaf2584. doi: 10.1126/scitranslmed.aaf2584
147. Grafton F, Ho J, Ranjbarvaziri S, Farshidfar F, Budan A, Steltzer S, et al. Deep learning detects cardiotoxicity in a high-content screen with induced pluripotent stem cell-derived cardiomyocytes. Elife. (2021) 10:e68714. doi: 10.7554/eLife.68714.sa2
148. Cai C, Guo P, Zhou Y, Zhou J, Wang Q, Zhang F, et al. Deep learning-based prediction of drug-induced cardiotoxicity. J Chem Inf Model. (2019) 59:1073–84. doi: 10.1021/acs.jcim.8b00769
149. Chaix MA, Parmar N, Kinnear C, Lafreniere-Roula M, Akinrinade O, Yao R, et al. Machine learning identifies clinical and genetic factors associated with anthracycline cardiotoxicity in pediatric cancer survivors. JACC: CardioOncology. (2020) 2:690–706. doi: 10.1016/j.jaccao.2020.11.004
150. Mamoshina P, Bueno-Orovio A, Rodriguez B. Dual transcriptomic and molecular machine learning predicts all major clinical forms of drug cardiotoxicity. Front Pharmacol. (2020) 11:639. doi: 10.3389/fphar.2020.00639
151. Zhou Y, Hou Y, Hussain M, Brown SA, Budd T, Tang WW, et al. Machine learning–based risk assessment for cancer therapy–related cardiac dysfunction in 4300 longitudinal oncology patients. J Am Heart Assoc. (2020) 9:e019628. doi: 10.1161/JAHA.120.019628
152. Heilbroner SP, Few R, Neilan TG, Mueller J, Chalwa J, Charest F, et al. Predicting cardiac adverse events in patients receiving immune checkpoint inhibitors: a machine learning approach. J ImmunoTherapy Cancer. (2021) 9:2545. doi: 10.1136/jitc-2021-002545
153. Curigliano G, Lenihan D, Fradley M, Ganatra S, Barac A, Blaes A, et al. Management of cardiac disease in cancer patients throughout oncological treatment: ESMO consensus recommendations. Ann Oncol. (2020) 31:171–90. doi: 10.1016/j.annonc.2019.10.023
154. Attia ZI, Kapa S, Lopez-Jimenez F, McKie PM, Ladewig DJ, Satam G, et al. Screening for cardiac contractile dysfunction using an artificial intelligence–enabled electrocardiogram. Nat Med. (2019) 25:70–4. doi: 10.1038/s41591-018-0240-2
155. Yao X, Rushlow DR, Inselman JW, McCoy RG, Thacher TD, Behnken EM, et al. Artificial intelligence–enabled electrocardiograms for identification of patients with low ejection fraction: a pragmatic, randomized clinical trial. Nat Med. (2021) 2021:27815-9. doi: 10.1038/s41591-021-01335-4
156. Attia ZI, Noseworthy PA, Lopez-Jimenez F, Asirvatham SJ, Deshmukh AJ, Gersh BJ, et al. An artificial intelligence-enabled ECG algorithm for the identification of patients with atrial fibrillation during sinus rhythm: a retrospective analysis of outcome prediction. Lancet. (2019) 394:861–7. doi: 10.1016/S0140-6736(19)31721-0
157. Güntürkün F, Akbilgic O, Davis RL, Armstrong GT, Howell RM, Jefferies JL, et al. Artificial intelligence–assisted prediction of late-onset cardiomyopathy among childhood cancer survivors. JCO Clin Cancer Inform. (2021) 5:459–68. doi: 10.1200/CCI.20.00176
158. Cardinale D, Iacopo F, Cipolla CM. Cardiotoxicity of anthracyclines. Front Cardiovasc Med. (2020) 7:26. doi: 10.3389/fcvm.2020.00026
159. Ganatra S, Sharma A, Shah S, Chaudhry GM, Martin DT, Neilan TG, et al. Ibrutinib-associated atrial fibrillation. JACC: Clin Electrophysiol. (2018) 4:1491–500. doi: 10.1016/j.jacep.2018.06.004
160. Ouyang D, He B, Ghorbani A, Yuan N, Ebinger J, Langlotz CP, et al. Video-based AI for beat-to-beat assessment of cardiac function. Nature. (2020) 580:252–6. doi: 10.1038/s41586-020-2145-8
161. Ghorbani A, Ouyang D, Abid A, He B, Chen JH, Harrington RA, et al. Deep learning interpretation of echocardiograms. NPJ Digital Med. (2020) 3:1–10. doi: 10.1038/s41746-019-0216-8
162. Goto S, Mahara K, Beussink-Nelson L, Ikura H, Katsumata Y, Endo J, et al. Artificial intelligence-enabled fully automated detection of cardiac amyloidosis using electrocardiograms and echocardiograms. Nat Commun. (2021) 12:1–12. doi: 10.1038/s41467-021-22877-8
163. Eng D, Chute C, Khandwala N, Rajpurkar P, Long J, Shleifer S, et al. Automated coronary calcium scoring using deep learning with multicenter external validation. NPJ Digital Medicine. (2021) 4:1–13. doi: 10.1038/s41746-021-00460-1
164. Zeleznik R, Foldyna B, Eslami P, Weiss J, Alexander I, Taron J, et al. Deep convolutional neural networks to predict cardiovascular risk from computed tomography. Nat Commun. (2021) 12:1–9. doi: 10.1038/s41467-021-20966-2
165. Bai W, Sinclair M, Tarroni G, Oktay O, Rajchl M, Vaillant G, et al. Automated cardiovascular magnetic resonance image analysis with fully convolutional networks. J Cardiovasc Magn Reson. (2018) 20:1–12. doi: 10.1186/s12968-018-0471-x
166. de Souza TF, Silva TQ, Antunes-Correa L, Drobni ZD, Costa FO, Dertkigil SS, et al. Cardiac magnetic resonance assessment of right ventricular remodeling after anthracycline therapy. Sci Rep. (2021) 11:1. doi: 10.1038/s41598-021-96630-y
167. Muehlberg F, Funk S, Zange L, von Knobelsdorff-Brenkenhoff F, Blaszczyk E, Schulz A, et al. Native myocardial T1 time can predict development of subsequent anthracycline-induced cardiomyopathy. ESC Heart Failure. (2018) 5:620–9. doi: 10.1002/ehf2.12277
168. Betancur J, Commandeur F, Motlagh M, Sharir T, Einstein AJ, Bokhari S, et al. Deep learning for prediction of obstructive disease from fast myocardial perfusion SPECT: a multicenter study. JACC: Cardiovasc Imag. (2018) 11:1654–63. doi: 10.1016/j.jcmg.2018.01.020
169. Kagiyama N, Shrestha S, Farjo PD, Sengupta PP. Artificial intelligence: practical primer for clinical research in cardiovascular disease. J Am Heart Assoc. (2019) 8:e012788. doi: 10.1161/JAHA.119.012788
170. Dey D, Slomka PJ, Leeson P, Comaniciu D, Shrestha S, Sengupta PP, et al. Artificial intelligence in cardiovascular imaging: JACC state-of-the-art review. J Am Coll Cardiol. (2019) 73:1317–35. doi: 10.1016/j.jacc.2018.12.054
171. Antoniades C, Oikonomou EK. Artificial intelligence in cardiovascular imaging—principles, expectations, and limitations. Eur Heart J. (2021). doi: 10.1093/eurheartj/ehab678. [Epub ahead of print].
172. Bonsu J, Charles L, Guha A, Awan F, Woyach J, Yildiz V, et al. Representation of patients with cardiovascular disease in pivotal cancer clinical trials. Circulation. (2019) 139:2594–6. doi: 10.1161/CIRCULATIONAHA.118.039180
173. Petch J, Di S, Nelson W. Opening the black box: the promise and limitations of explainable machine learning in cardiology. Can J Cardiol. (2021) 38:204–13. doi: 10.1016/j.cjca.2021.09.004
174. Dagogo-Jack I, Shaw AT. Tumour heterogeneity and resistance to cancer therapies. Nat Rev Clin Oncol. (2018) 15:81–94. doi: 10.1038/nrclinonc.2017.166
175. Noseworthy PA, Attia ZI, Brewer LC, Hayes SN, Yao X, Kapa S, et al. Assessing and mitigating bias in medical artificial intelligence: the effects of race and ethnicity on a deep learning model for ECG analysis. Circul Arrhyth Electrophysiol. (2020) 13:e007988. doi: 10.1161/CIRCEP.119.007988
176. Di Noto T, von Spiczak J, Mannil M, Gantert E, Soda P, Manka R, et al. Radiomics for distinguishing myocardial infarction from myocarditis at late gadolinium enhancement at MRI: Comparison with subjective visual analysis. Radiol Cardiothor Imag. (2019) 1:e180026. doi: 10.1148/ryct.2019180026
177. Kolossváry M, Maurovich-Horvat P. Radiomics: the link between radiology and histology? Circul Cardiovasc Imag. (2019) 12:e009990. doi: 10.1161/CIRCIMAGING.119.009990
178. Oikonomou EK, Williams MC, Kotanidis CP, Desai MY, Marwan M, Antonopoulos AS, et al. A novel machine learning-derived radiotranscriptomic signature of perivascular fat improves cardiac risk prediction using coronary CT angiography. Eur Heart J. (2019) 40:3529–43. doi: 10.1093/eurheartj/ehz592
179. Häggström I, Schmidtlein CR, Campanella G, Fuchs TJ. DeepPET: A deep encoder–decoder network for directly solving the PET image reconstruction inverse problem. Med Image Anal. (2019) 54:253–62. doi: 10.1016/j.media.2019.03.013
180. Cui J, Liu X, Wang Y, Liu H. Deep reconstruction model for dynamic PET images. PLoS ONE. (2017) 12:e0184667. doi: 10.1371/journal.pone.0184667
181. Kaplan S, Zhu YM. Full-dose PET image estimation from low-dose PET image using deep learning: a pilot study. J Digit Imaging. (2019) 32:773–8. doi: 10.1007/s10278-018-0150-3
182. Ouyang J, Chen KT, Gong E, Pauly J, Zaharchuk G. Ultra-low-dose PET reconstruction using generative adversarial network with feature matching and task-specific perceptual loss. Med Phys. (2019) 46:3555–64. doi: 10.1117/12.2512946
183. Zhang Q, Burrage MK, Lukaschuk E, Shanmuganathan M, Popescu IA, Nikolaidou C, et al. Toward replacing late gadolinium enhancement with artificial intelligence virtual native enhancement for gadolinium-free cardiovascular magnetic resonance tissue characterization in hypertrophic cardiomyopathy. Circulation. (2021) 144:589–9. doi: 10.1161/CIRCULATIONAHA.121.054432
Keywords: cardiotoxicity, cardiovascular imaging, big data, cancer therapy-associated cardiotoxicity, molecular imaging
Citation: Kwan JM, Oikonomou EK, Henry ML and Sinusas AJ (2022) Multimodality Advanced Cardiovascular and Molecular Imaging for Early Detection and Monitoring of Cancer Therapy-Associated Cardiotoxicity and the Role of Artificial Intelligence and Big Data. Front. Cardiovasc. Med. 9:829553. doi: 10.3389/fcvm.2022.829553
Received: 06 December 2021; Accepted: 12 January 2022;
Published: 15 March 2022.
Edited by:
Chun Liu, Stanford University, United StatesReviewed by:
Ian Chen, VA Palo Alto Health Care System, United StatesConcetta Zito, University of Messina, Italy
Copyright © 2022 Kwan, Oikonomou, Henry and Sinusas. This is an open-access article distributed under the terms of the Creative Commons Attribution License (CC BY). The use, distribution or reproduction in other forums is permitted, provided the original author(s) and the copyright owner(s) are credited and that the original publication in this journal is cited, in accordance with accepted academic practice. No use, distribution or reproduction is permitted which does not comply with these terms.
*Correspondence: Albert J. Sinusas, YWxiZXJ0LnNpbnVzYXMmI3gwMDA0MDt5YWxlLmVkdQ==