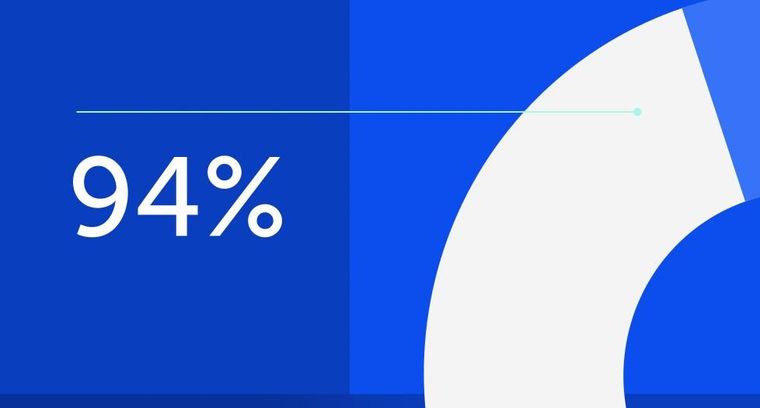
94% of researchers rate our articles as excellent or good
Learn more about the work of our research integrity team to safeguard the quality of each article we publish.
Find out more
ORIGINAL RESEARCH article
Front. Cardiovasc. Med., 08 April 2022
Sec. Atherosclerosis and Vascular Medicine
Volume 9 - 2022 | https://doi.org/10.3389/fcvm.2022.826495
This article is part of the Research TopicInnovations in Thoracic Aortic Aneurysm: Intersections of mechanistic discovery, epidemiology and therapeuticsView all 5 articles
Heterozygous, loss of function mutations in positive regulators of the Transforming Growth Factor-β (TGF-β) pathway cause hereditary forms of thoracic aortic aneurysm. It is unclear whether and how the initial signaling deficiency triggers secondary signaling upregulation in the remaining functional branches of the pathway, and if this contributes to maladaptive vascular remodeling. To examine this process in a mouse model in which time-controlled, partial interference with postnatal TGF-β signaling in vascular smooth muscle cells (VSMCs) could be assessed, we used a VSMC-specific tamoxifen-inducible system, and a conditional allele, to inactivate Smad3 at 6 weeks of age, after completion of perinatal aortic development. This intervention induced dilation and histological abnormalities in the aortic root, with minor involvement of the ascending aorta. To analyze early and late events associated with disease progression, we performed a comparative single cell transcriptomic analysis at 10- and 18-weeks post-deletion, when aortic dilation is undetectable and moderate, respectively. At the early time-point, Smad3-inactivation resulted in a broad reduction in the expression of extracellular matrix components and critical components of focal adhesions, including integrins and anchoring proteins, which was reflected histologically by loss of connections between VSMCs and elastic lamellae. At the later time point, however, expression of several transcripts belonging to the same functional categories was normalized or even upregulated; this occurred in association with upregulation of transcripts coding for TGF-β ligands, and persistent downregulation of negative regulators of the pathway. To interrogate how VSMC heterogeneity may influence this transition, we examined transcriptional changes in each of the four VSMC subclusters identified, regardless of genotype, as partly reflecting the proximal-to-distal anatomic location based on in situ RNA hybridization. The response to Smad3-deficiency varied depending on subset, and VSMC subsets over-represented in the aortic root, the site most vulnerable to dilation, most prominently upregulated TGF-β ligands and pro-pathogenic factors such as thrombospondin-1, angiotensin converting enzyme, and pro-inflammatory mediators. These data suggest that Smad3 is required for maintenance of focal adhesions, and that loss of contacts with the extracellular matrix has consequences specific to each VSMC subset, possibly contributing to the regional susceptibility to dilation in the aorta.
Loeys-Dietz syndrome (LDS) is an autosomal dominant connective tissue disorder characterized by skeletal, ocular, and cardiovascular manifestations including highly penetrant and aggressive aneurysms (1, 2). LDS is caused by heterozygous, inactivating mutations in positive regulators of the TGF-β signaling pathway (1–6). TGF-β ligands bind to TGF-β receptor type II, which recruits and phosphorylates TGF-β receptor type I (TβRI). Upon activation, TβRI phosphorylates the receptor regulated Smad proteins, Smad2 and Smad3. Phosphorylated Smad2 and Smad3 can then bind to Smad4 and translocate to the nucleus, where they regulate the expression of TGF-β responsive genes (7). Heterozygous loss-of-function mutations in the ligands, receptors, and positive intracellular signaling mediators have been associated with LDS and result in an array of phenotypic manifestations, typically including aggressive aortic aneurysm (8). These include mutations in SMAD3, the vast majority of which are missense or frameshift mutations in the MH2 domain (9), which disrupt its ability to interact with both effectors and regulators, including the TGF-β receptors and Smad4 (10). This domain is essential for Smad-dependent transcriptional regulation and loss of function mutations in this region result in impaired transcriptional response to TGF-β signaling (11). Complete abrogation of TGF-β signaling in vascular smooth muscle cells (VSMCs), either prenatally or postnatally, is severely deleterious to aortic wall homeostasis (12–14) and results in mechanical failure and dissections in mouse models. However, in both LDS and other familial non-syndromic forms of aortic disease caused by mutations in positive effectors of the pathway (15), signaling output is only partly impaired, leaving open the possibility that compensatory mechanisms may restore or even upregulate signaling output in specific in vivo contexts, which is consistent with observations made in both patients and mouse models (1–5, 16, 17). The timing, the cell type-specific consequences, and the adaptive or maladaptive nature of this secondary response during disease progression remains unclear (18). In this study, we examine the aortic phenotype and smooth muscle-specific transcriptional changes in a mouse model with bi-allelic smooth muscle-specific postnatal Smad3 deletion. In order to assess whether aortic dilation, and associated compensatory signaling upregulation, could be established independently of developmentally sensitive processes, bi-allelic Smad3 deletion was induced at 6-weeks of age, a time when perinatal matrix deposition and associated phenotypic changes is considered to be completed (18, 19). In addition, we used this controllable system to assess both early and late transcriptional changes that occur in vivo upon Smad3 inactivation and examined the smooth muscle specific transcriptional consequences of this intervention at either 10- or 18-weeks post-deletion, when aortic root dilation is undetectable or moderate, respectively.
All animal experiments adhered to protocols approved by the Animal Care and Use Committee at Johns Hopkins University School of Medicine. All mice were backcrossed to 129S6/SvEv mice (Taconic, 129SVE). Mice were housed in a pathogen-free facility with free access to standard chow and water, with a light/dark cycle of 10/14 h. Mice bearing CAG-Sun1/sfGFP (20) (The Jackson Lab, 021039) tracer allele with either a Mef2cSHF (21) (gifted by the K.R. Chien lab at the Cardiovascular Research Center, Massachusetts General Hospital, Boston, Massachusetts, USA) or Wnt1-Cre2 (22) (The Jackson Laboratory, 003829) were used for lineage tracing for bulk RNA- transcriptomic analysis. Smad3+/+ and Smad3lox/lox (23) (a kind gift of Dr. Matzuk, Baylor College of Medicine) with Myh11-CreER (24) (The Jackson Laboratory, 019079), some bearing the EGFP-L10a (25) (The Jackson Lab, 024750) conditional tracer allele, were injected with tamoxifen (2 mg/day) (MilliporeSigma, T5648) for five consecutive days starting at 6-weeks of age. As Myh11-CreER is integrated on the Y chromosome, only male mice were used. No phenotypic differences were observed between mice with and without the conditional EGFP-L10a allele. Smad3+/+; Myh11-CreERmice are referred to as Smad3+/+, while Smad3lox/lox; Myh11-CreER are referred to as Smad3SmKO, independently of the presence of the EGFP-L10a allele. Aortic dimensions of experimental mice were monitored by serial echocardiography using the Visual Sonics Vivo 2100 machine and a 30 mHz probe. Before being euthanized, tail cuff blood pressure measurements were taken for mice using the Visitech BP-2000 Non-Invasive tail cuff device. Mice were euthanized prior to tissue extraction using halothane (Millipore Sigma, H0150000) at a standard concentration (4%; 0.2 ml per liter of container volume).
Aortas were dissected, flash frozen, and stored at −80°C prior to protein isolation. Forty microliters of protein extraction buffer (Full Moon Biosystems, EXB100) containing phosphatase and protease inhibitor tablets (MilliporeSigma, 11836170001 and MilliporeSigma, 4906845001, respectively) and 8 lysis beads (Full Moon Biosystems, LB020) were added to each sample and protein was extracted using an MP Biomedicals FastPrep-24 5G automatic bead homogenizer. Immunoblot was performed using a 4–12% Bis-Tris Criterion XT gel (BioRad, 3450123) run for 1 h 30 mins at ~100 V (50 V for first 30 mins, then increased to 100 V for 30 mins, and 150 V for the final 30 mins). The gel was transferred to a PVDF membrane (BioRad, 1704273) using the BioRad Turbo Blot Transfer Standard 30-min protocol. The membrane was blocked for 45 mins in blocking buffer (LI-COR, 927-70003) diluted 1:1 with 1x PBS at room temperature. The following primary antibodies were used: anti-Smad3 from Invitrogen (MA5-14939), diluted 1:500 in blocking buffer, anti-beta-actin from Cell Signaling Technology (8H10D10) diluted 1:5000. The primary antibodies were diluted in blocking buffer and incubated for 72 h at 4°C. The membrane was washed 3 times for 5 mins in 1x PBS + 0.05% Tween20 (PBST). Appropriate secondary antibodies (LI-COR 926-68072 and 926-32213 diluted 1:10,000 in blocking buffer) were incubated in the dark at room temperature for 60 mins. The membrane was washed as before, 3 times in PBST, followed by 2 washes in 1x PBS before visualizing on the LI-COR Odyssey. For RNA assays, aortas were dissected and placed in 300 μl of TRIzol (ThermoFisher, 15596018) with 6 zirconium beads (OPS Diagnostics, BAWZ 3000-300-23). Samples were lysed using the MP Biomedicals FastPrep-24 5G automatic bead homogenizer. RNA was extracted and purified using the Direct-zol RNA MiniPrep kit (Zymo Research, R2052) according to the manufacturer's instructions. Complementary DNA (cDNA) was generated using the High-Capacity cDNA Reverse Transcription kit (Applied Biosystems, 4368813) as per the manufacturer's protocol. The qPCR was performed using the TaqMan Gene Expression Master Mix (Applied Biosystems, 4369016) and TaqMan probes (Smad3 Mm01170760_m1, Myh11 Mm00443013_m1) and run on the QuantStuido7 Flex with technical and biological replicates. Relative abundance of Smad3 was obtained by normalizing against Myh11 transcript abundance using the formula 2−(Smad3Ct−Myh11Ct).
After euthanasia by halothane inhalation, the heart and thoracic aorta were dissected en bloc and fixed overnight at 4°C in 4% paraformaldehyde (Electron Microscopy Sciences, 15710) in PBS as previously described (16). Samples were then stored overnight at 4°C in 70% ethanol and mounted in 2% agarose in deionized water prior to paraffin embedding. Paraffin blocks were cut into five-micron sections, exposing a longitudinal cross-section of the heart and thoracic aorta. These sections were stained with Verhoeff-van Gieson (VVG) (StatLab, STVGI) to visualize elastic fiber morphology or used for in situ methods as described below.
After euthanasia by halothane inhalation, the heart and thoracic aorta were dissected en bloc and fixed overnight at 4°C in a solution of 0.1M Sodium Cacodylate and 4% paraformaldehyde (Electron Microscopy Sciences, 15710) in deionized water. The next day, samples were transferred to a solution of 30% sucrose and incubated overnight at 4°C. Samples were then embedded in OCT compound (Sciegen, 4586). Each sample was first bathed in OCT then perfused with 50% OCT in deionized water through the left ventricle. Samples where then embedded in OCT in plastic molds on a bed of liquid nitrogen and dry ice and placed at −80°C overnight to freeze completely. OCT blocks were sectioned using a Cryostat to produce 20-micron sections exposing a longitudinal cross-section of the heart and thoracic aorta. Selected slides were fixed with 2.5% Glutaraldehyde followed by 2% Osmium in Cacodylate buffer. Sections were embedded in EPON resin and trimmed to the appropriate region (aortic root or ascending aorta) before sectioning. Sixty nm sections of aortic root or ascending aorta were triple stained with tannic acid, uranyl acetate, and lead citrate. Images were acquired at 120 Kv on a Thermo-Fisher Talos L120C G2 Electron microscope with a 16-megapixel CMOS camera.
For each animal, at least 14 regions were analyzed using FIJI (26). The length of visible elastic fibers was measured using the line tool, and cell protrusions less than 50nm from elastin were counted manually by a blinded researcher. The total number of protrusions counted were normalized to the total measured elastic fiber's length for each animal.
Paraffin embedded sections of Smad3+/+ and Smad3SmKOaortas were deparaffinized and prepared for RNA in situ hybridization using RNAscope probes from Advanced Cell Diagnostics. RNAscope Multiplex Fluorescent Reagent Kit v2 Assay (Catalog No. 323100) was performed as described in the manufacturer's protocol optimized for fixed samples. Probes used included Mm-Enpep (862211), Mm-Mgp (463381), Mm-Notch3 (425171), Mm-Ptprz1 (460991), and Mm-Tes (1092801). Images were acquired on a Zeiss LSM880 Airyscan FAST confocal microscope at 20× magnification. Images are presented as maximal intensity projection and equally adjusted across samples to enhance visualization of information present in the original.
The following protocol for aortic dissociation was adapted from published protocols (27, 28). The aorta was perfused with 5 ml of PBS injected through the left ventricle of the heart. The thoracic aorta from the aortic root to the arch (sinus of Valsalva to the branchpoint of the left subclavian artery) was collected and cut into small pieces and placed into 500 μl of solution A [4.5 ml HBSS (Thermo Fisher Scientific, 14175095), 500 μl 50% D-Trehalose (Quality Biosciences, A611-K480-95), 45 μl RNase inhibitor [20 U/μl] (Applied Biosystems, N8080119)], all at room temperature. One microliter of DyeCycle Violet (DCV) (ThermoFisher, V35003) was added to stain double stranded DNA. After all aortic samples were collected, the tissue was enzymatically digested by adding 500 μl of solution B (5 ml of HBSS, 8 mg Collagenase II) (315 U/mg) (Worthington-Biochem, LS004176), 100 μl of Elastase (MilliporeSigma, E1250), 1 mg of DNase I (2,610 U/mg) (Zymo Research, E1009) and incubating the sample in a thermomixer set to 800 rpm for 30 mins or 1 h at 37°C. After incubation, each sample was gently pushed through a 3 ml syringe with a 21G needle 8 times to mechanically disrupt the tissue. To inhibit the enzymes, 100 μl of 100% FBS (Corning, 35-010-CV) was added to each sample tube. The cells were pelleted at 800× g for 3.5 mins at room temperature. The supernatant was discarded, and cells were resuspended in 500 μl of solution A and 1 ul/ml of DCV. The cell suspension was carefully pipetted through a 40 μm filter cap tube. Propidium iodide (PI) (ThermoFisher Scientific, P1304MP) was added to each sample and cells were sorted by flow cytometry to produce a single cell suspension of DCV+, PIneg cells. Twenty microliters of 1% BSA in HBSS was used as catch buffer. A single-cell suspension of roughly 3,000–8,000 live, nucleated aortic cells per mouse was obtained by Fluorescence Activated Cell Sorting (FACS) (the exact number of cells varied per sample), processed using the 10× Genomics Chromium 3' v3 platform, and sequenced on the Illumina NovaSeq sequencer at a mean depth of approximately 400 million reads per library (corresponding to the recommended 50,000/cell read depth). A total of 66,261 aortic cells from 16 mice were sequenced.
Cell Ranger software (V6 from 10x Genomics) was used to process, align, and aggregate the raw data. Reads were mapped to the mouse mm10 genome that was modified to include the GFP sequence. The scRNA-seq data were filtered using the R package Seurat v4 (29) according to the following settings: >600 genes detected per cell but <6000, >2000 total molecules detected per cell but <50000, and <25% mitochondrial genes per cell. This filtering reduced the total cell number from 66,261 to 50,835 cells for further analysis. For clustering and cell-type specific analysis, the data were normalized using SCTransform, this function employs a negative binomial regression to normalize UMI count data. As samples were collected in several batches, the data were integrated using reciprocal PCA in Seurat (uses FindIntegrationAnchors function with k.anchors set to 20 and IntegrateData function). The data were then subjected to principal component analysis (RunPCA), uniform manifold approximation and projection (RunUMAP), and the FindNeighbors and FindClusters functions in Seurat. Clusters were visualized using the DimPlot function. For differential expression analysis, the raw data (RNA assay) were normalized and scaled using the NormalizeData (log transformation) and ScaleData functions. Cluster defining markers and differentially expressed genes between genotypes were found using the FindMarkers function which utilizes a Wilcoxon rank-sum test to identify differentially expressed genes between two groups of cells. Data were visualized using the DotPlot and FeaturePlot functions.
Analysis was conducted on 558 transcripts, filtered for detection in at least 10% of VSMCs at either 10- or 18-weeks post-deletion and an absolute logarithmic fold-change value (referred henceforth as Log2FC) of 0.2, and with a P-value below 0.05. After filtering of ribosomal and mitochondrial transcripts, 496 genes were submitted for gene over-representation analysis using EnrichR. A link to the full analysis, results and code can be found at this web address (https://maayanlab.cloud/Enrichr/enrich?dataset=e7883d5f07b8ca4112e10cdd8e32ebd6), courtesy of the Maayan lab cloud services (30–32).
The 558 transcripts detected in at least 10% of VSMCs at either 10- or 18- weeks post-deletion, with a minimum 0.2 Log2FC in either direction and a P-value below 0.05, were converted to their human orthologue, if available, for submission to the Pathway Enrichment analysis pipeline in the ReactomeFIVIz (33) package (https://reactome.org/tools/reactome-fiviz) using Cytoscape (34) (Version: 3.9.0). 540 transcripts were successfully mapped to a node, with 176 being unconnected nodes, which were excluded from further analysis. Non-mapped transcripts included gene segments, unmapped symbols, and non-protein coding/lncRNAs with no clear human orthologues. Cluster FI network and Analyze Module functions were used to identify ribosome and mitochondrial clusters, which were converted to single group node prior to executing the “Analyze Network Function, Pathway Enrichment” algorithm in ReactomeFIVIz. Relevant pathways identified by this analysis were visualized in the network using the Cytoscape “bypass” function to manually attribute specific fill-colors to nodes belonging to specific pathways.
Normalized expression data was imported as a table in Cytoscape and used to determine nodes “fill color” based on the value of average Log2FC of experimental samples relative to controls, according to the displayed legend. PathVisio 3.3.0 (35) was used to download and modify WikiPathways (36) of interest. The following WikiPathways were used to generate a merged a custom pathway: www.wikipathways.org/instance/WP523; www.wikipathways.org/instance/WP6; www.wikipathways.org/instance/WP85; www.wikipathways.org/instance/WP113. A similar process was used to overlay expression data on a merged diseased network downloaded from STRING Disease database for LDS1 to 5 (37).
The ClueGO (38) and CluePedia (39) pipeline was used for analysis of both global (only one list uploaded for all VSMCs) and subset-specific (four lists corresponding to each of the four VSMC subsets) transcriptional alterations caused by Smad3 deletion, using the appropriate settings for absolute and relative gene enrichment. In either case, the gene list was compared against four ontologies: GO Biological Function, KEGG, Reactome, and WikiPathways; ribosomal and mitochondrial transcripts were filtered prior to gene enrichment analysis. ClueGO log files for each analysis are provided as Supplementary Material. The individual analysis of VSMC-wide changes was performed on the subset of ~200 misregulated transcripts that displayed an opposite trend of dysregulation at 10- and 18-weeks post-deletion. Subset-specific analysis was conducted on the most dysregulated genes in each subset (regardless of direction) that had Log2FC of at least 0.25 and a nominal P-value of 0.01 after processing in Seurat.
Mice bearing a conditional CAG-Sun1/sfGFP (20) (The Jackson Lab, 021039) tracer allele with either a Mef2cSHF (21) (gifted by the K.R. Chien lab at the Cardiovascular Research Center, Massachusetts General Hospital, Boston, Massachusetts, USA) or Wnt1-Cre2 (22) (The Jackson Laboratory, 003829) allele were sacrificed at 8 weeks of age. The aortic root and ascending aorta were dissected and cleaned with PBS. Minced tissue was transferred to ice-cold HBSS (Thermo Fisher Scientific, 14175095) containing 0.2 U/μl RNase inhibitor (Applied Biosystems, N8080119) and 5% D-trehalose (Quality Biosciences, A611-K480-95) and then digested with 1 mg/ml collagenase II (Worthington-Biochem, LS004176), 100 ul elastase (MilliporeSigma, E1250), and 0.2 mg/ml DNase I (Zymo Research, E1009) in a 37°C rotating water bath for 30 mins with periodic agitation. After further trituration through a 21G needle, the digestion was stopped by addition of 10% Fetal Bovine Serum (FBS) (Corning, 35-011-CV). Cells were pelleted by centrifugation, resuspended in the previously mentioned HBSS solution, and passed through a 40 μm filter-cap tube. Cells were sorted using a DakoCytomation MoFlo at the Bloomberg Flow Cytometry and Immunology Core. Viability was determined by propidium iodide stain and GFP+ cells were sorted into a tube containing TriZol (ThermoFisher Scientific, 15596026). After separation by chloroform and addition of cold 70% ethanol, RNA was extracted using the PicoPure RNA Isolation Kit (ThermoFisher Scientific, KIT0204). Total bulk RNA was normalized according to sorted cell number, reverse transcribed and amplified according to the SmartSeq2 protocol used for single cell sequencing (40). The amplified cDNA was fragmented and barcoded using the Nextera XT DNA Library Preparation Kit (Illumina, FC-131-1024) and the resulting libraries were pooled and sequenced using an Illumina HiSeq2500.
RSEM (v1.2.25) (41) was used to align and create gene and isoform level expression estimates for genes in the mouse genome (10 mm). The function rsem-calculate-expression, using bowtie2 as the aligner, created a series of matrices of expression values that were then compared across groups and compiled into a single matrix. A matrix of gene level expression estimates (TPM), generated through RSEM, was filtered to identify transcripts that had an exclusive pattern of expression across all 3 replicates of either lineage, with at expression threshold of 5 TPM across the expressing lineage. This list of genes and associated expression values was uploaded in iDEP (42) for visualization of normalized expression as a heatmap.
The FindMarkers function in Seurat utilizes the Wilcoxon rank-sum test to determine significantly different transcripts between two groups of cells (i.e., clusters and/or genotypes). The adjusted P-value indicated in supplementary lists of cluster-defining transcripts and differentially expressed transcripts between genotypes is calculated using Bonferroni correction based on the total number of genes in the dataset.
For all other data analysis, statistical analysis and data visualization were performed in Graphpad Prism 9.0. Data were subjected to the Shapiro-Wilk test for normal distribution and F-test for equal variance. Upon verification of normal distribution and equal variance, control and experimental measurements were compared using unpaired, two-tailed Student's t-test. Unless otherwise indicated in figure legend, measurements are presented as “Box and whiskers,” with the whiskers indicating the minimum and maximum and dots representing all data points; horizontal bar in the box plot indicates the median. For comparison of growth curves, data was interpolated with a simple linear regression slope, which were then compared using the “Test whether slopes and intercepts are significantly different” function in Graphpad Prism 9.0. Whenever statistical significance is indicated in a figure, the statistical tests used are included in the figure caption.
To examine the VSMC-specific impact of postnatally impaired TGF-β/Smad signaling in a mouse model in which time-sensitive transcriptional changes could be examined, we bred conditional Smad3flox/flox mice to transgenic mice carrying a tamoxifen-inducible Cre recombinase that is specifically expressed in VSMCs (Myh11-CREER) (23). Smad3flox/floxand Smad3+/+control mice were treated with tamoxifen at 6 weeks of age (Figure 1A). Mice in which Smad3 is specifically deleted in VSMCs are referred to as Smad3SmKO, and controls as Smad3+/+. Smad3 protein and RNA expression was significantly reduced in the aortas of Smad3SmKO mice relative to controls (Supplementary Figure 1). The aortas of control and Smad3SmKO mice were monitored by serial echocardiography from 6 to 24 weeks (Figure 1B). This intervention was sufficient to induce significant dilation of the aortic root, but not the ascending aorta by 24 weeks of age (18 weeks post-deletion) (Figures 1C,D). Aortic dilation was associated with defective aortic wall architecture, which was also more prominent in the aortic root relative to the ascending aorta of the same Smad3SmKO animal (Figure 1E). A smaller cohort of heterozygous Smad3flox/+; Myh11-CREER mice was also analyzed; however, these animals did not develop aortic dilation and were thus not included in further experiments. Dilation of the aortic root in response to Smad3 deletion occurred with no systemic changes in blood pressure when compared to controls at 24 weeks of age (Supplementary Figure 2).
Figure 1. Postnatal deletion of Smad3 in VSMCs induces significant dilation of the aortic root but not ascending aorta. (A) Schematic of postnatal Smad3 deletion in smooth muscle cells using Myh11-CreER. Tamoxifen was administered at 6 weeks of age and mice were sacrificed at 24 weeks of age. Tamoxifen-treated Smad3flox/flox;Myh11-CreER are referred to as Smad3SmKO, while Tamoxifen-treated Smad3+/+;Myh11-CreER are indicated as Smad3+/+. (B) Representative echocardiographic images of Smad3+/+ and Smad3SmKO aortas at 24 weeks of age, blue arrows indicate aortic root dimensions and yellow arrows indicate dimensions of the ascending aorta. Scale bar is 1 mm. (C) Aortic root and ascending aortic growth of control and Smad3SmKO mice from 6 to 24 weeks of age as measured by echocardiography. P-value refers to two tailed Student's t-test. (D) Aortic root and ascending aortic size of Smad3SmKO mice measured by echocardiography at baseline and several intervals post-deletion. The dotted line indicates simple linear-regression, and P-value refers to an analysis of covariance comparing the two slopes conducted on Graphpad. (E) Representative sections of the aortic root and ascending aorta obtained from Smad3+/+ and Smad3SmKO animals and stained with Verhoeff Van Gieson; three individual biological replicates per condition are shown. Scale bar is 50 μm.
In order to investigate the transcriptional changes associated with aortic dilation in Smad3SmKO mice and assess how they may be affected by timing and cellular context, we performed single cell RNA transcriptomic analysis on thoracic aortas of control and Smad3SmKO mice at both 10- and 18-weeks post-deletion (Figure 2A; Supplementary Figure 3), when aortic root dilation is undetectable or moderate (Figure 2B). The segment of aorta dissected for this analysis included the aortic root, proximal and distal ascending aorta as well as the aortic arch, up to the boundary defined by the left subclavian artery. All major aortic cell types were identified using previously defined (27) lineage-specific transcripts (Figures 2C,D). We identified the same population of VSMCs regardless of genotype (Figure 2E). In mice in which VSMCs were traced by expression of a conditional fluorescent reporter, the VSMC cluster included cells expressing the associated transcript (although its expression was low), indicating that these cells expressed the VSMC-specific Myosin Heavy Chain 11 (Myh11)-promoter driven Cre recombinase at the time of tamoxifen administration (Figure 2F). To investigate the effect of VSMC-specific Smad3 deletion overtime we performed differential expression analysis to identify significant differences in transcript expression between control and Smad3SmKO mice at 10- and 18-weeks post deletion (Supplementary Tables 1, 2). 548 transcripts detected in at least 10% of cells, with an average Log2FC of 0.2 (in either direction) and an adjusted P value below 0.05, at either time-point were selected for further analysis (Supplementary Table 3).
Figure 2. Single cell transcriptomic analysis of control and Smad3SmKO mice at 10- and 18-weeks post-deletion. (A) Schematic of postnatal Smad3 deletion by tamoxifen administration at 6 weeks of age and aortic tissue collection for single cell RNA sequencing at 10- and 18-weeks post-deletion. (B) Aortic root size of Smad3SmKO mice at 10- and 18-weeks post-deletion as measured by echocardiography. P-value refers to two tailed Student's t-test. (C) Uniform manifold projection and approximation (UMAP) of aortic cells from Smad3+/+ and Smad3SmKO mice. (D) Dot plot showing lineage specific transcripts used to identify vascular smooth muscle cells (VSMCs), fibroblasts, endothelial cells, and leukocytes. The intensity of the dot's color corresponds to a scaled average expression of each transcript while the size of the dot indicates the percentage of cells in each cluster that express that transcript. (E) UMAP showing the distribution of VSMCs in Smad3+/+ and Smad3SmKO mice. (F) UMAPs showing the distribution of Myh11- and Gfp-expressing cells within the VSMC cluster. Mice with a Gfp lineage-tracer induced by Myh11-CreER were included in this analysis, as such cells expressing Gfp are those that were marked by Myh11-CreER at the time of tamoxifen induced Cre-mediated recombination.
Gene enrichment analysis was performed using EnrichR (30–32), selecting BioPlanet2019 (43), Kyoto Encyclopedia of Genes and Genomes (KEGG) (44), and TTRUST (45) as the databases for comparison. Major pathways significantly dysregulated in Smad3-deficient VSMCs included, as would be expected, those related to TGF-β regulation of the ECM, as well as to Smad3-transcriptional regulation. Brain-Derived Neurotrophic Factor (BDNF) signaling, focal adhesions, regulation of the actin cytoskeleton, and ECM-receptor interaction were also perturbed (Figures 3A,B).
Figure 3. Defective expression of extracellular matrix and focal adhesions components in Smad3-deficient VSMCs. (A) EnrichR gene over-representation analysis, showing the top 3 most significant results in the BioPlanet 2019, KEGG 2021, and TRRUST 2019 databases for genes dysregulated in aortic VSMCs after Smad3 deletion at either time point. (B) Scatter plot visualization of top dysregulated KEGG 2021 pathways in VSMCs after Smad3 deletion. Similar gene sets are clustered together, and larger, black-outlined points represent significantly enriched terms. Clusters were computed using the Leiden algorithm and points plotted on the first two UMAP dimensions by the EnrichR web application. (C) Dysregulated transcripts were converted to their human orthologue and analyzed using the REACTOME Functional Interaction (FI) Gene Set enrichment pipeline, which yielded a main network of approximately 300 transcripts. Each node represents a dysregulated gene in Smad3-deficient VSMCs. Nodes were manually colored-coded based on the main enriched pathway they belonged to, as indicated in the legend. R indicates a REACTOME pathway, K a KEGG and N and NCI Pathway Interaction Database; numbers listed in the legend represent number of transcripts belonging to the indicated group. The same network of dysregulated genes is also shown computationally colored based on corresponding transcript expression at 10- and 18-weeks post-deletion. Genes shown in blue are downregulated and genes shown in red are upregulated in Smad3SmKO relative to controls.
Additional pathways identified as dysregulated included those related to cardiomyopathy, with gene overlap including Tgfb2, Thbs1 (thrombospondin-1, also known as Tsp-1), and Ace (Angiotensin I Converting Enzyme, or ACE), all of which code for factors previously implicated in both vascular and cardiac pathogenesis (46). In particular, both ACE, which is a positive modulator of Angiotensin II signaling, and Thbs1, a multifunctional protein with both pro-inflammatory and cell signaling regulatory functions, have been shown to be upregulated in aneurysm tissue and implicated in its pathogenesis (46). Components of the Relaxin pathway, which signals primarily through its G protein coupled receptor and has been shown to have cardioprotective and anti-fibrotic effects, were also misregulated (47) (Gng11 and Adcy5) (Figure 3B). The full analysis can be accessed at the link provided in the Materials and Methods Section.
In order to visualize network interactions between dysregulated transcripts, and changes in expression as attributes of specific nodes, we also performed a Functional Interaction (FI) gene enrichment analysis using the ReactomeFI (33) plug-in for the network analysis software Cytoscape (34).
This analysis also showed widespread perturbation of the pathways related to ECM organization, focal adhesions, integrins, smooth muscle contraction and TGF-β signaling (Figure 3C). A closer investigation of VSMC-specific transcriptional differences between control and Smad3SmKOmice 10 weeks after Smad3 deletion shows that an early event in the pathogenesis of aortic dilation, and which occurs before aortic dilation is detectable by echographic assessment, is impaired expression of transcripts encoding for adaptor proteins and other actin-binding proteins that connect integrins to the actin cytoskeleton thorough focal adhesion (Fblim1, Tln1, Vcl, Lims2, Actn1, and Flna) (Supplementary Figure 4). Additionally, expression of integrins (Itga1, Itga7, Itga8, and Itga9), elastic fiber components (Eln, Fbln5, and Mfap4), and some but not all smooth muscle contractile genes (Cnn1, Myh11, Mylk, and Myocd) was also impaired (Supplementary Figure 4). Taken together these data suggest that assembly of focal adhesions in Smad3-deficient VSMCs may be defective because of decreased availability of both extracellular and intracellular components, and because of defective force-dependent maturation, which depends on myosin II activity (48). Accordingly, examination of aortic architecture 10 weeks after Smad3 deletion by electron microscopy shows that Smad3-deficient VSMCs form fewer connections and/or lose contact with the elastic lamellae as compared to controls (Figure 4). These early transcriptional changes were also accompanied by decreased expression of negative regulators of TGF-β (Pmepa1, Skil, Nr4a1, Wwp2) all of which participate in negative feedback control of TGF-β signaling by being both target genes and inhibitors of the pathway (49–52) (Supplementary Figure 4).
Figure 4. Transcriptional alterations associate with loss of connections between VSMCs and the elastic lamellae in vivo. Representative electron micrographs of longitudinal sections of the aortic root of Smad3+/+ and Smad3SmKO mice 10 weeks after Smad3 deletion (E, elastic lamellae; SMC, Smooth muscle cell, arrows indicate contact between SMCs and the ECM, note only some connections are labeled to help orient the reader to this feature). Three biological replicates, with three representative images from each animal, per condition are shown. Scale bar is 2 μm. Cell protrusions <50 nm from elastin were counted manually by a blinded researcher. The total number of protrusions counted were normalized to the total measured elastic fiber's length for each animal (Contact/length). P-value refers to two tailed Student's t-test.
The downregulation of transcripts coding for inhibitors of TGF-β/Smad signaling may partly explain why analysis of the expression differences that occur 18 weeks after Smad3-deletion showed a substantial reversal in the direction of change for several transcripts. Although the functional categories of dysregulated transcripts did not vary with time-point analyzed, many transcripts downregulated at the 10-week time point showed either normal expression, or even upregulation, at the 18-week time point (Supplementary Figure 4). Approximately 200 transcripts among those detectable by scRNA sequencing displayed time-sensitive transcriptional deregulation upon Smad3 deletion. These data suggested that the response of VSMCs to impaired Smad3 signaling is dynamic and that compensatory mechanisms, presumably acting on the remaining functional branches of the pathways such as those activated by TGF-β through Smad2, can modify the effect of the initial signaling loss.
To perform a gene enrichment analysis across multiple databases (Gene Ontology -Biological function, KEGG, Reactome and WikiPathways), and visualize the resulting enriched pathways as a functional network, this smaller list of genes was analyzed using ClueGO (38). ClueGO organizes enriched pathways and terms into “ClueGO groups” according to shared number of genes and/or terms related to a shared biological meaning; enriched pathways are then visualized in a network where each node represents a group of genes sharing a similar function (38, 39). Transcripts displaying time-sensitive transcriptional changes after Smad3-deletion in VSMCs represent all the major pathways known to be involved in the pathogenesis of aortic vasculopathies (15, 53), with a preponderance of transcripts being involved in cell-matrix interactions (Figures 5A,B). The results of this analysis are provided in Supplementary Table 4. This transcriptomic analysis suggests that widespread disruption of genes associated with the extracellular matrix and smooth muscle mechanosensing occurs early after Smad3 inactivation, while the onset of dilation is marked by potentially adaptive or maladaptive increases in expression of selected extracellular matrix and focal adhesions components, presumably through compensatory activation of Smad2 and other parallel pathways.
Figure 5. Smad3 deletion causes time-sensitive defects in expression of focal adhesion and ECM components. (A) ClueGO Gene enrichment analysis of transcripts whose direction of change in Smad3SmKO relative to controls is dependent on time elapsed since Smad3 inactivation. 153 transcripts had a negative Log2FC 10 weeks after Smad3 deletion, but a positive Log2FC at 18 weeks, and 105 showed the opposite behavior, for a total of 258 transcripts displaying a time-sensitive transcriptional dysregulation upon Smad3 deletion. Each node represents a group of genes sharing a similar function. The color of the node corresponds to a ClueGO functional group to which each node belongs. Nodes belonging to more than one ClueGO functional group are filled with multiple colors. Asterisks indicate significance as calculated by ClueGO algorithm and corrected using the Benjamini-Hochberg method for multiple testing correction. (B) Custom pathway diagram adapted from WikiPathways WP523, WP6, WP85 and WP113 using the PathVisio 3.0 software, and overlayed with relative expression data using Cytoscape. Each node shows expression of transcripts coding for extracellular matrix components, integrin ligands, integrins, and intracellular focal adhesion components in VSMCs 10 weeks and 18 weeks after Smad3 deletion. Genes shown in blue are downregulated and genes shown in red are upregulated in Smad3SmKO relative to controls. Scale indicates the average Log2FC in expression.
To better understand the contribution of cellular heterogeneity to the global changes observed in Smad3SmKO VSMCs, we investigated the substructure of this cluster. Four VSMC clusters were identified regardless of genotype (Figure 6A), as determined based on the distribution of cluster-defining transcripts (Supplementary Table 5). Cluster-defining transcripts included Mgp, which was enriched in VSMC cluster 4, Notch3, and Enpep enriched in VSMC cluster 3, and Ptprz1 and Tes, enriched in VSMC clusters 1 and 2 (Figure 6B). Visualization of the distribution of cells expressing these cluster-defining transcripts in all VSMCs (Notch3 vs. Ptprz1, Mgp vs. Ptprz1, and Enpep vs. Tes), showed very little overlap in cells expressing Mgp, Notch3 or Enpep and cells expressing Ptprz1 or Tes (Figure 6C). In order to test if these VSMC subclusters reflected the regional distribution along the aorta, we performed RNA in situ hybridization with probes specific for cluster-defining transcripts that showed the most consistent selective distribution across individual samples. Independently of genotype, we observed a gradient of expression for Notch3, Mgp, and Enpep, which were enriched in the aortic root and proximal ascending aorta and Ptprz1 and Tes, which were enriched in the more distal ascending aorta (Figure 6D). Notably, while cells expressing Notch3, Enpep, and Mgp were all enriched in the aortic root, cells expressing these transcripts showed little overlap by UMAP, suggesting that transcriptional differences beyond location further distinguish these cells.
Figure 6. Single cell transcriptomic analysis reveals four subpopulations of VSMCs and a gradient of cluster-defining transcripts that define the proximal to distal location in the murine aorta. (A) Uniform manifold approximation and projection (UMAP) of vascular smooth muscle cells (VSMCs) from Smad3+/+ and Smad3SmKO aortas reveals four subclusters irrespective of genotype. (B) Dot plot showing expression of cluster-defining transcripts for the VSMC subclusters. The intensity of the dot's color corresponds to a scaled average expression of each transcript while the size of the dot indicates the percentage of cells in each cluster that express that transcript. (C) Feature plots showing the distribution of Mgp, Enpep, Notch3, Tes, and Ptprz1 expressing-cells across all VSMC clusters. (D) Representative images of RNA fluorescence in situ hybridization for Notch3, Enpep, Mgp, Tes, and Ptprz1 in 16-week-old control aortas. Insets identify locations shown at higher magnification in subsequent panels. Images were acquired as a tile with x20 magnification. Scale bars are 100 μm and 20 μm as indicated on figure.
The distal-to-proximal distribution of expression of cluster-defining transcripts for VSMC cluster 1 to cluster 4 was reminiscent of the distribution of cardiac neural crest (CNC)- and secondary heart field (SHF)-derived VSMCs in the aorta (54). Having obtained a list of lineage-exclusive transcripts from bulk RNA sequencing of lineage-traced aortic VSMCs of either lineage (Supplementary Table 6), we examined their distribution across the four VSMC subsets identified by scRNA sequencing analysis (Supplementary Figures 5A–D). Among transcripts that were detectable in both datasets, CNC-exclusive transcripts were enriched in VSMC1 and 2, while SHF-exclusive transcripts were enriched in VSMC3 and 4 (Supplementary Figure 5E). However, the correlation was not perfect, and cluster distribution as defined by scRNA sequencing is likely the result of both embryological origin and anatomical location, suggesting that VSMC heterogeneity in the proximal aorta may be better defined by a gradient of location specific determinants rather than simply by lineage-of-origin.
To interrogate how intrinsic VSMC heterogeneity contributes to the dysregulation we observed in all Smad3-deficient VSMCs at 18 weeks post-deletion, we examined transcriptional changes in the four VSMC clusters, which in situ hybridization showed to partly reflect the proximal-to-distal anatomic location. The top 200 dysregulated transcripts for each subset, as defined by absolute Log2FC (agnostic as to the direction of change) and P-value, were analyzed using the multiple-clusters option in ClueGO, which allows for the simultaneous analysis and visualization of both cluster-specific and non-cluster specific pathways (and/or biological terms) in a functional network (Supplementary Table 7). This analysis revealed the existence of both shared and VSMC-cluster specific enriched pathways (Figures 7, 8), with transcripts misregulated in VSMC2 and VSMC4 showing the highest degree of subset-specific gene enrichment (Figure 7). Functional organization of enriched pathways into ClueGO functional groups showed functional segregation of pathways perturbed in VSMC2 and VSMC4, with perturbation of actomyosin structures and focal adhesion machinery being mostly affected in the former and expression of TGF-β dependent extracellular matrix proteins, including elastic fiber components and collagens, in the latter (Figures 8A,B). VSMC1 also showed perturbation of actomyosin structures, in addition to those related to metabolic regulation. Genes prominently misregulated in VSMC3 were related to response to injury (Figures 8A,B). All clusters except VSMC3 showed dysregulation of genes involved in modulation of signaling by transmembrane receptors with Serine/Threonine kinase activity, which includes signaling by TGF-β Receptors (Figures 8A,B). Taken together, these data indicate that perturbation of TGF-β-signaling by Smad3 deletion produces both global and subset-specific responses in VSMCs, which may reflect distinct roles played by each subset in the disease process.
Figure 7. Genes dysregulated in individual VSMC subsets show enrichment for specific pathways. Cluster-specific gene enrichment results as calculated by ClueGO for the top 200 dysregulated transcripts for each VSMC subset. Bar graphs shows the percent of genes belonging to a given term, while the absolute number of genes for each term is indicated as a number next to the corresponding bar. Asterisks refer to the significance of enrichment as calculated by ClueGO algorithm and corrected using the Benjamini-Hochberg method for multiple testing correction.
Figure 8. Functional network of global and subset-specific enriched terms for genes dysregulated in response to Smad3-deficiency in VSMCs. (A) The top 200 dysregulated transcripts for each subset (regardless of direction) were analyzed using the multiple-clusters option in ClueGO, which allows for the simultaneous analysis and visualization of both cluster-specific and non-cluster specific enriched pathways and biological terms in a functional network. In the left panel, enriched nodes are colored based on specificity for a given VSMC subset, while non-specific terms are left grey; on the right panel, network nodes fill color corresponds to the ClueGO functional group to which they belong, with terms belonging to more than one group filled-in by more than one color. Terms and pathways significantly enriched in only one VSMC cluster are identified by the color of the node's border. (B) The distribution of genes per ClueGO functional group for each VSMC subset.
In order to visualize the behavior of the different VSMC subsets in relation to pathways previously identified as involved in hereditary aneurysm, we downloaded networks related to LDS (LDS1 to LDS5) from the STRING Disease database (37). Expression changes in Smad3-deficient VSMCs relative to controls for transcripts coding for proteins in the network were visualized as fill color for each node (Figure 9; Supplementary Figure 6). This analysis revealed striking differences in the behavior of each subset, which included opposite direction of change for transcripts derived from genes critically involved in aneurysmal disease such as Myh11, Acta2, Flna, Smtn, and Tagln (55–59). For example, Rock1 was highly upregulated in VSMC1 and 2 but not in VSMC3 and 4. FlnA, a target gene of TGF-β/Smad signaling (60) that is necessary for vascular homeostasis (57, 61), was downregulated in VSMC1 and 2 but normalized and upregulated in VSMC3 and 4, respectively. Transcripts coding for Tgfb2 and Tgfb3 were upregulated in VSMC3, and those for Ace and Bdnf in VSMC4. Thbs1 was notably upregulated in both VSMC3 and VSMC4 (Figure 9A; Supplementary Figure 4), indicating that these subsets are the major contributor of Thbs1 upregulation observed in the analysis of all VSMCs combined (Figure 5B).
Figure 9. Intrinsic and disease-associated transcriptional signatures in the VSMC subclusters. (A) Networks related to LDS1-5 were downloaded from the STRING Disease database, and overlayed with relative expression data for each VSMC subset using Cytoscape. Genes shown in blue are downregulated and genes shown in red are upregulated in Smad3SmKO relative to controls. Scale indicates the average Log2FC in expression in Smad3-deficient VSMCs relative to controls for transcripts coding for proteins in the network. Size of the node indicates the percent of cells in the subcluster that express a given transcript. Color bar indicates the gradient of cells populating VSMC subsets in the aortic root and proximal ascending aorta (red) and distal ascending aorta and arch (cyan), with VSMC1 cells being most enriched in the distal ascending aorta and arch and VSMC4 cells being most enriched in the aortic root and proximal ascending aorta. (B) Dot plots of protein and non-protein coding transcripts that show VSMC subset-specific behavior. The intensity of the dot's color corresponds to a scaled average expression of each transcript while the size of the dot indicates the percentage of cells in each cluster that express that transcript. Vertical bar indicates the gradient of cells populating VSMC subsets in the aortic root and proximal ascending aorta (red) and distal ascending aorta and arch (cyan).
A more detailed analysis of subset-specific changes in our scRNA dataset (Figure 9B) also shows discrete transcriptional patterns both intrinsic and secondary to Smad3-deletion in each VSMC cluster. Notable differences include the type of ECM transcript present and/or upregulated in response to Smad3 deficiency, relative expression of specific integrins, and expression of injury-associated transcripts. Additionally, while VSMC4 cells upregulated putatively pathogenic transcripts associated with vascular injury (Cd74, Thbs1, Ace, B2m, Cxcl12) (46, 62–65), VSMC1 upregulated putatively protective genes such as those coding for enzymes that provide protection from oxidative stress (Gpx1 and Prdx1) (66, 67). We also noted that, in response to Smad3-deletion, VSMC1 and VSMC2 cells upregulated expression of specific regulators of TGF-β signaling such as Bambi (68), Fkbp1a (69), Ywhaq and Ywhah (whose gene product belongs to the 14-3-3 family of multifunctional adaptor proteins) (70), and Ube2d3 (71). Analysis of long-non-coding RNAs (lncRNAs) detected in our dataset also revealed that differential expression and/or Smad3-deficiency induced up- or downregulation in each subset. For example, VSMC4, and to a lesser extent VSMC3, intrinsically expressed lower levels of Cardiac mesoderm enhancer-associated non-coding RNA (Carmn), a lncRNA previously shown to have a cardiovascular protective effect (72), and higher expression of maternally expressed 3 (Meg3), a lncRNA that regulates VSMC phenotypes and is co-regulator of Smad2/Smad3 transcriptional activity (73, 74).
Although the ultimate combinatorial functional outcome of these subset-specific changes cannot be inferred simply by transcriptional profiling, taken together, these data suggested that the response to the global insult imposed by loss of Smad3 activity has a heterogenous impact on different VSMC subsets and that subset-specific changes, such as local upregulation of transcripts coding for pro-pathogenic factors such as Thbs1 and ACE (75) as well as intrinsically lower expression of protective factors such as the lncRNA Carmn (72), may predispose aortic segments where these cells are most abundant to increased pathogenesis.
Inactivating mutations in genes coding for positive effectors of the TGF-β signaling pathway cause hereditary forms of thoracic aortic disease, including those associated with LDS (2–6, 9, 76–82). The mutational repertoire clearly points to loss of canonical TGF-β signaling as an initiating event in the process that eventually leads to mechanical failure of the aortic wall, dilation, and then aneurysm. However, aneurysmal tissue from both patients and mouse models often shows evidence of either upregulated or normalized TGF-β activity, as measured by levels of Smad2 and Smad3 (Smad2/3) phosphorylation and expression of target genes of the pathway (2–6, 9, 16, 17, 77–80, 83, 84). Although the redundancy and overlapping nature of many signaling pathways in the vascular wall makes it difficult to establish whether upregulation of Smad2/3 phosphorylation and that of target genes (i.e., Ctgf , Serpine1, Col1a1) truly constitutes a signature of TGF-β signaling upregulation, the phenotype observed in tissue at later stages of disease is functionally indistinguishable from a state of “high TGF-β signaling.” Although this secondary compensatory response and associated features are broadly recognized, debate exists as to whether it represents a mostly adaptive, maladaptive, or simply irrelevant feature of aneurysm progression (85, 86).
Understanding the mechanisms and consequences of this transition is complicated by the fact that in mouse models carrying germline aneurysm-causing mutations, the transition from the initial deficiency in TGF-β/Smad signaling to moderate disease and eventual overt disease is entwined with prenatal and perinatal developmental processes that regulate morphogenesis and postnatal remodeling of the aortic wall (87–89). While other laboratories have shown that full postnatal ablation of TGF-β signaling by deletion of the gene coding for TGF-β Receptor II (12, 13, 90) causes quick mechanical failure of the aortic wall, such a severe provocation does not recapitulate the process of disease seen in patients carrying mutations that only partly impair TGF-β signaling output. In the context of LDS-causing mutations, retention of partly and/or fully functional signaling branches leaves open the possibility of secondary signaling upregulation that is not possible when TGF-β Receptor II signaling capability is fully inactivated. Of note, the work of Cook and colleagues in a mouse model of Marfan syndrome strongly indicated that whereas TGF-β signaling had a protective role at early stages of disease, its activation contributed to disease progression at later stages (91), suggesting that a similar process may also apply to aortic pathogenesis in LDS.
On the basis of these considerations, we set out to assess whether partial inhibition of the pathway by postnatal Smad3 inactivation (with TGF-β Receptor still able to activate Smad2) was sufficient to cause disease, and, if so, whether disease progression was also accompanied by a transition to an apparent excessive signaling, presumably mediated by Smad2. Smad3 inactivation at 6-weeks of age led to detectable aortic dilation within approximately 18 weeks, and to apparent defects in the aortic wall ultrastructure within 10 weeks (Figures 1, 2, 4). Although hereditary aneurysm in humans is caused by heterozygous, rather than bi-allelic, loss-of-function mutations in SMAD3 (4), neither tamoxifen-treated Smad3+/flox Myh11CreER nor mice heterozygous for a null Smad3 allele (not shown) developed dilation, suggesting a greater compensatory role for murine Smad2.
Smad3-inactivation causes broad reduction in expression of ECM matrix proteins, including those necessary for assembly and maturation of elastic fibers, as well as that of integrins, focal adhesion adaptor proteins, and selected cytoskeletal proteins (Figure 3; Supplementary Figure 4), all of which was reflected histologically by loss of connections between VSMCs and the elastic lamellae (Figure 4). These observations are consistent with a model in which TGF-β signaling is necessary for expression of genes that modulate assembly and maturation of focal adhesions between VSMCs and elastic lamellae (15, 92–94). This would include genes coding for contractile proteins such as smooth muscle actin (Acta2, also known as α-SMA) and smooth muscle myosin heavy chain (Myh11, also known as SM-MHC), given that myosin-dependent force generation regulates focal adhesion function by provoking tension-dependent conformational alterations in both integrins and associated signaling anchoring proteins (48, 95–98). Although our data is consistent with a model in which TGF-β signaling deficiency leads to dilation by inducing loss of the elastin-contractile unit (92), evidence from mouse models that are deficient in elastin (Eln), the principal component of elastic fibers, indicates that mere loss of connections between VSMCs and elastic lamellae is insufficient to cause aortic dilation. VSMC-specific elastin deficiency results in absence of elastic lamellae and thickening of the ascending aorta but not dilation or aneurysm (99). Additionally, in humans, elastin insufficiency is associated with inherited obstructive arterial disease but not aneurysm (100–107). The subsets of mutations in the elastin ELN gene that are known to cause aortic dilatation and rupture in patients are frameshift mutations predicted to result in abnormal tropoelastin monomer maturation into mature elastin, and thus deposition of abnormal elastin that may trigger cellular responses not caused by simple deficiency (107).
Thus, we favor a model whereby loss of proper contacts between VSMCs and the ECM is necessary but not sufficient to cause aortic dilation, with maladaptive compensatory responses to this initial loss being critical to disease progression. The broad “reversal” in expression of many of the same ECM actomyosin, and focal adhesion components, diminished at the 10-week time point and normalized and upregulated at the 18-week time point (Figures 3, 5), corresponds to the onset of detectable dilation in our mouse model, and seems to indicate that this attempt at compensation is part of the disease process. We propose that abnormal signaling downstream of integrins and focal adhesions, triggered by an irreparably dysfunctional matrix, and possibly imbalanced intracellular repertoire of signaling and adaptor proteins, is a key driver of disease progression. The reversal in expression of many components and regulators of these complexes was also accompanied by increased expression of TGF-β ligands as well as that of activators of the angiotensin II signaling pathway, such as transcripts coding for ACE and Thbs-1, all of which can promote pathogenic remodeling of the extracellular matrix (46, 108–110). Unfortunately, levels of Agtr1a, which codes for the angiotensin II Type I Receptor, a modulator of several pathogenic pathways driving aneurysm progression, were too low to be detected in this single cell transcriptomic analysis (86).
We also examined how VSMC heterogeneity may explain the peculiar vulnerability of the aortic root to dilation, observed both in our mouse model as well as in patients carrying analogous mutations (8). The proximal thoracic aorta is populated by VSMCs derived from cardiac neural crest (CNC) (111) and second heart field (SHF)-derived progenitors (54, 112). Our own work and that of others has shown that these two types of VSMCs respond differently to aneurysm-causing mutations, including those that cause Smad3 deficiency, and suggested the possibility that their peculiar distribution in the aortic root and ascending aorta may underlie regional susceptibility to aneurysmal disease (11, 84, 110, 113–115) (Supplementary Figure 5).
In our analysis, the VSMC clusters identified by scRNA sequencing analysis correlated but did not overlap with embryological lineage (Supplementary Figure 5). Cells expressing cluster-defining transcripts for each of the four VSMC clusters were present within the aortic media and distributed in a gradient along the distal to proximal axis, with cells expressing cluster-defining transcripts for VSMC3 and 4 being most abundant, but not exclusive, to the aortic root, and those expressing cluster-defining transcripts for VSMC1 and 2 being more abundant in the proximal and distal ascending aorta (Figure 6). We interpret the four VSMC clusters as being a function of both intrinsic factors derived from lineage-of-origin and extrinsic factors due to anatomical location, with influences of both blood flow and other environmental factors such as longitudinal and circumferential stretch imposed by heart contraction (116, 117). Although existence of a population of modulated VSMCs has been reported in the context of aneurysm in a mouse model of Marfan Syndrome (110), we did not find this population in our analysis, possibly because aortic dilation, even at 18 weeks, is still too mild in our model and the percent of modulated VSMCs, which has been described to increase with time, is still too low.
Analysis of transcripts misregulated after Smad3 deletion shows both shared and VSMC subset-specific alterations, including transcripts coding for a network of proteins previously shown to be involved in disease progression in LDS (STRING Disease LDS Network, Figure 9; Supplementary Figure 6). This subset-specific behavior may explain discordant findings among laboratories with respect to expression of some of these disease markers, given that assessment of expression, and possibly functional outcome, would be dependent on the precise mix of VSMCs that are assessed. The precise effect that these subset-specific differences have on the disease process are hard to predict simply on the basis of the transcriptional analysis described in this study; it is notable, however, that VSMC subsets more abundant in relatively protected portions of the aorta also express increased levels of likely protective factors, and show a distinct expression pattern for both matrix proteins and modulators of focal adhesions (Figures 7–9), while the converse is true for VSMC subsets more abundant in the aortic root, where disease is more pronounced (Figure 10).
Figure 10. Postnatal Smad3 inactivation in smooth muscle cells elicits a temporally and regionally distinct pathogenic response. Diagram summarizing the major findings of the study. Smad3 inactivation in VSMCs results in a broad reduction in the expression of components of the extracellular matrix and focal adhesions, including integrins and anchoring proteins, and results in loss of connections between VSMCs and elastic lamellae. Later in the disease process, normalization or upregulation of transcripts belonging to the same functional categories associates with upregulation of transcripts coding for putatively pro-pathogenic factors such as Ace, Thbs1, Cxcl12 as well as that of TGF-β ligands; these changes are most pronounced in VSMC subsets enriched in the aortic root, while upregulation of putatively protective transcripts is more pronounced in VSMC subsets enriched in the ascending aorta, which is less prone to dilation in this model. These data suggest that loss of functional matrix and focal adhesions has secondary consequences specific to each VSMC subset, possibly contributing to the regional susceptibility to dilation in the aorta.
The fact that these four VSMC subsets exist in control animals suggests that distinct properties of the aortic root as determined by the proportion of specific VSMC subsets, specifically VSMC4, VSMC3 and VSMC2, distinguishes this segment prior to any disease-causing insult, and renders it intrinsically more vulnerable to the effect of Smad3 inactivation. We speculate that different VSMC subsets may confer specific susceptibility to genetic and/or environmental factors that preferentially affect a given aortic segment, and that a better understanding of the molecular determinants of these vulnerabilities may guide development of specific therapies that target maladaptive responses that contribute to disease progression.
The datasets presented in this study can be found in NCBI's Gene Expression Omnibus (GEO) database under the following accession numbers: GSE189025 and GSE189544.
The animal study was reviewed and approved by Animal Care and Use Committee at Johns Hopkins University School of Medicine.
EB and TC have contributed equally to this work and share first authorship. EM is responsible for the conception and design of this study, prepared slides, and acquired microscope images. TC initiated the experimental work, including animal breeding, performed echocardiography for most of the animals and produced the initial single cell RNA (scRNA) sequencing dataset and preliminary analysis. EB assisted with echocardiography and animal breeding and contributed significantly to the analysis of the scRNA sequencing data. WC contributed to the analysis of the scRNA sequencing data and performed the in situ validation. LG provided guidance in the processing and analysis of the scRNA sequencing data. MS assisted in all phases of this work including animal breeding and genotyping, performed the histological staining, and collected the blood pressure measurements. EB assisted EM in creating figures and wrote the majority of the manuscript. WC, RB, TC, and MS wrote portions of the methods. RB performed the bulk RNA sequencing which was analyzed by EM and TC. LR stained and imaged aortic sections for electron microscopy and quantified the number of connections in the resulting electron micrographs. All authors contributed to manuscript revision, read, and approved the submitted version.
Research reported in this publication was supported by the National Heart, Lung, and Blood Institute of the National Institutes of Health under Award Number R01HL147947 and by a generous gift from the Loeys-Dietz Foundation. EM was also supported by funding provided to Johns Hopkins by the Broccoli family.
The authors declare that the research was conducted in the absence of any commercial or financial relationships that could be construed as a potential conflict of interest.
All claims expressed in this article are solely those of the authors and do not necessarily represent those of their affiliated organizations, or those of the publisher, the editors and the reviewers. Any product that may be evaluated in this article, or claim that may be made by its manufacturer, is not guaranteed or endorsed by the publisher.
We greatly appreciate the Johns Hopkins School of Medicine Microscope Core Facility for training and assistance in image acquisition (support provided by the National Institutes of Health under Award Number S10OD023548). We would also like to thank Hao Zhang at the Johns Hopkins Bloomberg School of Public Health Cell Sorting Core Facility and Linda Orzolek at the Johns Hopkins Medical Institute Single Cell and Transcriptomics Core for their assistance. We acknowledge ThuyVy Duong for her help in optimizing SmartSeq2 protocols for sequencing bulk RNA from sorted aortic cells. Finally, we would like to show our appreciation for Dr. Dietz and his laboratory for helpful discussions and sharing of space and resources.
The Supplementary Material for this article can be found online at: https://www.frontiersin.org/articles/10.3389/fcvm.2022.826495/full#supplementary-material
1. Loeys BL, Schwarze U, Holm T, Callewaert BL, Thomas GH, Pannu H, et al. Aneurysm syndromes caused by mutations in the Tgf-Beta receptor. N Engl J Med. (2006) 355:788–98. doi: 10.1056/NEJMoa055695
2. Loeys BL, Chen J, Neptune ER, Judge DP, Podowski M, Holm T, et al. A Syndrome of altered cardiovascular, craniofacial, neurocognitive and skeletal development caused by mutations in Tgfbr1 or Tgfbr2. Nat Genet. (2005) 37:275–81. doi: 10.1038/ng1511
3. Lindsay ME, Schepers D, Bolar NA, Doyle JJ, Gallo E, Fert-Bober J, et al. Loss-of-function mutations in Tgfb2 cause a syndromic presentation of thoracic aortic aneurysm. Nat Genet. (2012) 44:922–7. doi: 10.1038/ng.2349
4. van de Laar IM, Oldenburg RA, Pals G, Roos-Hesselink JW, de Graaf BM, Verhagen JM, et al. Mutations in Smad3 cause a syndromic form of aortic aneurysms and dissections with early-onset osteoarthritis. Nat Genet. (2011) 43:121–6. doi: 10.1038/ng.744
5. Bertoli-Avella AM, Gillis E, Morisaki H, Verhagen JM, de Graaf BM, van de Beek G, et al. Mutations in a Tgf-Beta Ligand, Tgfb3, cause syndromic aortic aneurysms and dissections. J Am Coll Cardiol. (2015) 65:1324–36. doi: 10.1016/j.jacc.2015.01.040
6. Boileau C, Guo DC, Hanna N, Regalado ES, Detaint D, Gong L, et al. Tgfb2 mutations cause familial thoracic aortic aneurysms and dissections associated with mild systemic features of marfan syndrome. Nat Genet. (2012) 44:916–21. doi: 10.1038/ng.2348
7. Shi Y, Massague J. Mechanisms of Tgf-beta signaling from cell membrane to the nucleus. Cell. (2003) 113:685–700. doi: 10.1016/S0092-8674(03)00432-X
8. MacCarrick G, Black JH 3rd, Bowdin S, El-Hamamsy I, Frischmeyer-Guerrerio PA, Guerrerio AL, et al. Loeys-Dietz syndrome: a primer for diagnosis and management. Genet Med. (2014) 16:576–87. doi: 10.1038/gim.2014.11
9. Schepers D, Tortora G, Morisaki H, MacCarrick G, Lindsay M, Liang D, et al. A mutation update on the Lds-associated genes Tgfb2/3 and Smad2/3. Hum Mutat. (2018) 39:621–34. doi: 10.1002/humu.23407
10. Macias MJ, Martin-Malpartida P, Massague J. Structural determinants of smad function in Tgf-Beta signaling. Trends Biochem Sci. (2015) 40:296–308. doi: 10.1016/j.tibs.2015.03.012
11. Gong J, Zhou D, Jiang L, Qiu P, Milewicz DM, Chen YE, et al. In vitro lineage-specific differentiation of vascular smooth muscle cells in response to Smad3 deficiency: implications for Smad3-related thoracic aortic aneurysm. Arterioscler Thromb Vasc Biol. (2020) 40:1651–63. doi: 10.1161/ATVBAHA.120.313033
12. Li W, Li Q, Jiao Y, Qin L, Ali R, Zhou J, et al. Tgfbr2 disruption in postnatal smooth muscle impairs aortic wall homeostasis. J Clin Invest. (2014) 124:755–67. doi: 10.1172/JCI69942
13. Angelov SN, Hu JH, Wei H, Airhart N, Shi M, Dichek DA. Tgf-beta (transforming growth factor-beta) signaling protects the thoracic and abdominal aorta from angiotensin Ii-induced pathology by distinct mechanisms. Arterioscler Thromb Vasc Biol. (2017) 37:2102–13. doi: 10.1161/ATVBAHA.117.309401
14. Wei H, Hu JH, Angelov SN, Fox K, Yan J, Enstrom R, et al. Aortopathy in a mouse model of marfan syndrome is not mediated by altered transforming growth factor beta signaling. J Am Heart Assoc. (2017) 6:e004968. doi: 10.1161/JAHA.116.004968
15. Pinard A, Jones GT, Milewicz DM. Genetics of thoracic and abdominal aortic diseases. Circ Res. (2019) 124:588–606. doi: 10.1161/CIRCRESAHA.118.312436
16. Gallo EM, Loch DC, Habashi JP, Calderon JF, Chen Y, Bedja D, et al. Angiotensin Ii-dependent Tgf-beta signaling contributes to Loeys-Dietz syndrome vascular pathogenesis. J Clin Invest. (2014) 124:448–60. doi: 10.1172/JCI69666
17. van der Pluijm I, van Vliet N, von der Thusen JH, Robertus JL, Ridwan Y, van Heijningen PM, et al. Defective connective tissue remodeling in Smad3 mice leads to accelerated aneurysmal growth through disturbed downstream Tgf-Beta signaling. EBioMedicine. (2016) 12:280–94. doi: 10.1016/j.ebiom.2016.09.006
18. Creamer TJ, Bramel EE, MacFarlane EG. Insights on the pathogenesis of aneurysm through the study of hereditary aortopathies. Genes. (2021) 12:183. doi: 10.3390/genes12020183
19. Wagenseil JE, Mecham RP. Vascular extracellular matrix and arterial mechanics. Physiol Rev. (2009) 89:957–89. doi: 10.1152/physrev.00041.2008
20. Mo A, Mukamel EA, Davis FP, Luo C, Henry GL, Picard S, et al. Epigenomic signatures of neuronal diversity in the mammalian brain. Neuron. (2015) 86:1369–84. doi: 10.1016/j.neuron.2015.05.018
21. Verzi MP, McCulley DJ, De Val S, Dodou E, Black BL. The right ventricle, outflow tract, and ventricular septum comprise a restricted expression domain within the secondary/anterior heart field. Dev Biol. (2005) 287:134–45. doi: 10.1016/j.ydbio.2005.08.041
22. Lewis AE, Vasudevan HN, O'Neill AK, Soriano P, Bush JO. The widely used Wnt1-Cre transgene causes developmental phenotypes by ectopic activation of Wnt signaling. Dev Biol. (2013) 379:229–34. doi: 10.1016/j.ydbio.2013.04.026
23. Li Q, Pangas SA, Jorgez CJ, Graff JM, Weinstein M, Matzuk MM. Redundant roles of Smad2 and Smad3 in ovarian granulosa cells in vivo. Mol Cell Biol. (2008) 28:7001–11. doi: 10.1128/MCB.00732-08
24. Wirth A, Benyo Z, Lukasova M, Leutgeb B, Wettschureck N, Gorbey S, et al. G12-G13-Larg-mediated signaling in vascular smooth muscle is required for salt-induced hypertension. Nat Med. (2008) 14:64–8. doi: 10.1038/nm1666
25. Liu J, Krautzberger AM, Sui SH, Hofmann OM, Chen Y, Baetscher M, et al. Cell-specific translational profiling in acute kidney injury. J Clin Invest. (2014) 124:1242–54. doi: 10.1172/JCI76261
26. Schindelin J, Arganda-Carreras I, Frise E, Kaynig V, Longair M, Pietzsch T, et al. Fiji: an open-source platform for biological-image analysis. Nat Methods. (2012) 9:676–82. doi: 10.1038/nmeth.2019
27. Kalluri AS, Vellarikkal SK, Edelman ER, Nguyen L, Subramanian A, Ellinor PT, et al. Single-cell analysis of the normal mouse aorta reveals functionally distinct endothelial cell populations. Circulation. (2019) 140:147–63. doi: 10.1161/CIRCULATIONAHA.118.038362
28. Butcher MJ, Herre M, Ley K, Galkina E. Flow cytometry analysis of immune cells within murine aortas. J Vis Exp. (2011) 53:2848. doi: 10.3791/2848
29. Hao Y, Hao S, Andersen-Nissen E, Mauck WM 3rd, Zheng S, et al. Integrated analysis of multimodal single-cell data. Cell. (2021) 184:3573–87e29. doi: 10.1016/j.cell.2021.04.048
30. Chen EY, Tan CM, Kou Y, Duan Q, Wang Z, Meirelles GV, et al. Enrichr: interactive and collaborative Html5 gene list enrichment analysis tool. BMC Bioinform. (2013) 14:128. doi: 10.1186/1471-2105-14-128
31. Kuleshov MV, Jones MR, Rouillard AD, Fernandez NF, Duan Q, Wang Z, et al. Enrichr: a comprehensive gene set enrichment analysis web server 2016 update. Nucleic Acids Res. (2016) 44:W90–7. doi: 10.1093/nar/gkw377
32. Xie Z, Bailey A, Kuleshov MV, Clarke DJB, Evangelista JE, Jenkins SL, et al. Gene set knowledge discovery with enrichr. Curr Protoc. (2021) 1:e90. doi: 10.1002/cpz1.90
33. Haw R, Loney F, Ong E, He Y, Wu G. Perform pathway enrichment analysis using reactomefiviz. Methods Mol Biol. (2020) 2074:165–79. doi: 10.1007/978-1-4939-9873-9_13
34. Franz M, Lopes CT, Huck G, Dong Y, Sumer O, Bader GD. CytoscapeJs: a graph theory library for visualisation and analysis. Bioinformatics. (2016) 32:309–11. doi: 10.1093/bioinformatics/btv557
35. Kutmon M, van Iersel MP, Bohler A, Kelder T, Nunes N, Pico AR, et al. Pathvisio 3: an extendable pathway analysis toolbox. PLoS Comput Biol. (2015) 11:e1004085. doi: 10.1371/journal.pcbi.1004085
36. Martens M, Ammar A, Riutta A, Waagmeester A, Slenter DN, Hanspers K, et al. Wikipathways: connecting communities. Nucleic Acids Res. (2021) 49:D613–D21. doi: 10.1093/nar/gkaa1024
37. Szklarczyk D, Gable AL, Nastou KC, Lyon D, Kirsch R, Pyysalo S, et al. The string database in 2021: customizable protein-protein networks, and functional characterization of user-uploaded gene/measurement sets. Nucleic Acids Res. (2021) 49:D605–D12. doi: 10.1093/nar/gkab835
38. Bindea G, Mlecnik B, Hackl H, Charoentong P, Tosolini M, Kirilovsky A, et al. Cluego: a cytoscape plug-in to decipher functionally grouped gene ontology and pathway annotation networks. Bioinformatics. (2009) 25:1091–3. doi: 10.1093/bioinformatics/btp101
39. Bindea G, Galon J, Mlecnik B. Cluepedia cytoscape plugin: pathway insights using integrated experimental and in silico data. Bioinformatics. (2013) 29:661–3. doi: 10.1093/bioinformatics/btt019
40. Picelli S, Faridani OR, Bjorklund AK, Winberg G, Sagasser S, Sandberg R. Full-length Rna-Seq from single cells using smart-Seq2. Nat Protoc. (2014) 9:171–81. doi: 10.1038/nprot.2014.006
41. Li B, Dewey CN. Rsem: accurate transcript quantification from Rna-seq data with or without a reference genome. BMC Bioinformatics. (2011) 12:323. doi: 10.1186/1471-2105-12-323
42. Ge SX, Son EW, Yao R. Idep: An integrated web application for differential expression and pathway analysis of Rna-Seq data. BMC Bioinformatics. (2018) 19:534. doi: 10.1186/s12859-018-2486-6
43. Huang R, Grishagin I, Wang Y, Zhao T, Greene J, Obenauer JC, et al. The Ncats bioplanet - an integrated platform for exploring the universe of cellular signaling pathways for toxicology, systems biology, and chemical genomics. Front Pharmacol. (2019) 10:445. doi: 10.3389/fphar.2019.00445
44. Kanehisa M, Goto S. Kegg: Kyoto encyclopedia of genes and genomes. Nucleic Acids Res. (2000) 28:27–30. doi: 10.1093/nar/28.1.27
45. Han H, Cho JW, Lee S, Yun A, Kim H, Bae D, et al. Trrust V2: an expanded reference database of human and mouse transcriptional regulatory interactions. Nucleic Acids Res. (2018) 46:D380–D6. doi: 10.1093/nar/gkx1013
46. Yamashiro Y, Thang BQ, Shin SJ, Lino CA, Nakamura T, Kim J, et al. Role of thrombospondin-1 in mechanotransduction and development of thoracic aortic aneurysm in mouse and humans. Circ Res. (2018) 123:660–72. doi: 10.1161/CIRCRESAHA.118.313105
47. Martin B, Romero G, Salama G. Cardioprotective actions of relaxin. Mol Cell Endocrinol. (2019) 487:45–53. doi: 10.1016/j.mce.2018.12.016
48. Pasapera AM, Schneider IC, Rericha E, Schlaepfer DD, Waterman CM. Myosin II activity regulates vinculin recruitment to focal adhesions through Fak-mediated paxillin phosphorylation. J Cell Biol. (2010) 188:877–90. doi: 10.1083/jcb.200906012
49. Fournier PG, Juarez P, Jiang G, Clines GA, Niewolna M, Kim HS, et al. The Tgf-Beta signaling regulator Pmepa1 suppresses prostate cancer metastases to bone. Cancer Cell. (2015) 27:809–21. doi: 10.1016/j.ccell.2015.04.009
50. Deheuninck J, Luo K. Ski and Snon, potent negative regulators of Tgf-Beta signaling. Cell Res. (2009) 19:47–57. doi: 10.1038/cr.2008.324
51. Palumbo-Zerr K, Zerr P, Distler A, Fliehr J, Mancuso R, Huang J, et al. Orphan nuclear receptor Nr4a1 regulates transforming growth factor-beta signaling and fibrosis. Nat Med. (2015) 21:150–8. doi: 10.1038/nm.3777
52. Soond SM, Chantry A. Selective targeting of activating and inhibitory Smads by distinct Wwp2 ubiquitin ligase isoforms differentially modulates Tgfbeta signalling and Emt. Oncogene. (2011) 30:2451–62. doi: 10.1038/onc.2010.617
53. Chou EL, Lindsay ME. The genetics of aortopathies: hereditary thoracic aortic aneurysms and dissections. Am J Med Genet C Semin Med Genet. (2020) 184:136–48. doi: 10.1002/ajmg.c.31771
54. Sawada H, Rateri DL, Moorleghen JJ, Majesky MW, Daugherty A. Smooth muscle cells derived from second heart field and cardiac neural crest reside in spatially distinct domains in the media of the ascending aorta-brief report. Arterioscler Thromb Vasc Biol. (2017) 37:1722–6. doi: 10.1161/ATVBAHA.117.309599
55. Pannu H, Tran-Fadulu V, Papke CL, Scherer S, Liu Y, Presley C, et al. Myh11 mutations result in a distinct vascular pathology driven by insulin-like growth factor 1 and angiotensin Ii. Hum Mol Genet. (2007) 16:2453–62. doi: 10.1093/hmg/ddm201
56. Guo DC, Pannu H, Tran-Fadulu V, Papke CL Yu RK, Avidan N, et al. Mutations in smooth muscle alpha-actin (Acta2) lead to thoracic aortic aneurysms and dissections. Nat Genet. (2007) 39:1488–93. doi: 10.1038/ng.2007.6
57. Chen MH, Choudhury S, Hirata M, Khalsa S, Chang B, Walsh CA. Thoracic aortic aneurysm in patients with loss of function filamin a mutations: clinical characterization, genetics, and recommendations. Am J Med Genet A. (2018) 176:337–50. doi: 10.1002/ajmg.a.38580
58. Wang L, Zhang J, Fu W, Guo D, Jiang J, Wang Y. Association of smooth muscle cell phenotypes with extracellular matrix disorders in thoracic aortic dissection. J Vasc Surg. (2012) 56:1698-709. doi: 10.1016/j.jvs.2012.05.084
59. Lino Cardenas CL, Kessinger CW, MacDonald C, Jassar AS, Isselbacher EM, Jaffer FA, et al. Inhibition of the methyltranferase Ezh2 improves aortic performance in experimental thoracic aortic aneurysm. JCI Insight. (2018) 3:97493. doi: 10.1172/jci.insight.97493
60. Sasaki A, Masuda Y, Ohta Y, Ikeda K, Watanabe K. Filamin associates with smads and regulates transforming growth factor-beta signaling. J Biol Chem. (2001) 276:17871–7. doi: 10.1074/jbc.M008422200
61. Chen Y, Wei X, Zhang Z, He Y, Huo B, Guo X, et al. Downregulation of filamin a expression in the aorta is correlated with aortic dissection. Front Cardiovasc Med. (2021) 8:690846. doi: 10.3389/fcvm.2021.690846
62. Valino-Rivas L, Baeza-Bermejillo C, Gonzalez-Lafuente L, Sanz AB, Ortiz A, Sanchez-Nino MD. Cd74 in kidney disease. Front Immunol. (2015) 6:483. doi: 10.3389/fimmu.2015.00483
63. Fishel RS, Thourani V, Eisenberg SJ, Shai SY, Corson MA, Nabel EG, et al. Fibroblast growth factor stimulates angiotensin converting enzyme expression in vascular smooth muscle cells. Possible mediator of the response to vascular injury. J Clin Invest. (1995) 95:377–87. doi: 10.1172/JCI117666
64. Amighi J, Hoke M, Mlekusch W, Schlager O, Exner M, Haumer M, et al. Beta 2 microglobulin and the risk for cardiovascular events in patients with asymptomatic carotid atherosclerosis. Stroke. (2011) 42:1826–33. doi: 10.1161/STROKEAHA.110.600312
65. Doring Y, Pawig L, Weber C, Noels H. The Cxcl12/Cxcr4 chemokine ligand/receptor axis in cardiovascular disease. Front Physiol. (2014) 5:212. doi: 10.3389/fphys.2014.00212
66. de Haan JB, Bladier C, Griffiths P, Kelner M, O'Shea RD, Cheung NS, et al. Mice with a homozygous null mutation for the most abundant glutathione peroxidase, Gpx1, show increased susceptibility to the oxidative stress-inducing agents paraquat and hydrogen peroxide. J Biol Chem. (1998) 273:22528–36. doi: 10.1074/jbc.273.35.22528
67. Turner-Ivey B, Manevich Y, Schulte J, Kistner-Griffin E, Jezierska-Drutel A, Liu Y, et al. Role for Prdx1 as a specific sensor in redox-regulated senescence in breast cancer. Oncogene. (2013) 32:5302–14. doi: 10.1038/onc.2012.624
68. Onichtchouk D, Chen YG, Dosch R, Gawantka V, Delius H, Massague J, et al. Silencing of Tgf-beta signalling by the pseudoreceptor bambi. Nature. (1999) 401:480–5. doi: 10.1038/46794
69. Rahman MS, Akhtar N, Jamil HM, Banik RS, Asaduzzaman SM. Tgf-Beta/Bmp signaling and other molecular events: regulation of osteoblastogenesis and bone formation. Bone Res. (2015) 3:15005. doi: 10.1038/boneres.2015.5
70. Gogl G, Tugaeva KV, Eberling P, Kostmann C, Trave G, Sluchanko NN. Hierarchized phosphotarget binding by the seven human 14-3-3 isoforms. Nat Commun. (2021) 12:1677. doi: 10.1038/s41467-021-21908-8
71. Lo RS, Massague J. Ubiquitin-dependent degradation of Tgf-Beta-activated Smad2. Nat Cell Biol. (1999) 1:472–8. doi: 10.1038/70258
72. Vacante F, Rodor J, Lalwani MK, Mahmoud AD, Bennett M, De Pace AL, et al. Carmn loss regulates smooth muscle cells and accelerates atherosclerosis in mice. Circ Res. (2021) 128:1258–75. doi: 10.1161/CIRCRESAHA.120.318688
73. Mondal T, Subhash S, Vaid R, Enroth S, Uday S, Reinius B, et al. Meg3 long noncoding Rna regulates the Tgf-Beta pathway genes through formation of Rna-DNA triplex structures. Nat Commun. (2015) 6:7743. doi: 10.1038/ncomms8743
74. Sun Z, Nie X, Sun S, Dong S, Yuan C, Li Y, et al. Long non-coding Rna Meg3 downregulation triggers human pulmonary artery smooth muscle cell proliferation and migration via the P53 signaling pathway. Cell Physiol Biochem. (2017) 42:2569–81. doi: 10.1159/000480218
75. Huang J, Yamashiro Y, Papke CL, Ikeda Y, Lin Y, Patel M, et al. Angiotensin-Converting Enzyme-Induced Activation of Local Angiotensin Signaling Is Required for Ascending Aortic Aneurysms in Fibulin-4-Deficient Mice. Sci Transl Med. (2013) 5(183):183ra58, 1-11. doi: 10.1126/scitranslmed.3005025
76. Inamoto S, Kwartler CS, Lafont AL, Liang YY, Fadulu VT, Duraisamy S, et al. Tgfbr2 mutations alter smooth muscle cell phenotype and predispose to thoracic aortic aneurysms and dissections. Cardiovasc Res. (2010) 88:520–9. doi: 10.1093/cvr/cvq230
77. van der Linde D, van de Laar IM, Bertoli-Avella AM, Oldenburg RA, Bekkers JA, Mattace-Raso FU, et al. Aggressive cardiovascular phenotype of aneurysms-osteoarthritis syndrome caused by pathogenic Smad3 variants. J Am Coll Cardiol. (2012) 60:397–403. doi: 10.1016/j.jacc.2011.12.052
78. van de Laar IM, van der Linde D, Oei EH, Bos PK, Bessems JH, Bierma-Zeinstra SM, et al. Phenotypic spectrum of the Smad3-related aneurysms-osteoarthritis syndrome. J Med Genet. (2012) 49:47–57. doi: 10.1136/jmedgenet-2011-100382
79. Wischmeijer A, Van Laer L, Tortora G, Bolar NA, Van Camp G, Fransen E, et al. Thoracic aortic aneurysm in infancy in aneurysms-osteoarthritis syndrome due to a novel Smad3 mutation: further delineation of the phenotype. Am J Med Genet A. (2013) 161A:1028–35. doi: 10.1002/ajmg.a.35852
80. Micha D, Guo DC, Hilhorst-Hofstee Y, van Kooten F, Atmaja D, Overwater E, et al. Smad2 mutations are associated with arterial aneurysms and dissections. Hum Mutat. (2015) 36:1145–9. doi: 10.1002/humu.22854
81. Guo DC, Regalado ES, Pinard A, Chen J, Lee K, Rigelsky C, et al. Ltbp3 pathogenic variants predispose individuals to thoracic aortic aneurysms and dissections. Am J Hum Genet. (2018) 102:706–12. doi: 10.1016/j.ajhg.2018.03.002
82. Zilberberg L, Phoon CK, Robertson I, Dabovic B, Ramirez F, Rifkin DB. Genetic analysis of the contribution of Ltbp-3 to thoracic aneurysm in marfan syndrome. Proc Natl Acad Sci USA. (2015) 112:14012–7. doi: 10.1073/pnas.1507652112
83. Schoenhoff FS. Increased Tgf-Beta signaling precedes aneurysm formation in Smad3 deficient mice. EBioMedicine. (2016) 12:26–7. doi: 10.1016/j.ebiom.2016.09.033
84. MacFarlane EG, Parker SJ, Shin JY, Ziegler SG, Creamer TJ, Bagirzadeh R, et al. Lineage-specific events underlie aortic root aneurysm pathogenesis in Loeys-Dietz syndrome. J Clin Invest. (2019). doi: 10.1172/JCI123547
85. Daugherty A, Chen Z, Sawada H, Rateri DL, Sheppard MB. Transforming growth factor-beta in thoracic aortic aneurysms: good, bad, or irrelevant? J Am Heart Assoc. (2017) 6:e005221. doi: 10.1161/JAHA.116.005221
86. van Dorst DCH, de Wagenaar NP, van der Pluijm I, Roos-Hesselink JW, Essers J, Danser AHJ. Transforming growth factor-beta and the renin-angiotensin system in syndromic thoracic aortic aneurysms: implications for treatment. Cardiovasc Drugs Ther. (2020). doi: 10.1007/s10557-020-07116-4
87. Azhar M, Schultz Jel J, Grupp I, Dorn GW 2nd, Meneton P, Molin DG, et al. Transforming growth factor beta in cardiovascular development and function. Cytokine Growth Factor Rev. (2003) 14:391–407. doi: 10.1016/S1359-6101(03)00044-3
88. Azhar M, Ware SM. Genetic and developmental basis of cardiovascular malformations. Clin Perinatol. (2016) 43:39–53. doi: 10.1016/j.clp.2015.11.002
89. Chakrabarti M, Al-Sammarraie N, Gebere MG, Bhattacharya A, Chopra S, Johnson J, et al. Transforming growth factor Beta3 is required for cardiovascular development. J Cardiovasc Dev Dis. (2020) 7:19. doi: 10.3390/jcdd7020019
90. Hu JH, Wei H, Jaffe M, Airhart N, Du L, Angelov SN, et al. Postnatal deletion of the type Ii transforming growth factor-beta receptor in smooth muscle cells causes severe aortopathy in mice. Arterioscler Thromb Vasc Biol. (2015) 35:2647–56. doi: 10.1161/ATVBAHA.115.306573
91. Cook JR, Clayton NP, Carta L, Galatioto J, Chiu E, Smaldone S, et al. Dimorphic effects of transforming growth factor-beta signaling during aortic aneurysm progression in mice suggest a combinatorial therapy for marfan syndrome. Arterioscler Thromb Vasc Biol. (2015) 35:911–7. doi: 10.1161/ATVBAHA.114.305150
92. Karimi A, Milewicz DM. Structure of the elastin-contractile units in the thoracic aorta and how genes that cause thoracic aortic aneurysms and dissections disrupt this structure. Can J Cardiol. (2016) 32:26–34. doi: 10.1016/j.cjca.2015.11.004
93. Michel JB, Jondeau G, Milewicz DM. From genetics to response to injury: vascular smooth muscle cells in aneurysms and dissections of the ascending aorta. Cardiovasc Res. (2018) 114:578–89. doi: 10.1093/cvr/cvy006
94. Lino Cardenas CL, Kessinger CW, Cheng Y, MacDonald C, MacGillivray T, Ghoshhajra B, et al. An Hdac9-Malat1-Brg1 complex mediates smooth muscle dysfunction in thoracic aortic aneurysm. Nat Commun. (2018) 9:1009. doi: 10.1038/s41467-018-03394-7
95. Fultz ME Li C, Geng W, Wright GL. Remodeling of the actin cytoskeleton in the contracting A7r5 smooth muscle cell. J Muscle Res Cell Motil. (2000) 21:775–87. doi: 10.1023/A:1010396429297
96. Cohen DM, Kutscher B, Chen H, Murphy DB, Craig SW. A Conformational switch in vinculin drives formation and dynamics of a talin-vinculin complex at focal adhesions. J Biol Chem. (2006) 281:16006–15. doi: 10.1074/jbc.M600738200
97. del Rio A, Perez-Jimenez R, Liu R, Roca-Cusachs P, Fernandez JM, Sheetz MP. Stretching single talin rod molecules activates vinculin binding. Science. (2009) 323:638–41. doi: 10.1126/science.1162912
98. Friedland JC, Lee MH, Boettiger D. Mechanically activated integrin switch controls alpha5beta1 function. Science. (2009) 323:642–4. doi: 10.1126/science.1168441
99. Lin CJ, Staiculescu MC, Hawes JZ, Cocciolone AJ, Hunkins BM, Roth RA, et al. Heterogeneous cellular contributions to elastic laminae formation in arterial wall development. Circ Res. (2019) 125:1006–18. doi: 10.1161/CIRCRESAHA.119.315348
100. Curran ME, Atkinson DL, Ewart AK, Morris CA, Leppert MF, Keating MT. The elastin gene is disrupted by a translocation associated with supravalvular aortic stenosis. Cell. (1993) 73:159–68. doi: 10.1016/0092-8674(93)90168-P
101. Ewart AK, Morris CA, Atkinson D, Jin W, Sternes K, Spallone P, et al. Hemizygosity at the elastin locus in a developmental disorder, Williams syndrome. Nat Genet. (1993) 5:11–6. doi: 10.1038/ng0993-11
102. Ewart AK, Jin W, Atkinson D, Morris CA, Keating MT. Supravalvular aortic stenosis associated with a deletion disrupting the elastin gene. J Clin Invest. (1994) 93:1071–7. doi: 10.1172/JCI117057
103. Tassabehji M, Metcalfe K, Donnai D, Hurst J, Reardon W, Burch M, et al. Elastin: genomic structure and point mutations in patients with supravalvular aortic stenosis. Hum Mol Genet. (1997) 6:1029–36. doi: 10.1093/hmg/6.7.1029
104. Li DY, Brooke B, Davis EC, Mecham RP, Sorensen LK, Boak BB, et al. Elastin is an essential determinant of arterial morphogenesis. Nature. (1998) 393:276–80. doi: 10.1038/30522
105. Koch A, Buheitel G, Hofbeck M, Rauch A, Kraus C, Tassabehji M, et al. Spectrum of arterial obstructions caused by one elastin gene point mutation. Eur J Pediatr. (2003) 162:53–4. doi: 10.1007/s00431-002-1111-9
106. Micale L, Turturo MG, Fusco C, Augello B, Jurado LA, Izzi C, et al. Identification and characterization of seven novel mutations of elastin gene in a cohort of patients affected by supravalvular aortic stenosis. Eur J Hum Genet. (2010) 18:317–23. doi: 10.1038/ejhg.2009.181
107. Duque Lasio ML, Kozel BA. Elastin-driven genetic diseases. Matrix Biol. (2018) 71–72:144–60. doi: 10.1016/j.matbio.2018.02.021
108. Bunton TE, Biery NJ, Myers L, Gayraud B, Ramirez F, Dietz HC. Phenotypic alteration of vascular smooth muscle cells precedes elastolysis in a mouse model of Marfan syndrome. Circ Res. (2001) 88:37–43. doi: 10.1161/01.RES.88.1.37
109. Chung AW, Au Yeung K, Sandor GG, Judge DP, Dietz HC, van Breemen C. Loss of elastic fiber integrity and reduction of vascular smooth muscle contraction resulting from the upregulated activities of matrix metalloproteinase-2 and−9 in the thoracic aortic aneurysm in marfan syndrome. Circ Res. (2007) 101:512–22. doi: 10.1161/CIRCRESAHA.107.157776
110. Pedroza AJ, Tashima Y, Shad R, Cheng P, Wirka R, Churovich S, et al. Single-cell transcriptomic profiling of vascular smooth muscle cell phenotype modulation in Marfan syndrome aortic aneurysm. Arterioscler Thromb Vasc Biol. (2020):ATVBAHA120314670. doi: 10.1161/ATVBAHA.120.314670
111. Jiang X, Rowitch DH, Soriano P, McMahon AP, Sucov HM. Fate of the mammalian cardiac neural crest. Development. (2000) 127:1607–16. doi: 10.1242/dev.127.8.1607
112. Buckingham M, Meilhac S, Zaffran S. Building the mammalian heart from two sources of myocardial cells. Nat Rev Genet. (2005) 6:826–35. doi: 10.1038/nrg1710
113. Granata A, Serrano F, Bernard WG, McNamara M, Low L, Sastry P, et al. An Ipsc-derived vascular model of marfan syndrome identifies key mediators of smooth muscle cell death. Nat Genet. (2017) 49:97–109. doi: 10.1038/ng.3723
114. Iosef C, Pedroza AJ, Cui JZ, Dalal AR, Arakawa M, Tashima Y, et al. Quantitative proteomics reveal lineage-specific protein profiles in Ipsc-derived Marfan syndrome smooth muscle cells. Sci Rep. (2020) 10:20392. doi: 10.1038/s41598-020-77274-w
115. Zhou D, Feng H, Yang Y, Huang T, Qiu P, Zhang C, et al. Hipsc modeling of lineage-specific smooth muscle cell defects caused by Tgfbr1(A230t) variant, and its therapeutic implications for Loeys-Dietz syndrome. Circulation. (2021) 144:1145–59. doi: 10.1161/CIRCULATIONAHA.121.054744
116. Humphrey JD, Tellides G. Central artery stiffness and thoracic aortopathy. Am J Physiol Heart Circ Physiol. (2019) 316:H169–H82. doi: 10.1152/ajpheart.00205.2018
Keywords: TGF-β, Smad3, scRNA seq, Loeys-Dietz syndrome, aortic aneurysm, smooth muscle
Citation: Bramel EE, Creamer TJ, Saqib M, Camejo Nunez WA, Bagirzadeh R, Roker LA, Goff LA and MacFarlane EG (2022) Postnatal Smad3 Inactivation in Murine Smooth Muscle Cells Elicits a Temporally and Regionally Distinct Transcriptional Response. Front. Cardiovasc. Med. 9:826495. doi: 10.3389/fcvm.2022.826495
Received: 30 November 2021; Accepted: 07 March 2022;
Published: 08 April 2022.
Edited by:
Julie A. Phillippi, University of Pittsburgh, United StatesReviewed by:
Yoshito Yamashiro, University of Tsukuba, JapanCopyright © 2022 Bramel, Creamer, Saqib, Camejo Nunez, Bagirzadeh, Roker, Goff and MacFarlane. This is an open-access article distributed under the terms of the Creative Commons Attribution License (CC BY). The use, distribution or reproduction in other forums is permitted, provided the original author(s) and the copyright owner(s) are credited and that the original publication in this journal is cited, in accordance with accepted academic practice. No use, distribution or reproduction is permitted which does not comply with these terms.
*Correspondence: Elena Gallo MacFarlane, ZWdhbGxvMUBqaG1pLmVkdQ==
†These authors have contributed equally to this work and share first authorship
Disclaimer: All claims expressed in this article are solely those of the authors and do not necessarily represent those of their affiliated organizations, or those of the publisher, the editors and the reviewers. Any product that may be evaluated in this article or claim that may be made by its manufacturer is not guaranteed or endorsed by the publisher.
Research integrity at Frontiers
Learn more about the work of our research integrity team to safeguard the quality of each article we publish.