- 1Division of Cardiology, Department of Internal Medicine, Tongji Hospital, Tongji Medical College, Huazhong University of Science and Technology, Wuhan, China
- 2Hubei Key Laboratory of Genetics and Molecular Mechanisms of Cardiological Disorders, Huazhong University of Science and Technology, Wuhan, China
- 3Department of Gastroenterology, Hubei No. 3 People’s Hospital of Jianghan University, Wuhan, China
Hawthorn leaves, which is a traditional Chinese medicine (TCM), has been used for treating coronary heart disease (CHD) for a long time in China. But the limited understanding of the main active components and molecular mechanisms of this traditional medicine has restricted its application and further research. The active compounds of hawthorn leaves were obtained from TCMSP database and SymMap database. The targets of it were predicted based on TCMSP, PubChem, Swiss Target Prediction, and SymMap database. The putative targets of CHD were gathered from multi-sources databases including the Online Mendelian Inheritance in Man (OMIM) database, the DrugBank database, the GeneCards database and the DisGeNet database. Network topology analysis, GO and KEGG pathway enrichment analyses were performed to select the key targets and pathways. Molecular docking was performed to demonstrate the binding capacity of the key compounds to the predicted targets. Furthermore, RAW264.7 cells stimulated by lipopolysaccharides (LPS) were treated with three effective compounds of hawthorn leaves to assess reliability of prediction. Quercetin, isorhamnetin and kaempferol were main active compounds in hawthorn leaves. Forty four candidate therapeutic targets were identified to be involved in protection of hawthorn leaves against CHD. Additionally, the effective compounds of it had good binding affinities to PTGS2, EGFR, and MMP2. Enrichment analyses suggested that immune inflammation related biological processes and pathways were possibly the potential mechanism. Besides, we found that three predicted effective compounds of hawthorn leaves decreased protein expression of PTGS2, MMP2, MMP9, IL6, IL1B, TNFα and inhibited activation of macrophage. In summary, the present study demonstrates that quercetin, kaempferol and isorhamnetin are proved to be the main effective compounds of hawthorn leaves in treatment of CHD, possibly by suppressing expression of PTGS2, MMP2, MMP9, inflammatory cytokines and macrophages viability. This study provides a new understanding of the active components and mechanisms of hawthorn leaves treating CHD from the perspective of network pharmacology.
Introduction
Coronary heart disease (CHD), one of the leading causes of human death in the world, is characterized by atherosclerosis of the coronary arteries, narrowing or occluding the vascular lumen, leading to myocardial ischemia, hypoxia or necrosis (1). Coronary artery stenosis or plaque rupture often causes myocardial ischemia or infarction, which can lead to heart failure even death (2). Drug therapy, one of the most important methods to treat CHD, includes nitrates drugs, β-receptor blockers, antithrombotic drugs, calcium channel blockers, renin-angiotensin system inhibitors and lipid-lowering drugs, which are usually aimed to act on individual targets (3). However, the effects of these drugs can’t eliminate vascular stenosis, especially, long-term use of some drugs may lead to side effects or drug resistance (4, 5). So, drugs with multiple active ingredients and multiple targets are urgently needed.
Traditional Chinese Medicine (TCM) has been used for ages to treat a variety of diseases including CHD and its related diseases. The diversity of components and mechanisms of action is an important feature of TCM (6). Hawthorn (Also known as Crataegus pinnatifida Bge) belongs to the genus crataegus of the rosaceae family. It is used as a source of food and medicine, and widely distributed in the northeast part of China (7). Currently, many researchers have put their focus on hawthorn not only for its fruit but also for its leaves. Hawthorn leaves, as raw materials of herbal medicines, have been proven to have a variety of pharmacological properties, including preventing or treating cardiovascular diseases, improving circulation of coronary, lowering blood lipids and preventing hypertension (8). Besides, adjusting digestive function disorder is also one of its important effects (9). A number of domestic and foreign studies have shown that hawthorn leaves have therapeutic effects on CHD (7, 10). Compared with single-target drugs, although hawthorn leaves have a richer array of active components and potential mechanisms, the details about bioactive compounds and mechanisms of action are rarely reported.
Network pharmacology is an important tool to explore the active components and action mechanism of TCM. It analyzes the mechanisms of action of drugs from a systematic and comprehensive perspective (11). So far, many studies exploring the active components and mechanisms of TCM in the prevention or treatment of CHD through network pharmacology have obtained satisfactory results (12–14). Approaches based on network pharmacology are promising research tools that can help us understand the relationship between drugs and disease in a more comprehensive and detailed way (15, 16).
In this study, approaches based on network pharmacology were used to investigate underlying mechanism of action of hawthorn leaves in treatment of CHD. The purpose of this study was to explore main active components and possible mechanisms of hawthorn leaves in the treatment of CHD from the perspective of network biology.
Materials and Methods
Identification of Active Ingredients
The Traditional Chinese Medicine Systems Pharmacology Database (TCMSP)1 (17) and SymMap Databases2 were used to identify bioactive ingredients of hawthorn leaves. Pharmacokinetic parameters of each compound [absorption, distribution, metabolism, and excretion (ADME)] in hawthorn leaves were determined. All compounds with oral bioavailability (OB) ≥ 30 % and drug-likeness (DL) ≥ 0.18 were retrieved for subsequent research. OB is a pharmacokinetic parameter, representing the relative amount of a drug absorbed into the systemic circulation after oral administration. DL is a qualitative characteristic used to evaluate the possibility of converting a compound into a drug.
Prediction of Putative Targets of Hawthorn Leaves
The supposed targets of effective compounds were picked up from the TCMSP database (17; see text footnote 1), the PubChem database (18),3 the Swiss Target Prediction database (19)4 and the SymMap Databases (see text footnote 2). Then, uniprot sites5 were used to process all the names of targets and normalize the gene information following the standards of human origin. Detailed information of supposed targets is showed in Supplementary Table 1.
Identification of Coronary Heart Disease Related Targets
Related CHD targets were collected from multiple databases including the Online Mendelian Inheritance in Man database (20) (OMIM),6 the DrugBank database (21),7 the GeneCards database (22)8 and the DisGeNet database (23).9 Detailed information is showed in Supplementary Table 4.
Construction of Protein-Protein Interaction Network
PPI is short for the interaction between proteins, indicating that multiple proteins form an interaction network with non-covalent bonds. STRING 11.0 database10 were used to construct the PPI network of compound-CHD targets. The protein interactions were recognized as owning high confidence when the scoring value > 0.4 (24). Then we used Cytoscape 3.9.0 to merge compound-CHD target PPI network file and get the intersection of PPI network and core genes in compound-target PPI network.
Gene Ontology and Kyoto Encyclopedia of Genes and Genomes Pathway Enrichment Analyses
The gene ontology (GO) and Kyoto Encyclopedia of Genes and Genomes (KEGG) pathway enrichment analyses were conducted using the functional annotation tool of DAVID Bioinformatics Resources.11 Official gene symbols were uploaded and the background was set to be homo sapiens due to the limitation of annotations. GO terms, which consists of molecular function (MF), biological process (BP) and cellular components (CC), were identified. The results of MF, BP, CC and KEGG were input into Hiplot database,12 which is a free and comprehensive scientific data analysis and visualization tool based on web technology and is supported by open source communities.
Network Construction
We constructed networks using Cytoscape as follows: Firstly, the “hawthorn leaves bioactive compound target network” was established by connecting the compounds and targets of hawthorn leaves. Secondly, the “compound target signal pathway network” was constructed by connecting the targets of the ingredients and related signal pathways. Thirdly, the “PPI network” was built by connecting targets with other interactive human proteins.
Identification of Differentially Expressed Genes Based on GSE12288
The expression profiles of GSE12288 was downloaded from the Gene Expression Omnibus (GEO) database in the NCBI portal13 and included for analysis. Limma package was performed to identify differentially expressed genes (DEGs) between healthy controls and coronary artery disease (CAD) samples. P < 0.05 were selected as thresholds to indicate a statistically significant difference. Logistic regression was performed to identify association between target genes and CAD and the model’s accuracy was evaluated using the receiver operating characteristics (ROC) curve. Statistical analyses were performed with the use of R software, version 4.0.5 (R Foundation for Statistical Computing).
Molecular Docking Simulation
Molecular docking was performed to further demonstrate the relationship between the key compounds and major target genes. The key compounds of hawthorn leaves were downloaded from the Pubchem database (see text footnote 4) and were changed to PDB format by a software called PyMol (the PyMol Molecular Graphics System). PyMol, which is an open-source molecular visualization system, has the ability to render high-quality 3D images of small molecules and biological macromolecules, and to remove original ligand, water molecules and phosphates (25). High-quality 2D images of small molecules and biological macromolecules was used Discovery Studio (4.5 Visualizer). Next, AutoDockTools (version 1.5.6) (26) was used for preparing receptors, including adding hydrogen and setting docking parameters. The blind docking was performed with the “Grid box” set to maximum. Subsequently, we set the key compounds as ligand and used Auto-DockTools to detect their structure torsions and roots. Then we evaluated and verified the binding affinity between compounds and targets, and the reliability of predicted results from network pharmacology by Autodock Vina (27), a freely available open-source packages. The docking affinity score below –5.0 kcal/mol is recognized as the criterion which suggests there is a strong binding interaction between the compounds and their target genes (28).
Cell Culture and Treatment
RAW264.7 cells were cultured with Dulbecco’s modified Eagle medium (DMEM, Gibco) containing 10% fetal bovine serum (Gibco) and 1% penicillin/streptomycin (Gibco) in a CO2 incubator (5% CO2 at 37°C). RAW264.7 cells were stimulated with LPS (1 μg/ml) (Sigma-Aldrich) in the presence or absence of quercetin (25, 50, 100 μM) (MCE, HY-18085), kaempferol (50, 100, 200 μM) (MCE, HY-14590), and isohamnetin (5, 10, 20 μM) (MCE, HY-N0776) for 24 h.
Cell Viability Assay
RAW264.7 cells were inoculated in 96-well plates at 2 × 103 cells/well. Following the treatments mentioned above, the Cell Counting Kit-8 solution (Promoter Biological, Wuhan, China) was added to the medium according to the operation manual, the plates were incubated for 2 h in the dark at 5% CO2, 37°C. The optical density (OD) was quantitated by microplate reader (Bio-Tek, Vermont, United States) at 450 nm wavelength.
Western Blotting
IP lysate including 1:100 phenylmethanesulfonyl was used to extract RAW264.7 cells. Protein concentration was measured with a BCA kit (Boster, Wuhan, China). 10–12% gradient gels and polyvinylidene fluoride membranes were used to separate and transfer the protein samples (20–40 μg) sequentially. After blocked with 5% bovine serum albumin for 1 h, the membranes were incubated with primary antibodies against PTGS2 (1:1,000, ABClonal, Wuhan, China), EGFR (1:1,000, ABClonal, Wuhan, China), MMP2 (1:1,000, ABClonal, Wuhan, China), MMP9 (1:1,000, ABClonal, Wuhan, China), ESR1 (1:1,000, ABClonal, Wuhan, China), IL6 (1:1,000, Affinity, Jiangsu, China), IL1B (1:1,000, Proteintech, Rocky Hill, NJ, United States), TNFα (1:1,000, ABClonal, Wuhan, China) and GAPDH (1:1,000, Proteintech, Rocky Hill, NJ, United States) at 4 °C overnight. After washed with TBST, the membranes were incubated with the corresponding secondary antibodies for 1 h at room temperature. Bands were detected by Electrochemiluminescence and protein levels were analyzed with Gel Pro analysis software.
Statistical Analysis
All statistical analyses were performed on GraphPad Prism 8.0 (GraphPad Software, United States). Significant differences were analyzed by one-way analysis of variance (ANOVA) followed by Tukey’s post-test among groups of three or more. P values < 0.05 were considered statistically significant.
Results
Identification of the Active Compounds and Target Genes in Hawthorn Leaves
The flow diagram of this study is summarized in Figure 1. With the criteria of OB ≥ 30 % and DL ≥ 0.18, 9 compounds of the hawthorn leaves were selected from TCMSP and SymMap Databases, including ent-epicatechin, quercetin, isorhamnetin, sitosterol, kaempferol, stigmasterol, leucodelphinidin, leucopelargonidin, and icariin (Table 1). Then, we identified 988 putative targets from TCMSP, Pubchem, Swiss Target Prediction and SymMap Databases. After combing all the target genes and removing the duplicated genes, we identified 835 unique target genes, the detailed information is showed in Supplementary Table 1. Subsequently, we constructed the compound-target network in order to obtain more information about the 9 compounds and their corresponding targets at the overall level (Figure 2). Adopting the average degree (93) as the threshold value, we identified top three compounds through a network topology analysis: Quercetin (degree = 310), Kaempferol (degree = 192) and Isorhamnetin (degree = 127). Moreover, 328 target genes attached to these three compounds were selected (Supplementary Table 2).
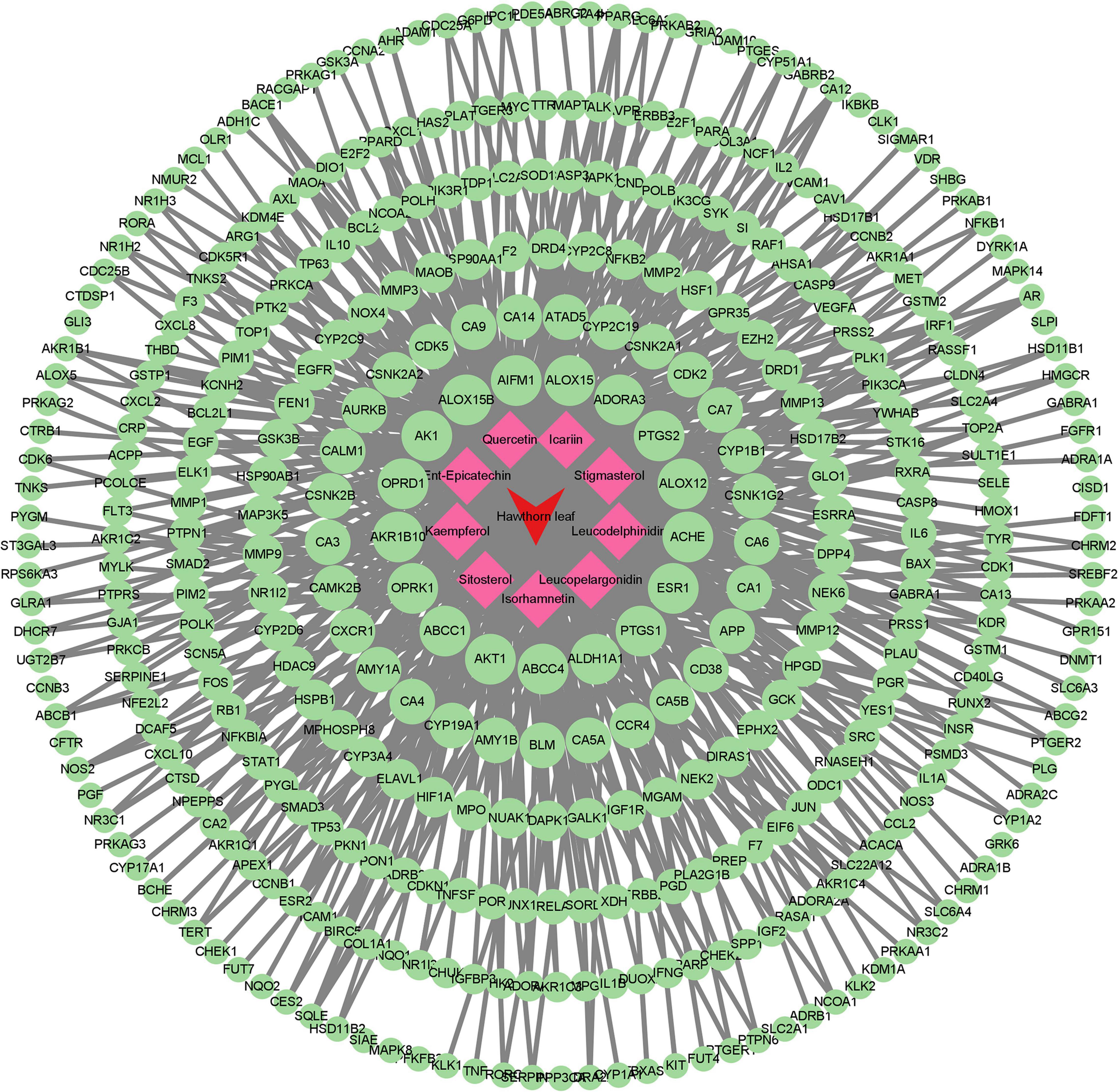
Figure 2. Compound–target network. Red and green circular nodes represent active compounds and corresponding targets, respectively.
Coronary Heart Disease Target Network
This study got 1892, 294, 35, and 546 targets involved in CHD from Genecard, Disgenet, Drugbank, and OMIM gene database, respectively. To improve the accuracy of the selected disease targets, we selected targets that appeared simultaneously in two or more gene databases. 194 disease targets were selected in this study (Figure 3A). Moreover, the target genes of CHD were validated by using dataset of 110 patients with CAD (CADi > 23) and 112 partially matched controls without CAD (CADi = 0) that obtained in GSE12288. Finally, 177 target genes for CHD were screened out (Figure 3B). The detailed information is described in Supplementary Table 3. Moreover, the core targets were confirmed by PPI network analysis for further analysis, and the nodes with high degree values were recognized as the hub targets related to CHD. 22 targets with the highest degree values (degree ≥ two fold of the average) were IL6, TNF, APOE, IL1B, VEGFA, CCL2, MMP9, CXCL8, IL10, TLR4, CRP, PPARG, EGFR, ICAM1, SERPINE1, NOS3, LEP, ADIPOQ, PTGS2, APOB, ACE, MMP2 (Figure 3C).
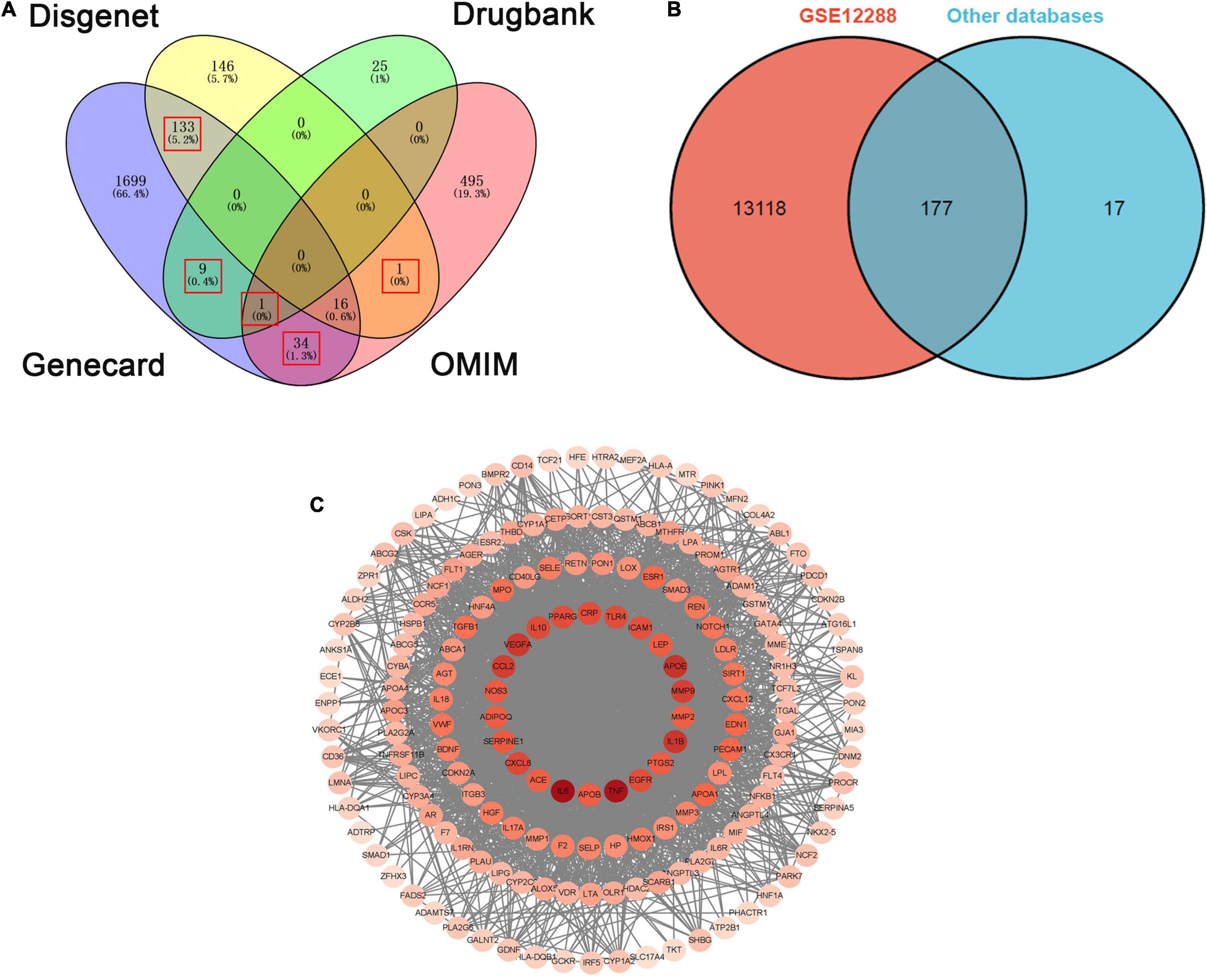
Figure 3. The overlapped targets of CHD from four databases and PPI network of CHD targets. (A) The overlapped targets of CHD from four databases (Disgenet, Dregbank, Genecard, OMIM). (B) The overlapped targets of CHD from GSE12288 and the other four databases. (C) PPI network of CHD targets.
Protein-Protein Interaction Network of Compound-Coronary Heart Disease Targets
Based on the results mentioned above, the 328 putative targets of hawthorn leaves mapped to the 177 CHD related targets to get the overlapped targets. The detailed information of CHD related targets is showed in Supplementary Table 4. Consequently, 44 targets were selected as the candidate targets responsible for CHD therapy (Figure 4A). PPI network of 44 common targets genes was constructed through STRING software (Figure 4B). 44 genes were ranked in descending order by degree (average degree = 20.32) after analyzing the topological feature of the PPI network, and the detailed information is showed in Supplementary Table 5. There were 44 nodes and 200 edges in this network. Finally, top fourteen target genes were selected out according to the topological properties of the network nodes (degree > 26). The target genes included IL6, VEGFA, IL1B, MMP9, CXCL8, CCL2, PTGS2, IL10, ESR1, EGFR, MMP2, CRP, SERPINE1 and ICAM1. These hub genes will be further used for the molecular docking study.
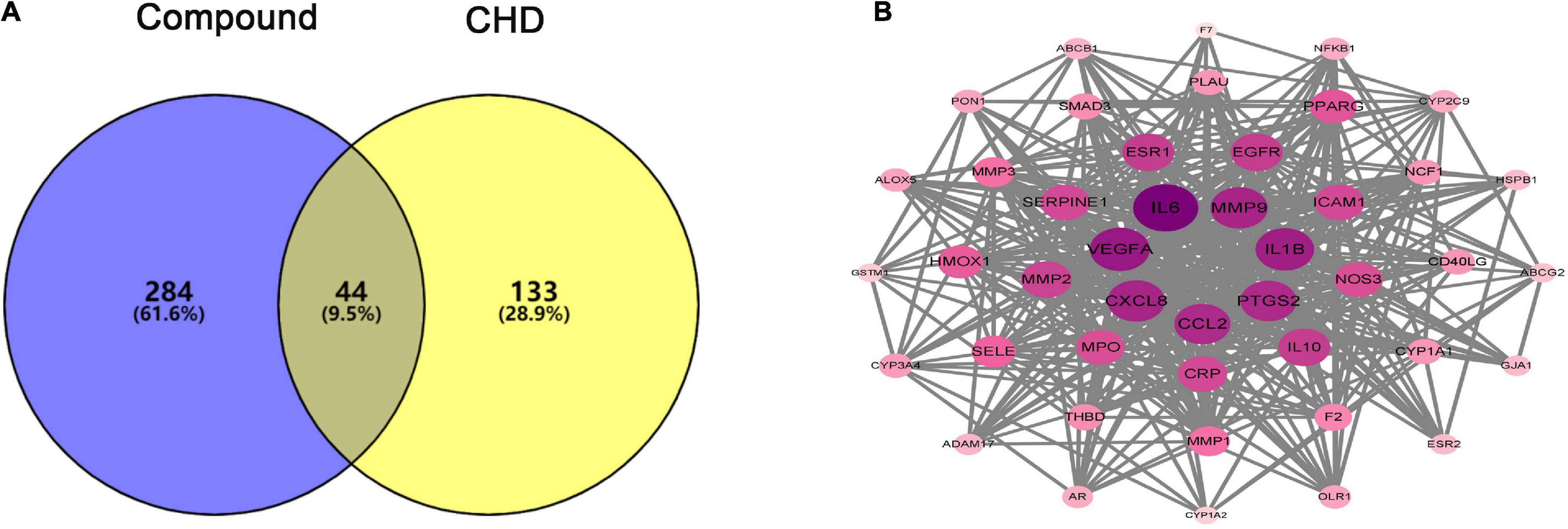
Figure 4. Venn diagram and PPI network of compound-CHD targets. (A) Venn diagram of intersecting targets of hawthorn leaves and CHD. (B) PPI network of compound-CHD targets.
Gene Ontology Analysis and Kyoto Encyclopedia of Genes and Genomes Pathway Enrichment Analysis
To clarify the mechanism of drug treatment ulteriorly, the enrichment analyses were performed. GO annotations including biological process (BP), cell composition (CC), and molecular function (MF) were analyzed. The results of enrichment included 115 BP terms, 12 CC terms and 39 MF terms. The top ten terms with a significant adjusted P-value were shown in Figures 5A–C. Main BP included the response to inflammatory response, negative regulation of apoptotic process and response to hypoxia; main CC involved extracellular space; main MF covered protein binding, enzyme binding, heme binding. 31 relevant pathways of the candidate targets were acquired through KEGG pathway enrichment (Figure 5D). The main KEGG pathways included TNF, NF-kappa B and HIF-1 signaling pathway. The detailed information is showed in Supplementary Table 6. These data suggested that the potential mechanism of action underlying CHD treatment might be the anti-inflammatory effects of hawthorn leaves.
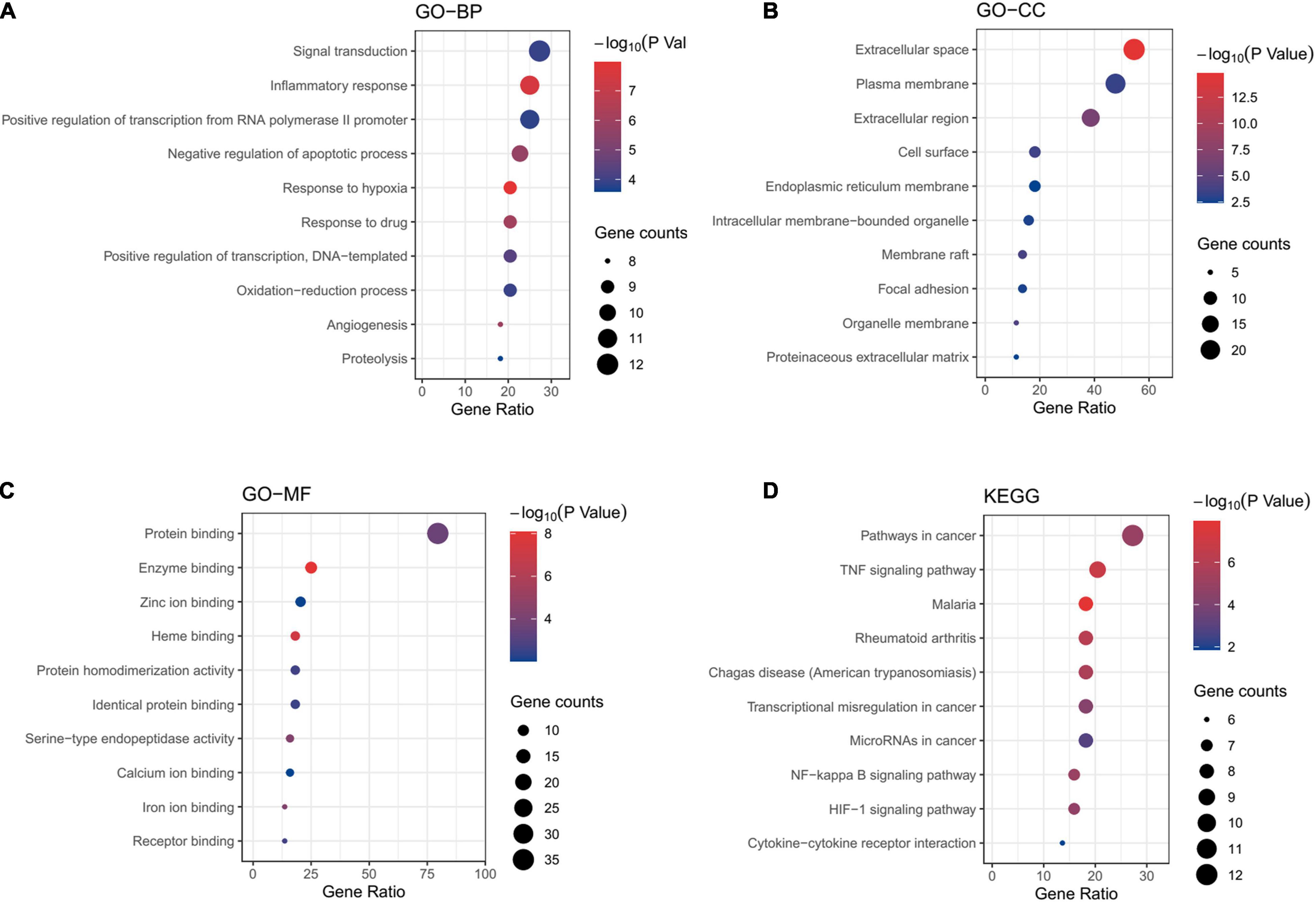
Figure 5. Enrichment analyses of potential targets. GO enrichment analysis. The top 10 terms of each part are shown: (A) GO-BP, biological processes, (B) GO-CC, cell component, (C) GO-MF, molecular function. (D) KEGG pathway analysis.
Molecular Docking Result Analysis
Key compounds and proteins expressed by major hub target genes were used for molecular docking. We used Autodock Vina to calculate the docking affinity score, as shown in Table 2. The Vina scores of critical compounds for treating CHD were all negative and less than -5. The results indicated that quercetin, kaempferol and isorhamnetin had good binding activities to PTGS2, EGFR, MMP2, MMP9 and ESR1. The docking conformations were shown in Figure 6. The additional docking conformations were showed in Supplementary Table 7.
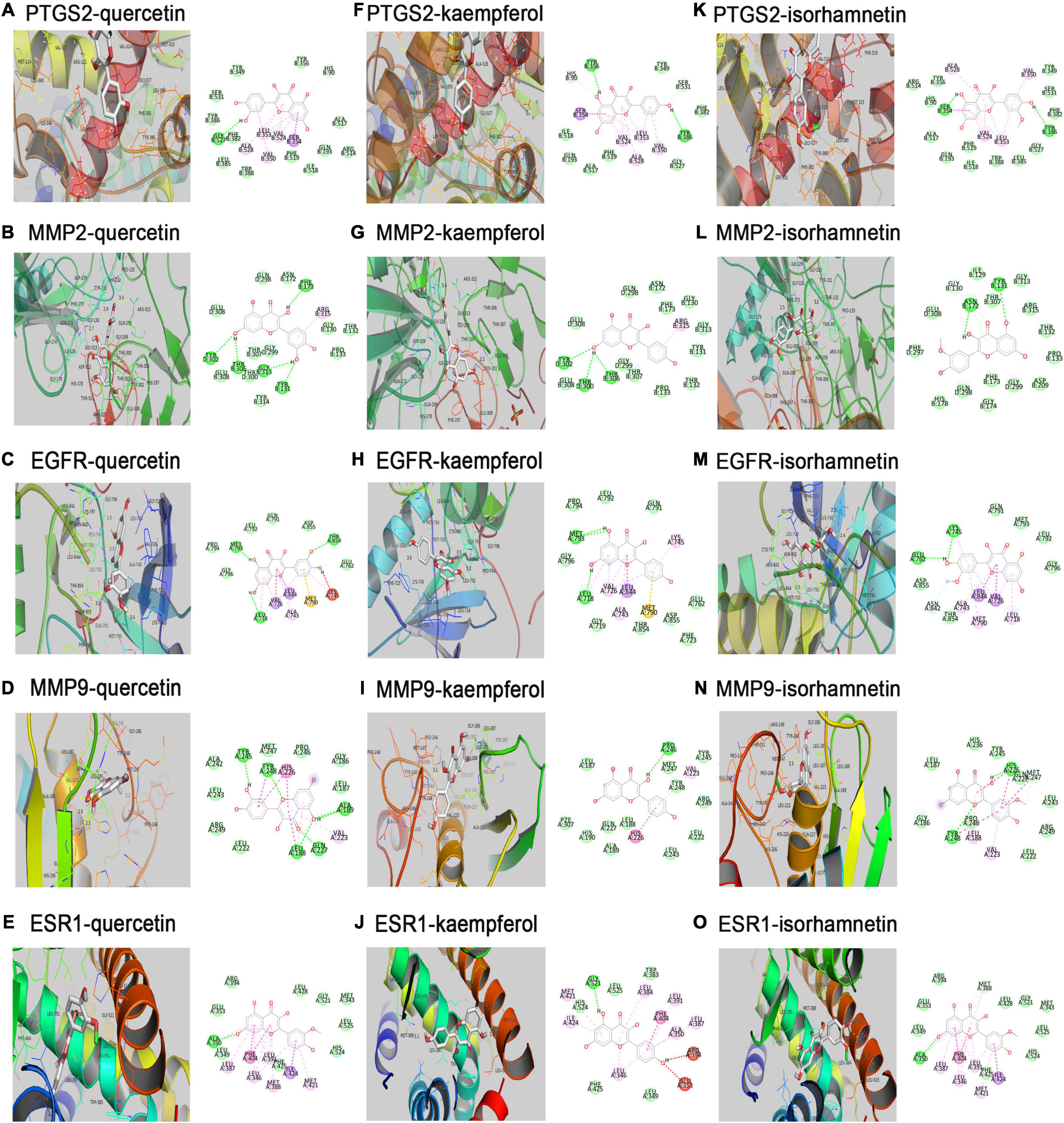
Figure 6. Molecular docking models of effective compounds binding to potential targets. (A–E) Quercetin interacting with PTGS2, MMP2, EGFR, MMP9, ESR1, respectively. (F–J) Kaempferol interacting with PTGS2, MMP2, EGFR, MMP9, ESR1, respectively. (K–O) Isorhamnetin interacting with PTGS2, MMP2, EGFR, MMP9, ESR1, respectively.
Quercetin, Kaempferol, Isorhamnetin Suppressed Lipopolysaccharides- Induced Inflammation and Viability in RAW264.7 Cells
The PTGS2, MMP2, and MMP9 protein levels were significantly increased after exposure to LPS (1 μg/ml), while reversed by quercetin (25, 50, and 100 μM), kaempferol (50, 100, and 200 μM) and isorhamnetin (5, 10, and 20 μM) treatment, respectively, in RAW264.7 cells. However, the expression of ESR1 and EGFR did not change. Moreover, quercetin, kaempferol and isorhamnetin treatment also reduced protein level of IL6, IL1B and TNFα induced by LPS (Figures 7A–F and Supplementary Figures 1A–F). Furthermore, we also detected the effects of these three main compounds on viability of RAW264.7 cells. The data showed that all these compounds could inhibit RAW264.7 cells viability induced by LPS (1 μg/ml) (Figures 7G–I). Overall, the results indicate that the key compounds of hawthorn leaves protect against CHD through anti-inflammatory and inhibiting macrophage viability.
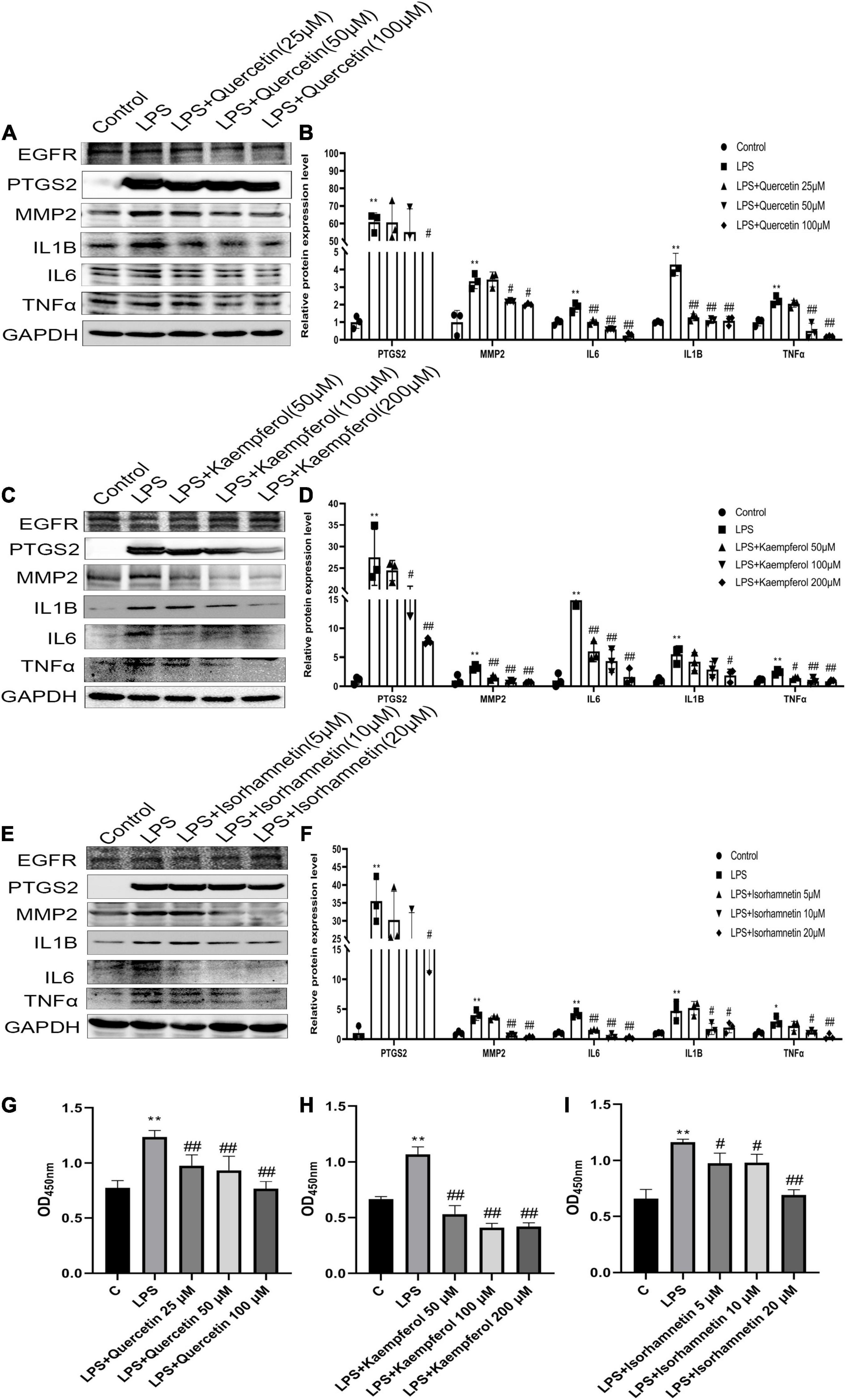
Figure 7. The effective compounds (quercetin, kaempferol, isorhamnetin) attenuate LPS-induced the elevation of PTGS2, MMP2 and pro- inflammation cytokines (IL1B, IL6, TNFα) of RAW264.7 cells. The protein expression of PTGS2, MMP2 and pro- inflammation cytokines (IL1B, IL6, TNFα) of RAW264.7 cells treated with quercetin (A,B), kaempferol (C,D), isorhamnetin (E,F).The viability of RAW264.7 cells treated with quercetin (G), kaempferol (H), isorhamnetin (I). *P < 0.05 vs. Control; **P < 0.01 vs. Control; #P < 0.05 vs. LPS; ##P < 0.01 vs. LPS.
Discussion
In recent years, increasing number of studies dedicated to discover new drugs and combined TCM to treat complex disease such as CHD, network pharmacology approach has been proved to play an important role in these researches. In this study, we built the following network to explore the potential targets and pathways of hawthorn leaves in the treatment of CHD: compound-target network, disease-target network, PPI network of compound-CHD targets and compound-target-pathway network. Furthermore, we verified the effects of three effective compounds on selected targets and possible mechanism in vitro.
After integrating information from different sources of available databases and formulating screening conditions, three main active compounds of hawthorn leaves acted on 44 different targets associated with CHD were screened out. Three compounds such as quercetin, kaempferol and isorhamnetin are highly connected with their supposed targets and can be thought as effective compounds in hawthorn leaves. As a naturally occurring polyphenol, quercetin was reported to possess antioxidant, anti-inflammatory, and antiallergic activity (29, 30). Kaempferol, which belongs to the family of flavonoids, is a secondary plant metabolite with a hydroxyl phenylchromenone structure (31). It was reported to attenuate oxidative stress and inflammatory processes in LPS-induced THP-1 cells by decreasing the mRNA expression of IL-1β, TNF, NF-κB, and IL6 level (32). Isorhamnetin, which is extracted from the fruits of hippophae rhamnoides L and the leaves of Ginkgo biloba L, is an important natural flavonoid (33), and has been shown to possess both anti-microbial and anti-inflammatory properties (34). Therefore, these suggest hawthorn leaves may have beneficial effects on CHD which is characterized by immune inflammatory response.
Actually, consistent with prior researches, the network of CHD targets in our study did reveal the underlying pathogenesis of CHD. Abundant data confirmed that inflammatory mechanisms play a vital role in the occurrence and development of atherosclerosis and CHD (35). Early atherogenesis is characterized by recruitment of leukocyte and expression of pro-inflammatory cytokines, the inflammation also promotes plaque rupture and thrombogenesis, which lead to myocardial infarctions and most strokes (36). Exploring the role of inflammation in atherosclerosis also provides a new perspective on the underlying mechanisms behind the clinical benefits of lipid-lowering therapy. All of these researches suggest that identifying triggers of inflammation and elucidating the details of inflammatory pathways may provide new therapeutic targets for treating CHD (37).
To better elucidate the mechanism of hawthorn leaves in the treatment of CHD, the 44 targets were screened out in the compound-CHD targets. Coincidentally, 14 targets, namely IL6, VEGFA, IL1B, MMP9, CXCL8, CCL2, PTGS2, IL10, ESR1, EGFR, MMP2, CRP, SERPINE1, and ICAM1, have been identified as key pathogenic genes in the PPI network of CHD target proteins. These results reveal that hawthorn leaves might protect against CHD through a multi-targets synergistic way.
As shown by the compound-target gene-pathway network, quercetin, kaempferol and isorhamnetin interacted with lots of targets, indicating their complex and comprehensive roles in the treatment of CHD. To further understand the binding ability between compounds and targets, molecular docking simulation was performed between 14 targets genes and three active compounds to find out major targets. Absolute value of the docking affinity are positively correlated with binding force between the compounds and the active site of targets. The data showed that some of the compound- target pairs had good docking affinity, such as, PTGS2, MMP2, EGFR, MMP9, and ESR1 (Figure 6), which means that the protective effects of hawthorn leaves in CHD may be achieved to some extent by regulating these genes above. PTGS2, a key enzyme in prostaglandin biosynthesis, is involved in prostaglandin biosynthesis and plays a significant role in inflammatory response (38, 39). Prior study has noted that PTGS2 may be potential therapeutic target for CHD (40), but there is no report confirming it. MMP2 and MMP9 are a kind of proteases that have been reported to mediate plaque rupture and inflammation in atherosclerosis (41–43). Previous study showed that selective EGFR deletion in myeloid cells limited their ability to produce IL-6, TNF-α, and to uptake lipids, thereby reducing the development of atherosclerosis (44). ESR1 was also reported to be related to the risk of CHD, one study showed that ESR1 genetic variants were associated with the increased risk of CHD among Finnish men (45). Our results demonstrated that the increased PTGS2, MMP2, and MMP9 protein levels induced by LPS could be reversed by quercetin, kaempferol and isorhamnetin, respectively, in RAW264.7 cells. However, the expression of EGFR and ESR1 did not change. These data suggested that PTGS2, MMP2 and MMP9 might be involved in the protection of hawthorn leaves against CHD. In addition, we added the ROC analysis for PTGS2 and MMP2 genes using logistic regression models based on GSE12288 datasets (Supplementary Figure 1G–J). However, there is no difference in these data, and we think there are several reasons: First, gene expression in circulating leukocytes was measured to identify patients with CAD or the controls in GSE12288 database. Therefore, differential genes do not necessarily play a role in CHD. Similarly, the non-differential genes in the database do not mean that they will not play an important role in the development of CHD. Second, it cannot rule out the possibility that these two genes are expressed differently in the myocardium of CHD patients and normal controls.
The potential therapeutic mechanism was also validated by GO functional annotation and pathway enrichment analysis. First, main BP in GO pathway enrichment analysis of common-targets included signal transduction and the response to inflammatory response, which means most key targets participate in these two biological process; Second, main CC of common-targets included extracellular space, plasma membrane, which means those targets that we may be interested in mostly locate at extracellular space and plasma membrane; Third, main MF of these targets is protein banding, which means the function of these targets is mainly protein binding. Besides, the results of KEGG pathway enrichment analysis showed that effects of hawthorn leaves on CHD may be due to its ability to target varied pathways simultaneously, such as pathways in cancer, TNF signaling pathway, malaria, and so on (Figure 5D). As for TNF signaling pathway, a classic pathway involved in inflammatory response, IL1β, IL6 and TNFα are key members of it. IL6 was verified to implicate in the pathogenesis of CHD, possibly due to its association with inflammation, insulin resistance, and plasma ceramides (46). It has been reported that reducing the production of IL6 mediated by ROS/p38//NF-κB signaling pathway can attenuate atherosclerosis (47). Inhibiting IL-6/STAT3 signaling pathway can protect against high-fat-induced atherosclerosis in ApoE (-/-) mice (47). IL-1β could activate NF-κB signaling pathway (48), leading to inflammation cascades through increasing the production of pro-inflammatory cytokines (TNF-α and IL-6) (49). Our research showed that quercetin, kaempferol and isorhamnetin reduced protein expression of IL-1β, IL6, TNFα induced by LPS in RAW264.7 cells. Additionally, the macrophages activation was suppressed by these three compounds mentioned above. These data further manifest that the effective compounds of hawthorn leaves could inhibit the production of pro-inflammatory cytokines stimulated by LPS in macrophage, confirming that hawthorn leaves may play a therapeutic role in CHD by inhibiting inflammatory pathways. However, about the effect of LPS on the viability of macrophage, there are some different views. Some studies suggested that LPS had no significant effect on the viability of macrophages (50–54), some literatures mentioned that LPS could reduce the viability of macrophages (55, 56), while other studies showed that LPS could increase the viability of macrophages (57, 58). Consistent with the latter, our results showed that LPS could increase the viability of macrophages. Through reviewing the literature, we found that these different results may be related to the differences in the time point or dose of LPS intervention. More confusingly, studies with exactly the same LPS dose and time of intervention had inconsistent results. For example, when RAW264.7 were interfered with 1ug/mL LPS for 24 h in three studies, the first study showed that LPS decreased the viability of cells (55), the second study showed that LPS increased the viability of cells (58), and the third study showed that LPS didn’t affect the viability of cells (53). One possible explanation is that the effectiveness of LPS from different sources may be different. We have to admit that we are puzzled by this complex situation, and further researches may be needed to clarify the causes of these different effects.
The results of our study are consistent with that of previous similar study which explored the effects of hawthorn leaves on different disease models. Fu et al. reported that hawthorn leaves flavonoids could decrease the degree and scope of myocardial ischemia by inhibiting inflammation in anesthetized dogs with acute myocardial ischemia/reperfusion (59). Min et al. reported hawthorn leaves flavonoids could protect against diabetic cardiomyopathy in rats through reducing oxidative stress and inflammation (60). The result of another study also showed that vitexin which is the active ingredient of hawthorn leaves extract could decrease DOX−induced cardio-toxicity by inhibiting inflammation, oxidative stress and apoptosis (61). These results further prove that hawthorn leaves really have a significant inhibitory effect on inflammation. Different from these studies, our study adopted the method of network pharmacology analysis to screen the effective components and action targets of hawthorn leaves in the treatment of CHD, and verified that the anti-inflammatory effect may be the key mechanism of its effect through in vitro experiments. This study further proves that network pharmacology is an effective method to screen the active components and action targets of TCM, and provides methodological inspiration for other studies about TCM in the future.
In fact, besides anti-inflammatory action, non-anti-inflammatory effects have been considered by several studies to be involved in the beneficial effects of hawthorn leaves. Previous studies have shown that hawthorn leaves could improve non-alcoholic fatty liver disease and diabetic cardiomyopathy by inhibiting oxidative stress (60, 62). Another study suggested that hawthorn leaves attenuated hepatic steatosis in high-fat fed rats by enhancing the Adiponectin/AMPK pathway (63). It is worth noting that one study indicated that hawthorn leaves may also have a blood thinning effect in addition to antioxidant stress. Therefore, for patients with heart diseases who require routine antiplatelet and anticoagulant drugs, they should be aware of the risk of bleeding when taking medicine containing hawthorn leaves (64).
However, there are still some limitations in this research. Firstly, as a kind of complex disease, the underlying mechanisms behind the occurrence and progression of CHD are complicated, the mechanism of action of hawthorn leaves in treatment of CHD needs more comprehensive in vitro and in vivo researches. Secondly, although three targets (PTGS2, MMP9 and MMP2) and three inflammatory factors (IL-1β, IL6, TNFα) were verified to be involved in mechanism of action, the potential upstream or downstream relationships between them still need to be further explored. Thirdly, we have only studied the anti-inflammatory effects and possible mechanisms of hawthorn leaves in macrophages, but lack of studies in endothelial cells and smooth muscle cells. Fourth, the results of enrichment analysis suggested that hawthorn leaves may also have non-anti-inflammatory effects, and it may be biased to focus only on its anti-inflammatory effects. Therefore, in order to better understand its potential mechanism of action, the non-anti-inflammatory effects of hawthorn leaves should be further studied in future.
Conclusion
In conclusion, the effective components and key targets of hawthorn leaves for CHD treatment were explored using network pharmacology approach. Quercetin, kaempferol and isorhamnetin were proved to be the main effective compounds in treatment of CHD, possibly by suppressing expression of PTGS2, MMP2, MMP9, inflammatory cytokines and activation of macrophages (Figure 8). The present study provides a more comprehensive understanding of hawthorn leaves on active components and targets, which is conducive to further optimization of the drug in the future.
Data Availability Statement
The original contributions presented in the study are included in the article/Supplementary Material, further inquiries can be directed to the corresponding author/s.
Author Contributions
QF designed the experiments, wrote the manuscript, and conducted the pharmacology information analyses. JW helped to conduct the pharmacology information analyses. SL and MH participated in visualizing the data. HRW helped with the data analyses. JD completed the in vitro experiments and helped to write and review the manuscript. YW organized the study. All authors read and approved the final manuscript.
Funding
This research was supported by the National Natural Science Foundation of China (Nos. 82070383 and 82000340).
Conflict of Interest
The authors declare that the research was conducted in the absence of any commercial or financial relationships that could be construed as a potential conflict of interest.
Publisher’s Note
All claims expressed in this article are solely those of the authors and do not necessarily represent those of their affiliated organizations, or those of the publisher, the editors and the reviewers. Any product that may be evaluated in this article, or claim that may be made by its manufacturer, is not guaranteed or endorsed by the publisher.
Supplementary Material
The Supplementary Material for this article can be found online at: https://www.frontiersin.org/articles/10.3389/fcvm.2022.804801/full#supplementary-material
Supplementary Figure 1 | The effective compounds (quercetin, kaempferol, isorhamnetin) attenuate LPS-induced the elevation of MMP9 in RAW264.7 cells. The protein expression of MMP9 and ESR1 of RAW264.7 cells treated with quercetin (A,B), kaempferol (C,D), isorhamnetin (E,F). Analysis of gene differential expression and ROC analyses for PTGS2 and MMP2 genes based on the data from GSE12288 (G–J). *P < 0.05 vs. Control; **P < 0.01 vs. Control; #P < 0.05 vs. LPS; ##P < 0.01 vs. LPS.
Abbreviations
CHD, coronary heart disease; LPS, lipopolysaccharides; TCM, Traditional Chinese Medicine; TCMSP, Traditional Chinese Medicine Systems Pharmacology; ADME, absorption distribution, metabolism and excretion; OB, oral bioavailability; DL, drug-likeness; OMIM, Online Mendelian Inheritance in Man; PPI, Protein-protein interaction; GO, Gene Ontology; KEGG, Kyoto Encyclopedia of Genes and Genomes; MF, molecular function; BP, biological process; CC, cellular components; CAD, Coronary artery disease; PyMol, PyMol Molecular; DMEM, Dulbecco’s modified Eagle medium; OD, optical density; ANOVA, one-way analysis of variance.
Footnotes
- ^ https://old.tcmsp-e.com/tcmsp.php
- ^ http://www.symmap.org/
- ^ http://pubchem.ncbi.nlm.nih.gov
- ^ http://www.swisstargetprediction.ch/
- ^ http://www.uniprot.org/
- ^ http://omim.org/
- ^ https://www.drugbank.ca/
- ^ https://www.genecards.org/
- ^ https://www.disgenet.org/home/
- ^ https://string-db.org/
- ^ https://david.ncifcrf.gov/search.jsp
- ^ https://hiplot.org
- ^ ncbi.nlm.nih.gov
References
1. Temporelli PL, Arca M, D’Erasmo L, De Caterina R. Lipid-lowering therapy in patients with coronary heart disease and prior stroke: mission impossible? J Clin Med. (2021) 10:886. doi: 10.3390/jcm10040886
2. Agewall S, Giannitsis E. Troponin elevation in coronary ischemia and necrosis. Curr Atheroscler Rep. (2014) 16:396. doi: 10.1007/s11883-013-0396-7
3. Dumler S, Krötz F, Siegmund T. [Which antidiabetic drugs improve coronary heart disease and heart failure?]. MMW Fortschr Med. (2021) 163:42–6. doi: 10.1007/s15006-021-9642-z
4. Ding M, Ma W, Wang X, Chen S, Zou S, Wei J, et al. A network pharmacology integrated pharmacokinetics strategy for uncovering pharmacological mechanism of compounds absorbed into the blood of Dan-Lou tablet on coronary heart disease. J Ethnopharmacol. (2019) 242:112055. doi: 10.1016/j.jep.2019.112055
5. Zhao Y, Nie S, Yi M, Wu N, Wang W, Zhang Z, et al. UPLC-QTOF/MS-based metabolomics analysis of plasma reveals an effect of Xue-Fu-Zhu-Yu capsules on blood-stasis syndrome in CHD rats. J Ethnopharmacol. (2019) 241:111908. doi: 10.1016/j.jep.2019.111908
6. Duan B, Han L, Ming S, Li J, Wang Q, Zhang D, et al. Fuling-guizhi herb pair in coronary heart disease: integrating network pharmacology and in vivo pharmacological evaluation. Evid Based Complement Alternat Med. (2020) 2020:1489036. doi: 10.1155/2020/1489036
7. Gao P, Li S, Liu K, Sun C, Song S, Li L. Antiplatelet aggregation and antithrombotic benefits of terpenes and flavones from hawthorn leaf extract isolated using the activity-guided method. Food Funct. (2019) 10:859–66. doi: 10.1039/c8fo01862f
8. Dong P, Pan L, Zhang X, Zhang W, Wang X, Jiang M, et al. Hawthorn (Crataegus pinnatifida Bunge) leave flavonoids attenuate atherosclerosis development in apoE knock-out mice. J Ethnopharmacol. (2017) 198:479–88. doi: 10.1016/j.jep.2017.01.040
9. Hu YH, Peng LQ, Wang QY, Yang J, Dong X, Wang SL, et al. Ecofriendly microwave-assisted reaction and extraction of bioactive compounds from hawthorn leaf. Phytochem Anal. (2019) 30:710–9. doi: 10.1002/pca.2849
10. Koch E, Malek FA. Standardized extracts from hawthorn leaves and flowers in the treatment of cardiovascular disorders–preclinical and clinical studies. Planta Med. (2011) 77:1123–8. doi: 10.1055/s-0030-1270849
12. Fang HY, Zeng HW, Lin LM, Chen X, Shen XN, Fu P, et al. A network-based method for mechanistic investigation of Shexiang Baoxin Pill’s treatment of cardiovascular diseases. Sci Rep. (2017) 7:43632. doi: 10.1038/srep43632
13. Li C, Zhang WY, Yu Y, Cheng CS, Han JY, Yao XS, et al. Discovery of the mechanisms and major bioactive compounds responsible for the protective effects of Gualou Xiebai Decoction on coronary heart disease by network pharmacology analysis. Phytomedicine. (2019) 56:261–8. doi: 10.1016/j.phymed.2018.11.010
14. Li F, Duan J, Zhao M, Huang S, Mu F, Su J, et al. A network pharmacology approach to reveal the protective mechanism of Salvia miltiorrhiza-Dalbergia odorifera coupled-herbs on coronary heart disease. Sci Rep. (2019) 9:19343. doi: 10.1038/s41598-019-56050-5
15. Cheng F, Desai RJ, Handy DE, Wang R, Schneeweiss S, Barabási AL, et al. Network-based approach to prediction and population-based validation of in silico drug repurposing. Nat Commun. (2018) 9:2691. doi: 10.1038/s41467-018-05116-5
16. Cheng F, Kovács IA, Barabási AL. Network-based prediction of drug combinations. Nat Commun. (2019) 10:1197. doi: 10.1038/s41467-019-09186-x
17. Ru J, Li P, Wang J, Zhou W, Li B, Huang C, et al. TCMSP: a database of systems pharmacology for drug discovery from herbal medicines. J Cheminform. (2014) 6:13. doi: 10.1186/1758-2946-6-13
18. Kim S, Chen J, Cheng T, Gindulyte A, He J, He S, et al. PubChem 2019 update: improved access to chemical data. Nucleic Acids Res. (2019) 47:D1102–9. doi: 10.1093/nar/gky1033
19. Gfeller D, Grosdidier A, Wirth M, Daina A, Michielin O, Zoete V. SwissTargetPrediction: a web server for target prediction of bioactive small molecules. Nucleic Acids Res. (2014) 42:W32–8. doi: 10.1093/nar/gku293
20. Boyadjiev SA, Jabs EW. Online Mendelian Inheritance in Man (OMIM) as a knowledgebase for human developmental disorders. Clin Genet. (2000) 57:253–66. doi: 10.1034/j.1399-0004.2000.570403.x
21. Wishart DS, Knox C, Guo AC, Cheng D, Shrivastava S, Tzur D, et al. DrugBank: a knowledgebase for drugs, drug actions and drug targets. Nucleic Acids Res. (2008) 36:D901–6. doi: 10.1093/nar/gkm958
22. Rebhan M, Chalifa-Caspi V, Prilusky J, Lancet D. GeneCards: a novel functional genomics compendium with automated data mining and query reformulation support. Bioinformatics. (1998) 14:656–64. doi: 10.1093/bioinformatics/14.8.656
23. Piñero J, Ramírez-Anguita JM, Saüch-Pitarch J, Ronzano F, Centeno E, Sanz F, et al. The DisGeNET knowledge platform for disease genomics: 2019 update. Nucleic Acids Res. (2020) 48:D845–55. doi: 10.1093/nar/gkz1021
24. Zhao H, Shan Y, Ma Z, Yu M, Gong B. A network pharmacology approach to explore active compounds and pharmacological mechanisms of epimedium for treatment of premature ovarian insufficiency. Drug Des Devel Ther. (2019) 13:2997–3007. doi: 10.2147/dddt.s207823
25. Pasi M, Tiberti M, Arrigoni A, Papaleo E. xPyder: a PyMOL plugin to analyze coupled residues and their networks in protein structures. J Chem Inf Model. (2012) 52:1865–74. doi: 10.1021/ci300213c
26. Morris GM, Huey R, Lindstrom W, Sanner MF, Belew RK, Goodsell DS, et al. AutoDock4 and AutoDockTools4: automated docking with selective receptor flexibility. J Comput Chem. (2009) 30:2785–91. doi: 10.1002/jcc.21256
27. Trott O, Olson AJ. AutoDock Vina: improving the speed and accuracy of docking with a new scoring function, efficient optimization, and multithreading. J Comput Chem. (2010) 31:455–61. doi: 10.1002/jcc.21334
28. Li X, Xu X, Wang J, Yu H, Wang X, Yang H, et al. A system-level investigation into the mechanisms of Chinese traditional medicine: compound Danshen formula for cardiovascular disease treatment. PLoS One. (2012) 7:e43918. doi: 10.1371/journal.pone.0043918
29. Karuppagounder V, Arumugam S, Thandavarayan RA, Sreedhar R, Giridharan VV, Watanabe K. Molecular targets of quercetin with anti-inflammatory properties in atopic dermatitis. Drug Discov Today. (2016) 21:632–9. doi: 10.1016/j.drudis.2016.02.011
30. Sadalage PS, Patil RV, Havaldar DV, Gavade SS, Santos AC, Pawar KD. Optimally biosynthesized, PEGylated gold nanoparticles functionalized with quercetin and camptothecin enhance potential anti-inflammatory, anti-cancer and anti-angiogenic activities. J Nanobiotechnology. (2021) 19:84. doi: 10.1186/s12951-021-00836-1
31. Hu WH, Dai DK, Zheng BZ, Duan R, Chan GK, Dong TT, et al. The binding of kaempferol-3-O-rutinoside to vascular endothelial growth factor potentiates anti-inflammatory efficiencies in lipopolysaccharide-treated mouse macrophage RAW264.7 cells. Phytomedicine. (2021) 80:153400. doi: 10.1016/j.phymed.2020.153400
32. Hofer S, Geisler S, Lisandrelli R, Nguyen Ngoc H, Ganzera M, Schennach H, et al. Pharmacological targets of kaempferol within inflammatory pathways-a hint towards the central role of tryptophan metabolism. Antioxidants. (2020) 9:180. doi: 10.3390/antiox9020180
33. Gong G, Guan YY, Zhang ZL, Rahman K, Wang SJ, Zhou S, et al. Isorhamnetin: a review of pharmacological effects. Biomed Pharmacother. (2020) 128:110301. doi: 10.1016/j.biopha.2020.110301
34. Tian X, Peng X, Lin J, Zhang Y, Zhan L, Yin J, et al. Isorhamnetin Ameliorates Aspergillus fumigatus keratitis by reducing fungal load, inhibiting pattern-recognition receptors and inflammatory cytokines. Invest Ophthalmol Vis Sci. (2021) 62:38. doi: 10.1167/iovs.62.3.38
35. Gao W, Yang H, Liu X, Liu Z, Tong L, Sun Y, et al. Reductively dissociable biomimetic nanoparticles for control of integrin-coupled inflammatory signaling to retard atherogenesis. Chem Commun. (2019) 55:11535–8. doi: 10.1039/c9cc06039a
36. van Leent MMT, Beldman TJ, Toner YC, Lameijer MA, Rother N, Bekkering S, et al. Prosaposin mediates inflammation in atherosclerosis. Sci Transl Med. (2021) 13:eabe1433. doi: 10.1126/scitranslmed.abe1433
37. Libby P. Inflammation in atherosclerosis-no longer a theory. Clin Chem. (2021) 67:131–42. doi: 10.1093/clinchem/hvaa275
38. Ruan Z, Wang S, Yu W, Deng F. LncRNA MALAT1 aggravates inflammation response through regulating PTGS2 by targeting miR-26b in myocardial ischemia-reperfusion injury. Int J Cardiol. (2019) 288:122. doi: 10.1016/j.ijcard.2019.04.015
39. Mursalin MH, Coburn PS, Miller FC, Livingston ET, Astley R, Callegan MC. Innate immune interference attenuates inflammation in Bacillus endophthalmitis. Invest Ophthalmol Vis Sci. (2020) 61:17. doi: 10.1167/iovs.61.13.17
40. Huo M, Wang Z, Wu D, Zhang Y, Qiao Y. Using coexpression protein interaction network analysis to identify mechanisms of danshensu affecting patients with coronary heart disease. Int J Mol Sci. (2017) 18:1298. doi: 10.3390/ijms18061298
41. Kai H, Ikeda H, Yasukawa H, Kai M, Seki Y, Kuwahara F, et al. Peripheral blood levels of matrix metalloproteases-2 and -9 are elevated in patients with acute coronary syndromes. J Am Coll Cardiol. (1998) 32:368–72. doi: 10.1016/s0735-1097(98)00250-2
42. Fitzsimmons PJ, Forough R, Lawrence ME, Gantt DS, Rajab MH, Kim H, et al. Urinary levels of matrix metalloproteinase 9 and 2 and tissue inhibitor of matrix metalloproteinase in patients with coronary artery disease. Atherosclerosis. (2007) 194:196–203. doi: 10.1016/j.atherosclerosis.2006.07.027
43. Volcik KA, Campbell S, Chambless LE, Coresh J, Folsom AR, Mosley TH, et al. MMP2 genetic variation is associated with measures of fibrous cap thickness: the atherosclerosis risk in communities carotid MRI study. Atherosclerosis. (2010) 210:188–93. doi: 10.1016/j.atherosclerosis.2009.12.006
44. Zeboudj L, Giraud A, Guyonnet L, Zhang Y, Laurans L, Esposito B, et al. Selective EGFR (Epidermal Growth Factor Receptor) deletion in myeloid cells limits atherosclerosis-brief report. Arterioscler Thromb Vasc Biol. (2018) 38:114–9. doi: 10.1161/atvbaha.117.309927
45. Kunnas T, Silander K, Karvanen J, Valkeapää M, Salomaa V, Nikkari S. ESR1 genetic variants, haplotypes and the risk of coronary heart disease and ischemic stroke in the Finnish population: a prospective follow-up study. Atherosclerosis. (2010) 211:200–2. doi: 10.1016/j.atherosclerosis.2010.01.026
46. de Mello VD, Lankinen M, Schwab U, Kolehmainen M, Lehto S, Seppänen-Laakso T, et al. Link between plasma ceramides, inflammation and insulin resistance: association with serum IL-6 concentration in patients with coronary heart disease. Diabetologia. (2009) 52:2612–5. doi: 10.1007/s00125-009-1482-9
47. Luo P, Shi W, Wang Y, Ma H, Liu T, Yan D, et al. Raloxifene inhibits IL-6/STAT3 signaling pathway and protects against high-fat-induced atherosclerosis in ApoE(-/-) mice. Life Sci. (2020) 261:118304. doi: 10.1016/j.lfs.2020.118304
48. Ren HY, Huang GL, Liu WM, Zhang W, Liu Y, Su GQ, et al. IL-1β induced RXRα overexpression through activation of NF-κB signaling in gastric carcinoma. Biomed Pharmacother. (2016) 78:329–34. doi: 10.1016/j.biopha.2016.01.033
49. Luo Y, Zheng SG. Hall of fame among pro-inflammatory cytokines: interleukin-6 gene and its transcriptional regulation mechanisms. Front Immunol. (2016) 7:604. doi: 10.3389/fimmu.2016.00604
50. Tardy FM, Tucci M, Baker R, Benghuzzi H. The evaluation of macrophages following bacterial or cytokine challenge. Biomed Sci Instrum. (2008) 44: 428–33.
51. Neranjan Tharuka MD, Bathige S, Oh M, Lee S, Kim MJ, Priyathilaka TT, et al. Molecular characterization and expression analysis of big-belly seahorse (Hippocampus abdominalis) interleukin-10 and analysis of its potent anti-inflammatory properties in LPS-induced murine macrophage RAW 264.7 cells. Gene. (2019) 685:1–11. doi: 10.1016/j.gene.2018.10.053
52. Park T, Park JS, Sim JH, Kim SY. 7-Acetoxycoumarin inhibits LPS-induced inflammatory cytokine synthesis by IkappaBalpha degradation and MAPK activation in macrophage cells. Molecules. (2020) 25:3124. doi: 10.3390/molecules25143124
53. Bonvini A, Rogero MM, Coqueiro AY, Raizel R, Bella LM, Fock RA, et al. Effects of different branched-chain amino acids supplementation protocols on the inflammatory response of LPS-stimulated RAW 264.7 macrophages. Amino Acids. (2021) 53:597–607. doi: 10.1007/s00726-021-02940-w
54. Karthikeyan A, Kim HH, Preethi V, Moniruzzaman M, Lee KH, Kalaiselvi S, et al. Assessment of Anti-Inflammatory and Antioxidant Effects of Citrus unshiu Peel (CUP) Flavonoids on LPS-Stimulated RAW 264.7 Cells. Plants. (2021) 10:2209. doi: 10.3390/plants10102209
55. Han JH, Jung J, Hwang L, Ko IG, Nam OH, Kim MS, et al. Anti-inflammatory effect of polydeoxyribonucleotide on zoledronic acid-pretreated and lipopolysaccharide-stimulated RAW 264.7 cells. Exp Ther Med. (2018) 16:400–5. doi: 10.3892/etm.2018.6186
56. Aresta A, De Santis S, Carocci A, Barbarossa A, Ragusa A, De Vietro N, et al. Determination of commercial animal and vegetable milks’ lipid profile and its correlation with cell viability and antioxidant activity on human intestinal Caco-2 cells. Molecules. (2021) 26:5645. doi: 10.3390/molecules26185645
57. Rozenberg K, Wollman A, Ben-Shachar M, Argaev-Frenkel L, Rosenzweig T. Anti-inflammatory effects of Sarcopoterium spinosum extract. J Ethnopharmacol. (2020) 249:112391. doi: 10.1016/j.jep.2019.112391
58. Ai M, Lin S, Zhang M, Wu T, Yang N, Li Y, et al. Cirsilineol attenuates LPS-induced inflammation in both in vivo and in vitro models via inhibiting TLR-4/NFkB/IKK signaling pathway. J Biochem Mol Toxicol. (2021) 35:e22799. doi: 10.1002/jbt.22799
59. Fu JH, Zheng YQ, Li P, Li XZ, Shang XH, Liu JX. Hawthorn leaves flavonoids decreases inflammation related to acute myocardial ischemia/reperfusion in anesthetized dogs. Chin J Integr Med. (2013) 19:582–8. doi: 10.1007/s11655-012-1250-4
60. Min Q, Bai Y, Zhang Y, Yu W, Zhang M, Liu D, et al. Hawthorn leaf flavonoids protect against diabetes-induced cardiomyopathy in rats via PKC-α signaling pathway. Evid Based Complement Alternat Med. (2017) 2017:2071952. doi: 10.1155/2017/2071952
61. Sun Z, Yan B, Yu WY, Yao X, Ma X, Sheng G, et al. Vitexin attenuates acute doxorubicin cardiotoxicity in rats via the suppression of oxidative stress, inflammation and apoptosis and the activation of FOXO3a. Exp Ther Med. (2016) 12:1879–84. doi: 10.3892/etm.2016.3518
62. Li XD, Chen ZY, Yu JS, Wang DJ, Yan MX. [Effect of total flavones of hawthorn leafonon expression of COX-2/Nrf2 in liver of rats with nonalcoholic steatohepatitis]. Zhongguo Zhong Yao Za Zhi. (2016) 41:711–5. doi: 10.4268/cjcmm20160428
63. Li Z, Xu J, Zheng P, Xing L, Shen H, Yang L, et al. Hawthorn leaf flavonoids alleviate nonalcoholic fatty liver disease by enhancing the adiponectin/AMPK pathway. Int J Clin Exp Med. (2015) 8:17295–307.
Keywords: hawthorn leaves, coronary heart disease, network pharmacology, molecular docking, inflammation
Citation: Ding J, Wu J, Wei H, Li S, Huang M, Wang Y and Fang Q (2022) Exploring the Mechanism of Hawthorn Leaves Against Coronary Heart Disease Using Network Pharmacology and Molecular Docking. Front. Cardiovasc. Med. 9:804801. doi: 10.3389/fcvm.2022.804801
Received: 07 December 2021; Accepted: 24 May 2022;
Published: 16 June 2022.
Edited by:
Jun Yu, Temple University, United StatesReviewed by:
Yifeng Zhang, Jiangxi University of Traditional Chinese Medicine, ChinaSanjay H. Deshpande, Regional Centre for Biotechnology (RCB), India
Copyright © 2022 Ding, Wu, Wei, Li, Huang, Wang and Fang. This is an open-access article distributed under the terms of the Creative Commons Attribution License (CC BY). The use, distribution or reproduction in other forums is permitted, provided the original author(s) and the copyright owner(s) are credited and that the original publication in this journal is cited, in accordance with accepted academic practice. No use, distribution or reproduction is permitted which does not comply with these terms.
*Correspondence: Qin Fang, ZmFuZ3FpbjE0MDcxNkAxMjYuY29t