- 1School of Sciences and Humanities, Nazarbayev University, Nur-Sultan, Kazakhstan
- 2Core Facilities, Nazarbayev University, Nur-Sultan, Kazakhstan
- 3National Research Center for Cardiac Surgery, Nur-Sultan, Kazakhstan
- 4School of Biomedical Sciences, The Chinese University of Hong Kong, Hong Kong, China
- 5Harvard Medical School, Brigham and Women's Hospital, Boston, MA, United States
Introduction: Coagulation parameters are important determinants for COVID-19 infection. We conducted meta-analysis to assess the association between early hemostatic parameters and infection severity.
Methods: Electronic search was made for papers that addressed clinical characteristics of COVID-19 patients and disease severity. Results were filtered using exclusion and inclusion criteria and then pooled into a meta-analysis to estimate the standardized mean difference (SMD) with 95% confidence interval (CI) for D-dimers, fibrinogen, prothrombin time, platelet count (PLT), activated partial thromboplastin time. To explore the heterogeneity and robustness of our fundings, sensitivity and subgroup analyses were conducted. Publication bias was assessed with contour-enhanced funnel plots and Egger's test by linear regression. Coagulation parameters data from retrospective cohort study of 451 patients with COVID-19 at National Research Center for Cardiac Surgery were included in meta-analysis of published studies.
Results: Overall, 41 original studies (17,601 patients) on SARS-CoV-2 were included. For the two groups of patients, stratified by severity, we identified that D-dimers, fibrinogen, activated partial thromboplastin time, and prothrombin time were significantly higher in the severe group [SMD 0.6985 with 95%CI (0.5155; 0.8815); SMD 0.661 with 95%CI (0.3387; 0.9833); SMD 0.2683 with 95%CI (0.1357; 0.4009); SMD 0.284 with 95%CI (0.1472; 0.4208)]. In contrast, PLT was significantly lower in patients with more severe cases of COVID-19 [SMD −0.1684 with 95%CI (−0.2826; −0.0542)]. Neither the analysis by the leave-one-out method nor the influence diagnostic have identified studies that solely cause significant change in the effect size estimates. Subgroup analysis showed no significant difference between articles originated from different countries but revealed that severity assessment criteria might have influence over estimated effect sizes for platelets and D-dimers. Contour-enhanced funnel plots and the Egger's test for D-dimers and fibrinogen revealed significant asymmetry that might be a sign of publication bias.
Conclusions: The hemostatic laboratory parameters, with exception of platelets, are significantly elevated in patients with severe COVID-19. The two variables with strongest association to disease severity were D-dimers and fibrinogen levels. Future research should aim outside conventional coagulation tests and include analysis of clotting formation and platelet/platelet progenitors characteristics.
Introduction
In <2 years of the outbreak, the COVID-19 pandemic took almost five million lives (1). SARS-CoV-2 is the seventh member of the large coronavirus family capable of inducing human disease (2). Viral respiratory infections, including severe respiratory acute syndrome coronavirus (SARS-CoV), Middle East respiratory syndrome coronavirus (MERS-CoV), SARS-CoV-2, may induce coagulopathy and lead to intravascular thrombi and deposition of fibrinogen (3–7).
The coagulation system is activated and dysregulated during COVID-19 infection; however, characteristics of COVID-19 associated coagulopathy are different from other coagulation disorders (8). Evidence of abnormal COVID-19-associated coagulation parameters has already appeared in early publications from Wuhan (9–12) and was supported by further publications worldwide [rev. (8, 13)]. The reported hemostatic abnormalities with COVID-19 infection include increased D-dimers (DD) levels (14, 15), fibrinogen levels (16, 17), changes in the quantities and levels of activation of platelets (14, 18–20), elevated von Willebrand factor (VWF) [(21, 22), rev. by Becker et al. (23)] and other coagulation parameters. Persistently elevated risk of thromboembolic events, even with the initiation of prophylactic anti-coagulation, suggest the presence of hypofibrinolysis, in addition to detected hypercoagulability in COVID-19 (24, 25). It is also consistent with the results of thromboelastography (TEG) reported by Wright et al. (26).
There is a need for early detection of elevated coagulation biomarkers to optimize risk stratification of patients with COVID-19. Importantly, multiple findings suggest that thromboembolic complications should be considered as a cause of clinical deterioration in severe COVID-19 infection and potentially beyond hospital discharge (27–29). However, due to the limitations of early pandemic research with small study sizes and highly heterogeneous datasets, it is still debated. The aim of the current study was to evaluate the validity of stratification based on early coagulation parameters provided by different clinical studies worldwide. We also aimed to use subgroup analysis to explore whether differences in patient subpopulations partly explained heterogeneity in results. The coagulation parameters of our own cohort were included in a systematic meta-analysis of published studies. Furthermore, we also discuss a complex mechanism of hemostatic dysregulation that fuels the coagulation cycle.
Materials and Methods
In this study, we aimed to identify the relationship between coagulation biomarkers taken at admission and severity of COVID-19 in adult (above 18) patients by estimating the effect size of five laboratory coagulation tests: D-dimers (DD), platelet count (PLT), fibrinogen (FIB), activated partial thromboplastin time (APTT), and prothrombin time (PT).
Search Strategy, Exclusion, and Inclusion Criteria
To broaden our search, we included Ovid, PubMed, Web of Sciences, and Google Scholar in the study for the period ending September 30th, 2021. They were thoroughly scanned using the following keywords: SARS-CoV-2, COVID-19, coagulation, severity, characteristics, features, D-dimers, platelets, fibrinogen, cohort, observational, retrospective. First, satisfying search results were exported to an Excel table, and duplicates were eliminated. Second, we conducted a preliminary review of retrieved articles' abstracts. Papers that focused on pregnant women, children, or specific age groups were avoided. Similarly, works that focused on the effect of medical treatment or patients with particular commodities were not included in the meta-analysis. We also excluded studies that compared mortality among groups of COVID-19 patients. While diagnostic and classification of COVID-19 severity follow official guidelines, the differentiation between whether COVID-19 caused deaths might be ambiguous. In order to avoid such bias, we only considered studies that grouped patients according to the severity of their symptoms. Then, each publication was checked according to the inclusion criteria: sample size, severity assessment criteria, presence of at least one coagulation marker of interest expressed as a continuous variable. To be included, a study should have had more than 15 patients and divided them according to the disease severity—WHO guidelines, intensive care unit (ICU) admission, disease aggravation, or need for oxygen therapy. Next, a list of biomarkers should have included at least some of the coagulation parameters: PLT, DD, FIB, APTT, PT, thrombin time (TT), Activated thrombin (AT), Factor VIII (FVIII), and VWF. Finally, the quality of selected articles was assessed with the NIH Quality Assessment Tool for Observational Cohort and Cross-Sectional Studies (30) (Supplementary Table 1). Questions 5, 6, 12, 13 were not applicable to selected studies due to their observational/retrospective nature; Question 8 was not applicable to studies with dichotomous exposure values, like ICU admission and need for oxygen therapy. Hence, studies with a quality score of <5 were excluded from the analysis. Search and quality check were conducted independently by two authors. Disagreements were resolved during a joint discussion with a third author.
Data Extraction
The primary goal was to retrieve data from each article about two groups of patients differing in disease severity by: group size, stratification criteria, and results of the laboratory tests. Apart from these, we kept a record of the country, hospital, and admission time to identify and exclude duplicate patients.
Cohort From the National Research Center for Cardiac Surgery
Participants and Data Collection
From a large cohort of 560 patients consecutively admitted from June 2020 to August 2021 due to coronavirus infection to the cardiology department of the National Research Center for Cardiac Surgery, Nur-Sultan, Kazakhstan, we selected 451 patients with a confirmed diagnosis of COVID-19, aged ≥18 years, and those with the onset of the disease ≤ 21 days, all of whom were hospitalized for at least 24 h with COVID-19. All demographic, clinical, laboratory data were extracted from the electronic records of the NRCSC. Laboratory tests included PLT, PT, international normalized ratio, APTT, FIB, DD. Blood samples were collected at admission by the clinical team. Clotting tests were performed according to standard methods. For coagulation tests (PT, international normalized ratio, APTT, FIB), we used AVATUBE® with sodium citrate, and it was measured on Sysmex CS-2500 (Sysmex, Japan). Blood for DD was collected in AVATUBE® with sodium citrate, and D-dimers were quantified on Cobas® 6000. PLT counts were measured on Sysmex XS-500 (Sysmex, Japan) with blood collection performed in AVATUBE® with K2 EDTA. The confirmation of SARS-CoV-2 infection was done by real-time quantitative reverse-transcription polymerase chain reaction (RT-PCR) assay on nose/throat swab or sputum samples using CFX96® Real-Time System (Bio-Rad Laboratories, Inc., USA).
Clinical Assessment
The COVID-19 population was initially divided into four groups according to disease severity following local guidelines “Diagnosis and treatment of Covid-19 in Adults” (http://www.rcrz.kz/index.php/ru/2017-03-12-10-51-13/klinicheskie-protokoly) (Supplementary Data 1). The study protocol was approved by the medical ethics committee of the National Research Center for Cardiac Surgery, Nur-Sultan, Kazakhstan waiving the need for written informed consent due to the retrospective design.
Data Analysis
Data Transformation
All data analysis was performed in the RStudio 1.4.1717 with R version 4.1.0 (Integrated Development Environment for R. RStudio, PBC, USA). In most articles, results were reported as the first, second (median), and third quartiles, reflecting that original data might be abnormally distributed. Since the authors of the primary studies do not report the underlying distributions, and there are no established methods to analyze the difference of medians, we performed data transformation according to the method described by McGrath et al. (31). The workflow takes advantage of the formulas proposed by Luo et al. (32) and Wan et al. (33) for estimation of mean and standard deviation, respectively. Both methods assume the input data to be normally distributed, so the package also includes the preceding transformation step by the Box-Cox method. In the scope of this meta-analysis, we compare two groups: more and less severe. Patients classified as severe, critical, admitted to ICU, with observed aggravation of a disease, or in need of oxygen therapy were assigned to the first group. The second group included mild, moderate, outpatients, general ward patients, recovered and stable patients (Supplementary Table 2). If, for example, a primary study had more than two groups—mild and moderate, severe and critical—then mild patients were combined with moderate, and severe were combined with critical.
Meta-Analysis
The “meta” and “metafor” packages were used for the meta-analysis. Heterogeneity was assessed using the I2, tau-squared, and prediction intervals. Following the recommendations of Veroniki et al. (34), Paule-Mandel (PM) tau-squared estimator and Q-Profile (QP) tau-squared confidence interval estimator were used in this meta-analysis. To account for different measurement scales, the standardized mean difference (SMD), or Hedges g was estimated. Before pooling point estimates, each study was assigned a weight based on inverse variance approach—smaller variance gives higher precision, thus yielding greater weight for a study. For datasets with high heterogeneity, the Knapp-Hartung adjusted random-effects model is implemented. In case of low heterogeneity, the fixed-effects model is applied.
Sensitivity and Subgroup Analysis
We also performed several sensitivity analyses using the “dmetar” package. First, we recalculated the effect size using the leave-one-out method. Second, basic outliers, studies whose confidence intervals do not overlap with the pooled effect confidence interval, were removed. Third, we ran the diagnostic for influential cases with Graphic Display of Study Heterogeneity (GOSH) plots and excluded them from the meta-analysis. To further explore the heterogeneity of the data, we conducted subgroup analyses: based on grouping criteria and location.
Publication Bias
We used the counter-enhanced Funnel plots and Egger's test by linear regression to assess the possibility for publication bias in selected studies.
Results
Results of the Web Search
The stepwise process of selection is depicted in Figure 1. Initially, there were 4,056 papers that matched search parameters. After the removal of duplicates and studies that did not meet the exclusion criteria, only 195 unique studies remained. A more thorough review based on the inclusion criteria has proven 125 studies to be unfitting. In addition to 70 that were left, 15 studies were extracted from the references lists of previously published meta-analyses and systematic reviews. Finally, we performed a quality check and removed duplicate patients, which resulted in 40 studies being included in the meta-analysis. Besides original articles, we have included data from the National Research Center for Cardiac Surgery located in Nur-Sultan, Kazakhstan. This data set included data from 451 patients with acute COVID-19.
Description of Selected Studies
Overall, this meta-analysis comprises 17,601 patients from 40 research papers and the NRCSC. Of the total, 24 studies originated from China, while the rest included data from France, Germany, Italy, Mexico, Singapore, South Korea, Turkey, the UK, and the USA. Most of the articles reported the division of patients according to the WHO guidelines. Out of the rest, 12 articles made groups based on the ICU admission, three focused on disease aggravation, and three on the need for oxygen therapy. This information is depicted in Figure 2 in more detail.
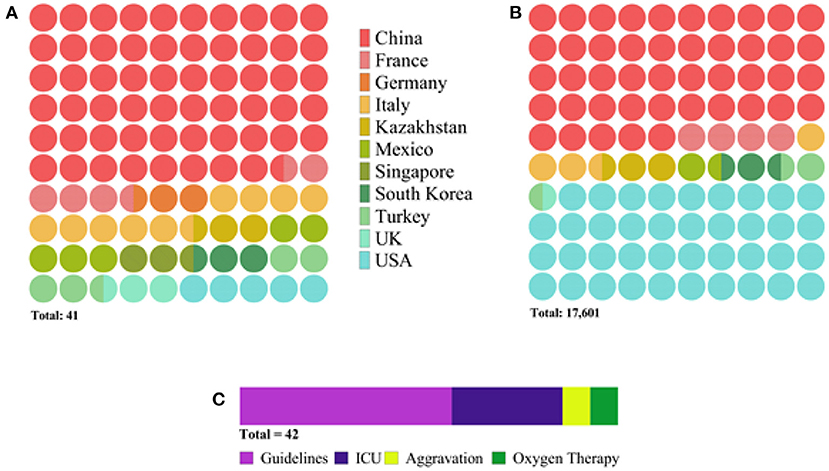
Figure 2. Diagram depicting characteristics of selected studies: (A) number of publications per country, (B) number of patients per country, and (C) number of publications per severity assessment criterion. The latter (C) indicates the total number of research articles to be 42 instead of 41 because one study reported two populations of patients admitted to the hospital at different periods.
Results of Meta-Analysis
Pooled Effect Sizes
We collected data for five coagulation parameters: PLT, DD, FIB, APTT, and PT. In all cases, the heterogeneity was extremely high (I2 > 80%), so the Knapp-Hartung adjusted random-effects model was applied in all parts of the meta-analysis. DD, FIB, APTT, and PT were significantly higher in more severe cases [SMD 0.6985 with 95% confidence interval (CI) (0.5155; 0.8815); SMD 0.661 with 95% CI (0.3387; 0.9833); SMD 0.2683 with 95% CI (0.1357; 0.4009); SMD 0.284 with 95% CI (0.1472; 0.4208)]. In contrast, PLT was significantly lower in patients with severe cases of COVID-19 [SMD −0.1684 with 95% CI (−0.2826; −0.0542)]. Forest plots in Figures 3, 4 summarize the results of meta-analyses. Point estimates are represented by red squares, and confidence intervals are shown by corresponding horizontal bars. The sizes of red squares are proportional to weights of respective studies, which are adjusted random-effects weights: reciprocal of the sum of squares of study's standard error and the variance of the distribution of true effect sizes. Pooled effect size and its confidence intervals are shown by blue diamond at the bottom. Detailed output of the meta-analysis is given in Table 1.
Sensitivity and Subgroup Analysis
Neither the analysis by the leave-one-out method nor the influence diagnostic has identified studies that solely cause a significant change in the effect size estimates. Removal of several outliers based on the pooled and individual confidence intervals allowed significant decrease in heterogeneity, yet it did not affect the interpretation of the results. Adjusted effect sizes and heterogeneity measurements are summarized in Table 2. All influence diagnostic plots, such as Baujat and leave-one-out plots, Cook's distance, Covariance Ratio, etc., are provided in the Supplementary Figures 1–16 along with resulting Forest plots.
GOSH plots were built for all five models. K-means, DBSCAN, and Gaussian Mixture Model were implemented as clustering methods. This diagnostic was not applied on PLT and DD since they only have one cluster each (Figure 5); for FIB, APTT, and PT, on the other hand, we constructed the plots and identified several possible influential cases. Original GOSH plots for these parameters are given at Supplementary Figures. For fibrinogen, models that include studies by Fu et al. (35) and Wang et al. (12) tend to show higher heterogeneity and greater effect size (Figures 6A,B). For activated partial thromboplastin time, the GOSH plot demonstrated that studies by Zhao et al. (36), Zhang et al. (37), and Yu et al. (38) increase the model's heterogeneity and slightly pull the effect size to the left (Figures 6C–E). GOSH plots of the prothrombin time effect size identified four studies, three of which (36, 39, 40) pull effect size to the left (Figures 6F–H), and one (41) pulls effect size to the right (Figure 6I). We have excluded these studies and recalculated the pooled effect size for each parameter. Results are summarized in Table 2.
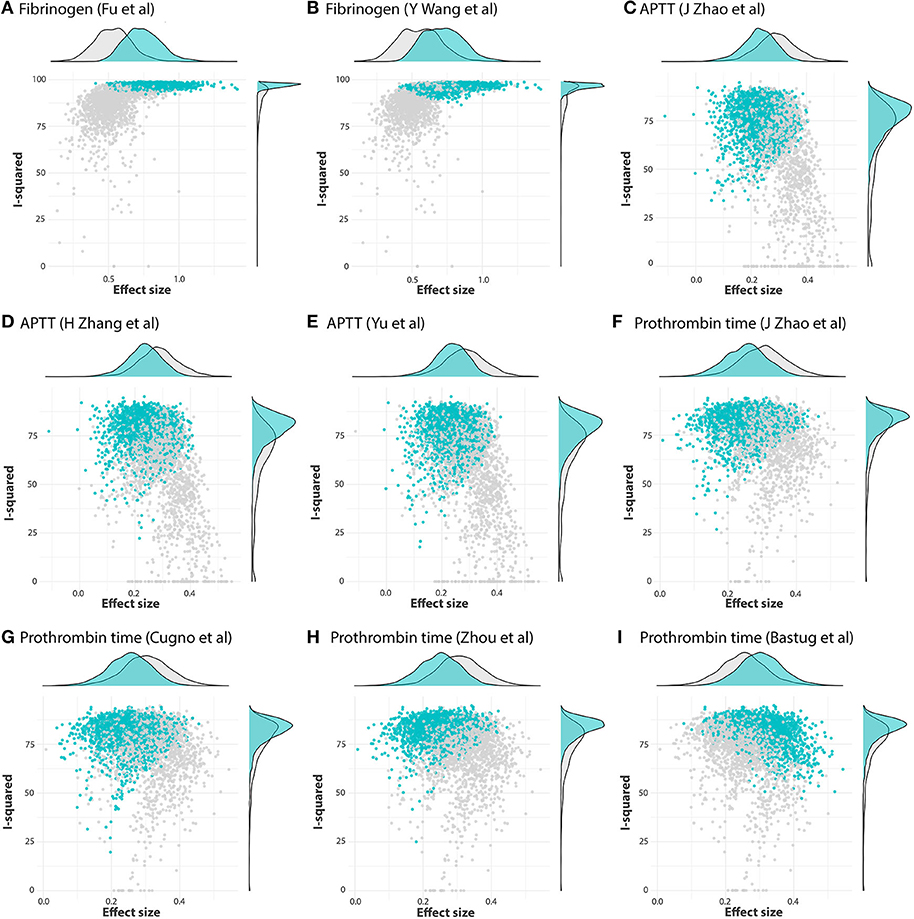
Figure 6. GOSH plots fixed for influential studies in meta-analysis models. (A,B) FIB, (C–E) APTT, and (F-I) PT.
To explore the possible sources of heterogeneity and test the robustness of our data, we have conducted subgroup analyses based on articles' country of origin and criteria for severity stratification. Results are summarized in Table 3. Subgroup analysis showed no significant difference between articles originated from different countries but revealed that severity assessment criteria might have an influence on overestimated effect sizes for PLT and DD. High heterogeneity levels seem to be persistent oven different subgroups. Being the only exception, values of APTT obtained from non-Chinese articles were less heterogenous–47.1% compared to 84.7% in studies originated in China.
Publication Bias
Contour-enhanced funnel plots for five coagulation parameters are depicted in Figure 7, and the results of Egger's test are summarized in Table 4. Based on the linear regression results, significant asymmetry is observed in published studies that included results for PLT, DD [Intercept (p-value): −1.297 (0.0336), 3.182 (0.0013)], and somewhat significant asymmetry was indicated for FIB [1.872 (0.0493)]. While it is impossible to predict whether asymmetry is coming solely from publication bias, contour-enhanced funnel plots for DD and FIB (Figures 6B,C) show that the number of papers with insignificant findings is very small (p > 0.1). This may advocate for the presence of some publication bias. In contrast, Figure 6A illustrates that even though the funnel plot for PLT has some asymmetry, there are plenty of publications with insignificant SMDs, thus decreasing the possibility of publication bias.
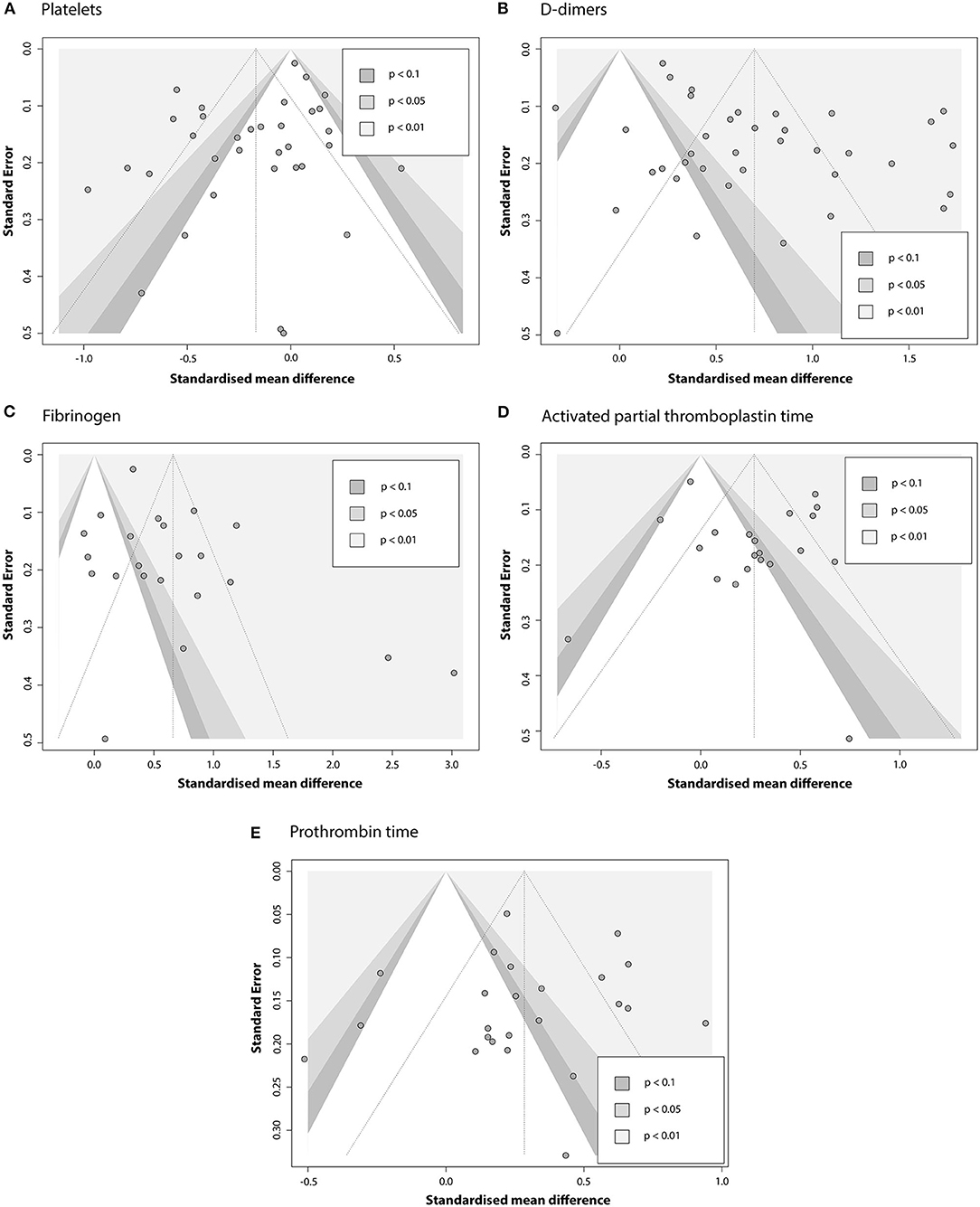
Figure 7. Contour-enhanced funnel plots evaluating the presence of publication bias in the pool of articles that report association between COVID-19 severity and (A) PLT; (B) DD; (C) FIB; (D) APTT; (E) PT.
To further explore the robustness of our findings, we have conducted several additional tests. As reported by Zwetsloot et al. (42), plotting SMD against its standard error to assess the publication bias can result in distorted funnel plots, leading to false-positive conclusions. Since SMD is necessary for our analysis and cannot be substituted, we used a sample size-based precision estimate instead of the standard error–1/√n. The resulting funnel plots (Supplementary Figure 16) showed no significant distortion.
Similar corrections were made to Egger's test: since standardized mean difference and standard error are not independent, we can expect the test to yield some false-positive results. In our calculations, we used the corrected SE suggested by Pustejovsky and Rodgers (43). Results are given in the Supplementary Table 3. The only model that was affected by this correction was FIB (intercept 2.142 with a p-value of 0.0526 compared to the initial 1.872 and 0.0493), indicating that previously concluded asymmetry might have been a false-positive. Interpretation of results for other parameters remained the same.
Discussion
There is a need to identify early indicators of the risk of critical patients progressing with COVID-19 to ICU. Abnormal coagulation parameters, including DD and FIB levels, and changes in PLT and PT, are observed in many patients with COVID-19 infection at admission [rev. (8, 13)]. However, as discussed by different authors, these indicators provide contradictory information regarding risk stratification and prognosis (44–46). Several previous meta-analysis reviews are researching abnormal coagulation and the severity of COVID-19. The majority of analyzed studies were conducted at the beginning of the pandemic and examined the Chinese population of patients [before April 2020—(14, 47–49); June 2020—(50)]. Our meta-analysis study found that DD and FIB levels, APTT, and prothrombin time were significantly higher in the severe group of COVID-19 patients. In contrast, PLT was significantly lower in these patients. There was no evidence of effects across different geographic subgroups, except for APTT values.
Platelet Changes
In this meta-analysis, we systematically analyze evidence for the utility of lowered PLT levels on admission in relation to the severity of infection. Platelets play a crucial role in the maintenance of hemostasis, and contribute to thromboinflammatory processes (51). At different stages of viral infection, changes in platelet production or destruction may result in coagulation imbalances leading to pro-thrombotic events or in platelet dysfunction and bleeding risks (52) and increased activation of platelets (19). Thrombocytopenia (platelet count <150 × 109/L) seems to represent an important marker of COVID-19 severity (8, 14, 18, 53) and mortality outcome (14, 18). The reasons for thrombocytopenia are multi-factorial may include early suppression of platelets progenitors' production and damage in bone marrow and lungs, hemophagocytosis and other reasons. Significant thrombocytopenia in COVID-19 is less frequent than it was reported for the largest SARS-1 cohort (45%) (54). SARS-1 induced platelet depletion was related to direct viral infection of megakaryocytes and hematopoietic progenitors [rev. by (4)]. However, almost half of patients developed thrombocytosis following initial thrombocytopenia during the SARS epidemics in 2003 (55). It is possible that opposite PLT changes may reflect different phases of COVID-19 infection (56)—acute and convalescent phases, though the explanation has not been established yet. The reactive thrombocytosis that may occur during the convalescent phase requires time observation, including a period preceding patient discharge. The increase of immature reticulated platelets reported recently in COVID-19 patients by different groups (57, 58) may play a role in the limiting effects of anti-platelet therapies (59–61). Change in platelets parameters (increased mean volume) (62) and reactivity (63, 64) are suggested to be associated with a severe COVID-19 infection (65). In addition to platelet changes, there are an increasing number of studies related to the dysfunction of megakaryocytes (platelets progenitors) in COVID-19 patients. Thus, elevated and abnormal megakaryocytes (MKs) were found in post-mortem and autopsy studies in the lungs (66–69), heart (68), brain (70, 71), bone marrow (72, 73). Recently, the concept that megakaryopoiesis occurs in the lung parenchyma with significant contribution of the lungs in platelet biogenesis has emerged (74–76). There is a close association between platelet biogenesis and alveolarization of the lung (77, 78), and hypoxia-induced thrombocytopenia associated with reduction of lung megakaryocytes and impaired generation of platelets in the lungs (79). The SARS-CoV-2 virus elicited up-regulation of megakaryocyte progenitors and elevated circulating megakaryocytes in blood in severe COVID-19 (80, 81), hypothetically through infection of early megakaryocyte progenitors. Recently, Fortmann et al. (80) detected infected calprotectin+ spike+ megakaryocytes in the blood of patients with severe COVID-19. We hypothesize that viral RNA next may be transferred from megakaryocytes to platelets and circulating plasma EVs. The strong supporting argument is a recent finding of Brunet-Ratnasingam et al. (82) that plasma SARS-CoV-2 RNA is strongly associated with increased mortality. There is a current gap of knowledge regarding platelet non-canonical function and regeneration, and further research is crucial for our understanding of COVID-19 pathogenesis and stratification of increased thromboembolic risk.
DD and FIB Levels and Thromboembolic Complications
Concordant with other studies, we found DD and FIB levels significantly elevated in severe COVID-19 infection. However, DD levels results may suffer from some publication bias as revealed by meta-regression analysis. It may be related to the variability of cut-offs for varying DD measurement assays used in the different studies [(83); rev. (84)]. The usefulness of hemostasis tests, particularly, D-dimers, is limited by interlaboratory variability reflecting differences in methodologies, antibody sources and specificity, different detection methods, calibrators, and diagnostic thresholds (85, 86). As confirmed by autopsy results, the elevated DD levels can be associated with fibrin deposits within pulmonary extravascular space and alveoli (67), but they may be non-specific to intravascular fibrin formation (87). High DD levels, together with elevated neutrophils, were reported to be predictive factors of pulmonary embolism in COVID-19 patients (88). DD and FIB levels are elevated in both COVID-19 and thromboembolism, and therefore as single tests are unspecific and unhelpful in the differentiation of these conditions. In part, it may be explained by the involvement in abnormal coagulation in COVID-19 of different systems, including endothelial cells, complement activation, and hypofibrinolysis resulting in changes undetected by routine tests (39, 88).
A significantly higher amount of neutrophil extracellular traps [and related markers such as cell-free DNA and myeloperoxidase-DNA (MPO-DNA)] was found when compared with non-COVID-19 thrombi (89). Neutrophil extracellular traps (NETs) markers, and cell-free DNA was most closely aligned with D-dimers, with inflammatory markers (C-reactive protein, lactate dehydrogenase) and more specific markers of NETs, MPO-DNA (90–92). The presence and high concentrations of NETs at COVID-19 patients' tracheal aspirates, blood samples (93, 94), and different organs (73) are associated with COVID-19 severity. The formation of NETs in the lungs and bloodstream can be critically associated with thrombosis (95). Platelet activation and endothelial cell damage (96, 97) in COVID-19 patients are also resulting in elevated levels of extracellular vesicles (EVs) (98–100) expressing tissue factor (101, 102) and associated with severity of disease (99, 101, 103).
PT, APTT, FIB, and Clot Formation Parameters
PT has been included in the International Society on Thrombosis and Hemostasis (ISTH) criteria for diagnosing disseminated intravascular coagulation (DIC) (104), prolonged at COVID-19 with acute distress respiratory syndrome (ARDS) (10) and is significantly elevated in severe COVID-19 patients (105). Progressive prolongation of PT is considered a predictor of fatal outcome.
The first reports from China described a shortened APTT which is considered to be a predictive marker of hypercoagulation. Later studies reported prolonged APTT (45, 106). A prolongation of APTT may indicate a deficiency of clotting factors (II, V, VIII, IX, X, XI, or XII) or the presence of inhibitor (heparin therapy). Another likely explanation is the presence of antiphospholipid antibodies (aPL) or lupus anticoagulant (LA) observed in 45% of COVID-19 patients in the Mulhouse series (107). In Bowles's study (106), most of the patients with prolonged APTT were positive for LA (91%). However, increasing of heparin therapy usage, especially in severe COVID-19 cases, may also lead to prolonged APTT.
Discordant reports exist concerning the fibrinogen levels and severity of COVID-19 disease. There are reports that COVID-19 is associated with high fibrinogen levels (16, 108) and alternative findings (109). Some authors reported that severe COVID-19 infection induces fibrinolysis shutdown (26, 45), which authors consider partly responsible for high DD and FIB levels. However, there are also reports of an increase in plasmin-antiplasmin complexes in severe COVID-19 and findings of mild consumption coagulopathy seen in a proportion of COVID-19 patients (110). Elevated tissue-type plasminogen activator was also found to be increased in the patients with severe COVID-19 (101).
Fibrinogen is a critical molecule that links coagulation, complement system, and inflammation, and at COVID-19, a significant association between FIB levels and interleukin-6 was described (16). Recent post-mortem studies of COVID-19 patients identified microvascular thrombi in multiple organs, including kidney (67), which may lead to increased DD even in the situation of reduced fibrinolysis. Depression of fibrinolysis was reported not only in ICU patients with severe infection and clinical signs of thromboembolism (26) but also in the general unselected ICU cohort (108, 109). Viscoelastic testing ([ROTEM, tissue-type plasminogen activator (tPA) ROTEM, TEG] and evaluation of clot formation by Clot Waveworm analysis have demonstrated a hypercoagulable state, characterized by increased clot stiffness and severely impaired fibrinolysis (17, 108, 111, 112). However, no consistent association between abnormal VET (viscoelastometric testing) pattern at COVID-19 and clinical outcome have been demonstrated [rev. (113)].
Elevated routine coagulation parameters taken at admission may help with the initial differentiation of severe and non-severe COVID-19 patient groups. The hypothetical mechanisms of fibrinolysis shutdown implicate lowering of fibrinolysis factors (plasminogen) and elevated amounts of inhibitors of fibrinolysis (such as α2-antiplasmin and plasminogen activator inhibitor PAI-1).
Longitudinal observational studies of COVID-19 infection are particularly important for understanding infection dynamics and disease outcomes (114–119). Hardy et al. (117) reported daily changes of relevant parameters of hemostasis of severe COVID-19 patients, including standard hemostatic tests (D-dimers, PT) as well as functional integrative tests for thrombin generation and fibrinolysis. Importantly, the day of hospital and/or ICU admission does not represent the same timepoint in the COVID-19 disease course that increases variability and complicates interpretation of studies results.
Although our findings were consistent across different geographic groups, they were tempered by significant heterogeneity. The DD, FIB, and PLT elevation trends speak about the necessity of investigating the potential of additional specific biomarkers for the stratification of COVID-19 patients. We suggest that thorough characterization of platelets and their progenitors (megakaryocytes), as well as clot formation testing could aid in the assessment of risk forthromboembolic events in COVID-19 patient groups.
Limitations of the Study
Our findings should be interpreted with some limitations. Firstly, most of the included studies were either retrospective and observational by design, thus prone to recall or misclassification bias. Second, such studies often imply recruitment by convenience sampling, in which case the representativeness of a study is questioned. In the context of this meta-analysis, several different endpoints were allowed: severity guidelines, ICU admissions, oxygen therapy requirement, and disease aggravation. In an attempt to overcome this issue, we have conducted a subgroup analysis. Third, uncontrolled variables might also pose a limitation–both the disease severity and coagulation parameters can be affected by several potential confounding variables, such as comorbidities, age, etc. Fourth, data transformation was applied to those studies that reported their results in quartiles or maximum and minimum values. This could potentially introduce some degree of error in the pooled estimate. Fifth, the random-effects model was used due to the high heterogeneity of research. Although sensitivity and subgroup analyses were conducted, some residual heterogeneity may affect the interpretation of results. Finally, the underlying mechanisms of coagulation disbalances at COVID-19 still need to be investigated.
Conclusions
Our findings support using a combination of coagulation parameters for risk stratification of patients with COVID-19 infection at the time of admission, in particular DD and FB levels. However, additional evaluation using clotting formation methods or combination with specific biomarkers of production and activation of platelet and platelet progenitors is required. This information may help physicians triage patients with thromboembolism. An important consideration is an intermediate and long follow-up of COVID-19 patients.
Data Availability Statement
The raw data supporting the conclusions of this article will be made available by the authors, without undue reservation.
Ethics Statement
The studies involving human participants were reviewed and approved by Ethics Committee of the National Research Center for Cardiac Surgery (NCJSC), Nur-Sultan, Kazakhstan. Written informed consent for participation was not required for this study in accordance with the national legislation and the institutional requirements.
Author Contributions
PL: conceptualization, methodology, formal analysis, investigation, data curation, and writing original draft. GI, ZS, and AK: methodology, data curation, formal analysis, and investigation. ATT, MS, AD, MA, and MB: methodology, formal analysis, investigation, data curation of NRCSC cohort, and writing a draft. EP and AT: methodology, analysis, and funding. NB: conceptualization, methodology, formal analysis, investigation, data curation, finding, and writing a draft. MB and NB: supervision. All authors read and edit a final draft. All authors contributed to the article and approved the submitted version.
Funding
NB and AT were supported by FDCRGP SSH2020028 and NB was funded by OPCRP2020018 grants from Nazarbayev University.
Conflict of Interest
The authors declare that the research was conducted in the absence of any commercial or financial relationships that could be construed as a potential conflict of interest.
Publisher's Note
All claims expressed in this article are solely those of the authors and do not necessarily represent those of their affiliated organizations, or those of the publisher, the editors and the reviewers. Any product that may be evaluated in this article, or claim that may be made by its manufacturer, is not guaranteed or endorsed by the publisher.
Acknowledgments
We are very grateful to Sadyk Khamitov and Aliya Sailybayeva from the Research Department of NCJSC National Research Center for Cardiac Surgery for their excellent organizational help and to Alexander Kovaldgi from 2nd Mathematical Liceum for helpful discussion.
Supplementary Material
The Supplementary Material for this article can be found online at: https://www.frontiersin.org/articles/10.3389/fcvm.2022.794092/full#supplementary-material
Supplementary Figure 1. Exploring influential cases in meta-analysis models with Baujat plots: (A) Platelets, (B) D-dimers, (C) Fibrinogen, (D) Activated partial thromboplastin time, (E) Prothrombin time.
Supplementary Figure 2. Influence diagnostic of meta-analysis model for the association of platelet count and COVID-19 severity.
Supplementary Figure 3. Influence diagnostic of meta-analysis model for the association of D-dimers concentration and COVID-19 severity.
Supplementary Figure 4. Influence diagnostic of meta-analysis model for the association of fibrinogen and COVID-19 severity.
Supplementary Figure 5. Influence diagnostic of meta-analysis model for the association of activated partial thromboplastin time and COVID-19 severity.
Supplementary Figure 6. Influence diagnostic of meta-analysis model for the association of prothrombin time and COVID-19 severity.
Supplementary Figure 7. Search for influential cases by leave-one-out method in meta-analysis models for the association of (A) platelets count, (B) D-dimers and COVID-19 severity.
Supplementary Figure 8. Search for influential cases by leave-one-out method in meta-analysis models for the association of (A) fibrinogen, (B) APTT and COVID-19 severity.
Supplementary Figure 9. Search for influential cases by leave-one-out method in meta-analysis model for the association of prothrombin time and COVID-19 severity.
Supplementary Figure 10. Forest plots of association of COVID-19 severity and (A) platelet counts and (B) D-dimers with outliers removed.
Supplementary Figure 11. Forest plots of association of COVID-19 severity and (A) fibrinogen, (B) activated partial thromboplastin time, and (C) prothrombin time with outliers removed.
Supplementary Figure 12. Exploring the influence of meta-analysis study composition on heterogeneity and pooled effect size: (A) fibrinogen, (B) activated partial thromboplastin time, and (C) prothrombin time.
Supplementary Figure 13. Results of three cauterization methods (A) K-means, (B) DBSCAN, and (C) Gaussian Mixture on the meta-analysis model for association of fibrinogen and COVID-19 severity.
Supplementary Figure 14. Results of three cauterization methods (A) K-means, (B) DBSCAN, and (C) Gaussian Mixture on the meta-analysis model for association of activated partial thromboplastin time and COVID-19 severity.
Supplementary Figure 15. Results of three cauterization methods (A) K-means, (B) DBSCAN, and (C) Gaussian Mixture on the meta-analysis model for association of prothrombin time and COVID-19 severity.
Supplementary Figure 16. Exploring presence of publication bias with corrected funnel plots: SMD plotted against sample size-based precision estimate: (A) platelet counts, (B) D-dimers, (C) fibrinogen, (D) activated partial thromboplastin time, (E) prothrombin time.
Supplementary Table 1. Results of the NIH Quality Assessment.
Supplementary Table 2. Composition of two groups stratified by severity.
Supplementary Table 3. Results of the Egger's test with Pustejovsky's corrected standard error.
Supplementary Data 1. Criteria for clinical severity of COVID-19 used by the National Research Center for Cardiac Surgery.
Abbreviations
APTT, activated partial thromboplastin time; CI, confidence interval; DD, D-dimer; DIC, disseminated intravascular coagulation; EVs, extracellular vesicles; FIB, fibrinogen; GOSH, graphical display of study heterogeneity; ICU, intensive care unit; MERS-CoV, Middle East respiratory syndrome coronavirus (MERS-CoV); MPO-DNA, myeloperoxidase-DNA; NRCSC, National Research Center for Cardiac Surgery; PAI-1, plasminogen activator inhibitor; PM, Paule-Mandel tau-squared estimator; PLT, platelets count; PT, prothrombin time; QP, Q-profile; ROTEM, rotation thromboelastometry; SARS-CoV, severe respiratory acute syndrome coronavirus (SARS-CoV); SMD, standardized mean difference; TEG, thromboelastography; tPA, tissue-type plasminogen activator; VET, viscoelastometric testing; VTE, venous thromboembolism; VWF, von Willebrand factor.
References
1. Johns Hopkins University Medicine. The Johns Hopkins Coronavirus Resource Center (CRC). Johns Hopkins University and Medicine (2021). Available online at: https://coronavirus.jhu.edu/ (accessed September 30, 2021).
2. Carsana L, Sonzogni A, Nasr A, Rossi RS, Pellegrinelli A, Zerbi P, et al. Pulmonary post-mortem findings in a series of COVID-19 cases from northern Italy: a two-centre descriptive study. Lancet Infect Dis. (2020) 20:1135–40. doi: 10.1016/S1473-3099(20)30434-5
3. Hwang DM, Chamberlain DW, Poutanen SM, Low DE, Asa SL, Butany J. Pulmonary pathology of severe acute respiratory syndrome in Toronto. Modern Pathol. (2005) 18:1–10. doi: 10.1038/modpathol.3800247
4. Yang M, Ng MH, Li CK. Thrombocytopenia in patients with severe acute respiratory syndrome. Hematology. (2005) 10:101–5. doi: 10.1080/10245330400026170
5. Goeijenbier M, Van Wissen M, Van De Weg C, Jong E, Gerdes VEA, Meijers JCM, et al. Review: viral infections and mechanisms of thrombosis and bleeding. J Med Virol. (2012) 84:1680–96. doi: 10.1002/jmv.23354
6. World Health Organization. Guidelines for Investigation of Cases of Human Infection With Middle East Respiratory Syndrome Coronavirus (MERS-CoV). WHO (2013). Available online at: https://cdn.who.int/media/docs/default-source/documents/emergencies/health-topics—mers/mers_cov_investigation_guideline_jul132d7b61a0-a593-4527-8246-25152b965baf.pdf?sfvrsn=3e2f6c65_1&download=true (accessed September 30, 2021).
7. Giannis D, Ziogas IA, Gianni P. Coagulation disorders in coronavirus infected patients: COVID-19, SARS-CoV-1, MERS-CoV and lessons from the past. J Clin Virol. (2020) 127:104362. doi: 10.1016/j.jcv.2020.104362
8. Iba T, Levy JH, Levi M, Connors JM, Thachil J. Coagulopathy of coronavirus disease-2019. Crit Care Med. (2020) 48:1358–64. doi: 10.1097/CCM.0000000000004458
9. Chen N, Li Y, Fan H, Tian A, Yuan H, Jiang Z, et al. Analysis of dynamic disturbance in blood coagulation function of patients with coronavirus disease 2019: a retrospective observational study. Medicine. (2020) 99:e22635. doi: 10.1097/MD.0000000000022635
10. Chen N, Zhou M, Dong X, Qu J, Gong F, Han Y, et al. Epidemiological and clinical characteristics of 99 cases of 2019 novel coronavirus pneumonia in Wuhan, China: a descriptive study. Lancet. (2020) 395:507–13. doi: 10.1016/S0140-6736(20)30211-7
11. Zhou C, Huang Z, Tan W, Li X, Yin W, Xiao Y, et al. Predictive factors of severe coronavirus disease-2019 in previously healthy young adults: a single-center, retrospective study. Respir Res. (2020) 21:157. doi: 10.1186/s12931-020-01412-1
12. Wang Y, Yao L, Zhang JP, Tang PJ, Ye ZJ, Shen XH, et al. Clinical characteristics and laboratory indicator analysis of 67 COVID-19 pneumonia patients in Suzhou, China. BMC Infect Dis. (2020) 20:747. doi: 10.1186/s12879-020-05468-8
13. Levi M, Thachil J, Iba T, Levi JH. Coagulation abnormalities and thrombosis in patients with COVID-19. Lancet Haematol. (2020) 7:e438–40. doi: 10.1016/S2352-3026(20)30145-9
14. Lippi G, Plebani M, Henry BM. Thrombocytopenia is associated with severe coronavirus disease 2019 (COVID-19) infections: a meta-analysis. Clin Chim Acta. (2020) 506:145–8. doi: 10.1016/j.cca.2020.03.022
15. Demelo-Rodriguez P, Cervilla-Munoz E, Ordieres-Ortega L, Parra-Virto A, Toledano-Macias M, et al. Influence of asymptomatic deep vein thrombosis in patients with COVID-19 pneumonia and elevated D-dimers levels. Thrombosis Res. (2020) 192:23–6. doi: 10.1016/j.thromres.2020.05.018
16. Ranucci M, Ballotta A, Di Dedda U, Bayshnikova E, Dei Poli M, Resta M, et al. The procoagulant pattern of patients with COVID-19 acute distress syndrome. J Thromb Haemost. (2020) 18:1747–51. doi: 10.1111/jth.14854
17. Panigada M, Bottino N, Tagliabue P, Grasselli G, Novembrino C, Chantarangkul V, et al. Hypercoagulability of COVID-19 patients in intensive care unit: a report of thromboelastograpgy and other parameters of hemostasis. J Thromb Haemost. (2020) 18:1738–42. doi: 10.1111/jth.14850
18. Yang X, Yang Q, Wang Y, Wu Y, Xu J, Yu Y, et al. Thrombocytopenia and its association with mortality in patients with COVID-19. J Thromb Haemost. (2020) 18:1469–72. doi: 10.1111/jth.14848
19. Althaus K, Marini I, Zlamal J, Pelzl L, Singh A, Häberle H, et al. Antibody-induced procoagulant platelets in severe COVID-19 infection. Blood. (2021) 137:1061–71. doi: 10.1182/blood.2020008762
20. Sjöström A, Wersäll JD, Warnquist A, Farm M, Magnusson M, Oldner A, et al. Platelet count rose while D-dimer levels dropped as deaths and thrombosis declined – an observational study on anticoagulation shift in COVID-19. Thromb Haemost. (2021) 121:1610–21. doi: 10.1055/a-1477-3829
21. Goshua G, Pine AB, Meizlish ML, Chang C.-H., Zhang H, et al. Endotheliopathy in COVID-19-associated coagulopathy: evidence from a single-centre, cross-sectional study. Lancet Haematol. (2020) 7:e575–82. doi: 10.1016/S2352-3026(20)30216-7
22. Doevelaar AA, Bachmann M, Hölzer B, Seibert FS, Rohn BJ, Bauer F, et al. Von Willebrand factor multimer formation contributes to immunothrombosis in Coronavirus disease 2019. Crit Care Med. (2021) 49:e512–20. doi: 10.1097/CCM.0000000000004918
23. Becker RC, Sexton C, Smyth S. COVID-19 and biomarkers of thrombosis: focus on von Willebrand factor and extracellular vesicles. J Thromb Thrombolysis. (2021) 52:1010–9. doi: 10.1007/s11239-021-02544-x
24. Creel-Bulos C, Auld SC, Caridi-Scheible M, Barker NA, Friend S, Gaddh M, et al. Fibrinolysis shutdown and a thrombosis in a COVID-19 ICU. Shock. (2021) 55:316–20. doi: 10.1097/SHK.0000000000001635
25. Bachler M, Bösch J, Stürzel DP, Hell T, Giebl A, Ströhle M, et al. Impaired fibrinolysis in critically ill COVID-19 patients. Br J Anaesthesia. (2021) 126:590–8. doi: 10.1016/j.bja.2020.12.010
26. Wright FL, Vogler TO, Moore EE, Moore HB, Wohlauer MV, Urban S, et al. Fibrinolysis shutdown correlation with thromboembolic events in severe COVID-19 infection. J Am Col Surg. (2020) 231:193–203. doi: 10.1016/j.jamcollsurg.2020.05.007
27. Klok FA, Kruip MJHA, Van der Meer NJM, Arbous MC, Gommers AMPJ, Kant KM, et al. Incidence of thrombotic complications in critically ill ICU patients with COVID-19. Thrombosis Res. (2020) 191:145–7. doi: 10.1016/j.thromres.2020.04.013
28. Thomas W, Varley J, Johnston A, Symington E, Robinson M, Sheares K, et al. Thrombotic complications of patients admitted to intensive care with COVID-19 at a teaching hospital in the United Kingdom. Thromb Res. (2020) 191:76–7. doi: 10.1016/j.thromres.2020.04.028
29. Lodigiani C, Iapichino G, Carenzo L, Cecconi M, Ferrazzi P, Sebastian T, et al. Venous and arterial thromboembolic complications in COVID-19 patients admitted to an academic hospital in Milan, Italy. Thromb Res. (2020) 191:9–14. doi: 10.1016/j.thromres.2020.04.024
30. National Institute of Health. Study Quality Assessment Tools: National Heart, Lung, and Blood Institute (NHLBI) (2021). Available online at: https://www.nhlbi.nih.gov/health-topics/study-quality-assessment-tools (accessed October 4, 2021).
31. McGrath S, Zhao X, Steele R, Thombs BD, Benedetti A, DEPRESsion Screening Data Collaboration. Estimating the sample mean and standard deviation from commonly reported quantiles in meta-analysis. Stat Meth Med Res. (2020) 29:2520–37. doi: 10.1177/0962280219889080
32. Luo D, Wan X, Liu J, Tong T. Optimally estimating the sample mean from the sample size, median, mid-range, and/or mid-quartile range. Stat. Meth. Med. Res. (2018) 27:1785–1805. doi: 10.1177/0962280216669183
33. Wan X, Wang W, Liu J, Tong T. Estimating the sample mean and standard deviation from the sample size, median, range and/or interquartile range. BMC Med Res Methodol. (2014) 14:135. doi: 10.1186/1471-2288-14-135
34. Veroniki AA, Jackson D, Viechtbauer W, Bender R, Bowden J, Knapp G, et al. Methods to estimate the between-study variance and its uncertainty in meta-analysis. Res Synth Methods. (2016) 7:55–79. doi: 10.1002/jrsm.1164
35. Fu J, Kong J, Wang W, Wu M, Yao L, Wang Z, et al. The clinical implication of dynamic neutrophil to lymphocyte ratio and D-dimer in COVID-19: a retrospective study in Suzhou China. Thromb Res. (2020) 192:3–8. doi: 10.1016/j.thromres.2020.05.006
36. Zhao J, Zhu M, Su X, Huang M, Yang Y, Huang J, et al. Clinical features and risk factors for severe-critically ill COVID-19 adult patients in Jiangsu, China. Medicine. (2021) 100:e24332. doi: 10.1097/MD.0000000000024332
37. Zhang H, Wang X, Fu Z, Luo M, Zhang Z, Zhang K, et al. Potential factors for prediction of disease severity of COVID-19 patients. medRxiv [Preprint]. (2020). doi: 10.1101/2020.03.20.20039818
38. Yu C, Lei Q, Li W, Wang X, Li W, Liu W. Epidemiological and clinical characteristics of 1663 hospitalized patients infected with COVID-19 in Wuhan, China: a single-center experience. J Infect Public Health. (2020) 13:1202–9. doi: 10.1016/j.jiph.2020.07.002
39. Cugno M, Meroni PL, Gualtierotti R, Griffini S, Grovetti E, Torri A, et al. Complement activation and endothelial perturbation parallel COVID-19 severity and activity. J Autoimmun. (2021) 116:102560. doi: 10.1016/j.jaut.2020.102560
40. Zhao Y, Yu C, Ni W, Shen H, Qiu M, Zhao Y. Peripheral blood inflammatory markers in predicting prognosis in patients with COVID-19. Some differences with influenza A. J Clin Lab Anal. (2021) 35:e23657. doi: 10.1002/jcla.23657
41. Bastug A, Bodur H, Erdogan S, Gokcinar D, Kazancioglu S, Deniz B, et al. Clinical and laboratory features of COVID-19: predictors of severe prognosis. Int Immunopharmacol. (2020) 88:106950. doi: 10.1016/j.intimp.2020.106950
42. Zwetsloot P.-P., Naald MVD, Sena ES, Howells WD, IntHout J, et al. Standardized means differences cause funnel plots distortion in publication bias assessments. eLife. (2017) 6:e24260. doi: 10.7554/eLife.24260
43. Pustejovsky MA, Rodgers JE. Testing for funnel plot asymmetry of standardized mean differences. Res Synthesis Meth. (2019) 10:57–71. doi: 10.1002/jrsm.1332
44. Luo L, Xu M, Du M, Kou H, Liao D, Cheng Z, et al. Early coagulation tests predict risk stratification and prognosis of COVID-19. Aging. (2020) 12:15918–937. doi: 10.18632/aging.103581
45. Tang N, Li D, Wang X, Sun Z. Abnormal coagulation parameters are associated with poor prognosis in patients with novel coronavirus pneumonia. J Thromb Haemost. (2020) 18:844–7. doi: 10.1111/jth.14768
46. Ding R, Yang Z, Huang D, Wang Y, Li X, Zhou X, et al. Identification of parameters in routine blood and coagulation tests related to the severity of COVID-19. Int J Med Sci. (2021) 18:1207–15. doi: 10.7150/ijms.47494
47. Xiong M, Liang X, Wei YD. Changes in blood coagulation in patients with severe coronavirus disease 2019 (COVID-2019): a meta-analysis. Br J Haematol. (2020) 189:1050–2. doi: 10.1111/bjh.16725
48. Zhang A, Leng Y, Zhang Y, Wu K, Ji Y, Lei S, et al. Meta-analysis of coagulation parameters associated with disease severity and poor prognosis of COVID-19. Inter J Infect Dis. (2020) 100:441–8. doi: 10.1016/j.ijid.2020.09.021
49. Zhu J, Pang J, Ji P, Zhong Z, Li H, Li B, et al. Coagulation dysfunction is associated with a severity of COVID-19. J Med Virol. (2021) 93:962–72. doi: 10.1002/jmv.26336
50. Di Minno MND, Calcaterra I, Lupoli R, Storino A, Spedicato GA, Maniscalco M, et al. Hemostatic changes in patients with COVID-19: a meta-analysis with meta-regressions. J Clin Med. (2020) 9:2244. doi: 10.3390/jcm9072244
51. Van der Meijden PEJ, Heemskerk JWM. Platelet biology and functions: new concepts and clinical perspectives. Nat Rev Cardiol. (2019) 16:166–79. doi: 10.1038/s41569-018-0110-0
52. Assinger A. Platelets and infection-an emerging role of platelets in viral infection. Front Immunol. (2014) 5:649. doi: 10.3389/fimmu.2014.00649
53. Qu R, Ling Y, Zhang YHZ, Wei LY, Chen X, Li XM, et al. Platelet-to lymphocyte ratio is associated with prognosis in patients with coronavirus disease-19. J Med Virol. (2020) 92:1533–41. doi: 10.1002/jmv.25767
54. Lee N, Hui D, Wu A, Chan P, Cameron P, Joynt GM, et al. A major outbreak of severe acute respiratory syndrome in Hong Kong. N Engl J Med. (2003) 348:1986–94. doi: 10.1056/NEJMoa030685
55. Wong RS, Wu A, To KF, Lee N, Lam CW, Wong CK, et al. Haematological manifestations in patients with severe acute respiratory syndrome: retrospective analysis. Br Med J. (2003) 326:1358–62. doi: 10.1136/bmj.326.7403.1358
56. Thachil J, Tang N, Gando S, Falanga A, Cattaneo M, Levi M, et al. ISTH interim guidance on recognition and management of coagulopathy in COVID-19. J Thromb Haemost. (2020) 18:1023–6. doi: 10.1111/jth.14810
57. Cohen A, Harari E, Cipok M, Laish-Farkash A, Bryk G, Yahud E, et al. Immature platelets in patients hospitalized with COVID-19. J Thromb Thrombolysis. (2021) 51:608–16. doi: 10.1007/s11239-020-02290-6
58. Welder D, Jeon-Slaughter H, Ashraf B, Choi S.-H., Chen W, et al. Immature platelets as a biomarker for disease severity and mortality in COVID-19 patients. Br J Haem. (2021) 194:530–6. doi: 10.1111/bjh.17656
59. Guthikonda S, Lev EI, Patel R, DeLao T, Bergeron AL, Dong JF, et al. Reticulated platelets and uninhibited COX-1 and COX-2 decrease the antiplatelet effects of aspirin. J Thromb Haemost. (2007) 5:490–6. doi: 10.1111/j.1538-7836.2007.02387.x
60. Ibrahim H, Nadipalli S, DeLao T, Guthikonda S, Kleiman NS. Immature platelet fraction (IPF) determined with an automated method predicts clopidogrel hyporesponsiveness. J Thromb Thrombolysis. (2012) 33:137–42. doi: 10.1007/s11239-011-0665-7
61. Armstrong PC, Hoefer T, Knowles RB, Tucker AT, Hayman MA, Ferreira PM, et al. Newly formed reticulated platelets undermine pharmacokinetically short-lived antiplatelet therapies. Atheroscler Thromb Vasc Biol. (2017) 37:949–56. doi: 10.1161/ATVBAHA.116.308763
62. Lippi G, Henry BM, Favaloro EJ. Mean platelet volume predicts severe COVID-19 illness. Semin Thromb Hemost. (2021) 47:456–9. doi: 10.1055/s-0041-1727283
63. Manne BK, Denorme F, Middleton EA, Portier I, Rowley JW, Stubben C, et al. Platelet expression and function in patients with COVID-19. Blood. (2020) 136:1317–29. doi: 10.1182/blood.2020007214
64. Barrett TJ, Cornwell M, Mynzdar K, Rolling CC, Xia Y, Drenkova K, et al. Platelets amplify endotheliopathy in COVID-19. Sci Adv. (2021) 7:eabh2434. doi: 10.1126/sciadv.abh2434
65. Yatim N, Boussier J, Chocron R, Hadjadj J, Philippe A, Gendron N. Platelet activation in critically ill COVID-19 patients. Ann Intens Care. (2021) 11:113. doi: 10.1186/s13613-021-00899-1
66. Duarte-Neto AN, Monteiro RAA, da Silva LFF, Malheiros D. M. A. C., de Oliveira EP, et al. Pulmonary and systemic involvement in COVID-19 patients assessed with ultrasound-guided minimally invasive autopsy. Histopathology. (2020) 77:186–97. doi: 10.1111/his.14160
67. Fox SE, Akmatbekov A, Harbert JL, Li G, Brown JQ, Vander Heide RS. Pulmonary and cardiac pathology in African American patients with COVID-19: an autopsy series from New Orleans. Lancet Respir Med. (2020) 8:681–6. doi: 10.1016/S2213-2600(20)30243-5
68. Rapkiewicz AV, Mai X, Carsons SE, Pittaluga S, Kleiner DE, Berger JS, et al. Megakaryocytes and platelet-fibrin thrombi characterize multi-organ thrombosis at autopsy in COVID-19: a case series. EClinicalMedicine. (2020) 24:100434. doi: 10.1016/j.eclinm.2020.100434
69. Valdivia-Mazeyra MF C, Salas JM, Nieves-Alonso L, Martin-Fragueiro C, Barcena P, et al. Increased number of pulmonary megakaryocytes in COVID-19 patients with diffuse alveolar damage: an autopsy study with clinical correlation and review of the literature. Virchows Arch. (2021) 478:487–96. doi: 10.21203/rs.3.rs-49093/v1
70. Nauen DW, Hooper JE, Stewart CM, Solomon IH. Assessing brain capillaries in coronavirus disease. JAMA Neurol. (2021) 78:760–2. doi: 10.1001/jamaneurol.2021.0225
71. McMullen P, Smith H, Pytel P. Entrapped megakaryocytes in the microvasculature of brain tissues are not specific to COVID-19 but can be seen across a spectrum of acute lung injuries. J Neuropathol Exp Neurol. (2021) 80:1078–80. doi: 10.1093/jnen/nlab048
72. Roncati L, Ligabue G, Nasillo V, Lusenti B, Gennari W, Fabbiani L, et al. A proof of evidence supporting abnormal immunothrombosis in severe COVID-19: naked megakaryocyte nuclei in the bone marrow and lungs of critically ill patients. Platelets. (2020) 31:1085–9. doi: 10.1080/09537104.2020.1810224
73. Schurink B, Radonic T, Barbe E, Bouman CSC, de Boer HH, de Bree GJ, et al. Viral presence and immunopathology in patients with lethal COVID-19: a prospective autopsy cohort study. Lancet Microbe. (2020) 1:e290–9. doi: 10.1016/S2666-5247(20)30144-0
74. Levine RF, Eldor A, Shoff PK, Kirwin S, Tenza D, Cramer EM, et al. Circulating megakaryocytes: delivery of large numbers of intact, mature megakaryocytes to the lungs. Eur J Haematol. (1993) 51:233–46. doi: 10.1111/j.1600-0609.1993.tb00637.x
75. Lefrancais E, Ortiz-Munoz G, Caudrillier A, Mallavia B, Liu F, Sayah DM, et al. The lung is a site of platelet biogenesis and a reservoir for hematopoietic progenitors. Nature. (2017) 544:105–9. doi: 10.1038/nature21706
76. Pariser DN, Hilt ZT, Ture SK, Blick-Nitko SK, Looney MR, Cleary SJ, et al. Lung megakaryocytes are immune modulatory cells. J Clin Invest. (2021) 131:e137377. doi: 10.1172/JCI137377
77. Chen X, Zhong J, Han D, Yao F, Zhao J, Wagenaar GTM, et al. Close association between platelet biogenesis and alveolarization of the developing lung. Front Pediatr. (2021) 9:625031. doi: 10.3389/fped.2021.625031
78. Chen Z, Zhang F, Hu W, Chen Q, Li C, Wu L, et al. Laboratory markers associated with COVID-19 progression in patients with or without comorbidity: A retrospective study. J Clin Lab Anal. (2021) 35:e23644. doi: 10.1002/jcla.23644
79. Wu L, Guo N, Xu Z, Wang W, Xiong Q, Hu J, et al. Low arterial oxygen partial pressure induces pulmonary thrombocytopenia in patients and a mouse model. BMC Pulm Med. (2021) 21:3. doi: 10.1186/s12890-020-01381-7
80. Fortmann SD, Patton MJ, Frey BF, Vieira CP, Reddy SB, Huls F, et al. Megakaryocytes are a novel SARS-CoV2 infection target and risk factor for mortality and multi-organ failure. medRxiv. (2021). doi: 10.1101/2021.08.05.21261552
81. Bernardes JP, Mishra N, Tran F, Bahmer T, Best L, Blasé JI, et al. (2020). Longitudinal multi-omics analyses identify responses of megakaryocytes, erythroid cells, and plasmablasts as hallmarks of severe COVID-19. Immunity. (2020) 53:1296–314.e9. doi: 10.1016/j.immuni.2020.11.017
82. Brunet-Ratnasingham E, Anand SP, Gantner P, Moquin-Beadry G, Dyachenko A, Brassard N, et al. Integrated immunovirological profiling validates plasma SARS-CoV2-RNA as an early predictor of COVID-19 mortality. medRxiv. (2021). doi: 10.1101/2021.03.18.21253907
83. Olson JD, Cunningham MT, Higgins RA, Eby CS, Brandt JT. D-dimer: simple test, tough problems. Arch Pathol Lab Med. (2013) 137:1030–8. doi: 10.5858/arpa.2012-0296-CP
84. Goodwin AJ, Higgins RA, Moser KA, Smock KJ, Chandler VL, Kottke-Marchant K, et al. Issues surrounding age-adjusted D-dimer cutoffs that practicing physicians need to know when evaluating patients with suspected pulmonary embolism. Ann Intern Med. (2017) 166:361–4. doi: 10.7326/M16-2030
85. Favaloro EJ, Thachil J. Reporting of D-dimer data in COVID-19: some confusion and potential for misinformation. Clin Chem Lab Med. (2020) 58:1191–9. doi: 10.1515/cclm-2020-0573
86. Hardy M, Lecompte T, Douxfils J, Lessire S, Dogné JM, Chatelain B, et al. Management of the thrombotic risk associated with COVID-19: guidance for the hemostasis laboratory. Thromb J. (2020) 18:17. doi: 10.1186/s12959-020-00230-1
87. Hunt BJ, Levi M. The source of elevated plasma D-dimer levels in COVID-19 infection. Br J Haematol. (2020) 190:e133–4. doi: 10.1111/bjh.16907
88. Thoreau B, Galland J, Delrue M, Neuwirth M, Stepanian A, Chauvin A, et al. D-Dimer level and neutrophils count as predictive and prognostic factors of pulmonary embolism in severe non-ICU COVID-19 patients. Viruses. (2021) 13:758. doi: 10.3390/v13050758
89. Dupont A, Rauch A, Staessens S, Moussa M, Rosa M, Corseaux D, et al. Vascular endothelial damage in the pathogenesis of organ injury in severe COVID-19. Arterioscler Thromb Vasc Biol. (2021) 41:1760–73. doi: 10.1161/ATVBAHA.120.315595
90. Zuo Y, Yalavarthi S, Shi H, Gockman K, Zuo M, Madison JA, et al. Neutrophil extracellular traps in COVID-19. JCI Insight. (2020) 5:e138999. doi: 10.1172/jci.insight.138999
91. Middleton EA, He X.-Y., Denorme F, Campbell RA, Ng D, et al. Neutrophil extracellular traps contribute to immunothrombosis in COVID-19 acute respiratory distress syndrome. Blood. (2020) 136:1169–79. doi: 10.1182/blood.2020007008
92. Skendros PA, Mitsios A, Chrysanthopoulou DC, Mastellos S, Metallidis P, Rafailidis M, et al. Complement and tissue factor-enriched neutrophil extracellular traps are key drivers in COVID-19 immunothrombosis. J Clin Invest. (2020) 130:6151–7. doi: 10.1172/JCI141374
93. Veras FP, Pontelli MC, Silva CM, Toller-Kawahisa JE, de Lima M, Nascimento DC, et al. SARS-CoV2-triggered neutrophil extracellular traps mediate COVID-19 pathology. J Exp Med. (2020) 217:e20201129. doi: 10.1084/jem.20201129
94. Ng H, Haverwall S, Rosell A, Aguilera K, von Meijenfeldt FA, Mackman N, et al. Circulating markers of neutrophil extracellular traps are of prognostic value in patients with COVID-19. Arterioscler Thromb Vasc Biol. (2021) 41:988–94. doi: 10.1161/ATVBAHA.120.315267
95. Martinod K, Wagner DD. Thrombosis: tangled up in NETs. Blood. (2014) 123:2768–76. doi: 10.1182/blood-2013-10-463646
96. Fraser DD, Patterson EK, Slessarev M, Gill SE, Martin C, Daley M. Endothelial injury and glycocalyx degradation in critically ill coronavirus disease 2019 patients: implications for microvascular platelet aggregation. Crit Care Explor. (2020) 2:e0194. doi: 10.1097/CCE.0000000000000194
97. Rauch A, Dupont A, Goutay J, Caplan M, Staessens S, Moussa M, et al. Endotheliopathy is induced by plasma from critically ill patients and associated with organ failure in severe COVID-19. Circulation. (2020) 142:1881–4. doi: 10.1161/CIRCULATIONAHA.120.050907
98. Cappellano G, Raineri D, Rolla R, Giordano M, Puricelli C, Vilardo B, et al. Circulating platelet-derived extracellular vesicles are a hallmark of SARS-Cov-2 infection. Cells. (2021) 10:85. doi: 10.3390/cells10010085
99. Guervilly C, Bonifay A, Burtey S, Sabatier F, Cauchois R, Abdili E, et al. Dissemination of extreme levels of extracellular vesicles: tissue factor activity in patients with severe COVID-19. Blood Adv. (2021) 5:628–34. doi: 10.1182/bloodadvances.2020003308
100. Traby L, Kollars M, Kussmann M, Karer M, Sinkovec H, Lobmeyr E, et al. Extracellular vesicles and citrinullated histone H3 in Coronavirus disease 2019 patients. Thromb Haemost. (2022) 122:113–22. doi: 10.1055/a-1522-4131
101. Francischetti IMB, Toomer K, Zhang Y, Jani J, Siddiqui Z, Brotman DJ, et al. Upregulation of pulmonary tissue factor, loss of thrombomodulin and immunothrombosis in SARS-CoV-2 infection. EClinicalMedicine. (2021) 39:101069. doi: 10.1016/j.eclinm.2021.101069
102. Balbi C, Burello J, Bolis S, Lazzarini E, Biemmi V, Pianezzi E, et al. Circulating extracellular vesicles are endowed with enhanced procoagulant activity in SARS-CoV-2 infection. EBioMedicine. (2021) 67:103369. doi: 10.1016/j.ebiom.2021.103369
103. Krishnamachary B, Cook S, Kumar A, Spikes L, Chalise P, Dhillon NK. Extracellular vesicle-mediated endothelial apoptosis and EV-associated proteins correlate with COVID-19 disease severity. J Extracel Vesicles. (2021) 10:e12117. doi: 10.1002/jev2.12117
104. Chandrashekar V. DIC score: statistical relationship with PT, aPTT, and simplified scoring systems with combinations pf PT and aPTT. ISRN Hematol. (2012) 2012:579420. doi: 10.5402/2012/579420
105. Huang C, Wang Y, Li X, Ren L, Zhao J, Hu Y, et al. Clinical features of patients infected with 2019 novel coronavirus in Wuhan, China. Lancet. (2020) 395:497–506. doi: 10.1016/S0140-6736(20)30183-5
106. Bowles L, Platton S, Yartey N, Dave M, Lee K, Hart DP, et al. Lupus anticoagulant and abnormal coagulation tests in patients with COVID-19. N Engl J Med. (2020) 383:288–90. doi: 10.1056/NEJMc2013656
107. Harzallah I, Debliquis A, Drenou B. Lupus anticoagulant is frequent in patients with Covid-19. J Thromb Haemost. (2020) 18:2064–5. doi: 10.1111/jth.14867
108. Nougier C, Benoit R, Simon M, Desmurs-Clavel H, Marcotte G, Argaud L., et al. Hypofibrinolytic state and high thrombin generation may play a major role in SARS-Cov2 associated thrombosis. J Thromb Haemost. (2020) 18:2215–9. doi: 10.1111/jth.15016
109. Correa TD, Cordioli RL, Guerra JCC, da Silva BC, Rodrigues RR, de Souza GM, et al. Coagulation profile of COVID-19 patients admitted to the ICU: exploratory study. PLoS ONE. (2020) 15:e0243604. doi: 10.1371/journal.pone.0243604
110. von Meijenfeldt FA, Havervali S, Adelmeijer J, Lundström A, Rudberg A.-S., et al. Prothrombotic changes in patients with COVID-19 are associated with disease severity and mortality. Res Pract Thromb Haemost. (2021) 5:132–41. doi: 10.1002/rth2.12462
111. Pavoni V, Gianesello L, Pazzi M, Stera C, Meconi T, Frigieri FC. Evaluation of coagulation function by rotation thromboelastometry in critically ill patients with severe COVID-19 pneumonia. J Thromb Thrombolysis. (2020) 50:281–6. doi: 10.1007/s11239-020-02130-7
112. Fan BE, Ng J, Chan SSW, Christopher D, Tso ACE, Ling AM, et al. COVID-19 associated coagulopathy in critically ill patients: a hypercoagulable state demonstrated by parameters of haemostasis and clot waveform analysis. J Thromb Thrombolysis. (2021) 51:663–74. doi: 10.1007/s11239-020-02318-x
113. Bareille M, Hardy M, Douxfils J, Roullet S, Lasne D, Levy JH, et al. Viscoelastometric testing to assess hemostasis of COVID-19: a systematic review. J Clin Med. (2021) 10:1740. doi: 10.3390/jcm10081740
114. Pawlowski C, Wagner T, Puranik A, Murugadoss K, Loscalzo L, Venkatakrishnan AJ, et al. Inference from longitudinal laboratory tests characterizes temporal evolution of COVID-19-associated coagulopathy. ELife. (2020) 9:e59209. doi: 10.7554/eLife.59209
115. Terpos E, Ntanasis-Stathopoulos I, Elalamy I, Kastritis E, Sergentanis TN, Politou M, et al. Hematological findings and complications of COVID-19. Am J Hematol. (2020) 95:834–47. doi: 10.1002/ajh.25829
116. Juneja GK, Castelo M, Yeh CH, Cerroni SE, Hansen BE, Chessum JE, et al. Biomarkers of coagulation, endothelial function, and fibrinolysis in critically ill patients with COVID-19: a single-center prospective longitudinal study. J Thromb Haemost. (2021) 19:1546–57. doi: 10.1111/jth.15327
117. Hardy M, Michaux I, Lessire S, Douxfils J, Dogné JM, Bareille M, et al. Prothrombotic disturbances of hemostasis of patients with severe COVID-19: a prospective longitudinal observational study. Thromb Res. (2021) 197:20–3. doi: 10.1016/j.thromres.2020.10.025
118. Weber GM, Hong C, Palmer NP, Avillach P, Murphy SN, Gutiérrez-Sacristán A, et al. International comparisons of harmonized laboratory value trajectories to predict severe COVID-19: leveraging the 4CE collaborative across 342 hospitals and 6 countries: a retrospective cohort study. medRxiv. (2021) 2020.12.16.20247684. doi: 10.1101/2020.12.16.20247684
Keywords: COVID-19, coagulopathy, thrombosis, D-dimers, platelets, fibrinogen, prothrombin time, megakaryocyte
Citation: Len P, Iskakova G, Sautbayeva Z, Kussanova A, Tauekelova AT, Sugralimova MM, Dautbaeva AS, Abdieva MM, Ponomarev ED, Tikhonov A, Bekbossynova MS and Barteneva NS (2022) Meta-Analysis and Systematic Review of Coagulation Disbalances in COVID-19: 41 Studies and 17,601 Patients. Front. Cardiovasc. Med. 9:794092. doi: 10.3389/fcvm.2022.794092
Received: 13 October 2021; Accepted: 11 January 2022;
Published: 11 March 2022.
Edited by:
Annika Lundström, Karolinska Institutet (KI), SwedenReviewed by:
Francois Mullier, CHU Dinant Godinne UCL Namur, BelgiumFrancesco Poletto, University of Padua, Italy
Copyright © 2022 Len, Iskakova, Sautbayeva, Kussanova, Tauekelova, Sugralimova, Dautbaeva, Abdieva, Ponomarev, Tikhonov, Bekbossynova and Barteneva. This is an open-access article distributed under the terms of the Creative Commons Attribution License (CC BY). The use, distribution or reproduction in other forums is permitted, provided the original author(s) and the copyright owner(s) are credited and that the original publication in this journal is cited, in accordance with accepted academic practice. No use, distribution or reproduction is permitted which does not comply with these terms.
*Correspondence: Makhabbat S. Bekbossynova, YXN0YW5hbWFoYSYjeDAwMDQwO2dtYWlsLmNvbQ==; Natasha S. Barteneva, bmF0YWxpZS5iYXJ0ZW5ldmEmI3gwMDA0MDtudS5lZHUua3o=
†These authors have contributed equally to this work