- 1Department of Ultrasound in Medicine, Shanghai Jiao Tong University Affiliated 6th People's Hospital, Shanghai Institute of Ultrasound in Medicine, Shanghai, China
- 2Department of Endocrinology and Metabolism, Shanghai Jiao Tong University Affiliated Sixth People's Hospital, Institute of Shanghai Diabetes, Shanghai, China
- 3Department of Cardiovascular Surgery, The Affiliated Hospital of Qingdao University, Qingdao, China
- 4Department of Ultrasound in Medicine, South Hospital of Shanghai Jiao Tong University Affiliated 6th People's Hospital, Shanghai, China
Objective: Vascular endothelial dysfunction is considered an early predictor of endothelial injury and the initiating factor of atherosclerosis (AS). Brachial artery flow-mediated dilation (FMD) can detect endothelial injury early and provide important prognostic information beyond traditional cardiovascular (CV) risk factors. This study aimed to find the influencing factors of FMD and develop a simple prediction model in populations with different body mass indices (BMIs).
Methods: In total, 420 volunteers with different BMIs were recruited in our study. Subjects were randomly assigned to the derivation and validation cohorts (the ratio of the two was 1:2) with simple random sampling. The former was used for influencing factors searching and model construction of FMD and the latter was used for verification and performance evaluation.
Results: The population was divided into two groups, i.e., 140 people in the derivation group and 280 people in the verification group. Analyzing in the training data, we found that females had higher FMD than males (p < 0.05), and FMD decreased with age (p < 0.05). In people with diabetes, hypertension or obesity, FMD was lower than that in normal individuals (p < 0.05). Through correlation analysis and linear regression, we found the main influencing factors of FMD: BMI, age, waist-to-hip radio (WHR), aspartate aminotransferase (AST) and low-density lipoprotein (LDL). And we developed a simple FMD prediction model: FMD = −0.096BMI−0.069age−4.551WHR−0.015AST−0.242LDL+17.938, where R2 = 0.599, and adjusted R2 = 0.583. There was no statistically significant difference between the actual FMD and the predicted FMD in the verification group (p > 0.05). The intra-class correlation coefficient (ICC) was 0.77. In a Bland-Altman plot, the actual FMD and the predicted FMD also showed good agreement. This prediction model had good hints in CV risk stratification (area under curve [AUC]: 0.780, 95 % confidence intervals [95% CI]: 0.708–0.852, p < 0.001), with a sensitivity and specificity of 73.8 and 72.1%, respectively.
Conclusions: Males, older, obesity, hypertension, diabetes, smoking, etc. were risk factors for FMD, which was closely related to CV disease (CVD). We developed a simple equation to predict FMD, which showed good agreement between the training and validation groups. And it would greatly simplify clinical work and may help physicians follow up the condition and monitor therapeutic effect. But further validation and modification bears great significance.
Introduction
AS is the most common pathological mechanism of coronary artery disease, peripheral artery disease and cerebrovascular disease (1). The chronic accumulation of subendothelial atherosclerotic plaque or the shedding of unstable plaque will cause luminal stenosis to restrict blood flow and cause severe tissue hypoxia, eventually causing myocardial infarction and stroke, which is the most common cause of death in the world (2). Vascular endothelial dysfunction is generally considered an early predictor of endothelial injury and the initiating factor of AS (3). Thus, early recognition of endothelial injury can help take intervention measures as soon as possible to effectively delay or even reverse the process of AS.
The evaluation methods of endothelial function are mainly divided into invasion and non-invasion. In the past, invasive inspections such as coronary angiography and forearm plethysmography (4) were often used. However, these invasive techniques were burdensome for the subjects with low repeatability and high risk characteristics. Especially for healthy people or asymptomatic patients, it was usually inappropriate to perform these examinations. Therefore, some non-invasive technologies have emerged with higher repeatability and accurate and reliable results. Carotid intima media thickness (CIMT) measurement is an effective method for the early assessment of AS (5). With its simple operation and low price, the ultrasound measurement of CIMT is widely used in the clinical screening of vascular diseases. However, there is interobserver variability in measurement, and its accuracy must be improved. Peripheral arterial tonometry (PAT) is an effective method to evaluate the microvascular function (6). The vasomotor function is evaluated by vasodilation after compression, and the results obtained by its automatic detection program are more reliable. Correspondingly, FMD is currently the most commonly used method to evaluate the endothelial function (7). Its result is accurate, reliable and highly repeatable, and it is considered the most important method to non-invasively evaluate the endothelial function.
However, there are many problems in the application of FMD in daily clinical practice. With its high requirements for operation skills, it requires well-trained operators. The discomfort caused by prolonged pressurization makes it unacceptable. The inspect equipment is also expensive, especially in primary hospitals, which results in much more difficult assessments.
Thus, we developed a prediction model based on FMD results with anthropometric measurements and laboratory analysis to facilitate the evaluation of endothelial damage in populations with different BMIs. It was helpful to popularize at primary hospitals and convenient for early detection and early intervention, which we hope to improve the prognosis of atherosclerosis.
Methods
Participant Selection and Design
This study consequently enrolled subjects from Jan 2021 to April 2021 in the Sixth People's Hospital Affiliated to Shanghai Jiao Tong University, China. Patients with the following conditions were excluded from our study: current malignancy with a life expectancy <6 months; undergoing radiotherapy, chemotherapy, or molecular targeted therapy; severe mental illness or language barriers that prevented normal communication with researchers. In total, 420 subjects aged 18–60 years were recruited, including 108 males and 312 females, all of whom were Chinese. FMD and anthropometric measurements and laboratory analysis were performed on all participants. Hypertension was defined as > 140/90 mm Hg according to the 2018 European Society of Cardiology (ESC)/European Society of Hypertension (ESH) guidelines (8). Criteria for the diagnosis of diabetes was fasting blood glucose (FBG) ≥ 7.0 mmol/L or 2-h plasma glucose value ≥ 11.1 mmol/L during a 75-g oral glucose tolerance test or the glycated hemoglobin (HbA1c) ≥ 6.5% or patient with classic symptoms of hyperglycemia or hyperglycemic crisis, a random plasma glucose ≥ 11.1 mmol/L (9). Given that total cholesterol (TC) and/or high-density lipoprotein (HDL) are included as risk assessment factors in many guidelines on the assessment of cardiovascular risk (10–12), we tentatively classified TC ≥ 5.2 mmol/l or HDL < 1.0 mmol/l as the dyslipidemia group for ease of analysis. Then, the population was randomly assigned to the derivation and validation cohorts (the ratio of the two was 1:2) with simple random sampling to search for the influencing factors of FMD and construct and verify the equation. The protocol was approved by the Shanghai Jiao Tong University Ethics Committee and conformed to the Declaration of Helsinki. All subjects provided written informed consent to participate in the study.
FMD and CIMT Measurement
FMD was measured according to the guideline (13) with an Omron UNEX EF 38G (UNEX Corporation, Nagoya, Japan) tester, and the probe frequency was 10 MHz. Prior to the FMD examination, subjects should fast (>6 h), avoid exercise (>24 h), and refrain from caffeine, vitamin C and alcohol (>12 h). Smokers must refrain from smoking for >6 h. When subjects take drugs, they should wait 4 times the half-life of the drug. Then, they should be studied in a quiet, temperature-controlled room and relaxed for at least 10 min to relieve mental stress and physical activity. The blood pressure was measured twice on the right arm in the sitting position, and the average value was obtained. Then, in the supine position with the right arm extended, the cuff was placed on the forearm, and the brachial artery was scanned 3–5 cm above the antecubital fossa. We used an H-shaped probe capturing two short-axis and one long-axis for automatic probe position correction to identify clear vascular boundaries. And a probe-holding device was used to further ensure optimal image. First, the baseline inner diameter of brachial artery was measured; then, the cuff was inflated to a pressure of 50 mmHg higher than the subject's systolic blood pressure and held for 5 min. Afterwards, the cuff was deflated, and the diameter of the artery was measured again over the next 3 min to capture the peak brachial artery diameter. The continuous measurement process was monitoring by automatic edge-detections systems. Finally, the FMD result was automatically calculated by the machine: FMD = [(maximum diameter – diameter at rest)/diameter at rest] × 100 (%). FMD was measured twice for each participant at an interval of 20 min, and the average value was obtained.
CIMT was performed using a MINDRAY DC-8 or a MINDRAY DC-80S ultrasound machine with a 10-MHz linear probe. Patients were examined in the supine position with a slight rotation of the neck to the contralateral side. CIMT was defined as the distance between the lumen-intima boundary and the media-adventitia boundary. And it was measured at ~1 cm posterior to the common carotid artery bifurcation at a site with uniform intima without plaque lesions. The proximal and distal walls were measured and averaged. Finally, the average value of the left and right common carotid arteries was taken as the average CIMT.
The entire inspection project for FMD and CIMT was completed by two experienced physician with at least 3 years of operating experience and the physicians did not know any information about them.
Clinical and Laboratory Analysis
Operators used a digital scale to measure the height and weight of participants, who had to take off shoes and wear light clothes. Then, we calculated the BMI = body weight (kg)/height squared (m2). The waist circumference was measured using tape around the abdomen through the midpoint of the lower edge of the costal arch and the anterior superior spine by a trained examiner. Then, the subjects stood upright with legs close together, and the tape was placed horizontally on their front pubic symphysis and the most convex part of the back gluteus maximus to measure the hip circumference. And the WHR was calculated using the formula: WHR = waist circumference (cm)/hip circumference (cm). The systolic blood pressure (SBP) and diastolic blood pressure (DBP) were measured twice in a quiet state for 1 day, and mean values were used for analysis. Mean arterial pressure (MAP) was expressed as MAP = (SBP+2 × DBP)/3.
We searched for cardiovascular risk factors proposed by some guidelines, and on this basis, added variables that may be associated with FMD for model construction: triglyceride (TG), TCHDL, LDL, alanine aminotransferase (ALT), AST, γ-glutamyl transpeptidase (γ-GT), alkaline phosphatase (ALP), free triiodothyronine (FT3), free tetraiodothyronine (FT4), thyroid stimulating hormone (TSH), prealbumin (PAB), total bile acid (TPA), total bilirubin (TBiL), direct bilirubin (DBiL), serum creatinine (Scr), serum uric acid (SUA), blood urea nitrogen (BUN), retinol-binding protein (RBP), cystatin C (CysC), FBG, HbA1c, insulin, and C-peptide (CP). Venous blood samples were drawn from all subjects in the early morning after 8 h of fasting. All biochemical determinations were performed in the same laboratory using standard laboratory methods. Insulin was measured by radioimmunoassay (ADVIA Insulin Ready Pack 100, Bayer Diagnostics, Milan, Italy) with intra- and inter-assay coefficients of variation < 5%.
Statistical Analysis
Statistical analyses were performed with the SPSS software, version 26 and MedCalc software, 20.0.3. The normality of distribution was checked with Kolmogorov–Smirnov test. Normally distributed data are expressed as the mean ± standard deviation, whereas data not normally distributed are expressed as the median and interquartile range. Categorical variables were expressed as percentages. Independent two-sample t-test and non-parametric test (Mann–Whitney) were used for comparison of quantitative data. Pearson's chi-square (χ2) was used to compare categorical data. We first analyzed the difference of FMD among subgroups of different genders, different ages and with or without hypertension, diabetes or dyslipidemia. And then we further searched for the influencing factors of FMD through correlation analysis (p < 0.01, r > 0.3) and linear regression (p < 0.01), and constructed a FMD prediction model based on multivariate linear regression (stepwise). Finally, validation and performance evaluation were performed in the validation sample by ICC and Bland–Altman plot. In addition, we analyzed the value of our predictive equation in identifying risk factors for CVD through receiver operating characteristic (ROC) curves. A p < 0.05 indicated statistical significance.
Results
Baseline Characteristics and Within-Group Analysis
In total, 420 subjects fulfilled the inclusion criteria and were divided into a derivation group and a verification group by random sampling. The ratio of the two was 1:2, i.e., 140 people in the derivation group and 280 people in the validation group. The demographic and clinical characteristics of the two groups are shown in Table 1. There was no statistically significant difference between them (p > 0.05).
In the derivation group, we performed sub-group analysis (Table 2, Figure 1). The initial assessment in gender subgroups revealed that women presented higher FMD than men (p < 0.05) (Figure 1A), whereas CIMT was not statistically different (p > 0.05). In different age groups, we found that younger people had higher FMD (Figure 1B) and lower CIMT (Figure 2A) than older people (p < 0.05). Analysis of BMI subgroups revealed that obese people presented lower FMD (Figure 1C) and higher CIMT (Figure 2B) than non-obese individuals (p < 0.05). People with hypertension and diabetes showed lower FMD (Figures 1D,E) and higher CIMT (Figures 2C,D) than those without such diseases (p < 0.05), while taking drugs or not had no significant effect on FMD or CIMT in population with such diseases (p > 0.05). Smokers had lower FMD than non-smokers (p < 0.05) (Figure 1F). However, analysis of the population with or without dyslipidemia revealed that there was no significant difference in FMD or CIMT (p > 0.05).
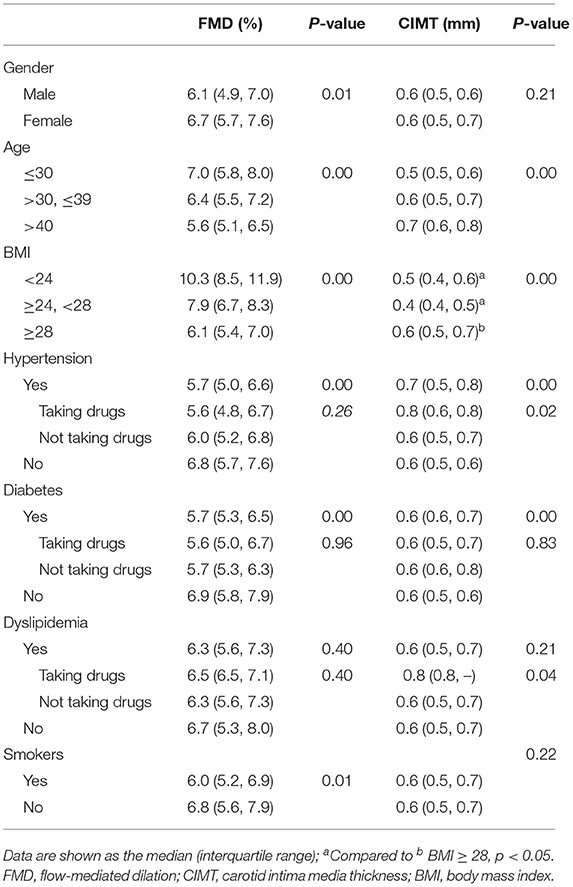
Table 2. Values of FMD and CIMT in subgroups of gender, age, BMI, hypertension, diabetes, dyslipidemia and smokers.
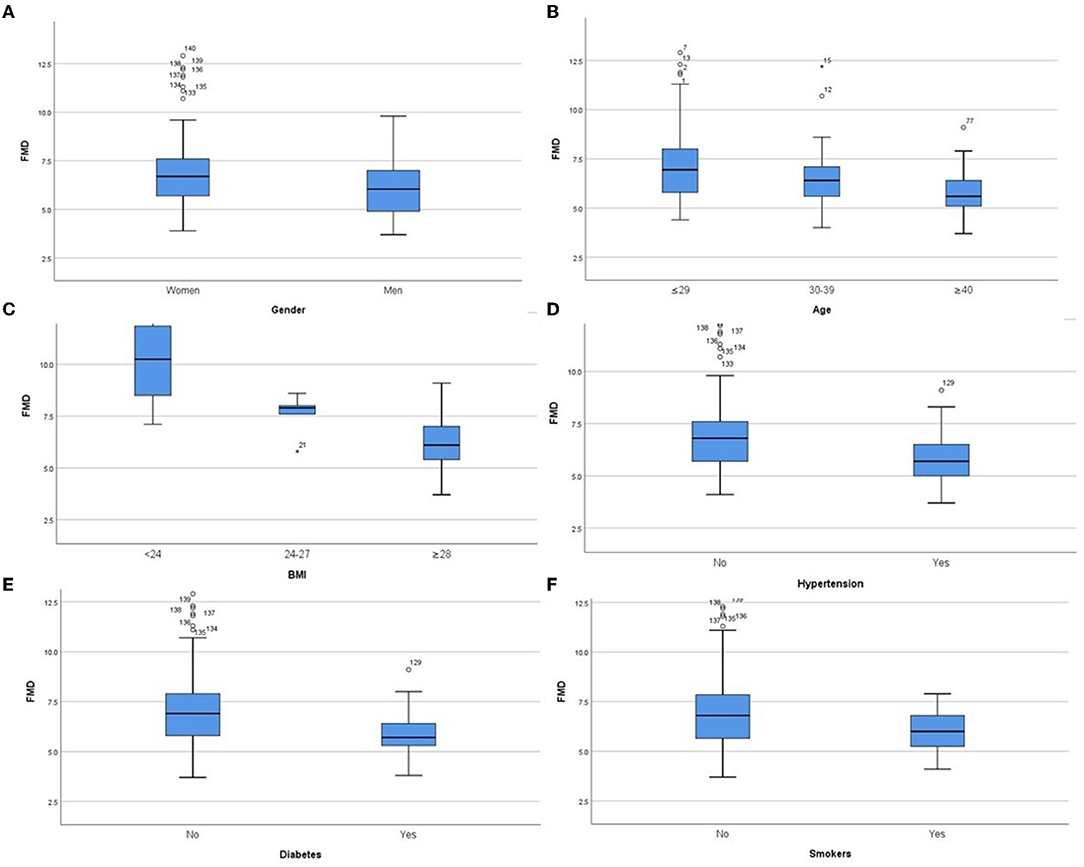
Figure 1. Comparison of FMD values in subgroups of both genders (A), different ages (B), different BMIs (C), hypertension (D), diabetes (E) and smoking (F). P < 0.05 in all subgroups.
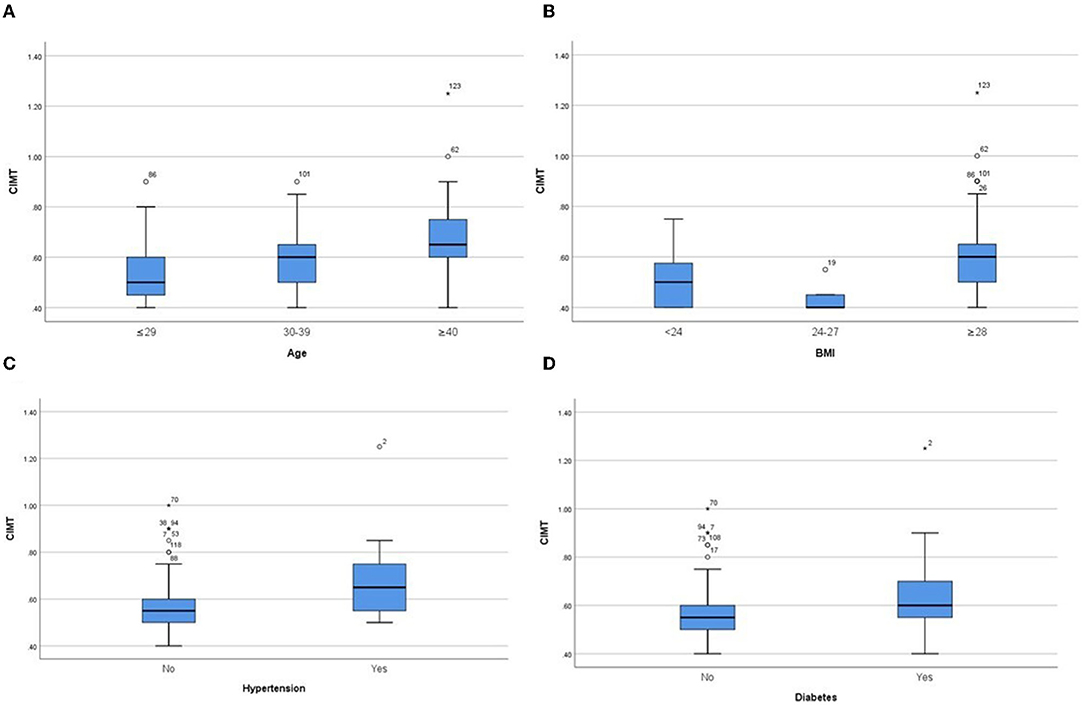
Figure 2. Comparison of CIMT values in subgroups of different ages (A), different BMIs (B), hypertension (C) and diabetes (D). P < 0.05 in all subgroups.
Correlation and Univariate Analysis
Before constructing the prediction equation, we first looked for variables that were clearly related to FMD. We performed bivariate correlation analysis and univariate regression analysis on the above variables and FMD, and screened out 16 potential risk factors (p < 0.01) after excluding multicollinearity issues, as shown in Table 3. Multivariate logistic regression was later performed to screen for significant variables associated with FMD.
Equation Development
After multiple variables combination and modification, we finally selected the following variables for modeling (stepwise, p < 0.01), and the expression was:
R2 = 0.598, and adjusted R2 = 0.582. The regression coefficient and 95% CI of each independent variable are shown in Table 4. The independence test of model residuals, i.e., Durbin-Watson test, was 2.184, which indicates good independence between variables. The collinearity analysis show that the VIF values between the independent variables were <2, which indicates that there was no synteny problem among the four independent variables that we introduced. In addition, we draw a residual scatter plot with the standardized predicted value on the X-axis and the standardized residual on the Y-axis (Figure 3). The scatter points were randomly distributed, and the slope was almost zero. We believe that there was no possibility of autocorrelation. The scatter plot of the standardized predicted value and dependent FMD shows a linear trend (Figure 4).
Verification of Equations
To verify the effectiveness of our model, we compared the actual FMD values to predicted FMD values in the verification group. ICC of them was 0.767 (95% CI: 0.704–0.816; p < 0.001). The two-related-sample test (Wilcoxon) shows that there was no statistically significant difference between the actual FMD and the predicted FMD (P > 0.05). Next, we drew a Bland–Altman plot (Figure 5), which shows that the average value of the differences (middle horizontal solid line) was close to zero (middle horizontal dotted line). Most of the differences between actual FMD and predicted FMD were within the 95% limits of agreement, and only 3% (13/420) of the points lied outside it. We believed that the actual FMD and predicted FMD had high consistency.
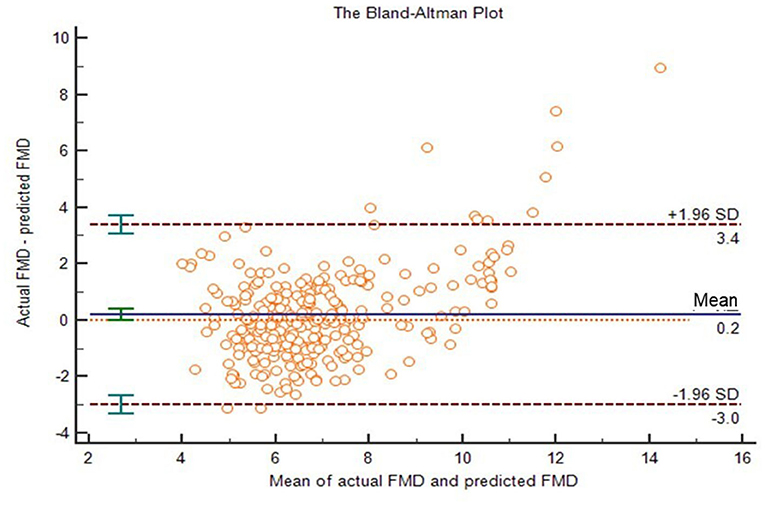
Figure 5. The Bland-Altman plot of actual FMD and predicted FMD. The upper and lower horizontal dotted lines in the picture represented the 95% limits of agreement. The horizontal solid line in the middle represented the average value of the difference. The middle horizontal dotted line indicated the position where the average value of the difference was zero.
To further evaluate the ability of our prediction model to assess CV risk, we divided the validation cohort into groups according to the Framingham Heart Study CV risk stratification updated in 2008 (10-year CV event risk: 0–6%, 6–20% and > 20%) (10). Our study population had no risk >20%, so it was divided into two groups. The AUC of FMD to identify different CV risk stratification was 0.780 (95% CI: 0.708–0.852, p < 0.001), with a sensitivity and specificity of 73.8 and 72.1%, respectively (Figure 6).
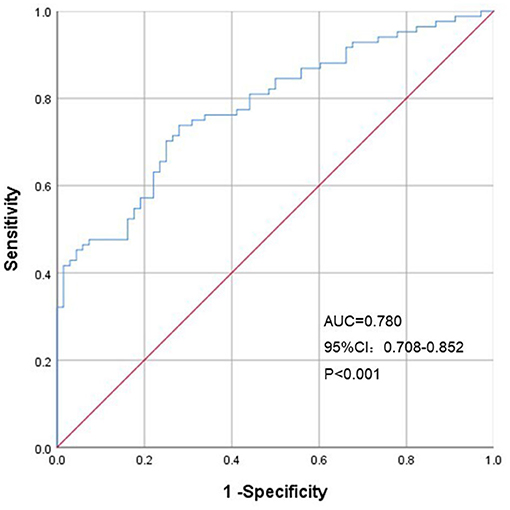
Figure 6. Receiver operating characteristic curves of predicted FMD for Framingham Heart Study CV risk stratification. AUC, area under the curve; CI, confidence interval.
Discussion
The main purpose of this article was to find the influencing factors of vascular endothelial function and establish a simple predictive model of FMD. Endothelial damage is often used as an early marker of various diseases, such as obesity, hypertension, diabetes, and AS. Many studies have shown that FMD can provide important prognostic information beyond traditional CV risk factors (14), and low FMD strongly predicts CV events (15). Therefore, early evaluation of endothelial function is of great significance in diagnosing or monitoring disease progression.
We divided the target population into a derivation group and a verification group through simple random sampling. The ratio of the two was 1:2, which ensured the reliability of the prediction model and reduced the phenomenon of overfitting. The balance test was first performed between them to ensure a random distribution of the indicators. Table 1 shows that their p values were both >0.05, which indicates that there was no significant difference in parameters between them.
We first analyzed the relationship between gender and FMD and found that men had lower FMD than women. Study revealed atherosclerotic CVD prevalence in men was greater than in women until menopause, and some studies showed earlier decrements in endothelial function in men compared to women (16, 17). But sex differences in endothelial function was controversial and others showed similar age-related declines between the sexes (18). After menopause, women may have a higher prevalence of CVD compared to age-matched men (19). This may be due to the lack of protective effects of estrogen. There were few postmenopausal women in our participants (total population: 6/420), the results showed that there was no statistical difference in FMD between men and women (p > 0.05), but the representativeness was insufficient, and the sample size will be expanded in the future, hoping to conduct in-depth research. The prevalence of CVD increases with age in both men and women. CVD in aging is partly a consequence of the vascular endothelial cell senescence and associated vascular dysfunction (20). Vascular oxidative stress and low-grade inflammation increase with age and is the key mechanism of endothelial diastolic dysfunction (21). Simultaneously, the reduction of estradiol associated with aging (postmenopausal females) (22) and epigenetic modifications (23) may also cause endothelial dilation disorders. This represents a major link between aging and cardiovascular risk.
Many studies have demonstrated impaired endothelial function in obesity, so we compered the FMD values in different BMIs. We found that the group with higher BMI had lower FMD and higher CIMT. This suggested the dual damage of vascular endothelial function and structure related to obesity. Prior study of the impact of obesity revealed FMD decreased in the moderately obese compared with the non-obese (24). This was consistent with our results. An increased CIMT had also been reported in obese patients and weight loss was associated with a reduction in CIMT, consistent with a lowering in risk of cardiovascular events (25, 26). And also, we found that people with diabetes, hypertension and smoking showed lower FMD, all of which suggested an association between FMD and cardiovascular risk factors (12, 27, 28).
Then, we performed bivariate correlation analysis and univariate regression analysis to screen out potential risk factors (p < 0.01) to the multivariate logistic regression. After multiple variables combination and modification, we developed a prediction model of FMD, which consisted of BMI, age, WHR, AST and LDL. BMI is a commonly used index to measure the degree of obesity, and BMI≥24 is considered overweight in the Asian population. As we said before, overweight and obesity are factors closely related to vascular endothelial damage (29). Obesity-induced long-term hypoxia, chronic inflammation, oxidative stress and mitochondrial dysfunction are all involved in the development of endothelial dysfunction (30–34). Epigenetics (35) and circulating particles (36, 37) have also been popular mechanisms in recent years. However, obesity-related endothelial damage involves the joint participation of multiple mechanisms, which awaits more in-depth research.
WHR is an important indicator to determine central obesity, and the latter is closely related to an increased risk of CV diseases, even among people with normal BMI (38). Pear-shaped females with normal weight but more fat in the hips and thighs have a lower risk of heart disease and stroke. Fat accumulated in the buttocks and thighs is subcutaneous fat, which has a protective effect. However, the fat of central obesity accumulates in the abdomen, which is visceral fat, and easily releases fatty acids into the blood to cause pathological conditions such as high cholesterol and insulin resistance. This pathological process easily induces early endothelial damage (39, 40).
AST is generally considered a marker of myocardium and liver damage. Perticone et al. reported that AST was closely related to the endothelial function, as evaluated by strain-gauge plethysmography, in both univariate linear analysis and a stepwise multivariate regression model (41). This is consistent with our study. Initial endothelial injury is characterized by endothelial glycocalyx injury, which mediates the release of Syndecan-1 from the endothelium and causes an increase in circulating concentration (42). Researchers found that the concentration of Syndecan-1 was significantly related to AST, and there was an obvious positive correlation between them (43). However, the specific mechanism between AST and vascular endothelial function cannot be fully explained at present, and chronic systemic effects and interorgan communication may promote development.
An increase in pro-atherogenic LDL and its oxidative modifications (ox-LDL) is well known to be a crucial factor in endothelial damage, a key early step and a predictor of the development of AS (44). When the body is continuously exposed to high levels of LDL, inflammatory pathways in vascular endothelial cells are activated to increase local and systemic inflammation, endothelial cell dysfunction and apoptosis, and smooth muscle cell proliferation, resulting in foam cell formation and genesis of AS plaque (45, 46). Therefore, dyslipidemia-induced hyperlipidemic stress is widely recognized as a powerful pathophysiological driver of AS.
However, analysis of these four risk factors also implies that we can improve the function of endotheliocytes through early intervention such as weight loss, a healthy diet and exercise. In some obese people with low FMD, physical exercise or bariatric surgery can increase FMD while losing weight, which suggests the recovery of endothelial injury (14, 47, 48). Aging of endotheliocyte is also not static. Researchers have found that aerobic exercise can significantly prevent endothelial cell aging and change the state of inflammation in the body, especially in elderly individuals (49, 50). The aging rate of endothelial cells after exercise is much lower than that of sedentary peers (51). Mitochondrial-targeted antioxidant supplementation may also play a role in improving age-related vascular dysfunction (52).
Impaired FMD has been associated with conditions predisposing to AS and CVD and represents an early step in the development of subclinical target organ injury and late clinical events (53). Therefore, we intended to evaluate our prediction model in identifying CVD risk stratification. The result showed that FMD model can well identify group with higher CVD risk (AUC = 0.780, p<0.001). Several studies have demonstrated the prognostic value of FMD for CVD events (54, 55). However, some scholars believe that the reproducibility of FMD is low and currently not recommend for the assessment of CV risk. That partly because poor standardization between laboratories and lack of guideline adherence. At present, evidence on these issues pertaining to FMD is incomplete. If FMD can be standardized, it may be an important supplement to CV risk stratification.
The 1:2 grouping method improved the adaptability of our equation. It was easy to operate and very suitable for primary hospitals. On one hand, chronic diseases such as hypertension, diabetes, and obesity occupy the majority of community diseases. Doctors in the community are the “gatekeepers” of the health care system, so monitoring and long-term follow-up of such diseases are very important. On the other hand, basic hospitals are not equipped with sufficient hardware facilities, especially expensive and high-tech machines such as FMD equipment. Thus, a simple and reliable evaluation model as an auxiliary method is of great significance.
Our study had several limitations. First, the sample for model construction and verification was relatively small and data was limited to Chinese individuals. In the future, a prediction model will require a larger sample for verification and we hope to extrapolate the model to other ethnic groups. Second, this was a single-center study. Although the ratio of our training group to the validation group was 1:2 to maximize the generalizability of our model, establishing a standardized and accurate model requires the joint participation of multi-center research. Third, the accuracy of our prediction equation was quite modest. It may be suitable for epidemiological purpose or for primary hospitals. Furthermore, there were differences in the ratio of sex and BMI, and the percentage of males and the non-obese was relatively small in our studied population. In response to these problems, our follow-up research must expand the sample size and maintain the internal balance of each variable.
Conclusion
Short-term changes or longer-term improvements of vascular endothelial function in interventional trials suggest protective or damaging effects. Therefore, using FMD as a surrogate endpoint to observe changes in endothelial function is timely and simple. We explored the influencing factors of FMD and developed a simple prediction equation. This model will greatly simplify clinical work, but further validation in external populations remains necessary.
Data Availability Statement
The data analyzed in this study is subject to the following licenses/restrictions: Requests to access these datasets should be directed to MTYyNzQ4NjE5NiYjeDAwMDQwO3FxLmNvbQ==.
Ethics Statement
The studies involving human participants were reviewed and approved by Chinese Clinical Trial Registry ChiCTR2100041860. The patients/participants provided their written informed consent to participate in this study.
Author Contributions
XL drafted the manuscript. HL performed the statistical analysis. YZ drafted the figure and legend. YG and LS wrote sections of the manuscript. WB and HY designed the outline of the topic and helped on revising the manuscript. All authors contributed to the article and approved the submitted version.
Funding
This project was funded by National Key R&D Program (2021YFC2009100), Shanghai Science and Technology Commission (21Y11910900), Shanghai Sixth People's Hospital Surface Cultivation Project (ynms202110), and Shanghai Pujiang Program (2019PJD036).
Conflict of Interest
The authors declare that the research was conducted in the absence of any commercial or financial relationships that could be construed as a potential conflictof interest.
Publisher's Note
All claims expressed in this article are solely those of the authors and do not necessarily represent those of their affiliated organizations, or those of the publisher, the editors and the reviewers. Any product that may be evaluated in this article, or claim that may be made by its manufacturer, is not guaranteed or endorsed by the publisher.
Acknowledgments
The authors would like to thank study participants for their contribution. Authors and participants consent for data to be shared.
Supplementary Material
The Supplementary Material for this article can be found online at: https://www.frontiersin.org/articles/10.3389/fcvm.2022.766565/full#supplementary-material
Supplementary Figure 1. The scatter plot of the predicted FMD value and the actual FMD value.
Supplementary Figure 2. The scatter plot of age and FMD.
Supplementary Figure 3. FMD in 3 different age groups and different genders.
Supplementary Figure 4. FMD in 4 different age groups and different genders.
Supplementary Figure 5. A figure (example captured in one patient of the study sample) of a FMD acquisition.
References
1. Gallino A, Aboyans V, Diehm F C, Cosentino Stricker H, Falk E, et al. Non-coronary atherosclerosis. Eur Heart J. (2014) 35:1112–19. doi: 10.1093/eurheartj/ehu071
2. Kruk ME, Gage AD, Joseph NT, Danaei G, García-Saisó S, Salomon JA. Mortality due to low-quality health systems in the universal health coverage era: a systematic analysis of amenable deaths in 137 countries [published correction appears in Lancet. 2018 Sep 20]. Lancet. (2018) 392:2203–12. doi: 10.1016/S0140-6736(18)31668-4
3. Balta S. Endothelial Dysfunction and Inflammatory Markers of Vascular Disease. Curr Vasc Pharmacol. (2021) 19:243–9. doi: 10.2174/1570161118666200421142542
4. Higashi Y. Assessment of endothelial function. History, methodological aspects, and clinical perspectives. Int Heart J. (2015) 56:125–34. doi: 10.1536/ihj.14-385
5. Polak JF, O'Leary DH. Carotid Intima-Media Thickness as Surrogate for and Predictor of CVD. Glob Heart. (2016) 11:295–312. doi: 10.1016/j.gheart.2016.08.006
6. Kishimoto S, Matsumoto T, Maruhashi T, Iwamoto Y, Kajikawa M, Oda N, et al. Reactive hyperemia-peripheral arterial tonometry is useful for assessment of not only endothelial function but also stenosis of the digital artery. Int J Cardiol. (2018) 260:178–183. doi: 10.1016/j.ijcard.2017.10.069
7. Rodriguez-Miguelez P, Seigler N, Harris RA. Ultrasound assessment of endothelial function: a technical guideline of the flow-mediated dilation test. J Vis Exp. (2016) 110:54011. doi: 10.3791/54011
8. Williams B, Mancia G, Spiering W, Agabiti Rosei E, Azizi M, Burnier M, et al. 2018 ESC/ESH Guidelines for the management of arterial hypertension [published correction appears in Eur Heart J. 2019 Feb 1;40(5):475]. Eur Heart J. (2018) 39:3021–104. doi: 10.1093/eurheartj/ehy339
9. American Diabetes Association. 2. Classification and Diagnosis of Diabetes: Standards of Medical Care in Diabetes-2019. Diabetes Care. (2019) 42:S13–S28. doi: 10.2337/dc19-S002
10. D'Agostino RB Sr, Vasan RS, Pencina MJ, Wolf PA, Cobain M, Massaro JM, et al. General cardiovascular risk profile for use in primary care: the Framingham Heart Study. Circulation. (2008) 117:743–53. doi: 10.1161/CIRCULATIONAHA.107.699579
11. Piepoli MF, Hoes AW, Agewall S, Albus C, Brotons C, Catapano AL, et al. 2016 European Guidelines on cardiovascular disease prevention in clinical practice: The Sixth Joint Task Force of the European Society of Cardiology and Other Societies on Cardiovascular Disease Prevention in Clinical Practice (constituted by representatives of 10 societies and by invited experts) Developed with the special contribution of the European Association for Cardiovascular Prevention & Rehabilitation (EACPR). Eur Heart J. (2016) 37:2315–81. doi: 10.1093/eurheartj/ehw106
12. Andrus B, Lacaille D. 2013 ACC/AHA guideline on the assessment of cardiovascular risk. J Am Coll Cardiol. (2014) 63:2886. doi: 10.1016/j.jacc.2014.02.606
13. Hhijssen DHJ, Bruno RM, van Mil ACCM, Holder SM, Faita F, Greyling A, et al. Expert consensus and evidence-based recommendations for the assessment of flow-mediated dilation in humans. Eur Heart J. (2019) 40:2534–47. doi: 10.1093/eurheartj/ehz350
14. Areas GPT, Mazzuco A, Caruso FR, Jaenisch RB, Cabiddu R, Phillips SA, et al. Flow-mediated dilation and heart failure: a review with implications to physical rehabilitation. Heart Fail Rev. (2019) 24:69–80. doi: 10.1007/s10741-018-9719-7
15. Maruhashi T, Soga J, Fujimura N, Idei N, Mikami S, Iwamoto Y, et al. Endothelial dysfunction, increased arterial stiffness, and cardiovascular risk prediction in patients with coronary artery disease: FMD-J (Flow-Mediated Dilation Japan) Study A. J Am Heart Assoc. (2018) 7:e008588. doi: 10.1161/JAHA.118.008588
16. Brar V, Gill S, Cardillo C, Tesauro M, Panza JA, Campia U. Sex-specific effects of cardiovascular risk factors on endothelium-dependent dilation and endothelin activity in middle-aged women and men. PLoS ONE. (2015) 10:e0121810. doi: 10.1371/journal.pone.0121810
17. Writing Group Members, Mozaffarian D, Benjamin EJ, Go AS, Arnett DK, Blaha MJ, et al. Heart Disease and Stroke Statistics-2016 Update: A Report From the American Heart Association [published correction appears in Circulation. 2016 Apr 12;133(15):e599]. Circulation. (2016) 133:e38–e360. doi: 10.1161/CIR.0000000000000350
18. Stanhewicz AE, Wenner MM, Stachenfeld NS. Sex differences in endothelial function important to vascular health and overall cardiovascular disease risk across the lifespan. Am J Physiol Heart Circ Physiol. (2018) 315:H1569–88. doi: 10.1152/ajpheart.00396.2018
19. Shenouda N, Priest SE, Rizzuto VI, MacDonald MJ. Brachial artery endothelial function is stable across a menstrual and oral contraceptive pill cycle but lower in premenopausal women than in age-matched men. Am J Physiol Heart Circ Physiol. (2018) 315:H366–74. doi: 10.1152/ajpheart.00102.2018
20. Jia G, Aroor AR, Jia C, Sowers JR. Endothelial cell senescence in aging-related vascular dysfunction. Biochim Biophys Acta Mol Basis Dis. (2019) 1865:1802–9. doi: 10.1016/j.bbadis.2018.08.008
21. Seals DR, Jablonski KL, Donato AJ. Aging and vascular endothelial function in humans. Clin Sci (Lond). (2011) 120:357–75. doi: 10.1042/CS20100476
22. Moreau KL. Intersection between gonadal function and vascular aging in women. J Appl Physiol (1985). (2018) 125:1881–7. doi: 10.1152/japplphysiol.00117.2018
23. Jin J, Liu Y, Huang L, Tan H. Advances in epigenetic regulation of vascular aging. Rev Cardiovasc Med. (2019) 20:19–25. doi: 10.31083/j.rcm.2019.01.3189
24. Suboc TM, Dharmashankar K, Wang J, Ying R, Couillard A, Tanner MJ, et al. Moderate obesity and endothelial dysfunction in humans: influence of gender and systemic inflammation. Physiol Rep. (2013) 1:e00058. doi: 10.1002/phy2.58
25. Sönmez HE, Canpolat N, Agbaş A, et al. The Relationship between the Waist Circumference and Increased Carotid Intima Thickness in Obese Children. Child Obes. (2019) 15:468–75. doi: 10.1089/chi.2019.0022
26. Skilton MR, Yeo SQ, Ne JY, Celermajer DS, Caterson ID, Lee CM. Weight loss and carotid intima-media thickness-a meta-analysis. Obesity (Silver Spring). (2017) 25:357–62. doi: 10.1002/oby.21732
27. Yang X, Li J, Hu D, Chen J, Li Y, Huang J, et al. Predicting the 10-Year Risks of Atherosclerotic Cardiovascular Disease in Chinese Population: The China-PAR Project (Prediction for ASCVD Risk in China). Circulation. (2016) 134:1430–40. doi: 10.1161/CIRCULATIONAHA.116.022367
28. Hippisley-Cox J, Coupland C, Brindle P. Development and validation of QRISK3 risk prediction algorithms to estimate future risk of cardiovascular disease: prospective cohort study. BMJ. (2017) 357:j2099. doi: 10.1136/bmj.j2099
29. Atawia RT, Bunch KL, Toque HA, Caldwell RB, Caldwell RW. Mechanisms of obesity-induced metabolic and vascular dysfunctions. Front Biosci (Landmark Ed). (2019) 24:890–934. doi: 10.2741/4758
30. Reilly SM, Saltiel AR. Adapting to obesity with adipose tissue inflammation. Nat Rev Endocrinol. (2017) 13:633–43. doi: 10.1038/nrendo.2017.90
31. Virdis A, Duranti E, Rossi C, Dell'Agnello U, Santini E, Anselmino M, et al. Tumour necrosis factor-alpha participates on the endothelin-1/nitric oxide imbalance in small arteries from obese patients: role of perivascular adipose tissue. Eur Heart J. (2015) 36:784–94. doi: 10.1093/eurheartj/ehu072
32. Lee J, Lee S, Zhang H, Hill MA, Zhang C, Park Y. Interaction of IL-6 and TNF-α contributes to endothelial dysfunction in type 2 diabetic mouse hearts. PLoS ONE. (2017) 12:e0187189. doi: 10.1371/journal.pone.0187189
33. Bianconi V, Sahebkar A, Atkin SL, Pirro M. The regulation and importance of monocyte chemoattractant protein-1. Curr Opin Hematol. (2018) 25:44–51. doi: 10.1097/MOH.0000000000000389
34. Yuan T, Yang T, Chen H, Fu D, Hu Y, Wang J, et al. New insights into oxidative stress and inflammation during diabetes mellitus-accelerated atherosclerosis. Redox Biol. (2019) 20:247–60. doi: 10.1016/j.redox.2018.09.025
35. Valencia-Morales Mdel P, Zaina S, Heyn H, Carmona FJ, Varol N, Sayols S, et al. The DNA methylation drift of the atherosclerotic aorta increases with lesion progression. BMC Med Genomics. (2015) 8:7. doi: 10.1186/s12920-015-0085-1
36. Leite AR, Borges-Canha M, Cardoso R, Neves JS, Castro-Ferreira R, Leite-Moreira A. Novel Biomarkers for Evaluation of Endothelial Dysfunction. Angiology. (2020) 71:397–410. doi: 10.1177/0003319720903586
37. Dimassi S, Chahed K, Boumiza S, Canault M, Tabka Z, Laurant P, et al. Role of eNOS- and NOX-containing microparticles in endothelial dysfunction in patients with obesity. Obesity (Silver Spring). (2016) 24:1305–12. doi: 10.1002/oby.21508
38. Chen GC, Arthur R, Iyengar NM, Kamensky V, Xue X, Wassertheil-Smoller S, et al. Association between regional body fat and cardiovascular disease risk among postmenopausal women with normal body mass index. Eur Heart J. (2019) 40:2849–55. doi: 10.1093/eurheartj/ehz391
39. Stefan N, Schick F, Häring HU. Causes, Characteristics, and Consequences of Metabolically Unhealthy Normal Weight in Humans. Cell Metab. (2017) 26:292–300. doi: 10.1016/j.cmet.2017.07.008
40. Shi J, Yang Z, Niu Y, Zhang W, Lin N, Li X, et al. Large thigh circumference is associated with lower blood pressure in overweight and obese individuals: a community-based study. Endocr Connect. (2020) 9:271–8. doi: 10.1530/EC-19-0539
41. Perticone M, Maio R, Caroleo B, Sciacqua A, Suraci E, Gigliotti S, et al. Serum γ-Glutamyltransferase Concentration Predicts Endothelial Dysfunction in Naïve Hypertensive Patients. Biomedicines. (2020) 8:207. doi: 10.3390/biomedicines8070207
42. Rehm M, Bruegger D, Christ F, Conzen P, Thiel M, Jacob M, et al. Shedding of the endothelial glycocalyx in patients undergoing major vascular surgery with global and regional ischemia. Circulation. (2007) 116:1896–906. doi: 10.1161/CIRCULATIONAHA.106.684852
43. Oda K, Okada H, Suzuki A, Tomita H, Kobayashi R, Sumi K, et al. Factors Enhancing Serum Syndecan-1 Concentrations: A Large-Scale Comprehensive Medical Examination. J Clin Med. (2019) 8:1320. doi: 10.3390/jcm8091320
44. Le Master E, Levitan I. Endothelial stiffening in dyslipidemia. Aging (Albany NY). (2019) 11:299–300. doi: 10.18632/aging.101778
45. Helkin A, Stein JJ, Lin S, Siddiqui S, Maier KG, Gahtan V. Dyslipidemia Part 1–Review of Lipid Metabolism and Vascular Cell Physiology. Vasc Endovascular Surg. (2016) 50:107–18. doi: 10.1177/1538574416628654
46. Hurtubise J, McLellan K, Durr K, Onasanya O, Nwabuko D, Ndisang JF. The Different Facets of Dyslipidemia and Hypertension in Atherosclerosis. Curr Atheroscler Rep. (2016) 18:82. doi: 10.1007/s11883-016-0632-z
47. Elitok A, Emet S, Bayramov F, Karaayvaz E, Türker F, Barbaros U, et al. Effect of bariatric surgery on flow-mediated dilation and carotid intima-media thickness in patients with morbid obesity: 1-year follow-up study. Anatol J Cardiol. (2020) 23:218–22. doi: 10.14744/AnatolJCardiol.2019.85249
48. Sanches E, Topal B, Proczko M, Stepaniak PS, Severin R, Philips SA, et al. Endothelial function in obesity and effects of bariatric and metabolic surgery. Expert Rev Cardiovasc Ther. (2020) 18:343–53. doi: 10.1080/14779072.2020.1767594
49. Wang Y, Xu D. Effects of aerobic exercise on lipids and lipoproteins. Lipids Health Dis. (2017) 16:132. doi: 10.1186/s12944-017-0515-5
50. He N, Ye H. Exercise and hyperlipidemia. Adv Exp Med Biol. (2020) 1228:79–90. doi: 10.1007/978-981-15-1792-1_5
51. Rossman MJ, Kaplon RE, Hill SD, McNamara MN, Santos-Parker JR, Pierce GL, et al. Endothelial cell senescence with aging in healthy humans: prevention by habitual exercise and relation to vascular endothelial function. Am J Physiol Heart Circ Physiol. (2017) 313:H890–H895. doi: 10.1152/ajpheart.00416.2017
52. Rossman MJ, Santos-Parker JR, Steward CAC, Bispham NZ, Cuevas LM, Rosenberg HL, et al. Chronic Supplementation With a Mitochondrial Antioxidant (MitoQ) Improves Vascular Function in Healthy Older Adults. Hypertension. (2018) 71:1056–63. doi: 10.1161/HYPERTENSIONAHA.117.10787
53. Lakshmanan S, Shekar C, Kinninger A, Birudaraju D, Dahal S, Onuegbu A, et al. Association of flow mediated vasodilation and burden of subclinical atherosclerosis by coronary CTA. Atherosclerosis. (2020) 302:15–9. doi: 10.1016/j.atherosclerosis.2020.04.009
54. Matsuzawa Y, Kwon TG, Lennon RJ, Lerman LO, Lerman A. Prognostic value of flow-mediated vasodilation in brachial artery and fingertip artery for cardiovascular events: a systematic review and meta-analysis. J Am Heart Assoc. (2015) 4:e002270. doi: 10.1161/JAHA.115.002270
Keywords: flow-mediated dilation, prediction equation, vascular endothelial function, atherosclerosis, body mass index
Citation: Li X, Liu H, Zhang Y, Gu Y, Sun L, Yu H and Bai W (2022) A Prediction Equation to Estimate Vascular Endothelial Function in Different Body Mass Index Populations. Front. Cardiovasc. Med. 9:766565. doi: 10.3389/fcvm.2022.766565
Received: 29 August 2021; Accepted: 14 February 2022;
Published: 10 March 2022.
Edited by:
Yun Fang, University of Chicago, United StatesReviewed by:
Jessica Faulkner, Augusta University, United StatesTelmo Pereira, Fisiologia Clínica, Portugal
Copyright © 2022 Li, Liu, Zhang, Gu, Sun, Yu and Bai. This is an open-access article distributed under the terms of the Creative Commons Attribution License (CC BY). The use, distribution or reproduction in other forums is permitted, provided the original author(s) and the copyright owner(s) are credited and that the original publication in this journal is cited, in accordance with accepted academic practice. No use, distribution or reproduction is permitted which does not comply with these terms.
*Correspondence: Haoyong Yu, WXVoYW95b25nMTExJiN4MDAwNDA7MTYzLmNvbQ==; Wenkun Bai, ZG9jdG9yNTA1JiN4MDAwNDA7aG90bWFpbC5jb20=
†These authors share first authorship