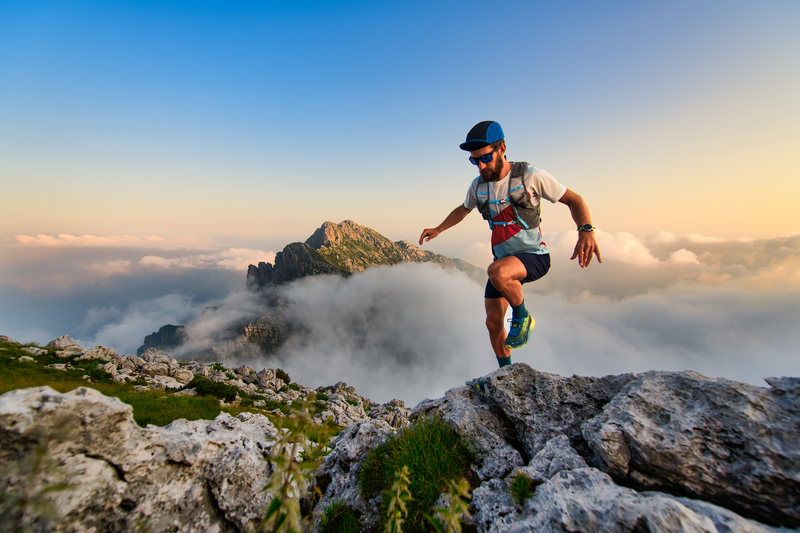
95% of researchers rate our articles as excellent or good
Learn more about the work of our research integrity team to safeguard the quality of each article we publish.
Find out more
ORIGINAL RESEARCH article
Front. Cardiovasc. Med. , 18 November 2022
Sec. Atherosclerosis and Vascular Medicine
Volume 9 - 2022 | https://doi.org/10.3389/fcvm.2022.1071533
This article is part of the Research Topic Lipids and Inflammation in Health and Disease, Volume II View all 11 articles
Objective: This study aimed to evaluate the potential mechanism by which Monocyte locomotion inhibitory factor (MLIF) improves the outcome of ischemic stroke (IS) inflammatory injury.
Methods: Potential MLIF-related targets were predicted using Swiss TargetPrediction and PharmMapper, while IS-related targets were found from GeneCards, PharmGKB, and Therapeutic Target Database (TTD). After obtaining the intersection from these two datasets, the Search Tool for Retrieval of Interacting Genes/Protein (STRING11.0) database was used to analyze the protein-protein interaction (PPI) network of the intersection and candidate genes for MLIF treatment of IS. The candidate genes were imported into the Metascape database for Gene Ontology (GO) functional analysis and Kyoto Encyclopedia of Genes and Genomes (KEGG) pathway enrichment. The top 20 core genes and the “MLIF-target-pathway” network were mapped using the Cytoscape3.9.1. Using AutoDock Vina1.1.2, the molecular docking validation of the hub targets and MLIF was carried out. In the experimental part, transient middle cerebral artery occlusion (tMCAO) and oxygen and glucose deprivation (OGD) models were used to evaluate the protective efficacy of MLIF and the expression of inflammatory cytokines and the putative targets.
Results: MLIF was expected to have an effect on 370 targets. When these targets were intersected with 1,289 targets for ischemic stroke, 119 candidate therapeutic targets were found. The key enriched pathways were PI3K-Akt signaling pathway and MAPK signaling pathway, etc. The GO analysis yielded 1,677 GO entries (P < 0.01), such as hormone stimulation, inflammatory response, etc. The top 20 core genes included AKT1, EGFR, IGF1, MAPK1, MAPK10, MAPK14, etc. The result of molecular docking demonstrated that MLIF had the strong binding capability to JNK (MAPK10). The in vitro and in vivo studies also confirmed that MLIF protected against IS by lowering JNK (MAPK10) and AP-1 levels and decreasing pro-inflammatory cytokines (IL-1, IL-6).
Conclusion: MLIF may exert a cerebral protective effect by inhibiting the inflammatory response through suppressing the JNK/AP-1 signaling pathway.
Stroke is a group of acute and serious cerebral vascular diseases with a high incidence, disability, death, and recurrence rate (1). More than 80% of all conditions result in ischemic stroke (IS), which is triggered by arterial blockage (2). However, the mechanisms involved in the stroke process are complex, such as excitotoxicity, calcium overload, oxidative stress, inflammatory and apoptosis (3, 4). Dyslipidemia is an important risk factor for IS and contributes to IS by several of these mechanisms (5). Elevated blood lipid levels can cause oxidative stress by increasing the production of excess oxygen free radicals, which can alter the arterial and microcirculatory systems (6). Thrombolysis is the most effective treatment for stroke worldwide, although its therapeutic efficacy is greatly controlled by time, and the prognosis is dismal because the majority of patients incur various degrees of neurological impairment after treatment (7). In summary, it is crucial to create effective neuroprotective medications in order to reduce brain damage in stroke patients and improve their prognosis.
Entamoeba histolytica produces a pentapeptide known as monocyte locomotion inhibitory factor (MLIF), which has blatant anti-inflammatory properties (8), such as inhibition of human monocyte motility and immune responses of monocytes and neutrophils, increase of anti-inflammatory cytokines (9), downregulation of LPS-induced IL-1β in U-937 cells (10), and inflammation and matrix metalloproteinases in a collagen-induced arthritis (CIA) mouse model. According to our findings (11), the amount of cerebral ischemia in the temporary middle cerebral artery occlusion (tMCAO) model is greatly decreased by MLIF. Currently, as an experimental novel medication with potential for neuroprotection in acute ischemic stroke, MLIF has been approved (11), but the exact mechanism is unknown.
The network pharmacology approach combines high-throughput data incorporation, metadata search, data analysis, target prediction, and simulation labs to independently present the “drug-target-pathway” associated with a particular disease and to show the connections and interactions between drugs and targets from a systematic perspective (12). It provides a novel tool for innovative drug discovery (13). The process of “molecular docking” involves interacting a drug molecule with a receptor to design new drugs (14), which has become frequently employed in drug discovery (15). Therefore, this study used Swiss TargetPrediction, PharmMapper databases to forecast the MLIF-related targets, and GeneGards, PharmGKB, and Therapeutic Target Database (TTD) to search for targets of IS. After obtaining common targets, the protein-protein interaction (PPI) network, Gene Ontology (GO) functional analysis, and pathway enrichment from the Kyoto Encyclopedia of Genes and Genomes (KEGG) were performed using online analytic platforms like STRING11.0, Metascape, and combined with the cytoHubba plug-in in Cytoscape3.9.1 to extract the top20 core genes. Finally, molecular docking was performed by AutoDock Vina1.1.2. The probable targets of MLIF were further confirmed by tests based on the findings of network pharmacology analysis and molecular docking analysis. From the perspective of network pharmacology, the scientific connotation of the anti-inflammatory effect of MLIF in stroke was elucidated in order to serve as a reference for its use in treating post-stroke neurological damage and to lay the foundation for further studies on its mechanism of action (the flow of the study is shown in Figure 1).
The MLIF structural data was retrieved from the PubChem database (https://pubchem.ncbi.nlm.nih.gov/) (16), and the MLIF-related targets were predicted in PharmMapper (http://www.lilab-ecust.cn/pharmmapper/) and the Swiss TargetPrediction databases (http://www.swisstargetprediction.ch/) (17, 18), respectively. To create a collection of MLIF-related targets, the targets from the two databases were combined and standardized with the Uniprot database (https://www.uniprot.org/) (19). The IS-related targets were looked for in GeneCards (https://www.genecards.org/) (20), PharmGKB (https://www.pharmgkb.org/) (21) and TTD (http://db.idrblab.net/ttd/) (22) databases using “ischemic stroke” and “cerebral ischemia” as the keywords, respectively.
The intersection of MLIF-related targets and the IS-related targets datasets was obtained by Venny diagram. The STRING11.0 database (https://cn.string-db.org/) was updated with the integrated target data to obtain the protein-protein interaction (PPI) network and the species must be restricted to “Homo sapiens,” with the confidence score of at least 0.9 (23). The PPI network was made visible by using Cytoscape 3.9.1 (https://cytoscape.org/) (24) and topology analysis was performed using the CytoHubba plug-in to obtain the top 20 targets in terms of degree as the core genes (25).
The Metascape database (https://metascape.org/gp/index.html#/main/step1) was used to import the targets of MLIF treatment IS for Kyoto Encyclopedia of Genes and Genomes (KEGG) pathway enrichment and Gene Ontology (GO) functional analysis with P < 0.01 (26).
The “MLIF-target-pathway” network was created using the data from the aforementioned procedures and Cytoscape 3.9.1 software (https://cytoscape.org/) (24), allowing us to see how each node interacts with the active substance (MLIF), potential targets, and enriched KEGG pathways.
The 3D structures of target proteins were obtained from the RCSB protein database (https://www.rcsb.org/) (27), and the 2D structure of MLIF was obtained using the PubChem database (https://pubchem.ncbi.nlm.nih.gov/), drawn in 3D using ChemDraw20 (https://www.chemdraw.com.cn/) (28) and converted to pdb file format using OpenBabel 3.1.1 (http://openbabel.org/wiki/Main_Page) (29). MLIF was set as a ligand and the hub targets were set as receptors. In order to delete water molecules and heteroatoms and add charge and hydrogen atoms, the drug and receptor proteins were added to PyMOL 2.4 (https://pymol.org/2/) and AutoDock 4.2.6 (https://autodock.scripps.edu/). Using AutoDock Vina 1.1.2 (https://autodock.scripps.edu/), the produced medication was molecularly docked to the target protein. PyMOL 2.4 was used to visualize the final conformation, which was chosen based on its best binding energy (30).
The rats were kept in a controlled setting with a 12-h cycle of light and dark and were fed a conventional rat diet along with water. The Institutional Animal Care and Use Committee at Shanghai University oversaw all animal studies and surgical operations to ensure that they adhered to international standards for the humane use of experimental animals. Male Sprague-Dawley rats weighing between 250 and 280 g were randomly assigned to one of three groups (N = 3–6): the Sham group (Sham), the MCAO group (MCAO), and the MLIF group (MLIF). Five minutes before reperfusion, 1 mg/kg of MLIF was injected into the tail vein for the MLIF group. Both the Sham group and the MCAO group received the identical dosage of normal saline by tail intravenous injection at the same time.
MLIF was created by the Chinese Peptide Company (Hangzhou, China), with a purity level of above 98% and in accordance with the recognized amino acid sequence (Met-Gln-Cys-Asn-Ser). 2,3,5-triphenyl tetrazolium chloride (TTC) was provided by Jiancheng Biotech Co. (Nanjing, China). The peptide was dissolved to its final concentration (50 g/ml) in PBS (pH 7.4) before being employed for cell therapy. Hyclone (Logan, Utah, USA) provided high/low glucose versions of Dulbecco's modified Eagle's medium (DMEM). Fetal bovine serum (FBS) and 0.25% tryptase were purchased from Gibco (Carlsbad, CA, USA). Penicillin-streptomycin solution was purchased from Biosera (France). R&D (Minneapolis, MN, USA) provided the enzyme-linked immunosorbent assay (ELISA) kits for IL-1 and IL-6, and Takara (Shiga, Japan) provided the kits for total RNA. All the other chemicals were bought from businesses.
Brain microvascular endothelial cells (bEnd3 cells) were purchased from the American Type Culture Collection (ATCC). The DMEM with high glucose, 10% fetal bovine serum, and 100 g/ml penicillin-streptomycin solution was used to cultivate the bEnd3 cells. At 37°C, cells were grown in 5% CO2 and 95% air.
Procedures for tMCAO were previously described (31). In a nutshell, pentobarbitone (350 mg/kg, i.p.) was used to anesthetize rats during surgery. The internal carotid artery was used to insert an 11-mm silicone-coated 8–0 filament into the left common carotid artery for 2 h. The filament was then gently removed to complete a 24-h reperfusion period. A heating lamp was used to keep the subject's body temperature at 37.5 ± 0.5°C. After reperfusion for 24 h, we assessed the efficacy of MLIF by TTC staining and neurological deficit score and measured pro-inflammatory cytokines, including IL-1 and IL-6, in the ischemic penumbra.
According to a prior description by Yang et al., an ischemic model was created in vitro by depriving cells of oxygen and glucose (32). The bEnd3 cells was planted in cell culture plates with 5% CO2 at 37°C at a density of 3 × 105 cells/ml. When the confluency reached to 80%, the pure culture was quickly changed to low glucose DMEM without FBS and quinolone solution, The cells were then starved for 2 h in a 95% atmosphere, 5% carbon dioxide atmosphere at 37°C. The OGD insult was administered after MLIF (50 g/ml) administration, and the cells were placed under hypoxic conditions (5% CO2 and 95% N2) for various time periods (0, 2, 4, 6, and 8 h) in different experiments. The ELISA assay and Quantitative real-time PCR (qRT-PCR) were performed after OGD to detect the inflammatory cytokines (IL-1 and IL-6).
We used Longa's approach to calculate the neurological deficit score for rats (0 = no neurological deficit, 1 = unable to extend left front paw, 2 = rats' crawling motion circled to the left, 3 = rats stood with a left-leaning posture, 4 = rats lost consciousness and were unable to walk on its own) (33).
Each group's brains were taken out and preserved for 15 min at −20°C. The brains were divided into six sections, each about 2 mm thick. The sections were immersed in 1% 2,3,5-triphenyl-tetrazolium chloride (TTC) for 30 min at 37°C. Sections were rotated every 5 min and then washed three times with ddH2O. Images were gathered for additional examination.
According to the instructions provided by the kit's manufacturer (R&D), the ELISA was used to measure the amounts of inflammatory cytokines (IL-1 and IL-6) in the hydrolysates of ischemic brain tissue and the effluent of the bEnd3 cells. The reactions were placed in ELISA plates, and the results were examined at a wave length of 450 nm. The standard curve was used to determine the concentrations of IL-1 and IL-6.
To evaluate the RNA levels of IL-1, IL-6, JNK, and AP-1, qRT-PCR was utilized. Total RNA was extracted from brain tissues and bEnd3 cells using the Total RNA Kit (Takara, Shiga, Japan). According to the manufacturer's instructions, cDNAs were created using 5 × Primescript reverse transcription reagents (Takara, Shiga, Japan). Table 1 displayed the primers being used in quantitative RT-PCR (qRT-PCR). qRT-PCR was carried out using SYBR Premix Ex TaqTM (Tli RnaseH Plus) (Takara, Shiga, Japan) on 7500 Real-Time PCR System (Applied Biosystems). The level of expression for each target genes was normalized to the control (GAPDH).
Every piece of data was reported as mean±SD. Using one-way analysis of variance (ANOVA), statistical analysis was carried out. The Student's t-test was applied to compare the two groups. Statistics were judged significant at P < 0.05. With GraphPad Prism 9.0 (SPSS Inc., United States), all statistical analysis was completed.
The MLIF 2D structure from the pubchem database (CID: 10325914 Figure 2A), MLIF-related targets prediction was performed using the Swiss TargetPrediction platform and PharmMapper databases, and 370 action objectives in total were found after de-duplication screening (Supplementary Table 1). After deleting entries with duplicate information from the GeneCards, PharmGKB, and TTD databases, 1,289 targets associated to IS were found.
The MLIF-related targets and IS-related targets were intersected to obtain 156 common targets between drug and disease (Figure 2B). The 156 common targets were imported into the STRING11.0 database, and targets with the confidence score > 0.9 were screened out, and isolated targets were excluded to obtain a PPI network containing 119 targets. It is suggested that these 119 shared targets may be candidate targets for MLIF treatment of IS. The PPI network was shown in Figure 3, where each edge represented a protein interaction and each node represented a target. The size of the nodes indicates the degree's magnitude. The network diagram demonstrates the intimate ties between the targets, demonstrating the complexity and diversity of the MLIF for the treatment of IS and the synergy between various targets. The top 20 core genes were determined using Cytoscape 3.9.1's cytoHubba plug-in, and they were shown in Figure 4. The node color and score are correlated, with darker and more red nodes signifying higher scores.
The GO analysis by Metascape database yielded 1,677 GO entries (P < 0.01), including 1,437 entries for biological process (BP), such as positive regulation of cell migration, hormone stimulation, positive regulation of cellular component movement, etc, 97 entries for cellular component (CC), such as cell membrane, cytoplasm, cytosol, nucleus, etc., 143 entries for molecular function (MF), such as endopeptidase activity, peptidase activity, protein kinase activity, etc. For visualization, the best 10 submissions for each category were selected and shown in Figure 5. The KEGG pathway enrichment of 119 candidate targets yielded 181 signaling pathways with P < 0.01, mainly involving neural, vascular, inflammation, oxidative stress, apoptosis and lipid metabolism. Ten pathways with more significant gene ratio in the enrichment results were selected for display (Figure 6), those related to other diseases including cancer signaling pathways, human papillomavirus infection, human cytomegalovirus infection, lipids and atherosclerosis. And those related to apoptosis: PI3K-Akt signaling pathway, RAS signaling pathway, two related to adhesion: Rap1 signaling pathways and Focal adhesion. The inflammation-related pathways: PI3K-Akt signaling pathway and mitogen-activated protein kinase (MAPK) signaling pathway. A total of 23 targets were involved in the MAPK signaling pathway (Table 2). The “MLIF -target-pathway” network was further built using nodes of various colors and shapes to represent various active compound, targets, and pathways. One compound (red V-shape), 119 targets (blue circles), and 10 KEGG pathways (green triangles) are among the nodes that represent active compounds, targets, and pathways (Figure 7).
Figure 7. MLIF-target-pathway network. MLIF, targets, and KEGG pathways are represented by the red V shape, blue circles, and green triangle circles, respectively.
Based on the results of KEGG analysis and top20 core genes analysis, AKT1 (PDB ID: 6s9w), EGFR (PDB ID:5Y9T), ERBB2 (PDB ID:2a91), HRAS (PDB ID:5P21), IGF1 (PDB ID:1b9g), IGF1R (PDB ID:6PYH), KDR/VEGFR2 (PDB ID:1y6a) MAPK1/ERK (PDB ID:6G54), MAPK10/JNK (PDB ID:7S1N), MAPK14/P38 (PDB ID:5ETI) were selected as the hub targets (Figure 8). As shown in Table 3, MLIF was selected as the ligand and the hub targets were selected as receptors. There's a strong possibility that MLIF binds well to the JNK receptor (≤ -7.0 kcal/mol) (Figure 9), a protein corresponding to MAPK10, and this indicates that MLIF has good binding potential to key targets of the JNK signaling pathway.
Figure 9. 3D docking conformation of MLIF with JNK (MAPK10). The orange stick in the diagram is a small molecule (MLIF), the blue cartoon is a protein (JNK), the yellow dashed line indicates hydrogen bonding and the gray dashed line indicates hydrophobic interactions.
The neurological deficit score of the MCAO group was considerably higher than that of the Sham group, as can be seen in Figure 10A (P < 0.01). Although the MLIF group's neurological deficit score was lower than the MCAO group's, there was no discernible difference between the two. The focal infarct volume was also measured 24 h following reperfusion. As shown in Figure 10B, the percentage of infarct volume in the MCAO group was considerably higher than that in the Sham group (P < 0.01), and MLIF significantly decreased the percentage of infarct volume in the MCAO group (P < 0.01).
Figure 10. MLIF played a protective role against neurological damage on the MCAO rats. Neurological deficit score (A) and infarct volume statistical results (B) of brain tissue in rats. (N = 6, ##P < 0.01 vs. Sham group, **P < 0.01 vs. MCAO group).
The expansion of the area with brain injury brought on by the release of pro-inflammatory cytokines into the extracellular space raises the neurological deficit and has a detrimental effect on the prognosis for survival (34). IL-1 receptor antagonist (IL-1Ra) administration significantly reduced peripheral markers of inflammation in patients with ischemic stroke (35, 36). We determined the effect of MLIF on cytokines which promote inflammation (IL-1 and IL-6) expression in the pathological process of brain ischemia by qRT-PCR and ELISA analysis in vivo and in vitro, respectively. We found that MLIF (1 mg/kg) could reduce the relative IL-1 and IL-6 mRNA expression (P < 0.05) in the MCAO rats (Figures 11A,B). It also showed a significant reduction of IL-1 (P < 0.01) and IL-6 (P < 0.05) in the MCAO rats (Figures 11C,D). In this in vitro study, we used the OGD model and found that the relative mRNA expression of IL-1 and IL-6 were significantly upregulated at the different time (2, 4, 6, and 8 h) after OGD (Figures 11E,G). Administration of MLIF (50 μg/ml) remarkably reduced the expression of IL-1 compared with the OGD model group (2, 4, 6, and 8 h) (P < 0.01). As for IL-6, MLIF has the similar effect at 2 h (P < 0.05),4 h, 6 h, and 8 h (P < 0.01) after OGD insult. Moreover, we also assessed protein expression of IL-1 and IL-6 in bEnd3 cells supernatant after OGD by ELISA. As shown in Figures 11F,H, MLIF significantly decreased the level of IL-1 (P < 0.05) at 8 h and IL-6 at 4 h (P < 0.05) in bEnd3 cells' supernatant after OGD insult. These results showed the anti-inflammatory effect of MLIF on brain ischemia.
Figure 11. MLIF reduced the expression of the pro-inflammatory cytokines IL-1 and IL-6 in the MCAO rats and bEnd3 cells at the different time after OGD. The relative mRNA expression levels of IL-1 (A,E) and IL-6 (C,G) in the MCAO rats and bEnd3 cells were determined by qRT-PCR. IL-1 (B,F) and IL-6 (D,H) in the rat brain tissue homogenate and OGD-insulted bEnd3 cells supernatant was measured by ELISA assay (N = 3, ##P < 0.01 vs. Sham group, **P < 0.01 vs. MCAO group, *P < 0.05 vs. MCAO group).
Studies have suggested that the JNK pathway's most significant downstream, AP-1 activation, may have a role in the expression of numerous genes linked to cell survival or death in ischemic brain injury (37). So we further measured JNK, AP-1 levels in the rats brain tissue after MCAO and bEnd3 cells during the OGD insult. MLIF (1 mg/kg) could reduce the relative mRNA expression of JNK (P < 0.05) (Figure 12A) and AP-1 (P < 0.001) (Figure 12B) in vivo. Treatment of MLIF (50 μg/ml) resulted in a significant reduction in the transcriptional level of JNK in bEnd3 cells after OGD insult when it was at 8 h (P < 0.05) (Figure 12C). And MLIF (50 μg/ml) could remarkably decreased OGD-induced the gene expression of AP-1 at 2, 4, 6, and 8 h (P < 0.01) (Figure 12D). These findings suggested that MLIF may inhibit inflammatory responses after IS in vivo and in vitro through the JNK/AP-1 signaling pathways.
Figure 12. JNK/AP-1 signaling pathways contributes to the anti-inflammatory effect of MLIF. The relative mRNA levels of JNK (A,C) and AP-1 (B,D) in rat's brain and bEnd3 cells were determined by qRT-PCR at the different time (0, 2, 4, 6, and 8 h) after OGD (N = 3, ###P < 0.001 vs. Sham group, #P < 0.05 vs. Sham group, ***P < 0.001 vs. MCAO group, **P < 0.01 vs. MCAO group, *P < 0.05 vs. MCAO group).
According to some researchers, the heightened inflammatory response may have a role in the development of stroke (38). In general, inflammatory indicators like IL-6 produce cardiovascular remodeling and arterial resistance (39) and increase the incidence of cardiovascular disease (CVD) (40).
Entamoeba Histolytica produces the heat-stable pentapeptide MLIF, which has a variety of anti-inflammatory effects (9, 41, 42), including regulating inflammation and immune responses through the NF-κB and MAPK pathways (43). The anti-inflammatory effect of MLIF makes the its increasing research on brain ischemia (44). Our previous study has proved that MLIF can reduce the risk of brain ischemia injury by focusing on the eEF1A1/eNOS pathway (45).
In recent years, network pharmacology has become frequently employed to forecast novel drug targets and pathways, lessening the blindness of research and improving the efficiency of drug discovery (46). Molecular docking techniques have been used to validate the predictions of network pharmacology by combining components with target proteins in a virtual evaluation (47). The network pharmacology was used in the study to further analyze the possible targets and pathways of MLIF for the treatment of IS and found that MLIF acts on top20 core genes, includingAKT1, EGFR, ERBB2, HRAS, IGF1, MAPK1, MAPK10, MAPK14, etc. The “MLIF-target-pathway” network analysis revealed that the effect of MLIF on IS therapy PI3K/AKT, MAPK, and other signaling pathways were involved. PI3K/AKT signaling pathway is a pro-cell survival pathway that regulates cell proliferation, differentiation, metabolism, and anti-apoptosis (48). This pathway is activated in the early stages of cerebral ischemia, inducing apoptosis and inflammatory response, and its activity is gradually inhibited as ischemia increases (49). The MAPK signaling pathway is crucial for controlling inflammation, neuronal apoptosis, brain edema, and ischemia-reperfusion injury. It also affects the development of stroke (50). Stress-activated protein kinase (SAPK) or Jun amino-terminal kinase (JNK) is a member of the MAPK family and is activated by a range of environmental stresses, inflammatory cytokines, and growth factors. It is crucial for stress reactions like apoptosis and inflammation (51). The JNK pathway is thought to be a marker of neuronal cell death. JNK phosphorylation can result in the transcription of inflammatory mediators like nitric oxide (NO), inducible nitric oxide synthase (iNOS), cyclooxygenase-2 (COX-2), prostaglandin E2 (PGE2), and the pro-inflammatory cytokines IL-6 and IL-1, which in turn can result in neuronal degeneration.
Based on the results of the network pharmacology, the study was conducted to validate the hub targets of the MAPK signaling pathway using molecular docking techniques and vivo/vitro experiments, respectively. And MLIF showed strong binding capacity ( ≤ -7.0 kcal/mol) to JNK (MAPK10). In addition, in vitro and in vivo experiments demonstrated that JNK and AP-1 RNA levels were considerably decreased in the MLIF group, and the transcript and expression of inflammatory factors (IL-1, IL-6) were reduced, further suggesting that MLIF has a therapeutic effect on IS inflammation, possibly by regulating the JNK/AP-1 signaling pathway (52).
The datasets presented in this study can be found in online repositories. The names of the repository/repositories and accession number(s) can be found in the article/Supplementary material.
The animal study was reviewed and approved by the Shanghai University Ethics Committee.
The tests were designed by YZ and JM. Data analysis, figure creation, and manuscript writing were all done by ML. The animal model was built by ML, QZ, XL, SD, and YG. The Western blot and cell tests were carried out by ML. The article's submission was reviewed and approved by all authors.
This research was made possible thanks to grants from the Shanghai University School of Medicine's Innovation Fund for Interdisciplinary New Medical Graduate Students and the National Natural Science Foundation of China (No. 81971017).
The authors declare that the research was conducted in the absence of any commercial or financial relationships that could be construed as a potential conflict of interest.
All claims expressed in this article are solely those of the authors and do not necessarily represent those of their affiliated organizations, or those of the publisher, the editors and the reviewers. Any product that may be evaluated in this article, or claim that may be made by its manufacturer, is not guaranteed or endorsed by the publisher.
The Supplementary Material for this article can be found online at: https://www.frontiersin.org/articles/10.3389/fcvm.2022.1071533/full#supplementary-material
1. Vos T, Lim SS, Abbafati C, Abbas KM, Abbasi M, Abbasifard M, et al. Global Burden of 369 Diseases and Injuries in 204 Countries and Territories, 1990–2019: A Systematic Analysis for the Global Burden of Disease Study 2019. Lancet. (2020) 396:1204–22. doi: 10.1016/S0140-6736(20)30925-9
3. Kelly PJ, Lemmens R, Tsivgoulis G. Inflammation and stroke risk: a new target for prevention. Stroke. (2021) 52:2697–706. doi: 10.1161/STROKEAHA.121.034388
4. Kuriakose D, Xiao Z. Pathophysiology and treatment of stroke: present status and future perspectives. Int J Mol Sci. (2020) 21:7609. doi: 10.3390/ijms21207609
5. Li Z, Zhang J, Luo Y. Impact of triglyceride playing on stroke severity correlated to bilirubin. Medicine (Baltimore). (2020) 99:e21792. doi: 10.1097/MD.0000000000021792
6. Ross R. Atherosclerosis–an Inflammatory disease. N Engl J Med. (1999) 340:115–26. doi: 10.1056/NEJM199901143400207
7. Ernst E, Matrai A, Paulsen F. Leukocyte rheology in recent stroke. Stroke. (1987) 18:59–62. doi: 10.1161/01.STR.18.1.59
8. Utrera-Barillas D, Velazquez JR, Enciso A, Cruz SM, Rico G, Curiel-Quesada E, et al. An Anti-inflammatory oligopeptide produced by entamoeba histolytica down-regulates the expression of pro-inflammatory chemokines. Parasite Immunol. (2003) 25:475–82. doi: 10.1111/j.1365-3024.2003.00657.x
9. Rojas-Dotor S, Araujo-Monsalvo VM, Sanchez-Rojas MJ, Dominguez-Hernandez VM. The monocyte locomotion inhibitory factor inhibits the expression of inflammation-induced cytokines following experimental contusion in rat tibia. Scand J Immunol. (2018) 88:e12702. doi: 10.1111/sji.12702
10. Hayward JA, Mathur A, Ngo C, Man SM. Cytosolic recognition of microbes and pathogens: inflammasomes in action. Microbiol Mol Biol Rev. (2018) 82:e00015–18. doi: 10.1128/MMBR.00015-18
11. Liu X, Hu P, Wang Y, Wang X, Huang J, Li J, et al. A validated Uplc-Ms/Ms Method for the quantitation of an unstable peptide, monocyte locomotion inhibitory factor (Mlif) in human plasma and its application to a pharmacokinetic study. J Pharm Biomed Anal. (2018) 157:75–83. doi: 10.1016/j.jpba.2018.04.009
12. Song X, Zhang Y, Dai E, Du H, Wang L. Mechanism of action of celastrol against rheumatoid arthritis: a network pharmacology analysis. Int Immunopharmacol. (2019) 74:105725. doi: 10.1016/j.intimp.2019.105725
13. Kibble M, Saarinen N, Tang J, Wennerberg K, Makela S, Aittokallio T. Network pharmacology applications to map the unexplored target space and therapeutic potential of natural products. Nat Prod Rep. (2015) 32:1249–66. doi: 10.1039/C5NP00005J
14. Pinzi L, Rastelli G. Molecular docking: shifting paradigms in drug discovery. Int J Mol Sci. (2019) 20:4331. doi: 10.3390/ijms20184331
15. Ferreira LG, Dos Santos RN, Oliva G, Andricopulo AD. Molecular docking and structure-based drug design strategies. Molecules. (2015) 20:13384–421. doi: 10.3390/molecules200713384
16. Li Q, Cheng T, Wang Y, Bryant SH. Pubchem as a public resource for drug discovery. Drug Discov Today. (2010) 15:1052–7. doi: 10.1016/j.drudis.2010.10.003
17. Zoete V, Daina A, Bovigny C, Michielin O. Swisssimilarity: a web tool for low to ultra high throughput ligand-based virtual screening. J Chem Inf Model. (2016) 56:1399–404. doi: 10.1021/acs.jcim.6b00174
18. Wang X, Shen Y, Wang S, Li S, Zhang W, Liu X, et al. Pharmmapper 2017 update: a web server for potential drug target identification with a comprehensive target pharmacophore database. Nucleic Acids Res. (2017) 45:W356–W60. doi: 10.1093/nar/gkx374
19. Zaru R, Magrane M, O'Donovan C, UniProt C. From the research laboratory to the database: the caenorhabditis elegans kinome in uniprotkb. Biochem J. (2017) 474:493–515. doi: 10.1042/BCJ20160991
20. Rebhan M, Chalifa-Caspi V, Prilusky J, Lancet D. Genecards: a novel functional genomics compendium with automated data mining and query reformulation support. Bioinformatics. (1998) 14:656–64. doi: 10.1093/bioinformatics/14.8.656
21. Barbarino JM, Whirl-Carrillo M, Altman RB, Klein TE. Pharmgkb: a worldwide resource for pharmacogenomic information. Wiley Interdiscip Rev Syst Biol Med. (2018) 10:e1417. doi: 10.1002/wsbm.1417
22. Wang Y, Zhang S, Li F, Zhou Y, Zhang Y, Wang Z, et al. Therapeutic target database 2020: enriched resource for facilitating research and early development of targeted therapeutics. Nucleic Acids Res. (2020) 48:D1031–D41. doi: 10.1093/nar/gkz981
23. Szklarczyk D, Gable AL, Nastou KC, Lyon D, Kirsch R, Pyysalo S, et al. The string database in 2021: customizable protein-protein networks, and functional characterization of user-uploaded gene/measurement sets. Nucleic Acids Res. (2021) 49:D605–D12. doi: 10.1093/nar/gkaa1074
24. Shannon P, Markiel A, Ozier O, Baliga NS, Wang JT, Ramage D, et al. Cytoscape: a software environment for integrated models of biomolecular interaction networks. Genome Res. (2003) 13:2498–504. doi: 10.1101/gr.1239303
25. Chin CH, Chen SH, Wu HH, Ho CW, Ko MT, Lin CY. Cytohubba: identifying hub objects and sub-networks from complex interactome. BMC Syst Biol. (2014) 8 Suppl 4:S11. doi: 10.1186/1752-0509-8-S4-S11
26. Zhou Y, Zhou B, Pache L, Chang M, Khodabakhshi AH, Tanaseichuk O, et al. Metascape provides a biologist-oriented resource for the analysis of systems-level datasets. Nat Commun. (2019) 10:1523. doi: 10.1038/s41467-019-09234-6
27. Goodsell DS, Zardecki C, Di Costanzo L, Duarte JM, Hudson BP, Persikova I, et al. Rcsb protein data bank: enabling biomedical research and drug discovery. Protein Sci. (2020) 29:52–65. doi: 10.1002/pro.3730
28. Evans DA. History of the harvard chemdraw project. Angew Chem Int Ed Engl. (2014) 53:11140–5. doi: 10.1002/anie.201405820
29. O'Boyle NM, Banck M, James CA, Morley C, Vandermeersch T, Hutchison GR. Open babel: an open chemical toolbox. J Cheminform. (2011) 3:33. doi: 10.1186/1758-2946-3-33
30. Nguyen NT, Nguyen TH, Pham TNH, Huy NT, Bay MV, Pham MQ, et al. Autodock Vina adopts more accurate binding poses but Autodock4 forms better binding affinity. J Chem Inf Model. (2020) 60:204–11. doi: 10.1021/acs.jcim.9b00778
31. Zhu Q, Zhang Y, Liu Y, Cheng H, Wang J, Zhang Y, et al. Mlif alleviates Sh-Sy5y neuroblastoma injury induced by oxygen-glucose deprivation by targeting eukaryotic translation elongation factor 1a2. PLoS ONE. (2016) 11:e0149965. doi: 10.1371/journal.pone.0149965
32. Chen YJ, Hsu CC, Shiao YJ, Wang HT, Lo YL, Lin AMY. Anti-inflammatory effect of afatinib (an Egfr-Tki) on ogd-induced neuroinflammation. Sci Rep. (2019) 9:2516. doi: 10.1038/s41598-019-38676-7
33. Longa EZ, Weinstein PR, Carlson S, Cummins R. Reversible middle cerebral artery occlusion without craniectomy in rats. Stroke. (1989) 20:84–91. doi: 10.1161/01.STR.20.1.84
34. Malone K, Amu S, Moore AC, Waeber C. The immune system and stroke: from current targets to future therapy. Immunol Cell Biol. (2019) 97:5–16. doi: 10.1111/imcb.12191
35. Levard D, Buendia I, Lanquetin A, Glavan M, Vivien D, Rubio M. Filling the gaps on stroke research: focus on inflammation and immunity. Brain Behav Immun. (2021) 91:649–67. doi: 10.1016/j.bbi.2020.09.025
36. Smith CJ, Hulme S, Vail A, Heal C, Parry-Jones AR, Scarth S, et al. Scil-stroke (Subcutaneous Interleukin-1 Receptor Antagonist in Ischemic Stroke). Stroke. (2018) 49:1210–6. doi: 10.1161/STROKEAHA.118.020750
37. Dong Y, Liu HD, Zhao R, Yang CZ, Chen XQ, Wang XH, et al. Ischemia activates Jnk/C-Jun/Ap-1 pathway to up-Regulate 14-3-3gamma in astrocyte. J Neurochem. (2009) 109 Suppl 1:182–8. doi: 10.1111/j.1471-4159.2009.05974.x
38. Tuttolomondo A, Di Raimondo D, Pecoraro R, Serio A, D'Aguanno G, Pinto A, et al. Immune-inflammatory markers and arterial stiffness indexes in subjects with acute ischemic stroke. Atherosclerosis. (2010) 213:311–8. doi: 10.1016/j.atherosclerosis.2010.08.065
39. Laurent S, Boutouyrie P, Asmar R, Gautier I, Laloux B, Guize L, et al. Aortic stiffness is an independent predictor of all-cause and cardiovascular mortality in hypertensive patients. Hypertension. (2001) 37:1236–41. doi: 10.1161/01.HYP.37.5.1236
40. Guerin AP, Blacher J, Pannier B, Marchais SJ, Safar ME, London GM. Impact of aortic stiffness attenuation on survival of patients in end-stage renal failure. Circulation. (2001) 103:987–92. doi: 10.1161/01.CIR.103.7.987
41. Scheiblecker L, Kollmann K, Sexl V. Cdk4/6 and Mapk-crosstalk as opportunity for cancer treatment. Pharmaceuticals (Basel). (2020) 13:418. doi: 10.3390/ph13120418
42. Godina-Gonzalez S, Furuzawa-Carballeda J, Utrera-Barillas D, Alcocer-Varela J, Teran LM, Vazquez-del Mercado M, et al. Amebic monocyte locomotion inhibitory factor peptide ameliorates inflammation in cia mouse model by downregulation of cell adhesion, inflammation/chemotaxis, and matrix metalloproteinases genes. Inflamm Res. (2010) 59:1041–51. doi: 10.1007/s00011-010-0224-2
43. Silva-Garcia R, Estrada-Garcia I, Ramos-Payan R, Torres-Salazar A, Morales-Martinez ME, Arenas-Aranda D, et al. The effect of an anti-inflammatory pentapeptide produced by entamoeba histolytica on gene expression in the U-937 monocytic cell line. Inflamm Res. (2008) 57:145–50. doi: 10.1007/s00011-007-6199-y
44. Wang X, Wang C, Yang Y, Ni J. New monocyte locomotion inhibitory factor analogs protect against cerebral ischemia-reperfusion injury in rats. Bosn J Basic Med Sci. (2017) 17:221–7. doi: 10.17305/bjbms.2017.1622
45. Zhang Y, Chen J, Li F, Li D, Xiong Q, Lin Y, et al. A pentapeptide monocyte locomotion inhibitory factor protects brain ischemia injury by targeting the Eef1a1/endothelial nitric oxide synthase pathway. Stroke. (2012) 43:2764–73. doi: 10.1161/STROKEAHA.112.657908
46. Zhou Z, Chen B, Chen S, Lin M, Chen Y, Jin S, et al. Applications of network pharmacology in traditional chinese medicine research. Evid Based Complement Alternat Med. (2020) 2020:1646905. doi: 10.1155/2020/1646905
47. Li T, Guo R, Zong Q, Ling G. Application of molecular docking in elaborating molecular mechanisms and interactions of supramolecular cyclodextrin. Carbohydr Polym. (2022) 276:118644. doi: 10.1016/j.carbpol.2021.118644
48. Hou Y, Wang K, Wan W, Cheng Y, Pu X, Ye X. Resveratrol provides neuroprotection by regulating the Jak2/Stat3/Pi3k/Akt/Mtor pathway after stroke in rats. Genes Dis. (2018) 5:245–55. doi: 10.1016/j.gendis.2018.06.001
49. Zhang Q, An R, Tian X, Yang M, Li M, Lou J, et al. Beta-caryophyllene pretreatment alleviates focal cerebral ischemia-reperfusion injury by activating Pi3k/Akt signaling pathway. Neurochem Res. (2017) 42:1459–69. doi: 10.1007/s11064-017-2202-3
50. Alam S, Liu Q, Liu S, Liu Y, Zhang Y, Yang X, et al. Up-regulated cathepsin C induces macrophage M1 polarization through Fak-Triggered P38 Mapk/Nf-Kappab pathway. Exp Cell Res. (2019) 382:111472. doi: 10.1016/j.yexcr.2019.06.017
51. Weston CR, Davis RJ. The Jnk signal transduction pathway. Curr Opin Cell Biol. (2007) 19:142–9. doi: 10.1016/j.ceb.2007.02.001
Keywords: monocyte locomotion inhibitory factor (MLIF), ischemic stroke (IS), network pharmacology, molecular docking, JNK
Citation: Lv M, Zhu Q, Li X, Deng S, Guo Y, Mao J and Zhang Y (2022) Network pharmacology and molecular docking-based analysis of protective mechanism of MLIF in ischemic stroke. Front. Cardiovasc. Med. 9:1071533. doi: 10.3389/fcvm.2022.1071533
Received: 16 October 2022; Accepted: 04 November 2022;
Published: 18 November 2022.
Edited by:
Alexander Nikolaevich Orekhov, Institute for Atherosclerosis Research, RussiaReviewed by:
He-Hui Xie, Shanghai Jiao Tong University, ChinaCopyright © 2022 Lv, Zhu, Li, Deng, Guo, Mao and Zhang. This is an open-access article distributed under the terms of the Creative Commons Attribution License (CC BY). The use, distribution or reproduction in other forums is permitted, provided the original author(s) and the copyright owner(s) are credited and that the original publication in this journal is cited, in accordance with accepted academic practice. No use, distribution or reproduction is permitted which does not comply with these terms.
*Correspondence: Yuefan Zhang, eXVlZmFuemhhbmdAc2h1LmVkdS5jbg==; Junqing Mao, bWFvanEyMDRAMTYzLmNvbQ==
†These authors have contributed equally to this work
Disclaimer: All claims expressed in this article are solely those of the authors and do not necessarily represent those of their affiliated organizations, or those of the publisher, the editors and the reviewers. Any product that may be evaluated in this article or claim that may be made by its manufacturer is not guaranteed or endorsed by the publisher.
Research integrity at Frontiers
Learn more about the work of our research integrity team to safeguard the quality of each article we publish.